diff --git a/.github/workflows/stale.yaml b/.github/workflows/stale.yaml
new file mode 100644
index 00000000000..0ca76b0677e
--- /dev/null
+++ b/.github/workflows/stale.yaml
@@ -0,0 +1,49 @@
+# This workflow warns and then closes issues and PRs that have had no activity for a specified amount of time.
+#
+# You can adjust the behavior by modifying this file.
+# For more information, see:
+# https://github.com/actions/stale
+name: Mark stale issues and pull requests
+
+on:
+ schedule:
+ # Scheduled to run at 1.30 UTC everyday
+ - cron: '30 1 * * *'
+ workflow_dispatch:
+
+jobs:
+ stale:
+
+ runs-on: ubuntu-latest
+ permissions:
+ issues: write
+ pull-requests: write
+
+ steps:
+ - uses: actions/stale@v9
+ with:
+ repo-token: ${{ secrets.GITHUB_TOKEN }}
+ days-before-issue-stale: 14
+ days-before-issue-close: 14
+ stale-issue-label: "status:stale"
+ close-issue-reason: not_planned
+ any-of-labels: "awaiting-contributor-response,cla:no"
+ stale-issue-message: >
+ Marking this issue as stale since it has been open for 14 days with no activity.
+ This issue will be closed if no further activity occurs.
+ close-issue-message: >
+ This issue was closed because it has been inactive for 28 days.
+ Please post a new issue if you need further assistance. Thanks!
+ days-before-pr-stale: 14
+ days-before-pr-close: 14
+ stale-pr-label: "status:stale"
+ stale-pr-message: >
+ Marking this pull request as stale since it has been open for 14 days with no activity.
+ This PR will be closed if no further activity occurs.
+ close-pr-message: >
+ This pull request was closed because it has been inactive for 28 days.
+ Please open a new pull request if you need further assistance. Thanks!
+ # Label that can be assigned to issues to exclude them from being marked as stale
+ exempt-issue-labels: 'override-stale'
+ # Label that can be assigned to PRs to exclude them from being marked as stale
+ exempt-pr-labels: "override-stale"
diff --git a/CONTRIBUTING.md b/CONTRIBUTING.md
index 1559b721f51..6f301eab782 100644
--- a/CONTRIBUTING.md
+++ b/CONTRIBUTING.md
@@ -6,9 +6,7 @@ This guide shows how to make contributions to [tensorflow.org](https://www.tenso
See the
[TensorFlow docs contributor guide](https://www.tensorflow.org/community/contribute/docs)
-for guidance. For questions, the
-[docs@tensorflow.org](https://groups.google.com/a/tensorflow.org/forum/#!forum/docs)
-mailing list is available.
+for guidance. For questions, check out [TensorFlow Forum](https://discuss.tensorflow.org/).
Questions about TensorFlow usage are better addressed on
[Stack Overflow](https://stackoverflow.com/questions/tagged/tensorflow) or the
diff --git a/README.md b/README.md
index 7b94ce5f90f..66b6d3fb065 100644
--- a/README.md
+++ b/README.md
@@ -16,7 +16,7 @@ To file a docs issue, use the issue tracker in the
[tensorflow/tensorflow](https://github.com/tensorflow/tensorflow/issues/new?template=20-documentation-issue.md) repo.
And join the TensorFlow documentation contributors on the
-[docs@tensorflow.org mailing list](https://groups.google.com/a/tensorflow.org/forum/#!forum/docs).
+[TensorFlow Forum](https://discuss.tensorflow.org/).
## Community translations
diff --git a/site/en/community/contribute/docs.md b/site/en/community/contribute/docs.md
index 29b2b5c9550..34b1619ca5d 100644
--- a/site/en/community/contribute/docs.md
+++ b/site/en/community/contribute/docs.md
@@ -32,7 +32,7 @@ To participate in the TensorFlow docs community:
For details, use the [TensorFlow API docs contributor guide](docs_ref.md). This
shows you how to find the
-[source file](https://www.tensorflow.org/code/tensorflow/python/)
+[source file](https://github.com/tensorflow/tensorflow/blob/master/tensorflow/python/)
and edit the symbol's
docstring.
Many API reference pages on tensorflow.org include a link to the source file
@@ -53,9 +53,9 @@ main
tensorflow/tensorflow
repo. The reference documentation is generated from code comments
and docstrings in the source code for
-Python,
-C++, and
-Java.
+Python,
+C++, and
+Java.
Previous versions of the TensorFlow documentation are available as
[rX.x branches](https://github.com/tensorflow/docs/branches) in the TensorFlow
diff --git a/site/en/community/contribute/docs_ref.md b/site/en/community/contribute/docs_ref.md
index fbf207a47f1..41fce4dde40 100644
--- a/site/en/community/contribute/docs_ref.md
+++ b/site/en/community/contribute/docs_ref.md
@@ -8,7 +8,7 @@ TensorFlow uses [DocTest](https://docs.python.org/3/library/doctest.html) to
test code snippets in Python docstrings. The snippet must be executable Python
code. To enable testing, prepend the line with `>>>` (three left-angle
brackets). For example, here's a excerpt from the `tf.concat` function in the
-[array_ops.py](https://www.tensorflow.org/code/tensorflow/python/ops/array_ops.py)
+[array_ops.py](https://github.com/tensorflow/tensorflow/blob/master/tensorflow/python/ops/array_ops.py)
source file:
```
@@ -178,7 +178,7 @@ There are two ways to test the code in the docstring locally:
* If you are only changing the docstring of a class/function/method, then you
can test it by passing that file's path to
- [tf_doctest.py](https://www.tensorflow.org/code/tensorflow/tools/docs/tf_doctest.py).
+ [tf_doctest.py](https://github.com/tensorflow/tensorflow/blob/master/tensorflow/tools/docs/tf_doctest.py).
For example:
diff --git a/site/en/community/contribute/docs_style.md b/site/en/community/contribute/docs_style.md
index eba78afa896..10f18e52699 100644
--- a/site/en/community/contribute/docs_style.md
+++ b/site/en/community/contribute/docs_style.md
@@ -63,10 +63,10 @@ repository like this:
* \[Basics\]\(../../guide/basics.ipynb\) produces
[Basics](../../guide/basics.ipynb).
-This is the prefered approach because this way the links on
+This is the preferred approach because this way the links on
[tensorflow.org](https://www.tensorflow.org),
-[GitHub](https://github.com/tensorflow/docs){:.external} and
-[Colab](https://github.com/tensorflow/docs/tree/master/site/en/guide/bazics.ipynb){:.external}
+[GitHub](https://github.com/tensorflow/docs) and
+[Colab](https://github.com/tensorflow/docs/tree/master/site/en/guide/bazics.ipynb)
all work. Also, the reader stays in the same site when they click a link.
Note: You should include the file extension—such as `.ipynb` or `.md`—for
@@ -83,10 +83,10 @@ To link to source code, use a link starting with
by the file name starting at the GitHub root.
When linking off of [tensorflow.org](https://www.tensorflow.org), include a
-`{:.external}` on the Markdown link so that the "external link" symbol is shown.
+`` on the Markdown link so that the "external link" symbol is shown.
-* `[GitHub](https://github.com/tensorflow/docs){:.external}` produces
- [GitHub](https://github.com/tensorflow/docs){:.external}
+* `[GitHub](https://github.com/tensorflow/docs)` produces
+ [GitHub](https://github.com/tensorflow/docs)
Do not include URI query parameters in the link:
diff --git a/site/en/guide/core/logistic_regression_core.ipynb b/site/en/guide/core/logistic_regression_core.ipynb
index 60e433c9759..5a9af324ad5 100644
--- a/site/en/guide/core/logistic_regression_core.ipynb
+++ b/site/en/guide/core/logistic_regression_core.ipynb
@@ -68,9 +68,9 @@
"id": "DauaqJ7WhIhO"
},
"source": [
- "This guide demonstrates how to use the [TensorFlow Core low-level APIs](https://www.tensorflow.org/guide/core) to perform [binary classification](https://developers.google.com/machine-learning/glossary#binary_classification){:.external} with [logistic regression](https://developers.google.com/machine-learning/crash-course/logistic-regression/){:.external}. It uses the [Wisconsin Breast Cancer Dataset](https://archive.ics.uci.edu/ml/datasets/breast+cancer+wisconsin+(original)){:.external} for tumor classification.\n",
+ "This guide demonstrates how to use the [TensorFlow Core low-level APIs](https://www.tensorflow.org/guide/core) to perform [binary classification](https://developers.google.com/machine-learning/glossary#binary_classification) with [logistic regression](https://developers.google.com/machine-learning/crash-course/logistic-regression/). It uses the [Wisconsin Breast Cancer Dataset](https://archive.ics.uci.edu/ml/datasets/breast+cancer+wisconsin+(original)) for tumor classification.\n",
"\n",
- "[Logistic regression](https://developers.google.com/machine-learning/crash-course/logistic-regression/){:.external} is one of the most popular algorithms for binary classification. Given a set of examples with features, the goal of logistic regression is to output values between 0 and 1, which can be interpreted as the probabilities of each example belonging to a particular class. "
+ "[Logistic regression](https://developers.google.com/machine-learning/crash-course/logistic-regression/) is one of the most popular algorithms for binary classification. Given a set of examples with features, the goal of logistic regression is to output values between 0 and 1, which can be interpreted as the probabilities of each example belonging to a particular class. "
]
},
{
@@ -81,7 +81,7 @@
"source": [
"## Setup\n",
"\n",
- "This tutorial uses [pandas](https://pandas.pydata.org){:.external} for reading a CSV file into a [DataFrame](https://pandas.pydata.org/docs/reference/api/pandas.DataFrame.html){:.external}, [seaborn](https://seaborn.pydata.org){:.external} for plotting a pairwise relationship in a dataset, [Scikit-learn](https://scikit-learn.org/){:.external} for computing a confusion matrix, and [matplotlib](https://matplotlib.org/){:.external} for creating visualizations."
+ "This tutorial uses [pandas](https://pandas.pydata.org) for reading a CSV file into a [DataFrame](https://pandas.pydata.org/docs/reference/api/pandas.DataFrame.html), [seaborn](https://seaborn.pydata.org) for plotting a pairwise relationship in a dataset, [Scikit-learn](https://scikit-learn.org/) for computing a confusion matrix, and [matplotlib](https://matplotlib.org/) for creating visualizations."
]
},
{
@@ -128,7 +128,7 @@
"source": [
"## Load the data\n",
"\n",
- "Next, load the [Wisconsin Breast Cancer Dataset](https://archive.ics.uci.edu/ml/datasets/breast+cancer+wisconsin+(original)){:.external} from the [UCI Machine Learning Repository](https://archive.ics.uci.edu/ml/){:.external}. This dataset contains various features such as a tumor's radius, texture, and concavity."
+ "Next, load the [Wisconsin Breast Cancer Dataset](https://archive.ics.uci.edu/ml/datasets/breast+cancer+wisconsin+(original)) from the [UCI Machine Learning Repository](https://archive.ics.uci.edu/ml/). This dataset contains various features such as a tumor's radius, texture, and concavity."
]
},
{
@@ -156,7 +156,7 @@
"id": "A3VR1aTP92nV"
},
"source": [
- "Read the dataset into a pandas [DataFrame](){:.external} using [`pandas.read_csv`](https://pandas.pydata.org/docs/reference/api/pandas.read_csv.html){:.external}:"
+ "Read the dataset into a pandas [DataFrame]() using [`pandas.read_csv`](https://pandas.pydata.org/docs/reference/api/pandas.read_csv.html):"
]
},
{
@@ -207,7 +207,7 @@
"id": "s4-Wn2jzVC1W"
},
"source": [
- "Split the dataset into training and test sets using [`pandas.DataFrame.sample`](https://pandas.pydata.org/docs/reference/api/pandas.DataFrame.sample.html){:.external}, [`pandas.DataFrame.drop`](https://pandas.pydata.org/docs/reference/api/pandas.DataFrame.drop.html){:.external} and [`pandas.DataFrame.iloc`](https://pandas.pydata.org/docs/reference/api/pandas.DataFrame.iloc.html){:.external}. Make sure to split the features from the target labels. The test set is used to evaluate your model's generalizability to unseen data."
+ "Split the dataset into training and test sets using [`pandas.DataFrame.sample`](https://pandas.pydata.org/docs/reference/api/pandas.DataFrame.sample.html), [`pandas.DataFrame.drop`](https://pandas.pydata.org/docs/reference/api/pandas.DataFrame.drop.html) and [`pandas.DataFrame.iloc`](https://pandas.pydata.org/docs/reference/api/pandas.DataFrame.iloc.html). Make sure to split the features from the target labels. The test set is used to evaluate your model's generalizability to unseen data."
]
},
{
@@ -277,7 +277,7 @@
"\n",
"This dataset contains the mean, standard error, and largest values for each of the 10 tumor measurements collected per example. The `\"diagnosis\"` target column is a categorical variable with `'M'` indicating a malignant tumor and `'B'` indicating a benign tumor diagnosis. This column needs to be converted into a numerical binary format for model training.\n",
"\n",
- "The [`pandas.Series.map`](https://pandas.pydata.org/docs/reference/api/pandas.Series.map.html){:.external} function is useful for mapping binary values to the categories.\n",
+ "The [`pandas.Series.map`](https://pandas.pydata.org/docs/reference/api/pandas.Series.map.html) function is useful for mapping binary values to the categories.\n",
"\n",
"The dataset should also be converted to a tensor with the `tf.convert_to_tensor` function after the preprocessing is complete."
]
@@ -301,7 +301,7 @@
"id": "J4ubs136WLNp"
},
"source": [
- "Use [`seaborn.pairplot`](https://seaborn.pydata.org/generated/seaborn.pairplot.html){:.external} to review the joint distribution of a few pairs of mean-based features from the training set and observe how they relate to the target:"
+ "Use [`seaborn.pairplot`](https://seaborn.pydata.org/generated/seaborn.pairplot.html) to review the joint distribution of a few pairs of mean-based features from the training set and observe how they relate to the target:"
]
},
{
@@ -343,7 +343,7 @@
"id": "_8pDCIFjMla8"
},
"source": [
- "Given the inconsistent ranges, it is beneficial to standardize the data such that each feature has a zero mean and unit variance. This process is called [normalization](https://developers.google.com/machine-learning/glossary#normalization){:.external}."
+ "Given the inconsistent ranges, it is beneficial to standardize the data such that each feature has a zero mean and unit variance. This process is called [normalization](https://developers.google.com/machine-learning/glossary#normalization)."
]
},
{
@@ -384,11 +384,11 @@
"\n",
"### Logistic regression fundamentals\n",
"\n",
- "Linear regression returns a linear combination of its inputs; this output is unbounded. The output of a [logistic regression](https://developers.google.com/machine-learning/glossary#logistic_regression){:.external} is in the `(0, 1)` range. For each example, it represents the probability that the example belongs to the _positive_ class.\n",
+ "Linear regression returns a linear combination of its inputs; this output is unbounded. The output of a [logistic regression](https://developers.google.com/machine-learning/glossary#logistic_regression) is in the `(0, 1)` range. For each example, it represents the probability that the example belongs to the _positive_ class.\n",
"\n",
"Logistic regression maps the continuous outputs of traditional linear regression, `(-∞, ∞)`, to probabilities, `(0, 1)`. This transformation is also symmetric so that flipping the sign of the linear output results in the inverse of the original probability.\n",
"\n",
- "Let $Y$ denote the probability of being in class `1` (the tumor is malignant). The desired mapping can be achieved by interpreting the linear regression output as the [log odds](https://developers.google.com/machine-learning/glossary#log-odds){:.external} ratio of being in class `1` as opposed to class `0`:\n",
+ "Let $Y$ denote the probability of being in class `1` (the tumor is malignant). The desired mapping can be achieved by interpreting the linear regression output as the [log odds](https://developers.google.com/machine-learning/glossary#log-odds) ratio of being in class `1` as opposed to class `0`:\n",
"\n",
"$$\\ln(\\frac{Y}{1-Y}) = wX + b$$\n",
"\n",
@@ -396,7 +396,7 @@
"\n",
"$$Y = \\frac{e^{z}}{1 + e^{z}} = \\frac{1}{1 + e^{-z}}$$\n",
"\n",
- "The expression $\\frac{1}{1 + e^{-z}}$ is known as the [sigmoid function](https://developers.google.com/machine-learning/glossary#sigmoid_function){:.external} $\\sigma(z)$. Hence, the equation for logistic regression can be written as $Y = \\sigma(wX + b)$.\n",
+ "The expression $\\frac{1}{1 + e^{-z}}$ is known as the [sigmoid function](https://developers.google.com/machine-learning/glossary#sigmoid_function) $\\sigma(z)$. Hence, the equation for logistic regression can be written as $Y = \\sigma(wX + b)$.\n",
"\n",
"The dataset in this tutorial deals with a high-dimensional feature matrix. Therefore, the above equation must be rewritten in a matrix vector form as follows:\n",
"\n",
@@ -437,7 +437,7 @@
"source": [
"### The log loss function\n",
"\n",
- "The [log loss](https://developers.google.com/machine-learning/glossary#Log_Loss){:.external}, or binary cross-entropy loss, is the ideal loss function for a binary classification problem with logistic regression. For each example, the log loss quantifies the similarity between a predicted probability and the example's true value. It is determined by the following equation:\n",
+ "The [log loss](https://developers.google.com/machine-learning/glossary#Log_Loss), or binary cross-entropy loss, is the ideal loss function for a binary classification problem with logistic regression. For each example, the log loss quantifies the similarity between a predicted probability and the example's true value. It is determined by the following equation:\n",
"\n",
"$$L = -\\frac{1}{m}\\sum_{i=1}^{m}y_i\\cdot\\log(\\hat{y}_i) + (1- y_i)\\cdot\\log(1 - \\hat{y}_i)$$\n",
"\n",
@@ -471,7 +471,7 @@
"source": [
"### The gradient descent update rule\n",
"\n",
- "The TensorFlow Core APIs support automatic differentiation with `tf.GradientTape`. If you are curious about the mathematics behind the logistic regression [gradient updates](https://developers.google.com/machine-learning/glossary#gradient_descent){:.external}, here is a short explanation:\n",
+ "The TensorFlow Core APIs support automatic differentiation with `tf.GradientTape`. If you are curious about the mathematics behind the logistic regression [gradient updates](https://developers.google.com/machine-learning/glossary#gradient_descent), here is a short explanation:\n",
"\n",
"In the above equation for the log loss, recall that each $\\hat{y}_i$ can be rewritten in terms of the inputs as $\\sigma({\\mathrm{X_i}}w + b)$.\n",
"\n",
@@ -754,7 +754,7 @@
"\n",
"For this problem, the FPR is the proportion of malignant tumor predictions amongst tumors that are actually benign. Conversely, the FNR is the proportion of benign tumor predictions among tumors that are actually malignant.\n",
"\n",
- "Compute a confusion matrix using [`sklearn.metrics.confusion_matrix`](https://scikit-learn.org/stable/modules/generated/sklearn.metrics.confusion_matrix.html#sklearn.metrics.confusion_matrix){:.external}, which evaluates the accuracy of the classification, and use matplotlib to display the matrix:"
+ "Compute a confusion matrix using [`sklearn.metrics.confusion_matrix`](https://scikit-learn.org/stable/modules/generated/sklearn.metrics.confusion_matrix.html#sklearn.metrics.confusion_matrix), which evaluates the accuracy of the classification, and use matplotlib to display the matrix:"
]
},
{
diff --git a/site/en/guide/core/quickstart_core.ipynb b/site/en/guide/core/quickstart_core.ipynb
index 6e7d824f548..70586fd3f0c 100644
--- a/site/en/guide/core/quickstart_core.ipynb
+++ b/site/en/guide/core/quickstart_core.ipynb
@@ -68,13 +68,13 @@
"id": "04QgGZc9bF5D"
},
"source": [
- "This quickstart tutorial demonstrates how you can use the [TensorFlow Core low-level APIs](https://www.tensorflow.org/guide/core) to build and train a multiple linear regression model that predicts fuel efficiency. It uses the [Auto MPG](https://archive.ics.uci.edu/ml/datasets/auto+mpg){:.external} dataset which contains fuel efficiency data for late-1970s and early 1980s automobiles.\n",
+ "This quickstart tutorial demonstrates how you can use the [TensorFlow Core low-level APIs](https://www.tensorflow.org/guide/core) to build and train a multiple linear regression model that predicts fuel efficiency. It uses the [Auto MPG](https://archive.ics.uci.edu/ml/datasets/auto+mpg) dataset which contains fuel efficiency data for late-1970s and early 1980s automobiles.\n",
"\n",
"You will follow the typical stages of a machine learning process:\n",
"\n",
"1. Load the dataset.\n",
"2. Build an [input pipeline](../data.ipynb).\n",
- "3. Build a multiple [linear regression](https://developers.google.com/machine-learning/glossary#linear-regression){:.external} model.\n",
+ "3. Build a multiple [linear regression](https://developers.google.com/machine-learning/glossary#linear-regression) model.\n",
"4. Evaluate the performance of the model."
]
},
@@ -114,7 +114,7 @@
"source": [
"## Load and preprocess the dataset\n",
"\n",
- "Next, you need to load and preprocess the [Auto MPG dataset](https://archive.ics.uci.edu/ml/datasets/auto+mpg){:.external} from the [UCI Machine Learning Repository](https://archive.ics.uci.edu/ml/){:.external}. This dataset uses a variety of quantitative and categorical features such as cylinders, displacement, horsepower and weight to predict the fuel efficiencies of automobiles in the late-1970s and early 1980s.\n",
+ "Next, you need to load and preprocess the [Auto MPG dataset](https://archive.ics.uci.edu/ml/datasets/auto+mpg) from the [UCI Machine Learning Repository](https://archive.ics.uci.edu/ml/). This dataset uses a variety of quantitative and categorical features such as cylinders, displacement, horsepower and weight to predict the fuel efficiencies of automobiles in the late-1970s and early 1980s.\n",
"\n",
"The dataset contains a few unknown values. Make sure to drop any missing values with `pandas.DataFrame.dropna`, and convert the dataset to a `tf.float32` tensor type with the `tf.convert_to_tensor` and `tf.cast` functions."
]
@@ -376,7 +376,7 @@
"source": [
"Next, write a training loop to iteratively update your model's parameters by making use of the MSE loss function and its gradients with respect to the input parameters.\n",
"\n",
- "This iterative method is referred to as [gradient descent](https://developers.google.com/machine-learning/glossary#gradient-descent){:.external}. At each iteration, the model's parameters are updated by taking a step in the opposite direction of their computed gradients. The size of this step is determined by the learning rate, which is a configurable hyperparameter. Recall that the gradient of a function indicates the direction of its steepest ascent; therefore, taking a step in the opposite direction indicates the direction of steepest descent, which ultimately helps to minimize the MSE loss function."
+ "This iterative method is referred to as [gradient descent](https://developers.google.com/machine-learning/glossary#gradient-descent). At each iteration, the model's parameters are updated by taking a step in the opposite direction of their computed gradients. The size of this step is determined by the learning rate, which is a configurable hyperparameter. Recall that the gradient of a function indicates the direction of its steepest ascent; therefore, taking a step in the opposite direction indicates the direction of steepest descent, which ultimately helps to minimize the MSE loss function."
]
},
{
@@ -434,7 +434,7 @@
"id": "4mDAAPFqVVgn"
},
"source": [
- "Plot the changes in MSE loss over time. Calculating performance metrics on a designated [validation set](https://developers.google.com/machine-learning/glossary#validation-set){:.external} or [test set](https://developers.google.com/machine-learning/glossary#test-set){:.external} ensures the model does not overfit to the training dataset and can generalize well to unseen data."
+ "Plot the changes in MSE loss over time. Calculating performance metrics on a designated [validation set](https://developers.google.com/machine-learning/glossary#validation-set) or [test set](https://developers.google.com/machine-learning/glossary#test-set) ensures the model does not overfit to the training dataset and can generalize well to unseen data."
]
},
{
diff --git a/site/en/guide/create_op.md b/site/en/guide/create_op.md
index 3c84204844c..fa4f573fa32 100644
--- a/site/en/guide/create_op.md
+++ b/site/en/guide/create_op.md
@@ -152,17 +152,17 @@ REGISTER_KERNEL_BUILDER(Name("ZeroOut").Device(DEVICE_CPU), ZeroOutOp);
> Important: Instances of your OpKernel may be accessed concurrently.
> Your `Compute` method must be thread-safe. Guard any access to class
> members with a mutex. Or better yet, don't share state via class members!
-> Consider using a [`ResourceMgr`](https://www.tensorflow.org/code/tensorflow/core/framework/resource_mgr.h)
+> Consider using a [`ResourceMgr`](https://github.com/tensorflow/tensorflow/blob/master/tensorflow/core/framework/resource_mgr.h)
> to keep track of op state.
### Multi-threaded CPU kernels
To write a multi-threaded CPU kernel, the Shard function in
-[`work_sharder.h`](https://www.tensorflow.org/code/tensorflow/core/util/work_sharder.h)
+[`work_sharder.h`](https://github.com/tensorflow/tensorflow/blob/master/tensorflow/core/util/work_sharder.h)
can be used. This function shards a computation function across the
threads configured to be used for intra-op threading (see
intra_op_parallelism_threads in
-[`config.proto`](https://www.tensorflow.org/code/tensorflow/core/protobuf/config.proto)).
+[`config.proto`](https://github.com/tensorflow/tensorflow/blob/master/tensorflow/core/protobuf/config.proto)).
### GPU kernels
@@ -519,13 +519,13 @@ This asserts that the input is a vector, and returns having set the
* The `context`, which can either be an `OpKernelContext` or
`OpKernelConstruction` pointer (see
- [`tensorflow/core/framework/op_kernel.h`](https://www.tensorflow.org/code/tensorflow/core/framework/op_kernel.h)),
+ [`tensorflow/core/framework/op_kernel.h`](https://github.com/tensorflow/tensorflow/blob/master/tensorflow/core/framework/op_kernel.h)),
for its `SetStatus()` method.
* The condition. For example, there are functions for validating the shape
of a tensor in
- [`tensorflow/core/framework/tensor_shape.h`](https://www.tensorflow.org/code/tensorflow/core/framework/tensor_shape.h)
+ [`tensorflow/core/framework/tensor_shape.h`](https://github.com/tensorflow/tensorflow/blob/master/tensorflow/core/framework/tensor_shape.h)
* The error itself, which is represented by a `Status` object, see
- [`tensorflow/core/platform/status.h`](https://www.tensorflow.org/code/tensorflow/core/platform/status.h). A
+ [`tensorflow/core/platform/status.h`](https://github.com/tensorflow/tensorflow/blob/master/tensorflow/core/platform/status.h). A
`Status` has both a type (frequently `InvalidArgument`, but see the list of
types) and a message. Functions for constructing an error may be found in
[`tensorflow/core/platform/errors.h`][validation-macros].
@@ -668,7 +668,7 @@ There are shortcuts for common type constraints:
The specific lists of types allowed by these are defined by the functions (like
`NumberTypes()`) in
-[`tensorflow/core/framework/types.h`](https://www.tensorflow.org/code/tensorflow/core/framework/types.h).
+[`tensorflow/core/framework/types.h`](https://github.com/tensorflow/tensorflow/blob/master/tensorflow/core/framework/types.h).
In this example the attr `t` must be one of the numeric types:
```c++
@@ -1226,7 +1226,7 @@ There are several ways to preserve backwards-compatibility.
type into a list of varying types).
The full list of safe and unsafe changes can be found in
-[`tensorflow/core/framework/op_compatibility_test.cc`](https://www.tensorflow.org/code/tensorflow/core/framework/op_compatibility_test.cc).
+[`tensorflow/core/framework/op_compatibility_test.cc`](https://github.com/tensorflow/tensorflow/blob/master/tensorflow/core/framework/op_compatibility_test.cc).
If you cannot make your change to an operation backwards compatible, then create
a new operation with a new name with the new semantics.
@@ -1243,16 +1243,16 @@ made when TensorFlow changes major versions, and must conform to the
You can implement different OpKernels and register one for CPU and another for
GPU, just like you can [register kernels for different types](#polymorphism).
There are several examples of kernels with GPU support in
-[`tensorflow/core/kernels/`](https://www.tensorflow.org/code/tensorflow/core/kernels/).
+[`tensorflow/core/kernels/`](https://github.com/tensorflow/tensorflow/blob/master/tensorflow/core/kernels/).
Notice some kernels have a CPU version in a `.cc` file, a GPU version in a file
ending in `_gpu.cu.cc`, and some code shared in common in a `.h` file.
For example, the `tf.pad` has
everything but the GPU kernel in [`tensorflow/core/kernels/pad_op.cc`][pad_op].
The GPU kernel is in
-[`tensorflow/core/kernels/pad_op_gpu.cu.cc`](https://www.tensorflow.org/code/tensorflow/core/kernels/pad_op_gpu.cu.cc),
+[`tensorflow/core/kernels/pad_op_gpu.cu.cc`](https://github.com/tensorflow/tensorflow/blob/master/tensorflow/core/kernels/pad_op_gpu.cu.cc),
and the shared code is a templated class defined in
-[`tensorflow/core/kernels/pad_op.h`](https://www.tensorflow.org/code/tensorflow/core/kernels/pad_op.h).
+[`tensorflow/core/kernels/pad_op.h`](https://github.com/tensorflow/tensorflow/blob/master/tensorflow/core/kernels/pad_op.h).
We organize the code this way for two reasons: it allows you to share common
code among the CPU and GPU implementations, and it puts the GPU implementation
into a separate file so that it can be compiled only by the GPU compiler.
@@ -1273,16 +1273,16 @@ kept on the CPU, add a `HostMemory()` call to the kernel registration, e.g.:
#### Compiling the kernel for the GPU device
Look at
-[cuda_op_kernel.cu.cc](https://www.tensorflow.org/code/tensorflow/examples/adding_an_op/cuda_op_kernel.cu.cc)
+[cuda_op_kernel.cu.cc](https://github.com/tensorflow/tensorflow/blob/master/tensorflow/examples/adding_an_op/cuda_op_kernel.cu.cc)
for an example that uses a CUDA kernel to implement an op. The
`tf_custom_op_library` accepts a `gpu_srcs` argument in which the list of source
files containing the CUDA kernels (`*.cu.cc` files) can be specified. For use
with a binary installation of TensorFlow, the CUDA kernels have to be compiled
with NVIDIA's `nvcc` compiler. Here is the sequence of commands you can use to
compile the
-[cuda_op_kernel.cu.cc](https://www.tensorflow.org/code/tensorflow/examples/adding_an_op/cuda_op_kernel.cu.cc)
+[cuda_op_kernel.cu.cc](https://github.com/tensorflow/tensorflow/blob/master/tensorflow/examples/adding_an_op/cuda_op_kernel.cu.cc)
and
-[cuda_op_kernel.cc](https://www.tensorflow.org/code/tensorflow/examples/adding_an_op/cuda_op_kernel.cc)
+[cuda_op_kernel.cc](https://github.com/tensorflow/tensorflow/blob/master/tensorflow/examples/adding_an_op/cuda_op_kernel.cc)
into a single dynamically loadable library:
```bash
@@ -1412,7 +1412,7 @@ be set to the first input's shape. If the output is selected by its index as in
There are a number of common shape functions
that apply to many ops, such as `shape_inference::UnchangedShape` which can be
-found in [common_shape_fns.h](https://www.tensorflow.org/code/tensorflow/core/framework/common_shape_fns.h) and used as follows:
+found in [common_shape_fns.h](https://github.com/tensorflow/tensorflow/blob/master/tensorflow/core/framework/common_shape_fns.h) and used as follows:
```c++
REGISTER_OP("ZeroOut")
@@ -1459,7 +1459,7 @@ provides access to the attributes of the op).
Since shape inference is an optional feature, and the shapes of tensors may vary
dynamically, shape functions must be robust to incomplete shape information for
-any of the inputs. The `Merge` method in [`InferenceContext`](https://www.tensorflow.org/code/tensorflow/core/framework/shape_inference.h)
+any of the inputs. The `Merge` method in [`InferenceContext`](https://github.com/tensorflow/tensorflow/blob/master/tensorflow/core/framework/shape_inference.h)
allows the caller to assert that two shapes are the same, even if either
or both of them do not have complete information. Shape functions are defined
for all of the core TensorFlow ops and provide many different usage examples.
@@ -1484,7 +1484,7 @@ If you have a complicated shape function, you should consider adding a test for
validating that various input shape combinations produce the expected output
shape combinations. You can see examples of how to write these tests in some
our
-[core ops tests](https://www.tensorflow.org/code/tensorflow/core/ops/array_ops_test.cc).
+[core ops tests](https://github.com/tensorflow/tensorflow/blob/master/tensorflow/core/ops/array_ops_test.cc).
(The syntax of `INFER_OK` and `INFER_ERROR` are a little cryptic, but try to be
compact in representing input and output shape specifications in tests. For
now, see the surrounding comments in those tests to get a sense of the shape
@@ -1497,20 +1497,20 @@ To build a `pip` package for your op, see the
guide shows how to build custom ops from the TensorFlow pip package instead
of building TensorFlow from source.
-[core-array_ops]:https://www.tensorflow.org/code/tensorflow/core/ops/array_ops.cc
-[python-user_ops]:https://www.tensorflow.org/code/tensorflow/python/user_ops/user_ops.py
-[tf-kernels]:https://www.tensorflow.org/code/tensorflow/core/kernels/
-[user_ops]:https://www.tensorflow.org/code/tensorflow/core/user_ops/
-[pad_op]:https://www.tensorflow.org/code/tensorflow/core/kernels/pad_op.cc
-[standard_ops-py]:https://www.tensorflow.org/code/tensorflow/python/ops/standard_ops.py
-[standard_ops-cc]:https://www.tensorflow.org/code/tensorflow/cc/ops/standard_ops.h
-[python-BUILD]:https://www.tensorflow.org/code/tensorflow/python/BUILD
-[validation-macros]:https://www.tensorflow.org/code/tensorflow/core/platform/errors.h
-[op_def_builder]:https://www.tensorflow.org/code/tensorflow/core/framework/op_def_builder.h
-[register_types]:https://www.tensorflow.org/code/tensorflow/core/framework/register_types.h
-[FinalizeAttr]:https://www.tensorflow.org/code/tensorflow/core/framework/op_def_builder.cc
-[DataTypeString]:https://www.tensorflow.org/code/tensorflow/core/framework/types.cc
-[python-BUILD]:https://www.tensorflow.org/code/tensorflow/python/BUILD
-[types-proto]:https://www.tensorflow.org/code/tensorflow/core/framework/types.proto
-[TensorShapeProto]:https://www.tensorflow.org/code/tensorflow/core/framework/tensor_shape.proto
-[TensorProto]:https://www.tensorflow.org/code/tensorflow/core/framework/tensor.proto
+[core-array_ops]:https://github.com/tensorflow/tensorflow/blob/master/tensorflow/core/ops/array_ops.cc
+[python-user_ops]:https://github.com/tensorflow/tensorflow/blob/master/tensorflow/python/user_ops/user_ops.py
+[tf-kernels]:https://github.com/tensorflow/tensorflow/blob/master/tensorflow/core/kernels/
+[user_ops]:https://github.com/tensorflow/tensorflow/blob/master/tensorflow/core/user_ops/
+[pad_op]:https://github.com/tensorflow/tensorflow/blob/master/tensorflow/core/kernels/pad_op.cc
+[standard_ops-py]:https://github.com/tensorflow/tensorflow/blob/master/tensorflow/python/ops/standard_ops.py
+[standard_ops-cc]:https://github.com/tensorflow/tensorflow/blob/master/tensorflow/cc/ops/standard_ops.h
+[python-BUILD]:https://github.com/tensorflow/tensorflow/blob/master/tensorflow/python/BUILD
+[validation-macros]:https://github.com/tensorflow/tensorflow/blob/master/tensorflow/core/platform/errors.h
+[op_def_builder]:https://github.com/tensorflow/tensorflow/blob/master/tensorflow/core/framework/op_def_builder.h
+[register_types]:https://github.com/tensorflow/tensorflow/blob/master/tensorflow/core/framework/register_types.h
+[FinalizeAttr]:https://github.com/tensorflow/tensorflow/blob/master/tensorflow/core/framework/op_def_builder.cc
+[DataTypeString]:https://github.com/tensorflow/tensorflow/blob/master/tensorflow/core/framework/types.cc
+[python-BUILD]:https://github.com/tensorflow/tensorflow/blob/master/tensorflow/python/BUILD
+[types-proto]:https://github.com/tensorflow/tensorflow/blob/master/tensorflow/core/framework/types.proto
+[TensorShapeProto]:https://github.com/tensorflow/tensorflow/blob/master/tensorflow/core/framework/tensor_shape.proto
+[TensorProto]:https://github.com/tensorflow/tensorflow/blob/master/tensorflow/core/framework/tensor.proto
diff --git a/site/en/guide/data.ipynb b/site/en/guide/data.ipynb
index d9c8fff8982..739ef131005 100644
--- a/site/en/guide/data.ipynb
+++ b/site/en/guide/data.ipynb
@@ -1385,7 +1385,7 @@
"The simplest form of batching stacks `n` consecutive elements of a dataset into\n",
"a single element. The `Dataset.batch()` transformation does exactly this, with\n",
"the same constraints as the `tf.stack()` operator, applied to each component\n",
- "of the elements: i.e. for each component *i*, all elements must have a tensor\n",
+ "of the elements: i.e., for each component *i*, all elements must have a tensor\n",
"of the exact same shape."
]
},
diff --git a/site/en/guide/dtensor_overview.ipynb b/site/en/guide/dtensor_overview.ipynb
index 95a50f3465f..1b55ee0283f 100644
--- a/site/en/guide/dtensor_overview.ipynb
+++ b/site/en/guide/dtensor_overview.ipynb
@@ -281,7 +281,7 @@
"id": "Eyp_qOSyvieo"
},
"source": [
- "\n"
+ "\n"
]
},
{
@@ -303,7 +303,7 @@
"source": [
"For the same `mesh_2d`, the layout `Layout([\"x\", dtensor.UNSHARDED], mesh_2d)` is a layout for a rank-2 `Tensor` that is replicated across `\"y\"`, and whose first axis is sharded on mesh dimension `x`.\n",
"\n",
- "\n"
+ "\n"
]
},
{
diff --git a/site/en/guide/estimator.ipynb b/site/en/guide/estimator.ipynb
index e58ef46cf86..05e8fb4012a 100644
--- a/site/en/guide/estimator.ipynb
+++ b/site/en/guide/estimator.ipynb
@@ -68,7 +68,7 @@
"id": "rILQuAiiRlI7"
},
"source": [
- "> Warning: Estimators are not recommended for new code. Estimators run `v1.Session`-style code which is more difficult to write correctly, and can behave unexpectedly, especially when combined with TF 2 code. Estimators do fall under our [compatibility guarantees](https://tensorflow.org/guide/versions), but will receive no fixes other than security vulnerabilities. See the [migration guide](https://tensorflow.org/guide/migrate) for details."
+ "> Warning: TensorFlow 2.15 included the final release of the `tf-estimator` package. Estimators will not be available in TensorFlow 2.16 or after. See the [migration guide](https://www.tensorflow.org/guide/migrate/migrating_estimator) for more information about how to convert off of Estimators."
]
},
{
diff --git a/site/en/guide/extension_type.ipynb b/site/en/guide/extension_type.ipynb
index 76e20e8d283..7e8edeea7c9 100644
--- a/site/en/guide/extension_type.ipynb
+++ b/site/en/guide/extension_type.ipynb
@@ -1822,13 +1822,17 @@
" transpose_a=False, transpose_b=False,\n",
" adjoint_a=False, adjoint_b=False,\n",
" a_is_sparse=False, b_is_sparse=False,\n",
- " output_type=None):\n",
+ " output_type=None,\n",
+ " grad_a=False, grad_b=False,\n",
+ " name=None,\n",
+ " ):\n",
" if isinstance(a, MaskedTensor):\n",
" a = a.with_default(0)\n",
" if isinstance(b, MaskedTensor):\n",
" b = b.with_default(0)\n",
" return tf.matmul(a, b, transpose_a, transpose_b, adjoint_a,\n",
- " adjoint_b, a_is_sparse, b_is_sparse, output_type)"
+ " adjoint_b, a_is_sparse, b_is_sparse,\n",
+ " output_type)"
]
},
{
diff --git a/site/en/guide/function.ipynb b/site/en/guide/function.ipynb
index 9f3d93db057..f4677f21eb8 100644
--- a/site/en/guide/function.ipynb
+++ b/site/en/guide/function.ipynb
@@ -146,7 +146,7 @@
"source": [
"### Usage\n",
"\n",
- "A `Function` you define (for example by applying the `@tf.function` decorator) is just like a core TensorFlow operation: You can execute it eagerly; you can compute gradients; and so on."
+ "A `tf.function` that you define (for example by applying the `@tf.function` decorator) is just like a core TensorFlow operation: You can execute it eagerly; you can compute gradients; and so on."
]
},
{
@@ -157,7 +157,7 @@
},
"outputs": [],
"source": [
- "@tf.function # The decorator converts `add` into a `Function`.\n",
+ "@tf.function # The decorator converts `add` into a `PolymorphicFunction`.\n",
"def add(a, b):\n",
" return a + b\n",
"\n",
@@ -184,7 +184,7 @@
"id": "ocWZvqrmHnmX"
},
"source": [
- "You can use `Function`s inside other `Function`s."
+ "You can use `tf.function`s inside other `tf.function`s."
]
},
{
@@ -208,7 +208,7 @@
"id": "piBhz7gYsHqU"
},
"source": [
- "`Function`s can be faster than eager code, especially for graphs with many small ops. But for graphs with a few expensive ops (like convolutions), you may not see much speedup.\n"
+ "`tf.function`s can be faster than eager code, especially for graphs with many small ops. But for graphs with a few expensive ops (like convolutions), you may not see much speedup.\n"
]
},
{
@@ -242,7 +242,7 @@
"source": [
"### Tracing\n",
"\n",
- "This section exposes how `Function` works under the hood, including implementation details *which may change in the future*. However, once you understand why and when tracing happens, it's much easier to use `tf.function` effectively!"
+ "This section exposes how `tf.function` works under the hood, including implementation details *which may change in the future*. However, once you understand why and when tracing happens, it's much easier to use `tf.function` effectively!"
]
},
{
@@ -253,17 +253,17 @@
"source": [
"#### What is \"tracing\"?\n",
"\n",
- "A `Function` runs your program in a [TensorFlow Graph](https://www.tensorflow.org/guide/intro_to_graphs#what_are_graphs). However, a `tf.Graph` cannot represent all the things that you'd write in an eager TensorFlow program. For instance, Python supports polymorphism, but `tf.Graph` requires its inputs to have a specified data type and dimension. Or you may perform side tasks like reading command-line arguments, raising an error, or working with a more complex Python object; none of these things can run in a `tf.Graph`.\n",
+ "A `tf.function` runs your program in a [TensorFlow Graph](https://www.tensorflow.org/guide/intro_to_graphs#what_are_graphs). However, a `tf.Graph` cannot represent all the things that you'd write in an eager TensorFlow program. For instance, Python supports polymorphism, but `tf.Graph` requires its inputs to have a specified data type and dimension. Or you may perform side tasks like reading command-line arguments, raising an error, or working with a more complex Python object; none of these things can run in a `tf.Graph`.\n",
"\n",
- "`Function` bridges this gap by separating your code in two stages:\n",
+ "`tf.function` bridges this gap by separating your code in two stages:\n",
"\n",
- " 1) In the first stage, referred to as \"**tracing**\", `Function` creates a new `tf.Graph`. Python code runs normally, but all TensorFlow operations (like adding two Tensors) are *deferred*: they are captured by the `tf.Graph` and not run.\n",
+ " 1) In the first stage, referred to as \"**tracing**\", `tf.function` creates a new `tf.Graph`. Python code runs normally, but all TensorFlow operations (like adding two Tensors) are *deferred*: they are captured by the `tf.Graph` and not run.\n",
"\n",
" 2) In the second stage, a `tf.Graph` which contains everything that was deferred in the first stage is run. This stage is much faster than the tracing stage.\n",
"\n",
- "Depending on its inputs, `Function` will not always run the first stage when it is called. See [\"Rules of tracing\"](#rules_of_tracing) below to get a better sense of how it makes that determination. Skipping the first stage and only executing the second stage is what gives you TensorFlow's high performance.\n",
+ "Depending on its inputs, `tf.function` will not always run the first stage when it is called. See [\"Rules of tracing\"](#rules_of_tracing) below to get a better sense of how it makes that determination. Skipping the first stage and only executing the second stage is what gives you TensorFlow's high performance.\n",
"\n",
- "When `Function` does decide to trace, the tracing stage is immediately followed by the second stage, so calling the `Function` both creates and runs the `tf.Graph`. Later you will see how you can run only the tracing stage with [`get_concrete_function`](#obtaining_concrete_functions)."
+ "When `tf.function` does decide to trace, the tracing stage is immediately followed by the second stage, so calling the `tf.function` both creates and runs the `tf.Graph`. Later you will see how you can run only the tracing stage with [`get_concrete_function`](#obtaining_concrete_functions)."
]
},
{
@@ -272,7 +272,7 @@
"id": "K7scSzLx662f"
},
"source": [
- "When you pass arguments of different types into a `Function`, both stages are run:\n"
+ "When you pass arguments of different types into a `tf.function`, both stages are run:\n"
]
},
{
@@ -302,7 +302,7 @@
"id": "QPfouGUQrcNb"
},
"source": [
- "Note that if you repeatedly call a `Function` with the same argument type, TensorFlow will skip the tracing stage and reuse a previously traced graph, as the generated graph would be identical."
+ "Note that if you repeatedly call a `tf.function` with the same argument type, TensorFlow will skip the tracing stage and reuse a previously traced graph, as the generated graph would be identical."
]
},
{
@@ -346,10 +346,11 @@
"So far, you've seen that `tf.function` creates a cached, dynamic dispatch layer over TensorFlow's graph tracing logic. To be more specific about the terminology:\n",
"\n",
"- A `tf.Graph` is the raw, language-agnostic, portable representation of a TensorFlow computation.\n",
- "- A `ConcreteFunction` wraps a `tf.Graph`.\n",
- "- A `Function` manages a cache of `ConcreteFunction`s and picks the right one for your inputs.\n",
- "- `tf.function` wraps a Python function, returning a `Function` object.\n",
- "- **Tracing** creates a `tf.Graph` and wraps it in a `ConcreteFunction`, also known as a **trace.**\n"
+ "- Tracing is the process through which new `tf.Graph`s are generated from Python code.\n",
+ "- An instance of `tf.Graph` is specialized to the specific input types it was traced with. Differing types require retracing.\n",
+ "- Each traced `tf.Graph` has a corresponding `ConcreteFunction`.\n",
+ "- A `tf.function` manages a cache of `ConcreteFunction`s and picks the right one for your inputs.\n",
+ "- `tf.function` wraps the Python function that will be traced, returning a `tf.types.experimental.PolymorphicFunction` object.\n"
]
},
{
@@ -360,7 +361,7 @@
"source": [
"#### Rules of tracing\n",
"\n",
- "When called, a `Function` matches the call arguments to existing `ConcreteFunction`s using `tf.types.experimental.TraceType` of each argument. If a matching `ConcreteFunction` is found, the call is dispatched to it. If no match is found, a new `ConcreteFunction` is traced.\n",
+ "When called, a `tf.function` first evaluates the type of each input argument using the `tf.types.experimental.TraceType` of each argument. This is used to construct a `tf.types.experimental.FunctionType` describing the signature of the desired `ConcreteFunction`. We compare this `FunctionType` to the `FunctionType`s of existing `ConcreteFunction`s. If a matching `ConcreteFunction` is found, the call is dispatched to it. If no match is found, a new `ConcreteFunction` is traced for the desired `FunctionType`.\n",
"\n",
"If multiple matches are found, the most specific signature is chosen. Matching is done by [subtyping](https://en.wikipedia.org/wiki/Subtyping), much like normal function calls in C++ or Java, for instance. For example, `TensorShape([1, 2])` is a subtype of `TensorShape([None, None])` and so a call to the tf.function with `TensorShape([1, 2])` can be dispatched to the `ConcreteFunction` produced with `TensorShape([None, None])` but if a `ConcreteFunction` with `TensorShape([1, None])` also exists then it will be prioritized since it is more specific.\n",
"\n",
@@ -369,13 +370,13 @@
"* For `Variable`, the type is similar to `Tensor`, but also includes a unique resource ID of the variable, necessary to correctly wire control dependencies\n",
"* For Python primitive values, the type corresponds to the **value** itself. For example, the `TraceType` of the value `3` is `LiteralTraceType<3>`, not `int`.\n",
"* For Python ordered containers such as `list` and `tuple`, etc., the type is parameterized by the types of their elements; for example, the type of `[1, 2]` is `ListTraceType, LiteralTraceType<2>>` and the type for `[2, 1]` is `ListTraceType, LiteralTraceType<1>>` which is different.\n",
- "* For Python mappings such as `dict`, the type is also a mapping from the same keys but to the types of values instead the actual values. For example, the type of `{1: 2, 3: 4}`, is `MappingTraceType<>>, >>>`. However, unlike ordered containers, `{1: 2, 3: 4}` and `{3: 4, 1: 2}` have equivalent types.\n",
- "* For Python objects which implement the `__tf_tracing_type__` method, the type is whatever that method returns\n",
- "* For any other Python objects, the type is a generic `TraceType`, its matching precedure is:\n",
- " * First it checks if the object is the same object used in the previous trace (using python `id()` or `is`). Note that this will still match if the object has changed, so if you use python objects as `tf.function` arguments it's best to use *immutable* ones.\n",
- " * Next it checks if the object is equal to the object used in the previous trace (using python `==`).\n",
+ "* For Python mappings such as `dict`, the type is also a mapping from the same keys but to the types of values instead of the actual values. For example, the type of `{1: 2, 3: 4}`, is `MappingTraceType<>>, >>>`. However, unlike ordered containers, `{1: 2, 3: 4}` and `{3: 4, 1: 2}` have equivalent types.\n",
+ "* For Python objects which implement the `__tf_tracing_type__` method, the type is whatever that method returns.\n",
+ "* For any other Python objects, the type is a generic `TraceType`, and the matching precedure is:\n",
+ " * First it checks if the object is the same object used in the previous trace (using Python `id()` or `is`). Note that this will still match if the object has changed, so if you use Python objects as `tf.function` arguments it's best to use *immutable* ones.\n",
+ " * Next it checks if the object is equal to the object used in the previous trace (using Python `==`).\n",
" \n",
- " Note that this procedure only keeps a [weakref](https://docs.python.org/3/library/weakref.html) to the object and hence only works as long as the object is in scope/not deleted.)\n"
+ " Note that this procedure only keeps a [weakref](https://docs.python.org/3/library/weakref.html) to the object and hence only works as long as the object is in scope/not deleted.\n"
]
},
{
@@ -384,7 +385,7 @@
"id": "GNNN4lgRzpIs"
},
"source": [
- "Note: `TraceType` is based on the `Function` input parameters so changes to global and [free variables](https://docs.python.org/3/reference/executionmodel.html#binding-of-names) alone will not create a new trace. See [this section](#depending_on_python_global_and_free_variables) for recommended practices when dealing with Python global and free variables."
+ "Note: `TraceType` is based on the `tf.function` input parameters so changes to global and [free variables](https://docs.python.org/3/reference/executionmodel.html#binding-of-names) alone will not create a new trace. See [this section](#depending_on_python_global_and_free_variables) for recommended practices when dealing with Python global and free variables."
]
},
{
@@ -395,7 +396,7 @@
"source": [
"### Controlling retracing\n",
"\n",
- "Retracing, which is when your `Function` creates more than one trace, helps ensure that TensorFlow generates correct graphs for each set of inputs. However, tracing is an expensive operation! If your `Function` retraces a new graph for every call, you'll find that your code executes more slowly than if you didn't use `tf.function`.\n",
+ "Retracing, which is when your `tf.function` creates more than one trace, helps ensure that TensorFlow generates correct graphs for each set of inputs. However, tracing is an expensive operation! If your `tf.function` retraces a new graph for every call, you'll find that your code executes more slowly than if you didn't use `tf.function`.\n",
"\n",
"To control the tracing behavior, you can use the following techniques:"
]
@@ -406,7 +407,9 @@
"id": "EUtycWJa34TT"
},
"source": [
- "#### Pass a fixed `input_signature` to `tf.function`"
+ "#### Pass a fixed `input_signature` to `tf.function`\n",
+ "\n",
+ "This forces `tf.function` to constrain itself to only one `tf.types.experimental.FunctionType` composed of the types enumerated by the `input_signature`. Calls that cannot be dispatched to this `FunctionType` will throw an error."
]
},
{
@@ -440,7 +443,7 @@
"source": [
"#### Use unknown dimensions for flexibility\n",
"\n",
- " Since TensorFlow matches tensors based on their shape, using a `None` dimension as a wildcard will allow `Function`s to reuse traces for variably-sized input. Variably-sized input can occur if you have sequences of different length, or images of different sizes for each batch. You can check out the [Transformer](https://www.tensorflow.org/text/tutorials/transformer) and [Deep Dream](../tutorials/generative/deepdream.ipynb) tutorials for examples."
+ " Since TensorFlow matches tensors based on their shape, using a `None` dimension as a wildcard will allow `tf.function`s to reuse traces for variably-sized input. Variably-sized input can occur if you have sequences of different length, or images of different sizes for each batch. You can check out the [Transformer](https://www.tensorflow.org/text/tutorials/transformer) and [Deep Dream](../tutorials/generative/deepdream.ipynb) tutorials for examples."
]
},
{
@@ -461,6 +464,41 @@
"print(g(tf.constant([1, 2, 3, 4, 5])))\n"
]
},
+ {
+ "cell_type": "markdown",
+ "metadata": {
+ "id": "37cc12f93cbd"
+ },
+ "source": [
+ "#### Use `reduce_retracing` for automatic flexibility\n",
+ "\n",
+ "When `reduce_retracing` is enabled, `tf.function` automatically identifies supertypes of the input types it is observing and chooses to trace more generalized graphs automatically. It is less efficient than setting the `input_signature` directly but useful when many types need to be supported."
+ ]
+ },
+ {
+ "cell_type": "code",
+ "execution_count": null,
+ "metadata": {
+ "id": "0403fae03a1f"
+ },
+ "outputs": [],
+ "source": [
+ "@tf.function(reduce_retracing=True)\n",
+ "def g(x):\n",
+ " print('Tracing with', x)\n",
+ " return x\n",
+ "\n",
+ "# Traces once.\n",
+ "print(g(tf.constant([1, 2, 3])))\n",
+ "\n",
+ "# Traces again, but more generalized this time.\n",
+ "print(g(tf.constant([1, 2, 3, 4, 5])))\n",
+ "\n",
+ "# No more tracing!\n",
+ "print(g(tf.constant([1, 2, 3, 4, 5, 6, 7])))\n",
+ "print(g(tf.constant([1, 2, 3, 4, 5, 6, 7, 8, 9])))"
+ ]
+ },
{
"cell_type": "markdown",
"metadata": {
@@ -508,7 +546,7 @@
"id": "4pJqkDR_Q2wz"
},
"source": [
- "If you need to force retracing, create a new `Function`. Separate `Function` objects are guaranteed not to share traces."
+ "If you need to force retracing, create a new `tf.function`. Separate `tf.function` objects are guaranteed not to share traces."
]
},
{
@@ -537,7 +575,7 @@
"\n",
"Where possible, you should prefer converting the Python type into a `tf.experimental.ExtensionType` instead. Moreover, the `TraceType` of an `ExtensionType` is the `tf.TypeSpec` associated with it. Therefore, if needed, you can simply override the default `tf.TypeSpec` to take control of an `ExtensionType`'s `Tracing Protocol`. Refer to the _Customizing the ExtensionType's TypeSpec_ section in the [Extension types](extension_type.ipynb) guide for details.\n",
"\n",
- "Otherwise, for direct control over when `Function` should retrace in regards to a particular Python type, you can implement the `Tracing Protocol` for it yourself."
+ "Otherwise, for direct control over when `tf.function` should retrace in regards to a particular Python type, you can implement the `Tracing Protocol` for it yourself."
]
},
{
@@ -689,8 +727,7 @@
},
"outputs": [],
"source": [
- "print(double_strings.structured_input_signature)\n",
- "print(double_strings.structured_outputs)"
+ "print(double_strings.function_type)"
]
},
{
@@ -761,7 +798,7 @@
"source": [
"### Obtaining graphs\n",
"\n",
- "Each concrete function is a callable wrapper around a `tf.Graph`. Although retrieving the actual `tf.Graph` object is not something you'll normally need to do, you can obtain it easily from any concrete function."
+ "Although retrieving the actual `tf.Graph` object is not something you'll normally need to do, you can obtain it easily from any concrete function."
]
},
{
@@ -777,6 +814,36 @@
" print(f'{node.input} -> {node.name}')\n"
]
},
+ {
+ "cell_type": "markdown",
+ "metadata": {
+ "id": "2d49c486ccd4"
+ },
+ "source": [
+ "In reality, `tf.Graph`s are not directly callable. We actually use an `tf.types.experimental.AtomicFunction` to perform the computations described by the `tf.Graph`. You can access the `AtomicFunction` describing the traced `tf.Graph` and call it directly instead of the `ConcreteFunction`:"
+ ]
+ },
+ {
+ "cell_type": "code",
+ "execution_count": null,
+ "metadata": {
+ "id": "4c3879aa0be0"
+ },
+ "outputs": [],
+ "source": [
+ "atomic_fn = double_strings.inference_fn\n",
+ "atomic_fn(tf.constant(\"a\"))"
+ ]
+ },
+ {
+ "cell_type": "markdown",
+ "metadata": {
+ "id": "c3bd1036c18c"
+ },
+ "source": [
+ "This has the advantage of having lower Python overhead for high-performance scenarios. But it should only be used for forward inference (no gradient support), and captured tensor values (if any) would need to be explicitly supplied."
+ ]
+ },
{
"cell_type": "markdown",
"metadata": {
@@ -833,7 +900,7 @@
"id": "KxwJ8znPI0Cg"
},
"source": [
- "If you're curious you can inspect the code autograph generates."
+ "If you're curious you can inspect the code AutoGraph generates."
]
},
{
@@ -1029,7 +1096,7 @@
"source": [
"## Limitations\n",
"\n",
- "TensorFlow `Function` has a few limitations by design that you should be aware of when converting a Python function to a `Function`."
+ "`tf.function` has a few limitations by design that you should be aware of when converting a Python function to a `tf.function`."
]
},
{
@@ -1040,7 +1107,7 @@
"source": [
"### Executing Python side effects\n",
"\n",
- "Side effects, like printing, appending to lists, and mutating globals, can behave unexpectedly inside a `Function`, sometimes executing twice or not all. They only happen the first time you call a `Function` with a set of inputs. Afterwards, the traced `tf.Graph` is reexecuted, without executing the Python code.\n",
+ "Side effects, like printing, appending to lists, and mutating globals, can behave unexpectedly inside a `tf.function`, sometimes executing twice or not all. They only happen the first time you call a `tf.function` with a set of inputs. Afterwards, the traced `tf.Graph` is reexecuted, without executing the Python code.\n",
"\n",
"The general rule of thumb is to avoid relying on Python side effects in your logic and only use them to debug your traces. Otherwise, TensorFlow APIs like `tf.data`, `tf.print`, `tf.summary`, `tf.Variable.assign`, and `tf.TensorArray` are the best way to ensure your code will be executed by the TensorFlow runtime with each call."
]
@@ -1069,7 +1136,7 @@
"id": "e1I0dPiqTV8H"
},
"source": [
- "If you would like to execute Python code during each invocation of a `Function`, `tf. py_function` is an exit hatch. The drawbacks of `tf.py_function` are that it's not portable or particularly performant, cannot be saved with SavedModel, and does not work well in distributed (multi-GPU, TPU) setups. Also, since `tf.py_function` has to be wired into the graph, it casts all inputs/outputs to tensors."
+ "If you would like to execute Python code during each invocation of a `tf.function`, `tf. py_function` is an exit hatch. The drawbacks of `tf.py_function` are that it's not portable or particularly performant, cannot be saved with `SavedModel`, and does not work well in distributed (multi-GPU, TPU) setups. Also, since `tf.py_function` has to be wired into the graph, it casts all inputs/outputs to tensors."
]
},
{
@@ -1170,7 +1237,7 @@
"id": "5eZTFRv_k_nR"
},
"source": [
- "Sometimes unexpected behaviors are very hard to notice. In the example below, the `counter` is intended to safeguard the increment of a variable. However because it is a python integer and not a TensorFlow object, it's value is captured during the first trace. When the `tf.function` is used, the `assign_add` will be recorded unconditionally in the underlying graph. Therefore `v` will increase by 1, every time the `tf.function` is called. This issue is common among users that try to migrate their Grpah-mode Tensorflow code to Tensorflow 2 using `tf.function` decorators, when python side-effects (the `counter` in the example) are used to determine what ops to run (`assign_add` in the example). Usually, users realize this only after seeing suspicious numerical results, or significantly lower performance than expected (e.g. if the guarded operation is very costly)."
+ "Sometimes unexpected behaviors are very hard to notice. In the example below, the `counter` is intended to safeguard the increment of a variable. However because it is a python integer and not a TensorFlow object, it's value is captured during the first trace. When the `tf.function` is used, the `assign_add` will be recorded unconditionally in the underlying graph. Therefore `v` will increase by 1, every time the `tf.function` is called. This issue is common among users that try to migrate their Graph-mode Tensorflow code to Tensorflow 2 using `tf.function` decorators, when python side-effects (the `counter` in the example) are used to determine what ops to run (`assign_add` in the example). Usually, users realize this only after seeing suspicious numerical results, or significantly lower performance than expected (e.g. if the guarded operation is very costly)."
]
},
{
@@ -1243,7 +1310,7 @@
"id": "pbFG5CX4LwQA"
},
"source": [
- "In summary, as a rule of thumb, you should avoid mutating python objects such as integers or containers like lists that live outside the `Function`. Instead, use arguments and TF objects. For example, the section [\"Accumulating values in a loop\"](#accumulating_values_in_a_loop) has one example of how list-like operations can be implemented.\n",
+ "In summary, as a rule of thumb, you should avoid mutating python objects such as integers or containers like lists that live outside the `tf.function`. Instead, use arguments and TF objects. For example, the section [\"Accumulating values in a loop\"](#accumulating_values_in_a_loop) has one example of how list-like operations can be implemented.\n",
"\n",
"You can, in some cases, capture and manipulate state if it is a [`tf.Variable`](https://www.tensorflow.org/guide/variable). This is how the weights of Keras models are updated with repeated calls to the same `ConcreteFunction`."
]
@@ -1437,7 +1504,7 @@
"source": [
"### Recursive tf.functions are not supported\n",
"\n",
- "Recursive `Function`s are not supported and could cause infinite loops. For example,"
+ "Recursive `tf.function`s are not supported and could cause infinite loops. For example,"
]
},
{
@@ -1465,7 +1532,7 @@
"id": "LyRyooKGUxNV"
},
"source": [
- "Even if a recursive `Function` seems to work, the python function will be traced multiple times and could have performance implication. For example,"
+ "Even if a recursive `tf.function` seems to work, the Python function will be traced multiple times and could have performance implications. For example,"
]
},
{
@@ -1495,7 +1562,7 @@
"source": [
"## Known Issues\n",
"\n",
- "If your `Function` is not evaluating correctly, the error may be explained by these known issues which are planned to be fixed in the future."
+ "If your `tf.function` is not evaluating correctly, the error may be explained by these known issues which are planned to be fixed in the future."
]
},
{
@@ -1506,7 +1573,7 @@
"source": [
"### Depending on Python global and free variables\n",
"\n",
- "`Function` creates a new `ConcreteFunction` when called with a new value of a Python argument. However, it does not do that for the Python closure, globals, or nonlocals of that `Function`. If their value changes in between calls to the `Function`, the `Function` will still use the values they had when it was traced. This is different from how regular Python functions work.\n",
+ "`tf.function` creates a new `ConcreteFunction` when called with a new value of a Python argument. However, it does not do that for the Python closure, globals, or nonlocals of that `tf.function`. If their value changes in between calls to the `tf.function`, the `tf.function` will still use the values they had when it was traced. This is different from how regular Python functions work.\n",
"\n",
"For that reason, you should follow a functional programming style that uses arguments instead of closing over outer names."
]
@@ -1552,7 +1619,7 @@
"id": "ZoPg5w1Pjqnb"
},
"source": [
- "Another way to update a global value, is to make it a `tf.Variable` and use the `Variable.assign` method instead.\n"
+ "Another way to update a global value is to make it a `tf.Variable` and use the `Variable.assign` method instead.\n"
]
},
{
@@ -1648,11 +1715,11 @@
"id": "Ytcgg2qFWaBF"
},
"source": [
- "Using the same `Function` to evaluate the modified instance of the model will be buggy since it still has the [same instance-based TraceType](#rules_of_tracing) as the original model.\n",
+ "Using the same `tf.function` to evaluate the modified instance of the model will be buggy since it still has the [same instance-based TraceType](#rules_of_tracing) as the original model.\n",
"\n",
- "For that reason, you're recommended to write your `Function` to avoid depending on mutable object attributes or implement the [Tracing Protocol](#use_the_tracing_protocol) for the objects to inform `Function` about such attributes.\n",
+ "For that reason, you're recommended to write your `tf.function` to avoid depending on mutable object attributes or implement the [Tracing Protocol](#use_the_tracing_protocol) for the objects to inform `tf.function` about such attributes.\n",
"\n",
- "If that is not possible, one workaround is to make new `Function`s each time you modify your object to force retracing:"
+ "If that is not possible, one workaround is to make new `tf.function`s each time you modify your object to force retracing:"
]
},
{
@@ -1668,7 +1735,7 @@
"\n",
"new_model = SimpleModel()\n",
"evaluate_no_bias = tf.function(evaluate).get_concrete_function(new_model, x)\n",
- "# Don't pass in `new_model`, `Function` already captured its state during tracing.\n",
+ "# Don't pass in `new_model`. `tf.function` already captured its state during tracing.\n",
"print(evaluate_no_bias(x))"
]
},
@@ -1682,7 +1749,7 @@
"source": [
"print(\"Adding bias!\")\n",
"new_model.bias += 5.0\n",
- "# Create new Function and ConcreteFunction since you modified new_model.\n",
+ "# Create new `tf.function` and `ConcreteFunction` since you modified `new_model`.\n",
"evaluate_with_bias = tf.function(evaluate).get_concrete_function(new_model, x)\n",
"print(evaluate_with_bias(x)) # Don't pass in `new_model`."
]
@@ -1739,7 +1806,7 @@
"source": [
"### Creating tf.Variables\n",
"\n",
- "`Function` only supports singleton `tf.Variable`s created once on the first call, and reused across subsequent function calls. The code snippet below would create a new `tf.Variable` in every function call, which results in a `ValueError` exception.\n",
+ "`tf.function` only supports singleton `tf.Variable`s created once on the first call, and reused across subsequent function calls. The code snippet below would create a new `tf.Variable` in every function call, which results in a `ValueError` exception.\n",
"\n",
"Example:"
]
@@ -1800,7 +1867,7 @@
},
"source": [
"#### Using with multiple Keras optimizers\n",
- "You may encounter `ValueError: tf.function only supports singleton tf.Variables created on the first call.` when using more than one Keras optimizer with a `tf.function`. This error occurs because optimizers internally create `tf.Variables` when they apply gradients for the first time."
+ "You may encounter `ValueError: tf.function only supports singleton tf.Variables created on the first call.` when using more than one Keras optimizer with a `tf.function`. This error occurs because optimizers internally create `tf.Variable`s when they apply gradients for the first time."
]
},
{
@@ -1901,7 +1968,7 @@
"x = tf.constant([-1.])\n",
"y = tf.constant([2.])\n",
"\n",
- "# Make a new Function and ConcreteFunction for each optimizer.\n",
+ "# Make a new tf.function and ConcreteFunction for each optimizer.\n",
"train_step_1 = tf.function(train_step)\n",
"train_step_2 = tf.function(train_step)\n",
"for i in range(10):\n",
@@ -1919,9 +1986,9 @@
"source": [
"#### Using with multiple Keras models\n",
"\n",
- "You may also encounter `ValueError: tf.function only supports singleton tf.Variables created on the first call.` when passing different model instances to the same `Function`.\n",
+ "You may also encounter `ValueError: tf.function only supports singleton tf.Variables created on the first call.` when passing different model instances to the same `tf.function`.\n",
"\n",
- "This error occurs because Keras models (which [do not have their input shape defined](https://www.tensorflow.org/guide/keras/custom_layers_and_models#best_practice_deferring_weight_creation_until_the_shape_of_the_inputs_is_known)) and Keras layers create `tf.Variables`s when they are first called. You may be attempting to initialize those variables inside a `Function`, which has already been called. To avoid this error, try calling `model.build(input_shape)` to initialize all the weights before training the model.\n"
+ "This error occurs because Keras models (which [do not have their input shape defined](https://www.tensorflow.org/guide/keras/custom_layers_and_models#best_practice_deferring_weight_creation_until_the_shape_of_the_inputs_is_known)) and Keras layers create `tf.Variable`s when they are first called. You may be attempting to initialize those variables inside a `tf.function`, which has already been called. To avoid this error, try calling `model.build(input_shape)` to initialize all the weights before training the model.\n"
]
},
{
@@ -1932,7 +1999,7 @@
"source": [
"## Further reading\n",
"\n",
- "To learn about how to export and load a `Function`, see the [SavedModel guide](../../guide/saved_model). To learn more about graph optimizations that are performed after tracing, see the [Grappler guide](../../guide/graph_optimization). To learn how to optimize your data pipeline and profile your model, see the [Profiler guide](../../guide/profiler.md)."
+ "To learn about how to export and load a `tf.function`, see the [SavedModel guide](../../guide/saved_model). To learn more about graph optimizations that are performed after tracing, see the [Grappler guide](../../guide/graph_optimization). To learn how to optimize your data pipeline and profile your model, see the [Profiler guide](../../guide/profiler.md)."
]
}
],
diff --git a/site/en/guide/intro_to_graphs.ipynb b/site/en/guide/intro_to_graphs.ipynb
index 0392a160d55..4fe442632ba 100644
--- a/site/en/guide/intro_to_graphs.ipynb
+++ b/site/en/guide/intro_to_graphs.ipynb
@@ -87,7 +87,7 @@
"source": [
"### What are graphs?\n",
"\n",
- "In the previous three guides, you ran TensorFlow **eagerly**. This means TensorFlow operations are executed by Python, operation by operation, and returning results back to Python.\n",
+ "In the previous three guides, you ran TensorFlow **eagerly**. This means TensorFlow operations are executed by Python, operation by operation, and return results back to Python.\n",
"\n",
"While eager execution has several unique advantages, graph execution enables portability outside Python and tends to offer better performance. **Graph execution** means that tensor computations are executed as a *TensorFlow graph*, sometimes referred to as a `tf.Graph` or simply a \"graph.\"\n",
"\n",
@@ -174,7 +174,7 @@
"source": [
"## Taking advantage of graphs\n",
"\n",
- "You create and run a graph in TensorFlow by using `tf.function`, either as a direct call or as a decorator. `tf.function` takes a regular function as input and returns a `Function`. **A `Function` is a Python callable that builds TensorFlow graphs from the Python function. You use a `Function` in the same way as its Python equivalent.**\n"
+ "You create and run a graph in TensorFlow by using `tf.function`, either as a direct call or as a decorator. `tf.function` takes a regular function as input and returns a `tf.types.experimental.PolymorphicFunction`. **A `PolymorphicFunction` is a Python callable that builds TensorFlow graphs from the Python function. You use a `tf.function` in the same way as its Python equivalent.**\n"
]
},
{
@@ -191,7 +191,8 @@
" x = x + b\n",
" return x\n",
"\n",
- "# `a_function_that_uses_a_graph` is a TensorFlow `Function`.\n",
+ "# The Python type of `a_function_that_uses_a_graph` will now be a\n",
+ "# `PolymorphicFunction`.\n",
"a_function_that_uses_a_graph = tf.function(a_regular_function)\n",
"\n",
"# Make some tensors.\n",
@@ -200,7 +201,7 @@
"b1 = tf.constant(4.0)\n",
"\n",
"orig_value = a_regular_function(x1, y1, b1).numpy()\n",
- "# Call a `Function` like a Python function.\n",
+ "# Call a `tf.function` like a Python function.\n",
"tf_function_value = a_function_that_uses_a_graph(x1, y1, b1).numpy()\n",
"assert(orig_value == tf_function_value)"
]
@@ -211,7 +212,7 @@
"id": "PNvuAYpdrTOf"
},
"source": [
- "On the outside, a `Function` looks like a regular function you write using TensorFlow operations. [Underneath](https://github.com/tensorflow/tensorflow/blob/master/tensorflow/python/eager/def_function.py), however, it is *very different*. A `Function` **encapsulates several `tf.Graph`s behind one API** (learn more in the _Polymorphism_ section). That is how a `Function` is able to give you the benefits of graph execution, like speed and deployability (refer to _The benefits of graphs_ above)."
+ "On the outside, a `tf.function` looks like a regular function you write using TensorFlow operations. [Underneath](https://github.com/tensorflow/tensorflow/blob/master/tensorflow/python/eager/polymorphic_function/polymorphic_function.py), however, it is *very different*. The underlying `PolymorphicFunction` **encapsulates several `tf.Graph`s behind one API** (learn more in the _Polymorphism_ section). That is how a `tf.function` is able to give you the benefits of graph execution, like speed and deployability (refer to _The benefits of graphs_ above)."
]
},
{
@@ -236,7 +237,8 @@
" x = x + b\n",
" return x\n",
"\n",
- "# Use the decorator to make `outer_function` a `Function`.\n",
+ "# Using the `tf.function` decorator makes `outer_function` into a\n",
+ "# `PolymorphicFunction`.\n",
"@tf.function\n",
"def outer_function(x):\n",
" y = tf.constant([[2.0], [3.0]])\n",
@@ -283,7 +285,8 @@
" else:\n",
" return 0\n",
"\n",
- "# `tf_simple_relu` is a TensorFlow `Function` that wraps `simple_relu`.\n",
+ "# Using `tf.function` makes `tf_simple_relu` a `PolymorphicFunction` that wraps\n",
+ "# `simple_relu`.\n",
"tf_simple_relu = tf.function(simple_relu)\n",
"\n",
"print(\"First branch, with graph:\", tf_simple_relu(tf.constant(1)).numpy())\n",
@@ -338,13 +341,13 @@
"id": "sIpc_jfjEZEg"
},
"source": [
- "### Polymorphism: one `Function`, many graphs\n",
+ "### Polymorphism: one `tf.function`, many graphs\n",
"\n",
"A `tf.Graph` is specialized to a specific type of inputs (for example, tensors with a specific [`dtype`](https://www.tensorflow.org/api_docs/python/tf/dtypes/DType) or objects with the same [`id()`](https://docs.python.org/3/library/functions.html#id)).\n",
"\n",
- "Each time you invoke a `Function` with a set of arguments that can't be handled by any of its existing graphs (such as arguments with new `dtypes` or incompatible shapes), `Function` creates a new `tf.Graph` specialized to those new arguments. The type specification of a `tf.Graph`'s inputs is known as its **input signature** or just a **signature**. For more information regarding when a new `tf.Graph` is generated and how that can be controlled, go to the _Rules of tracing_ section of the [Better performance with `tf.function`](./function.ipynb) guide.\n",
+ "Each time you invoke a `tf.function` with a set of arguments that can't be handled by any of its existing graphs (such as arguments with new `dtypes` or incompatible shapes), it creates a new `tf.Graph` specialized to those new arguments. The type specification of a `tf.Graph`'s inputs is represented by `tf.types.experimental.FunctionType`, also referred to as the **signature**. For more information regarding when a new `tf.Graph` is generated, how that can be controlled, and how `FunctionType` can be useful, go to the _Rules of tracing_ section of the [Better performance with `tf.function`](./function.ipynb) guide.\n",
"\n",
- "The `Function` stores the `tf.Graph` corresponding to that signature in a `ConcreteFunction`. **A `ConcreteFunction` is a wrapper around a `tf.Graph`.**\n"
+ "The `tf.function` stores the `tf.Graph` corresponding to that signature in a `ConcreteFunction`. **A `ConcreteFunction` can be thought of as a wrapper around a `tf.Graph`.**\n"
]
},
{
@@ -359,7 +362,7 @@
"def my_relu(x):\n",
" return tf.maximum(0., x)\n",
"\n",
- "# `my_relu` creates new graphs as it observes more signatures.\n",
+ "# `my_relu` creates new graphs as it observes different input types.\n",
"print(my_relu(tf.constant(5.5)))\n",
"print(my_relu([1, -1]))\n",
"print(my_relu(tf.constant([3., -3.])))"
@@ -371,7 +374,7 @@
"id": "1qRtw7R4KL9X"
},
"source": [
- "If the `Function` has already been called with that signature, `Function` does not create a new `tf.Graph`."
+ "If the `tf.function` has already been called with the same input types, it does not create a new `tf.Graph`."
]
},
{
@@ -383,8 +386,8 @@
"outputs": [],
"source": [
"# These two calls do *not* create new graphs.\n",
- "print(my_relu(tf.constant(-2.5))) # Signature matches `tf.constant(5.5)`.\n",
- "print(my_relu(tf.constant([-1., 1.]))) # Signature matches `tf.constant([3., -3.])`."
+ "print(my_relu(tf.constant(-2.5))) # Input type matches `tf.constant(5.5)`.\n",
+ "print(my_relu(tf.constant([-1., 1.]))) # Input type matches `tf.constant([3., -3.])`."
]
},
{
@@ -393,7 +396,7 @@
"id": "UohRmexhIpvQ"
},
"source": [
- "Because it's backed by multiple graphs, a `Function` is **polymorphic**. That enables it to support more input types than a single `tf.Graph` could represent, and to optimize each `tf.Graph` for better performance."
+ "Because it's backed by multiple graphs, a `tf.function` is (as the name \"PolymorphicFunction\" suggests) **polymorphic**. That enables it to support more input types than a single `tf.Graph` could represent, and to optimize each `tf.Graph` for better performance."
]
},
{
@@ -428,7 +431,7 @@
"source": [
"### Graph execution vs. eager execution\n",
"\n",
- "The code in a `Function` can be executed both eagerly and as a graph. By default, `Function` executes its code as a graph:\n"
+ "The code in a `tf.function` can be executed both eagerly and as a graph. By default, `tf.function` executes its code as a graph:\n"
]
},
{
@@ -476,7 +479,7 @@
"id": "cyZNCRcQorGO"
},
"source": [
- "To verify that your `Function`'s graph is doing the same computation as its equivalent Python function, you can make it execute eagerly with `tf.config.run_functions_eagerly(True)`. This is a switch that **turns off `Function`'s ability to create and run graphs**, instead of executing the code normally."
+ "To verify that your `tf.function`'s graph is doing the same computation as its equivalent Python function, you can make it execute eagerly with `tf.config.run_functions_eagerly(True)`. This is a switch that **turns off `tf.function`'s ability to create and run graphs**, instead of executing the code normally."
]
},
{
@@ -519,7 +522,7 @@
"id": "DKT3YBsqy0x4"
},
"source": [
- "However, `Function` can behave differently under graph and eager execution. The Python [`print`](https://docs.python.org/3/library/functions.html#print) function is one example of how these two modes differ. Let's check out what happens when you insert a `print` statement to your function and call it repeatedly."
+ "However, `tf.function` can behave differently under graph and eager execution. The Python [`print`](https://docs.python.org/3/library/functions.html#print) function is one example of how these two modes differ. Let's check out what happens when you insert a `print` statement to your function and call it repeatedly."
]
},
{
@@ -567,7 +570,7 @@
"source": [
"Is the output surprising? **`get_MSE` only printed once even though it was called *three* times.**\n",
"\n",
- "To explain, the `print` statement is executed when `Function` runs the original code in order to create the graph in a process known as \"tracing\" (refer to the _Tracing_ section of the [`tf.function` guide](./function.ipynb). **Tracing captures the TensorFlow operations into a graph, and `print` is not captured in the graph.** That graph is then executed for all three calls **without ever running the Python code again**.\n",
+ "To explain, the `print` statement is executed when `tf.function` runs the original code in order to create the graph in a process known as \"tracing\" (refer to the _Tracing_ section of the [`tf.function` guide](./function.ipynb). **Tracing captures the TensorFlow operations into a graph, and `print` is not captured in the graph.** That graph is then executed for all three calls **without ever running the Python code again**.\n",
"\n",
"As a sanity check, let's turn off graph execution to compare:"
]
@@ -615,7 +618,7 @@
"id": "PUR7qC_bquCn"
},
"source": [
- "`print` is a *Python side effect*, and there are other differences that you should be aware of when converting a function into a `Function`. Learn more in the _Limitations_ section of the [Better performance with `tf.function`](./function.ipynb) guide."
+ "`print` is a *Python side effect*, and there are other differences that you should be aware of when converting a function into a `tf.function`. Learn more in the _Limitations_ section of the [Better performance with `tf.function`](./function.ipynb) guide."
]
},
{
@@ -637,7 +640,7 @@
"\n",
"\n",
"\n",
- "Graph execution only executes the operations necessary to produce the observable effects, which includes:\n",
+ "Graph execution only executes the operations necessary to produce the observable effects, which include:\n",
"\n",
"- The return value of the function\n",
"- Documented well-known side-effects such as:\n",
@@ -697,7 +700,7 @@
"source": [
"### `tf.function` best practices\n",
"\n",
- "It may take some time to get used to the behavior of `Function`. To get started quickly, first-time users should play around with decorating toy functions with `@tf.function` to get experience with going from eager to graph execution.\n",
+ "It may take some time to get used to the behavior of `tf.function`. To get started quickly, first-time users should play around with decorating toy functions with `@tf.function` to get experience with going from eager to graph execution.\n",
"\n",
"*Designing for `tf.function`* may be your best bet for writing graph-compatible TensorFlow programs. Here are some tips:\n",
"- Toggle between eager and graph execution early and often with `tf.config.run_functions_eagerly` to pinpoint if/ when the two modes diverge.\n",
@@ -787,7 +790,7 @@
"\n",
"Graphs can speed up your code, but the process of creating them has some overhead. For some functions, the creation of the graph takes more time than the execution of the graph. **This investment is usually quickly paid back with the performance boost of subsequent executions, but it's important to be aware that the first few steps of any large model training can be slower due to tracing.**\n",
"\n",
- "No matter how large your model, you want to avoid tracing frequently. The [`tf.function` guide](./function.ipynb) discusses how to set input specifications and use tensor arguments to avoid retracing in the _Controlling retracing_ section. If you find you are getting unusually poor performance, it's a good idea to check if you are retracing accidentally."
+ "No matter how large your model, you want to avoid tracing frequently. In the _Controlling retracing_ section, the [`tf.function` guide](./function.ipynb) discusses how to set input specifications and use tensor arguments to avoid retracing. If you find you are getting unusually poor performance, it's a good idea to check if you are retracing accidentally."
]
},
{
@@ -796,9 +799,9 @@
"id": "F4InDaTjwmBA"
},
"source": [
- "## When is a `Function` tracing?\n",
+ "## When is a `tf.function` tracing?\n",
"\n",
- "To figure out when your `Function` is tracing, add a `print` statement to its code. As a rule of thumb, `Function` will execute the `print` statement every time it traces."
+ "To figure out when your `tf.function` is tracing, add a `print` statement to its code. As a rule of thumb, `tf.function` will execute the `print` statement every time it traces."
]
},
{
diff --git a/site/en/guide/migrate/evaluator.ipynb b/site/en/guide/migrate/evaluator.ipynb
index fd8bd12d1e1..c8f848e4406 100644
--- a/site/en/guide/migrate/evaluator.ipynb
+++ b/site/en/guide/migrate/evaluator.ipynb
@@ -122,7 +122,7 @@
"\n",
"In TensorFlow 1, you can configure a `tf.estimator` to evaluate the estimator using `tf.estimator.train_and_evaluate`.\n",
"\n",
- "In this example, start by defining the `tf.estimator.Estimator` and speciyfing training and evaluation specifications:"
+ "In this example, start by defining the `tf.estimator.Estimator` and specifying training and evaluation specifications:"
]
},
{
diff --git a/site/en/guide/migrate/migrating_feature_columns.ipynb b/site/en/guide/migrate/migrating_feature_columns.ipynb
index ea12a5ef391..b2dbc5fe7c0 100644
--- a/site/en/guide/migrate/migrating_feature_columns.ipynb
+++ b/site/en/guide/migrate/migrating_feature_columns.ipynb
@@ -654,17 +654,17 @@
"source": [
"categorical_col = tf1.feature_column.categorical_column_with_identity(\n",
" 'type', num_buckets=one_hot_dims)\n",
- "# Convert index to one-hot; e.g. [2] -> [0,0,1].\n",
+ "# Convert index to one-hot; e.g., [2] -> [0,0,1].\n",
"indicator_col = tf1.feature_column.indicator_column(categorical_col)\n",
"\n",
- "# Convert strings to indices; e.g. ['small'] -> [1].\n",
+ "# Convert strings to indices; e.g., ['small'] -> [1].\n",
"vocab_col = tf1.feature_column.categorical_column_with_vocabulary_list(\n",
" 'size', vocabulary_list=vocab, num_oov_buckets=1)\n",
"# Embed the indices.\n",
"embedding_col = tf1.feature_column.embedding_column(vocab_col, embedding_dims)\n",
"\n",
"normalizer_fn = lambda x: (x - weight_mean) / math.sqrt(weight_variance)\n",
- "# Normalize the numeric inputs; e.g. [2.0] -> [0.0].\n",
+ "# Normalize the numeric inputs; e.g., [2.0] -> [0.0].\n",
"numeric_col = tf1.feature_column.numeric_column(\n",
" 'weight', normalizer_fn=normalizer_fn)\n",
"\n",
@@ -727,12 +727,12 @@
" 'size': tf.keras.Input(shape=(), dtype='string'),\n",
" 'weight': tf.keras.Input(shape=(), dtype='float32'),\n",
"}\n",
- "# Convert index to one-hot; e.g. [2] -> [0,0,1].\n",
+ "# Convert index to one-hot; e.g., [2] -> [0,0,1].\n",
"type_output = tf.keras.layers.CategoryEncoding(\n",
" one_hot_dims, output_mode='one_hot')(inputs['type'])\n",
- "# Convert size strings to indices; e.g. ['small'] -> [1].\n",
+ "# Convert size strings to indices; e.g., ['small'] -> [1].\n",
"size_output = tf.keras.layers.StringLookup(vocabulary=vocab)(inputs['size'])\n",
- "# Normalize the numeric inputs; e.g. [2.0] -> [0.0].\n",
+ "# Normalize the numeric inputs; e.g., [2.0] -> [0.0].\n",
"weight_output = tf.keras.layers.Normalization(\n",
" axis=None, mean=weight_mean, variance=weight_variance)(inputs['weight'])\n",
"outputs = {\n",
diff --git a/site/en/guide/migrate/migration_debugging.ipynb b/site/en/guide/migrate/migration_debugging.ipynb
index 86c86680dc9..25cb7f9065f 100644
--- a/site/en/guide/migrate/migration_debugging.ipynb
+++ b/site/en/guide/migrate/migration_debugging.ipynb
@@ -128,7 +128,7 @@
"\n",
" a. Check training behaviors with TensorBoard\n",
"\n",
- " * use simple optimizers e.g. SGD and simple distribution strategies e.g.\n",
+ " * use simple optimizers e.g., SGD and simple distribution strategies e.g.\n",
" `tf.distribute.OneDeviceStrategy` first\n",
" * training metrics\n",
" * evaluation metrics\n",
diff --git a/site/en/guide/profiler.md b/site/en/guide/profiler.md
index 1cd19c109fe..dee8a5a84af 100644
--- a/site/en/guide/profiler.md
+++ b/site/en/guide/profiler.md
@@ -55,7 +55,7 @@ found.
When you run profiling with CUDA® Toolkit in a Docker environment or on Linux,
you may encounter issues related to insufficient CUPTI privileges
(`CUPTI_ERROR_INSUFFICIENT_PRIVILEGES`). Go to the
-[NVIDIA Developer Docs](https://developer.nvidia.com/nvidia-development-tools-solutions-ERR_NVGPUCTRPERM-permission-issue-performance-counters){:.external}
+[NVIDIA Developer Docs](https://developer.nvidia.com/nvidia-development-tools-solutions-ERR_NVGPUCTRPERM-permission-issue-performance-counters)
to learn more about how you can resolve these issues on Linux.
To resolve CUPTI privilege issues in a Docker environment, run
@@ -694,7 +694,7 @@ first few batches to avoid inaccuracies due to initialization overhead.
An example for profiling multiple workers:
```python
- # E.g. your worker IP addresses are 10.0.0.2, 10.0.0.3, 10.0.0.4, and you
+ # E.g., your worker IP addresses are 10.0.0.2, 10.0.0.3, 10.0.0.4, and you
# would like to profile for a duration of 2 seconds.
tf.profiler.experimental.client.trace(
'grpc://10.0.0.2:8466,grpc://10.0.0.3:8466,grpc://10.0.0.4:8466',
@@ -845,7 +845,7 @@ more efficient by casting to different data types after applying
spatial transformations, such as flipping, cropping, rotating, etc.
Note: Some ops like `tf.image.resize` transparently change the `dtype` to
-`fp32`. Make sure you normalize your data to lie between `0` and `1` if its not
+`fp32`. Make sure you normalize your data to lie between `0` and `1` if it's not
done automatically. Skipping this step could lead to `NaN` errors if you have
enabled [AMP](https://developer.nvidia.com/automatic-mixed-precision).
diff --git a/site/en/guide/ragged_tensor.ipynb b/site/en/guide/ragged_tensor.ipynb
index d36010699db..ba0be2928ce 100644
--- a/site/en/guide/ragged_tensor.ipynb
+++ b/site/en/guide/ragged_tensor.ipynb
@@ -674,14 +674,14 @@
"source": [
"### Keras\n",
"\n",
- "[tf.keras](https://www.tensorflow.org/guide/keras) is TensorFlow's high-level API for building and training deep learning models. Ragged tensors may be passed as inputs to a Keras model by setting `ragged=True` on `tf.keras.Input` or `tf.keras.layers.InputLayer`. Ragged tensors may also be passed between Keras layers, and returned by Keras models. The following example shows a toy LSTM model that is trained using ragged tensors."
+ "[tf.keras](https://www.tensorflow.org/guide/keras) is TensorFlow's high-level API for building and training deep learning models. It doesn't have ragged support. But it does support masked tensors. So the easiest way to use a ragged tensor in a Keras model is to convert the ragged tensor to a dense tensor, using `.to_tensor()` and then using Keras's builtin masking:"
]
},
{
"cell_type": "code",
"execution_count": null,
"metadata": {
- "id": "pHls7hQVJlk5"
+ "id": "ucYf2sSzTvQo"
},
"outputs": [],
"source": [
@@ -691,26 +691,77 @@
" 'She turned me into a newt.',\n",
" 'A newt?',\n",
" 'Well, I got better.'])\n",
- "is_question = tf.constant([True, False, True, False])\n",
- "\n",
+ "is_question = tf.constant([True, False, True, False])"
+ ]
+ },
+ {
+ "cell_type": "code",
+ "execution_count": null,
+ "metadata": {
+ "id": "MGYKmizJTw8B"
+ },
+ "outputs": [],
+ "source": [
"# Preprocess the input strings.\n",
"hash_buckets = 1000\n",
"words = tf.strings.split(sentences, ' ')\n",
"hashed_words = tf.strings.to_hash_bucket_fast(words, hash_buckets)\n",
- "\n",
+ "hashed_words.to_list()"
+ ]
+ },
+ {
+ "cell_type": "code",
+ "execution_count": null,
+ "metadata": {
+ "id": "7FTujwOlUT8J"
+ },
+ "outputs": [],
+ "source": [
+ "hashed_words.to_tensor()"
+ ]
+ },
+ {
+ "cell_type": "code",
+ "execution_count": null,
+ "metadata": {
+ "id": "vzWudaESUBOZ"
+ },
+ "outputs": [],
+ "source": [
+ "tf.keras.Input?"
+ ]
+ },
+ {
+ "cell_type": "code",
+ "execution_count": null,
+ "metadata": {
+ "id": "pHls7hQVJlk5"
+ },
+ "outputs": [],
+ "source": [
"# Build the Keras model.\n",
"keras_model = tf.keras.Sequential([\n",
- " tf.keras.layers.Input(shape=[None], dtype=tf.int64, ragged=True),\n",
- " tf.keras.layers.Embedding(hash_buckets, 16),\n",
- " tf.keras.layers.LSTM(32, use_bias=False),\n",
+ " tf.keras.layers.Embedding(hash_buckets, 16, mask_zero=True),\n",
+ " tf.keras.layers.LSTM(32, return_sequences=True, use_bias=False),\n",
+ " tf.keras.layers.GlobalAveragePooling1D(),\n",
" tf.keras.layers.Dense(32),\n",
" tf.keras.layers.Activation(tf.nn.relu),\n",
" tf.keras.layers.Dense(1)\n",
"])\n",
"\n",
"keras_model.compile(loss='binary_crossentropy', optimizer='rmsprop')\n",
- "keras_model.fit(hashed_words, is_question, epochs=5)\n",
- "print(keras_model.predict(hashed_words))"
+ "keras_model.fit(hashed_words.to_tensor(), is_question, epochs=5)\n"
+ ]
+ },
+ {
+ "cell_type": "code",
+ "execution_count": null,
+ "metadata": {
+ "id": "1IAjjmdTU9OU"
+ },
+ "outputs": [],
+ "source": [
+ "print(keras_model.predict(hashed_words.to_tensor()))"
]
},
{
@@ -799,7 +850,7 @@
"source": [
"### Datasets\n",
"\n",
- "[tf.data](https://www.tensorflow.org/guide/data) is an API that enables you to build complex input pipelines from simple, reusable pieces. Its core data structure is `tf.data.Dataset`, which represents a sequence of elements, in which each element consists of one or more components. "
+ "[tf.data](https://www.tensorflow.org/guide/data) is an API that enables you to build complex input pipelines from simple, reusable pieces. Its core data structure is `tf.data.Dataset`, which represents a sequence of elements, in which each element consists of one or more components."
]
},
{
@@ -1078,9 +1129,11 @@
"import tempfile\n",
"\n",
"keras_module_path = tempfile.mkdtemp()\n",
- "tf.saved_model.save(keras_model, keras_module_path)\n",
- "imported_model = tf.saved_model.load(keras_module_path)\n",
- "imported_model(hashed_words)"
+ "keras_model.save(keras_module_path+\"/my_model.keras\")\n",
+ "\n",
+ "imported_model = tf.keras.models.load_model(keras_module_path+\"/my_model.keras\")\n",
+ "\n",
+ "imported_model(hashed_words.to_tensor())"
]
},
{
@@ -2125,7 +2178,6 @@
],
"metadata": {
"colab": {
- "collapsed_sections": [],
"name": "ragged_tensor.ipynb",
"toc_visible": true
},
diff --git a/site/en/guide/random_numbers.ipynb b/site/en/guide/random_numbers.ipynb
index 5212a10a49a..f8b824ad906 100644
--- a/site/en/guide/random_numbers.ipynb
+++ b/site/en/guide/random_numbers.ipynb
@@ -166,7 +166,7 @@
"source": [
"See the *Algorithms* section below for more information about it.\n",
"\n",
- "Another way to create a generator is with `Generator.from_non_deterministic_state`. A generator created this way will start from a non-deterministic state, depending on e.g. time and OS."
+ "Another way to create a generator is with `Generator.from_non_deterministic_state`. A generator created this way will start from a non-deterministic state, depending on e.g., time and OS."
]
},
{
diff --git a/site/en/guide/sparse_tensor.ipynb b/site/en/guide/sparse_tensor.ipynb
index cd38fdf55ab..45f1e3fd3c3 100644
--- a/site/en/guide/sparse_tensor.ipynb
+++ b/site/en/guide/sparse_tensor.ipynb
@@ -620,7 +620,7 @@
"\n",
"However, there are a few cases where it can be useful to distinguish zero values from missing values. In particular, this allows for one way to encode missing/unknown data in your training data. For example, consider a use case where you have a tensor of scores (that can have any floating point value from -Inf to +Inf), with some missing scores. You can encode this tensor using a sparse tensor where the explicit zeros are known zero scores but the implicit zero values actually represent missing data and not zero. \n",
"\n",
- "Note: This is generally not the intended usage of `tf.sparse.SparseTensor`s; and you might want to also consier other techniques for encoding this such as for example using a separate mask tensor that identifies the locations of known/unknown values. However, exercise caution while using this approach, since most sparse operations will treat explicit and implicit zero values identically."
+ "Note: This is generally not the intended usage of `tf.sparse.SparseTensor`s; and you might want to also consider other techniques for encoding this such as for example using a separate mask tensor that identifies the locations of known/unknown values. However, exercise caution while using this approach, since most sparse operations will treat explicit and implicit zero values identically."
]
},
{
diff --git a/site/en/guide/tensor.ipynb b/site/en/guide/tensor.ipynb
index b9b72973db1..2eb261aad75 100644
--- a/site/en/guide/tensor.ipynb
+++ b/site/en/guide/tensor.ipynb
@@ -82,7 +82,7 @@
"source": [
"Tensors are multi-dimensional arrays with a uniform type (called a `dtype`). You can see all supported `dtypes` at `tf.dtypes`.\n",
"\n",
- "If you're familiar with [NumPy](https://numpy.org/devdocs/user/quickstart.html){:.external}, tensors are (kind of) like `np.arrays`.\n",
+ "If you're familiar with [NumPy](https://numpy.org/devdocs/user/quickstart.html), tensors are (kind of) like `np.arrays`.\n",
"\n",
"All tensors are immutable like Python numbers and strings: you can never update the contents of a tensor, only create a new one.\n"
]
@@ -571,7 +571,7 @@
"source": [
"### Single-axis indexing\n",
"\n",
- "TensorFlow follows standard Python indexing rules, similar to [indexing a list or a string in Python](https://docs.python.org/3/tutorial/introduction.html#strings){:.external}, and the basic rules for NumPy indexing.\n",
+ "TensorFlow follows standard Python indexing rules, similar to [indexing a list or a string in Python](https://docs.python.org/3/tutorial/introduction.html#strings), and the basic rules for NumPy indexing.\n",
"\n",
"* indexes start at `0`\n",
"* negative indices count backwards from the end\n",
@@ -1035,7 +1035,7 @@
"source": [
"## Broadcasting\n",
"\n",
- "Broadcasting is a concept borrowed from the [equivalent feature in NumPy](https://numpy.org/doc/stable/user/basics.broadcasting.html){:.external}. In short, under certain conditions, smaller tensors are \"stretched\" automatically to fit larger tensors when running combined operations on them.\n",
+ "Broadcasting is a concept borrowed from the [equivalent feature in NumPy](https://numpy.org/doc/stable/user/basics.broadcasting.html). In short, under certain conditions, smaller tensors are \"stretched\" automatically to fit larger tensors when running combined operations on them.\n",
"\n",
"The simplest and most common case is when you attempt to multiply or add a tensor to a scalar. In that case, the scalar is broadcast to be the same shape as the other argument. "
]
@@ -1161,7 +1161,7 @@
"source": [
"Unlike a mathematical op, for example, `broadcast_to` does nothing special to save memory. Here, you are materializing the tensor.\n",
"\n",
- "It can get even more complicated. [This section](https://jakevdp.github.io/PythonDataScienceHandbook/02.05-computation-on-arrays-broadcasting.html){:.external} of Jake VanderPlas's book _Python Data Science Handbook_ shows more broadcasting tricks (again in NumPy)."
+ "It can get even more complicated. [This section](https://jakevdp.github.io/PythonDataScienceHandbook/02.05-computation-on-arrays-broadcasting.html) of Jake VanderPlas's book _Python Data Science Handbook_ shows more broadcasting tricks (again in NumPy)."
]
},
{
diff --git a/site/en/guide/tf_numpy_type_promotion.ipynb b/site/en/guide/tf_numpy_type_promotion.ipynb
index a9e176c5db6..f984310822a 100644
--- a/site/en/guide/tf_numpy_type_promotion.ipynb
+++ b/site/en/guide/tf_numpy_type_promotion.ipynb
@@ -178,7 +178,7 @@
"* `f32*` means Python `float` or weakly-typed `f32`\n",
"* `c128*` means Python `complex` or weakly-typed `c128`\n",
"\n",
- "The asterik (*) denotes that the corresponding type is “weak” - such a dtype is temporarily inferred by the system, and could defer to other dtypes. This concept is explained more in detail [here](#weak_tensor)."
+ "The asterisk (*) denotes that the corresponding type is “weak” - such a dtype is temporarily inferred by the system, and could defer to other dtypes. This concept is explained more in detail [here](#weak_tensor)."
]
},
{
@@ -449,13 +449,13 @@
"source": [
"### WeakTensor Construction\n",
"\n",
- "WeakTensors are created if you create a tensor without specifing a dtype the result is a WeakTensor. You can check whether a Tensor is \"weak\" or not by checking the weak attribute at the end of the Tensor's string representation."
+ "WeakTensors are created if you create a tensor without specifying a dtype the result is a WeakTensor. You can check whether a Tensor is \"weak\" or not by checking the weak attribute at the end of the Tensor's string representation."
]
},
{
"cell_type": "markdown",
"metadata": {
- "id": "7UmunnJ8Tru3"
+ "id": "7UmunnJ8True3"
},
"source": [
"**First Case**: When `tf.constant` is called with an input with no user-specified dtype."
diff --git a/site/en/guide/tpu.ipynb b/site/en/guide/tpu.ipynb
index c37b9af576e..49eee544bec 100644
--- a/site/en/guide/tpu.ipynb
+++ b/site/en/guide/tpu.ipynb
@@ -6,7 +6,7 @@
"id": "Tce3stUlHN0L"
},
"source": [
- "##### Copyright 2018 The TensorFlow Authors.\n"
+ "##### Copyright 2024 The TensorFlow Authors.\n"
]
},
{
@@ -81,7 +81,7 @@
"id": "ebf7f8489bb7"
},
"source": [
- "Before you run this Colab notebook, make sure that your hardware accelerator is a TPU by checking your notebook settings: **Runtime** > **Change runtime type** > **Hardware accelerator** > **TPU**.\n",
+ "Before you run this Colab notebook, make sure that your hardware accelerator is a TPU by checking your notebook settings: **Runtime** > **Change runtime type** > **Hardware accelerator** > **TPU v2**.\n",
"\n",
"Import some necessary libraries, including TensorFlow Datasets:"
]
@@ -128,7 +128,7 @@
},
"outputs": [],
"source": [
- "resolver = tf.distribute.cluster_resolver.TPUClusterResolver(tpu='')\n",
+ "resolver = tf.distribute.cluster_resolver.TPUClusterResolver(tpu='local')\n",
"tf.config.experimental_connect_to_cluster(resolver)\n",
"# This is the TPU initialization code that has to be at the beginning.\n",
"tf.tpu.experimental.initialize_tpu_system(resolver)\n",
@@ -416,7 +416,7 @@
"source": [
"### Train the model using a custom training loop\n",
"\n",
- "You can also create and train your model using `tf.function` and `tf.distribute` APIs directly. You can use the `Strategy.experimental_distribute_datasets_from_function` API to distribute the `tf.data.Dataset` given a dataset function. Note that in the example below the batch size passed into the `Dataset` is the per-replica batch size instead of the global batch size. To learn more, check out the [Custom training with `tf.distribute.Strategy`](../tutorials/distribute/custom_training.ipynb) tutorial.\n"
+ "You can also create and train your model using `tf.function` and `tf.distribute` APIs directly. You can use the `Strategy.distribute_datasets_from_function` API to distribute the `tf.data.Dataset` given a dataset function. Note that in the example below the batch size passed into the `Dataset` is the per-replica batch size instead of the global batch size. To learn more, check out the [Custom training with `tf.distribute.Strategy`](../tutorials/distribute/custom_training.ipynb) tutorial.\n"
]
},
{
@@ -590,7 +590,8 @@
"colab": {
"name": "tpu.ipynb",
"toc_visible": true,
- "provenance": []
+ "machine_shape": "hm",
+ "gpuType": "V28"
},
"kernelspec": {
"display_name": "Python 3",
diff --git a/site/en/guide/versions.md b/site/en/guide/versions.md
index df0d75114ef..8443e549f42 100644
--- a/site/en/guide/versions.md
+++ b/site/en/guide/versions.md
@@ -59,7 +59,7 @@ patch versions. The public APIs consist of
* The TensorFlow C API:
- * [tensorflow/c/c_api.h](https://github.com/tensorflow/tensorflow/blob/master/tensorflow/c/c_api.h))
+ * [tensorflow/c/c_api.h](https://github.com/tensorflow/tensorflow/blob/master/tensorflow/c/c_api.h)
* The following protocol buffer files:
@@ -171,12 +171,10 @@ incrementing the major version number for TensorFlow Lite, or vice versa.
The API surface that is covered by the TensorFlow Lite Extension APIs version
number is comprised of the following public APIs:
-```
* [tensorflow/lite/c/c_api_opaque.h](https://github.com/tensorflow/tensorflow/blob/master/tensorflow/lite/c/c_api_opaque.h)
* [tensorflow/lite/c/common.h](https://github.com/tensorflow/tensorflow/blob/master/tensorflow/lite/c/common.h)
* [tensorflow/lite/c/builtin_op_data.h](https://github.com/tensorflow/tensorflow/blob/master/tensorflow/lite/c/builtin_op_data.h)
* [tensorflow/lite/builtin_ops.h](https://github.com/tensorflow/tensorflow/blob/master/tensorflow/lite/builtin_ops.h)
-```
Again, experimental symbols are not covered; see [below](#not_covered) for
details.
@@ -203,7 +201,7 @@ These include:
such as:
- [C++](../install/lang_c.ipynb) (exposed through header files in
- [`tensorflow/cc`](https://github.com/tensorflow/tensorflow/tree/master/tensorflow/cc)).
+ [`tensorflow/cc/`](https://github.com/tensorflow/tensorflow/tree/master/tensorflow/cc)).
- [Java](../install/lang_java_legacy.md),
- [Go](https://github.com/tensorflow/build/blob/master/golang_install_guide/README.md)
- [JavaScript](https://www.tensorflow.org/js)
@@ -212,7 +210,7 @@ These include:
Objective-C, and Swift, in particular
- **C++** (exposed through header files in
- [`tensorflow/lite/`]\(https://github.com/tensorflow/tensorflow/tree/master/tensorflow/lite/\))
+ [`tensorflow/lite/`](https://github.com/tensorflow/tensorflow/tree/master/tensorflow/lite/))
* **Details of composite ops:** Many public functions in Python expand to
several primitive ops in the graph, and these details will be part of any
@@ -471,7 +469,7 @@ existing producer scripts will not suddenly use the new functionality.
1. Add a new similar op named `SomethingV2` or similar and go through the
process of adding it and switching existing Python wrappers to use it.
To ensure forward compatibility use the checks suggested in
- [compat.py](https://www.tensorflow.org/code/tensorflow/python/compat/compat.py)
+ [compat.py](https://github.com/tensorflow/tensorflow/blob/master/tensorflow/python/compat/compat.py)
when changing the Python wrappers.
2. Remove the old op (Can only take place with a major version change due to
backward compatibility).
diff --git a/site/en/hub/common_saved_model_apis/images.md b/site/en/hub/common_saved_model_apis/images.md
index 9754d52feed..5413f0adc07 100644
--- a/site/en/hub/common_saved_model_apis/images.md
+++ b/site/en/hub/common_saved_model_apis/images.md
@@ -70,7 +70,7 @@ consumer. The SavedModel itself should not perform dropout on the actual outputs
Reusable SavedModels for image feature vectors are used in
* the Colab tutorial
- [Retraining an Image Classifier](https://colab.research.google.com/github/tensorflow/docs/blob/master/g3doc/en/hub/tutorials/tf2_image_retraining.ipynb),
+ [Retraining an Image Classifier](https://colab.research.google.com/github/tensorflow/docs/blob/master/site/en/hub/tutorials/tf2_image_retraining.ipynb),
diff --git a/site/en/hub/common_saved_model_apis/text.md b/site/en/hub/common_saved_model_apis/text.md
index 1c45b8ea026..209319f27a9 100644
--- a/site/en/hub/common_saved_model_apis/text.md
+++ b/site/en/hub/common_saved_model_apis/text.md
@@ -94,7 +94,7 @@ distributed way. For example
### Examples
* Colab tutorial
- [Text Classification with Movie Reviews](https://colab.research.google.com/github/tensorflow/docs/blob/master/g3doc/en/hub/tutorials/tf2_text_classification.ipynb).
+ [Text Classification with Movie Reviews](https://colab.research.google.com/github/tensorflow/docs/blob/master/site/en/hub/tutorials/tf2_text_classification.ipynb).
@@ -132,8 +132,8 @@ preprocessor = hub.load("path/to/preprocessor") # Must match `encoder`.
encoder_inputs = preprocessor(text_input)
encoder = hub.load("path/to/encoder")
-enocder_outputs = encoder(encoder_inputs)
-embeddings = enocder_outputs["default"]
+encoder_outputs = encoder(encoder_inputs)
+embeddings = encoder_outputs["default"]
```
Recall from the [Reusable SavedModel API](../reusable_saved_models.md) that
@@ -304,8 +304,8 @@ provisions from the [Reusable SavedModel API](../reusable_saved_models.md).
#### Usage synopsis
```python
-enocder = hub.load("path/to/encoder")
-enocder_outputs = encoder(encoder_inputs)
+encoder = hub.load("path/to/encoder")
+encoder_outputs = encoder(encoder_inputs)
```
or equivalently in Keras:
diff --git a/site/en/hub/installation.md b/site/en/hub/installation.md
index 33594cd3079..2381fbea614 100644
--- a/site/en/hub/installation.md
+++ b/site/en/hub/installation.md
@@ -50,8 +50,8 @@ $ pip install --upgrade tf-hub-nightly
- [Library overview](lib_overview.md)
- Tutorials:
- - [Text classification](https://github.com/tensorflow/docs/blob/master/g3doc/en/hub/tutorials/tf2_text_classification.ipynb)
- - [Image classification](https://github.com/tensorflow/docs/blob/master/g3doc/en/hub/tutorials/tf2_image_retraining.ipynb)
+ - [Text classification](https://github.com/tensorflow/docs/blob/master/site/en/hub/tutorials/tf2_text_classification.ipynb)
+ - [Image classification](https://github.com/tensorflow/docs/blob/master/site/en/hub/tutorials/tf2_image_retraining.ipynb)
- Additional examples
[on GitHub](https://github.com/tensorflow/hub/blob/master/examples/README.md)
- Find models on [tfhub.dev](https://tfhub.dev).
\ No newline at end of file
diff --git a/site/en/hub/migration_tf2.md b/site/en/hub/migration_tf2.md
index 24c1bf14c4d..c2cc4b50759 100644
--- a/site/en/hub/migration_tf2.md
+++ b/site/en/hub/migration_tf2.md
@@ -46,10 +46,10 @@ model = tf.keras.Sequential([
...])
```
-Many tutorials show these APIs in action. See in particular
+Many tutorials show these APIs in action. Here are some examples:
-* [Text classification example notebook](https://github.com/tensorflow/docs/blob/master/g3doc/en/hub/tutorials/tf2_text_classification.ipynb)
-* [Image classification example notebook](https://github.com/tensorflow/docs/blob/master/g3doc/en/hub/tutorials/tf2_image_retraining.ipynb)
+* [Text classification example notebook](https://github.com/tensorflow/docs/blob/master/site/en/hub/tutorials/tf2_text_classification.ipynb)
+* [Image classification example notebook](https://github.com/tensorflow/docs/blob/master/site/en/hub/tutorials/tf2_image_retraining.ipynb)
### Using the new API in Estimator training
diff --git a/site/en/hub/portability_and_deletion.md b/site/en/hub/portability_and_deletion.md
index 30341306bea..67fa401d161 100644
--- a/site/en/hub/portability_and_deletion.md
+++ b/site/en/hub/portability_and_deletion.md
@@ -1,14 +1,14 @@
## I want to see what I’ve uploaded to TensorFlow Hub. Can I get a copy of my data?
-Yes. If you’d like the TensorFlow Hub team to **send you a copy** of all of the
-data you have uploaded, please send us an email at [hi-tf-hub@google.com](mailto:hi-tf-hub@google.com)
+Yes. If you’d like the Kaggle Team to **send you a copy** of all of the
+data you have uploaded, please send us an email at [support@kaggle.com](mailto:support@kaggle.com)
and we’ll respond as soon as possible.
## How do I delete what I’ve uploaded to TensorFlow Hub?
Similarly, if you’d like us to **delete or remove content**, please send us an
-email at [hi-tf-hub@google.com](mailto:hi-tf-hub@google.com) and we’ll delete
+email at [support@kaggle.com](mailto:support@kaggle.com) and we’ll delete
all copies that we have and stop serving it on tfhub.dev. Please note:
* Because TensorFlow Hub is an open-source platform, copies of your assets may
diff --git a/site/en/hub/tf2_saved_model.md b/site/en/hub/tf2_saved_model.md
index 7a7220d0a2e..e41337b2548 100644
--- a/site/en/hub/tf2_saved_model.md
+++ b/site/en/hub/tf2_saved_model.md
@@ -51,7 +51,7 @@ model = tf.keras.Sequential([
```
The [Text classification
-colab](https://colab.research.google.com/github/tensorflow/docs/blob/master/g3doc/en/hub/tutorials/tf2_text_classification.ipynb)
+colab](https://colab.research.google.com/github/tensorflow/docs/blob/master/site/en/hub/tutorials/tf2_text_classification.ipynb)
is a complete example how to train and evaluate such a classifier.
The model weights in a `hub.KerasLayer` are set to non-trainable by default.
@@ -82,7 +82,7 @@ and uncompressed SavedModels. For details, see [Caching](caching.md).
SavedModels can be loaded from a specified `handle`, where the `handle` is a
filesystem path, valid TFhub.dev model URL (e.g. "https://tfhub.dev/...").
Kaggle Models URLs mirror TFhub.dev handles in accordance with our Terms and the
-license associated with the model assets, e.g. "https://www.kaggle.com/...".
+license associated with the model assets, e.g., "https://www.kaggle.com/...".
Handles from Kaggle Models are equivalent to their corresponding TFhub.dev
handle.
@@ -244,7 +244,7 @@ to the Keras model, and runs the SavedModel's computation in training
mode (think of dropout etc.).
The [image classification
-colab](https://github.com/tensorflow/docs/blob/master/g3doc/en/hub/tutorials/tf2_image_retraining.ipynb)
+colab](https://github.com/tensorflow/docs/blob/master/site/en/hub/tutorials/tf2_image_retraining.ipynb)
contains an end-to-end example with optional fine-tuning.
#### Re-exporting the fine-tuning result
diff --git a/site/en/hub/tutorials/action_recognition_with_tf_hub.ipynb b/site/en/hub/tutorials/action_recognition_with_tf_hub.ipynb
index b4a1e439621..3f586991ba9 100644
--- a/site/en/hub/tutorials/action_recognition_with_tf_hub.ipynb
+++ b/site/en/hub/tutorials/action_recognition_with_tf_hub.ipynb
@@ -184,7 +184,7 @@
" return list(_VIDEO_LIST)\n",
"\n",
"def fetch_ucf_video(video):\n",
- " \"\"\"Fetchs a video and cache into local filesystem.\"\"\"\n",
+ " \"\"\"Fetches a video and cache into local filesystem.\"\"\"\n",
" cache_path = os.path.join(_CACHE_DIR, video)\n",
" if not os.path.exists(cache_path):\n",
" urlpath = request.urljoin(UCF_ROOT, video)\n",
diff --git a/site/en/hub/tutorials/boundless.ipynb b/site/en/hub/tutorials/boundless.ipynb
index 570e9413362..f53fc5bb004 100644
--- a/site/en/hub/tutorials/boundless.ipynb
+++ b/site/en/hub/tutorials/boundless.ipynb
@@ -82,9 +82,9 @@
"id": "hDKbpAEZf8Lt"
},
"source": [
- "## Imports and Setup\n",
+ "## Imports and setup\n",
"\n",
- "Lets start with the base imports."
+ "Start with the base imports:"
]
},
{
@@ -110,9 +110,9 @@
"id": "pigUDIXtciQO"
},
"source": [
- "## Reading image for input\n",
+ "## Create a function for reading an image\n",
"\n",
- "Lets create a util method to help load the image and format it for the model (257x257x3). This method will also crop the image to a square to avoid distortion and you can use with local images or from the internet."
+ "Create a utility function to help load an image and format it for the model (257x257x3). This method will also crop the image to a square to avoid distortion and you can use it with local images or from the internet."
]
},
{
@@ -147,9 +147,9 @@
"id": "lonrLxuKcsL0"
},
"source": [
- "## Visualization method\n",
+ "## Create a visualization function\n",
"\n",
- "We will also create a visuzalization method to show the original image side by side with the masked version and the \"filled\" version, both generated by the model."
+ "Create a visualization function to show the original image side-by-side with the masked version and the \"filled\" version, both generated by the model."
]
},
{
@@ -183,9 +183,9 @@
"id": "8rwaCWmxdJGH"
},
"source": [
- "## Loading an Image\n",
+ "## Load an image\n",
"\n",
- "We will load a sample image but fell free to upload your own image to the colab and try with it. Remember that the model have some limitations regarding human images."
+ "Now you can load a sample image. Feel free to use your own image by uploading it to the Colab notebook. Remember that the model may have some limitations regarding human images."
]
},
{
@@ -210,10 +210,10 @@
"id": "4lIkmZL_dtyX"
},
"source": [
- "## Selecting a model from TensorFlow Hub\n",
+ "## Select a model from TensorFlow Hub\n",
"\n",
- "On TensorFlow Hub we have 3 versions of the Boundless model: Half, Quarter and Three Quarters.\n",
- "In the following cell you can chose any of them and try on your image. If you want to try with another one, just chose it and execute the following cells."
+ "On TensorFlow Hub there are three versions of the Boundless model: Half, Quarter and Three Quarters.\n",
+ "In the following cell you can choose any of the models and apply them on your image. If you want to pick another model, select it below and then run the following cells."
]
},
{
@@ -241,9 +241,9 @@
"id": "aSJFeNNSeOn8"
},
"source": [
- "Now that we've chosen the model we want, lets load it from TensorFlow Hub.\n",
+ "After choosing your model, you can load it from TensorFlow Hub.\n",
"\n",
- "**Note**: You can point your browser to the model handle to read the model's documentation."
+ "**Note**: You can point to a model handle to read the model's documentation."
]
},
{
@@ -264,14 +264,14 @@
"id": "L4G7CPOaeuQb"
},
"source": [
- "## Doing Inference\n",
+ "## Perform inference\n",
"\n",
- "The boundless model have two outputs:\n",
+ "The boundless model has two outputs:\n",
"\n",
"* The input image with a mask applied\n",
"* The masked image with the extrapolation to complete it\n",
"\n",
- "we can use these two images to show a comparisson visualization."
+ "You can compare these two images with a visualization as follows:"
]
},
{
diff --git a/site/en/hub/tutorials/cropnet_cassava.ipynb b/site/en/hub/tutorials/cropnet_cassava.ipynb
index 18f41c00da1..926b5395e41 100644
--- a/site/en/hub/tutorials/cropnet_cassava.ipynb
+++ b/site/en/hub/tutorials/cropnet_cassava.ipynb
@@ -199,7 +199,7 @@
"id": "QT3XWAtR6BRy"
},
"source": [
- "The *cassava* dataset has images of cassava leaves with 4 distinct diseases as well as healthy cassava leaves. The model can predict all of these classes as well as sixth class for \"unknown\" when the model is not confident in it's prediction."
+ "The *cassava* dataset has images of cassava leaves with 4 distinct diseases as well as healthy cassava leaves. The model can predict all of these classes as well as sixth class for \"unknown\" when the model is not confident in its prediction."
]
},
{
diff --git a/site/en/hub/tutorials/cross_lingual_similarity_with_tf_hub_multilingual_universal_encoder.ipynb b/site/en/hub/tutorials/cross_lingual_similarity_with_tf_hub_multilingual_universal_encoder.ipynb
index 31fc037dfe7..920d197811e 100644
--- a/site/en/hub/tutorials/cross_lingual_similarity_with_tf_hub_multilingual_universal_encoder.ipynb
+++ b/site/en/hub/tutorials/cross_lingual_similarity_with_tf_hub_multilingual_universal_encoder.ipynb
@@ -271,7 +271,7 @@
"spanish_sentences = ['perro', 'Los cachorros son agradables.', 'Disfruto de dar largos paseos por la playa con mi perro.']\n",
"\n",
"# Multilingual example\n",
- "multilingual_example = [\"Willkommen zu einfachen, aber\", \"verrassend krachtige\", \"multilingüe\", \"compréhension du langage naturel\", \"модели.\", \"大家是什么意思\" , \"보다 중요한\", \".اللغة التي يتحدثونها\"]\n",
+ "multilingual_example = [\"Willkommen zu einfachen, aber\", \"verrassend krachtige\", \"multilingüe\", \"compréhension du language naturel\", \"модели.\", \"大家是什么意思\" , \"보다 중요한\", \".اللغة التي يتحدثونها\"]\n",
"multilingual_example_in_en = [\"Welcome to simple yet\", \"surprisingly powerful\", \"multilingual\", \"natural language understanding\", \"models.\", \"What people mean\", \"matters more than\", \"the language they speak.\"]\n"
]
},
@@ -4174,7 +4174,7 @@
"id": "Dxu66S8wJIG9"
},
"source": [
- "### Semantic-search crosss-lingual capabilities\n",
+ "### Semantic-search cross-lingual capabilities\n",
"\n",
"In this section we show how to retrieve sentences related to a set of sample English sentences. Things to try:\n",
"\n",
diff --git a/site/en/hub/tutorials/image_enhancing.ipynb b/site/en/hub/tutorials/image_enhancing.ipynb
index 4c9496b79ae..3710ebd6d66 100644
--- a/site/en/hub/tutorials/image_enhancing.ipynb
+++ b/site/en/hub/tutorials/image_enhancing.ipynb
@@ -346,7 +346,7 @@
"cell_type": "code",
"execution_count": null,
"metadata": {
- "id": "r_dautO6qbTV"
+ "id": "r_defaultO6qbTV"
},
"outputs": [],
"source": [
diff --git a/site/en/hub/tutorials/image_feature_vector.ipynb b/site/en/hub/tutorials/image_feature_vector.ipynb
index 29ac0c97ddd..b5283c45b3d 100644
--- a/site/en/hub/tutorials/image_feature_vector.ipynb
+++ b/site/en/hub/tutorials/image_feature_vector.ipynb
@@ -357,7 +357,7 @@
"source": [
"## Train the network\n",
"\n",
- "Now that our model is built, let's train it and see how it perfoms on our test set."
+ "Now that our model is built, let's train it and see how it performs on our test set."
]
},
{
diff --git a/site/en/hub/tutorials/movenet.ipynb b/site/en/hub/tutorials/movenet.ipynb
index 2b6ffc6eb54..f7955a5253b 100644
--- a/site/en/hub/tutorials/movenet.ipynb
+++ b/site/en/hub/tutorials/movenet.ipynb
@@ -450,7 +450,7 @@
"id": "ymTVR2I9x22I"
},
"source": [
- "This session demonstrates the minumum working example of running the model on a **single image** to predict the 17 human keypoints."
+ "This session demonstrates the minimum working example of running the model on a **single image** to predict the 17 human keypoints."
]
},
{
@@ -697,7 +697,7 @@
" return output_image\n",
"\n",
"def run_inference(movenet, image, crop_region, crop_size):\n",
- " \"\"\"Runs model inferece on the cropped region.\n",
+ " \"\"\"Runs model inference on the cropped region.\n",
"\n",
" The function runs the model inference on the cropped region and updates the\n",
" model output to the original image coordinate system.\n",
diff --git a/site/en/hub/tutorials/movinet.ipynb b/site/en/hub/tutorials/movinet.ipynb
index 61609dbf72a..24600256cf9 100644
--- a/site/en/hub/tutorials/movinet.ipynb
+++ b/site/en/hub/tutorials/movinet.ipynb
@@ -890,7 +890,7 @@
" steps = video.shape[0]\n",
" # estimate duration of the video (in seconds)\n",
" duration = steps / video_fps\n",
- " # estiamte top_k probabilities and corresponding labels\n",
+ " # estimate top_k probabilities and corresponding labels\n",
" top_probs, top_labels, _ = get_top_k_streaming_labels(probs, k=top_k)\n",
"\n",
" images = []\n",
@@ -950,7 +950,7 @@
" logits, states = model({**states, 'image': image})\n",
" all_logits.append(logits)\n",
"\n",
- "# concatinating all the logits\n",
+ "# concatenating all the logits\n",
"logits = tf.concat(all_logits, 0)\n",
"# estimating probabilities\n",
"probs = tf.nn.softmax(logits, axis=-1)"
diff --git a/site/en/hub/tutorials/s3gan_generation_with_tf_hub.ipynb b/site/en/hub/tutorials/s3gan_generation_with_tf_hub.ipynb
index d8efd802ae0..bd73cffebdf 100644
--- a/site/en/hub/tutorials/s3gan_generation_with_tf_hub.ipynb
+++ b/site/en/hub/tutorials/s3gan_generation_with_tf_hub.ipynb
@@ -86,7 +86,7 @@
"2. Click **Runtime > Run all** to run each cell in order.\n",
" * Afterwards, the interactive visualizations should update automatically when you modify the settings using the sliders and dropdown menus.\n",
"\n",
- "Note: if you run into any issues, youn can try restarting the runtime and rerunning all cells from scratch by clicking **Runtime > Restart and run all...**.\n",
+ "Note: if you run into any issues, you can try restarting the runtime and rerunning all cells from scratch by clicking **Runtime > Restart and run all...**.\n",
"\n",
"[1] Mario Lucic\\*, Michael Tschannen\\*, Marvin Ritter\\*, Xiaohua Zhai, Olivier\n",
" Bachem, Sylvain Gelly, [High-Fidelity Image Generation With Fewer Labels](https://arxiv.org/abs/1903.02271), ICML 2019."
diff --git a/site/en/hub/tutorials/senteval_for_universal_sentence_encoder_cmlm.ipynb b/site/en/hub/tutorials/senteval_for_universal_sentence_encoder_cmlm.ipynb
index b152d3deee8..c33dce64c92 100644
--- a/site/en/hub/tutorials/senteval_for_universal_sentence_encoder_cmlm.ipynb
+++ b/site/en/hub/tutorials/senteval_for_universal_sentence_encoder_cmlm.ipynb
@@ -117,7 +117,7 @@
"id": "7a2ohPn8vMe2"
},
"source": [
- "#Execute a SentEval evaulation task\n",
+ "#Execute a SentEval evaluation task\n",
"The following code block executes a SentEval task and output the results, choose one of the following tasks to evaluate the USE CMLM model:\n",
"\n",
"```\n",
diff --git a/site/en/hub/tutorials/spice.ipynb b/site/en/hub/tutorials/spice.ipynb
index b58d07e46da..9ff6cd3bd62 100644
--- a/site/en/hub/tutorials/spice.ipynb
+++ b/site/en/hub/tutorials/spice.ipynb
@@ -658,7 +658,7 @@
"cell_type": "code",
"execution_count": null,
"metadata": {
- "id": "eMUTI4L52ZHA"
+ "id": "eMULTI4L52ZHA"
},
"outputs": [],
"source": [
diff --git a/site/en/hub/tutorials/text_cookbook.md b/site/en/hub/tutorials/text_cookbook.md
index 0ac9c6d6df3..dee9c1cf466 100644
--- a/site/en/hub/tutorials/text_cookbook.md
+++ b/site/en/hub/tutorials/text_cookbook.md
@@ -34,7 +34,7 @@ library for tokenization and preprocessing.
### Kaggle
-[IMDB classification on Kaggle](https://github.com/tensorflow/docs/blob/master/g3doc/en/hub/tutorials/text_classification_with_tf_hub_on_kaggle.ipynb) -
+[IMDB classification on Kaggle](https://github.com/tensorflow/docs/blob/master/site/en/hub/tutorials/text_classification_with_tf_hub_on_kaggle.ipynb) -
shows how to easily interact with a Kaggle competition from a Colab, including
downloading the data and submitting the results.
@@ -43,14 +43,14 @@ downloading the data and submitting the results.
[Text classification](https://www.tensorflow.org/hub/tutorials/text_classification_with_tf_hub) |  | | | | |
[Text classification with Keras](https://www.tensorflow.org/tutorials/keras/text_classification_with_hub) | |  |  |  | |
[Predicting Movie Review Sentiment with BERT on TF Hub](https://github.com/google-research/bert/blob/master/predicting_movie_reviews_with_bert_on_tf_hub.ipynb) |  | | | |  |
-[IMDB classification on Kaggle](https://github.com/tensorflow/docs/blob/master/g3doc/en/hub/tutorials/text_classification_with_tf_hub_on_kaggle.ipynb) |  | | | | | 
+[IMDB classification on Kaggle](https://github.com/tensorflow/docs/blob/master/site/en/hub/tutorials/text_classification_with_tf_hub_on_kaggle.ipynb) |  | | | | | 
### Bangla task with FastText embeddings
TensorFlow Hub does not currently offer a module in every language. The
following tutorial shows how to leverage TensorFlow Hub for fast experimentation
and modular ML development.
-[Bangla Article Classifier](https://github.com/tensorflow/docs/blob/master/g3doc/en/hub/tutorials/bangla_article_classifier.ipynb) -
+[Bangla Article Classifier](https://github.com/tensorflow/docs/blob/master/site/en/hub/tutorials/bangla_article_classifier.ipynb) -
demonstrates how to create a reusable TensorFlow Hub text embedding, and use it
to train a Keras classifier for
[BARD Bangla Article dataset](https://github.com/tanvirfahim15/BARD-Bangla-Article-Classifier).
@@ -64,24 +64,24 @@ setup (no training examples).
### Basic
-[Semantic similarity](https://github.com/tensorflow/docs/blob/master/g3doc/en/hub/tutorials/semantic_similarity_with_tf_hub_universal_encoder.ipynb) -
+[Semantic similarity](https://github.com/tensorflow/docs/blob/master/site/en/hub/tutorials/semantic_similarity_with_tf_hub_universal_encoder.ipynb) -
shows how to use the sentence encoder module to compute sentence similarity.
### Cross-lingual
-[Cross-lingual semantic similarity](https://github.com/tensorflow/docs/blob/master/g3doc/en/hub/tutorials/cross_lingual_similarity_with_tf_hub_multilingual_universal_encoder.ipynb) -
+[Cross-lingual semantic similarity](https://github.com/tensorflow/docs/blob/master/site/en/hub/tutorials/cross_lingual_similarity_with_tf_hub_multilingual_universal_encoder.ipynb) -
shows how to use one of the cross-lingual sentence encoders to compute sentence
similarity across languages.
### Semantic retrieval
-[Semantic retrieval](https://github.com/tensorflow/docs/blob/master/g3doc/en/hub/tutorials/retrieval_with_tf_hub_universal_encoder_qa.ipynb) -
+[Semantic retrieval](https://github.com/tensorflow/docs/blob/master/site/en/hub/tutorials/retrieval_with_tf_hub_universal_encoder_qa.ipynb) -
shows how to use Q/A sentence encoder to index a collection of documents for
retrieval based on semantic similarity.
### SentencePiece input
-[Semantic similarity with universal encoder lite](https://github.com/tensorflow/docs/blob/master/g3doc/en/hub/tutorials/semantic_similarity_with_tf_hub_universal_encoder_lite.ipynb) -
+[Semantic similarity with universal encoder lite](https://github.com/tensorflow/docs/blob/master/site/en/hub/tutorials/semantic_similarity_with_tf_hub_universal_encoder_lite.ipynb) -
shows how to use sentence encoder modules that accept
[SentencePiece](https://github.com/google/sentencepiece) ids on input instead of
text.
diff --git a/site/en/hub/tutorials/tf2_object_detection.ipynb b/site/en/hub/tutorials/tf2_object_detection.ipynb
index 38b162068d9..d06ad401824 100644
--- a/site/en/hub/tutorials/tf2_object_detection.ipynb
+++ b/site/en/hub/tutorials/tf2_object_detection.ipynb
@@ -291,7 +291,7 @@
"id": "yX3pb_pXDjYA"
},
"source": [
- "Intalling the Object Detection API"
+ "Installing the Object Detection API"
]
},
{
@@ -554,7 +554,7 @@
"\n",
"Among the available object detection models there's Mask R-CNN and the output of this model allows instance segmentation.\n",
"\n",
- "To visualize it we will use the same method we did before but adding an aditional parameter: `instance_masks=output_dict.get('detection_masks_reframed', None)`\n"
+ "To visualize it we will use the same method we did before but adding an additional parameter: `instance_masks=output_dict.get('detection_masks_reframed', None)`\n"
]
},
{
diff --git a/site/en/hub/tutorials/tf_hub_generative_image_module.ipynb b/site/en/hub/tutorials/tf_hub_generative_image_module.ipynb
index 4669f3b2dc3..4937bc2eb22 100644
--- a/site/en/hub/tutorials/tf_hub_generative_image_module.ipynb
+++ b/site/en/hub/tutorials/tf_hub_generative_image_module.ipynb
@@ -421,7 +421,7 @@
"If image is from the module space, the descent is quick and converges to a reasonable sample. Try out descending to an image that is **not from the module space**. The descent will only converge if the image is reasonably close to the space of training images.\n",
"\n",
"How to make it descend faster and to a more realistic image? One can try:\n",
- "* using different loss on the image difference, e.g. quadratic,\n",
+ "* using different loss on the image difference, e.g., quadratic,\n",
"* using different regularizer on the latent vector,\n",
"* initializing from a random vector in multiple runs,\n",
"* etc.\n"
diff --git a/site/en/hub/tutorials/wiki40b_lm.ipynb b/site/en/hub/tutorials/wiki40b_lm.ipynb
index e696160faca..ad94ce0aab8 100644
--- a/site/en/hub/tutorials/wiki40b_lm.ipynb
+++ b/site/en/hub/tutorials/wiki40b_lm.ipynb
@@ -214,7 +214,7 @@
" # Generate the tokens from the language model\n",
" generation_outputs = module(generation_input_dict, signature=\"prediction\", as_dict=True)\n",
"\n",
- " # Get the probablities and the inputs for the next steps\n",
+ " # Get the probabilities and the inputs for the next steps\n",
" probs = generation_outputs[\"probs\"]\n",
" new_mems = [generation_outputs[\"new_mem_{}\".format(i)] for i in range(n_layer)]\n",
"\n",
diff --git a/site/en/install/docker.md b/site/en/install/docker.md
index 8a2b1347668..836d771c31e 100644
--- a/site/en/install/docker.md
+++ b/site/en/install/docker.md
@@ -1,36 +1,36 @@
# Docker
-[Docker](https://docs.docker.com/install/){:.external} uses *containers* to
+[Docker](https://docs.docker.com/install/) uses *containers* to
create virtual environments that isolate a TensorFlow installation from the rest
of the system. TensorFlow programs are run *within* this virtual environment that
can share resources with its host machine (access directories, use the GPU,
connect to the Internet, etc.). The
-[TensorFlow Docker images](https://hub.docker.com/r/tensorflow/tensorflow/){:.external}
+[TensorFlow Docker images](https://hub.docker.com/r/tensorflow/tensorflow/)
are tested for each release.
Docker is the easiest way to enable TensorFlow [GPU support](./pip.md) on Linux since only the
-[NVIDIA® GPU driver](https://github.com/NVIDIA/nvidia-docker/wiki/Frequently-Asked-Questions#how-do-i-install-the-nvidia-driver){:.external}
+[NVIDIA® GPU driver](https://github.com/NVIDIA/nvidia-docker/wiki/Frequently-Asked-Questions#how-do-i-install-the-nvidia-driver)
is required on the *host* machine (the *NVIDIA® CUDA® Toolkit* does not need to
be installed).
## TensorFlow Docker requirements
-1. [Install Docker](https://docs.docker.com/install/){:.external} on
+1. [Install Docker](https://docs.docker.com/install/) on
your local *host* machine.
-2. For GPU support on Linux, [install NVIDIA Docker support](https://github.com/NVIDIA/nvidia-docker){:.external}.
+2. For GPU support on Linux, [install NVIDIA Docker support](https://github.com/NVIDIA/nvidia-docker).
* Take note of your Docker version with `docker -v`. Versions __earlier than__ 19.03 require nvidia-docker2 and the `--runtime=nvidia` flag. On versions __including and after__ 19.03, you will use the `nvidia-container-toolkit` package and the `--gpus all` flag. Both options are documented on the page linked above.
Note: To run the `docker` command without `sudo`, create the `docker` group and
add your user. For details, see the
-[post-installation steps for Linux](https://docs.docker.com/install/linux/linux-postinstall/){:.external}.
+[post-installation steps for Linux](https://docs.docker.com/install/linux/linux-postinstall/).
## Download a TensorFlow Docker image
The official TensorFlow Docker images are located in the
-[tensorflow/tensorflow](https://hub.docker.com/r/tensorflow/tensorflow/){:.external}
-Docker Hub repository. Image releases [are tagged](https://hub.docker.com/r/tensorflow/tensorflow/tags/){:.external}
+[tensorflow/tensorflow](https://hub.docker.com/r/tensorflow/tensorflow/)
+Docker Hub repository. Image releases [are tagged](https://hub.docker.com/r/tensorflow/tensorflow/tags/)
using the following format:
| Tag | Description |
@@ -64,7 +64,7 @@ To start a TensorFlow-configured container, use the following command form:
docker run [-it] [--rm] [-p hostPort:containerPort] tensorflow/tensorflow[:tag] [command]
-For details, see the [docker run reference](https://docs.docker.com/engine/reference/run/){:.external}.
+For details, see the [docker run reference](https://docs.docker.com/engine/reference/run/).
### Examples using CPU-only images
@@ -98,7 +98,7 @@ docker run -it --rm -v $PWD:/tmp -w /tmp tensorflow/tensorflow python ./script.p
Permission issues can arise when files created within a container are exposed to
the host. It's usually best to edit files on the host system.
-Start a [Jupyter Notebook](https://jupyter.org/){:.external} server using
+Start a [Jupyter Notebook](https://jupyter.org/) server using
TensorFlow's nightly build:
@@ -112,13 +112,13 @@ Follow the instructions and open the URL in your host web browser:
## GPU support
Docker is the easiest way to run TensorFlow on a GPU since the *host* machine
-only requires the [NVIDIA® driver](https://github.com/NVIDIA/nvidia-docker/wiki/Frequently-Asked-Questions#how-do-i-install-the-nvidia-driver){:.external}
+only requires the [NVIDIA® driver](https://github.com/NVIDIA/nvidia-docker/wiki/Frequently-Asked-Questions#how-do-i-install-the-nvidia-driver)
(the *NVIDIA® CUDA® Toolkit* is not required).
-Install the [Nvidia Container Toolkit](https://github.com/NVIDIA/nvidia-docker/blob/master/README.md#quickstart){:.external}
+Install the [Nvidia Container Toolkit](https://github.com/NVIDIA/nvidia-docker/blob/master/README.md#quickstart)
to add NVIDIA® GPU support to Docker. `nvidia-container-runtime` is only
available for Linux. See the `nvidia-container-runtime`
-[platform support FAQ](https://github.com/NVIDIA/nvidia-docker/wiki/Frequently-Asked-Questions#platform-support){:.external}
+[platform support FAQ](https://github.com/NVIDIA/nvidia-docker/wiki/Frequently-Asked-Questions#platform-support)
for details.
Check if a GPU is available:
diff --git a/site/en/install/errors.md b/site/en/install/errors.md
index d1ad9e50af5..938ba8b454f 100644
--- a/site/en/install/errors.md
+++ b/site/en/install/errors.md
@@ -1,8 +1,8 @@
# Build and install error messages
-TensorFlow uses [GitHub issues](https://github.com/tensorflow/tensorflow/issues){:.external},
-[Stack Overflow](https://stackoverflow.com/questions/tagged/tensorflow){:.external} and
-[TensorFlow Forum](https://discuss.tensorflow.org/c/general-discussion/6){:.external}
+TensorFlow uses [GitHub issues](https://github.com/tensorflow/tensorflow/issues),
+[Stack Overflow](https://stackoverflow.com/questions/tagged/tensorflow) and
+[TensorFlow Forum](https://discuss.tensorflow.org/c/general-discussion/6)
to track, document, and discuss build and installation problems.
The following list links error messages to a solution or discussion. If you find
diff --git a/site/en/install/gpu_plugins.md b/site/en/install/gpu_plugins.md
index 5abfbc1ef03..39e3cf09b29 100644
--- a/site/en/install/gpu_plugins.md
+++ b/site/en/install/gpu_plugins.md
@@ -4,7 +4,7 @@ Note: This page is for non-NVIDIA® GPU devices. For NVIDIA® GPU support, go to
the [Install TensorFlow with pip](./pip.md) guide.
TensorFlow's
-[pluggable device](https://github.com/tensorflow/community/blob/master/rfcs/20200624-pluggable-device-for-tensorflow.md){:.external}
+[pluggable device](https://github.com/tensorflow/community/blob/master/rfcs/20200624-pluggable-device-for-tensorflow.md)
architecture adds new device support as separate plug-in packages that are
installed alongside the official TensorFlow package.
@@ -58,23 +58,23 @@ run() # PluggableDevices also work with tf.function and graph mode.
Metal `PluggableDevice` for macOS GPUs:
* Works with TF 2.5 or later.
-* [Getting started guide](https://developer.apple.com/metal/tensorflow-plugin/){:.external}.
+* [Getting started guide](https://developer.apple.com/metal/tensorflow-plugin/).
* For questions and feedback, please visit the
- [Apple Developer Forum](https://developer.apple.com/forums/tags/tensorflow-metal){:.external}.
+ [Apple Developer Forum](https://developer.apple.com/forums/tags/tensorflow-metal).
DirectML `PluggableDevice` for Windows and WSL (preview):
* Works with `tensorflow-cpu` package, version 2.10 or later.
-* [PyPI wheel](https://pypi.org/project/tensorflow-directml-plugin/){:.external}.
-* [GitHub repo](https://github.com/microsoft/tensorflow-directml-plugin){:.external}.
+* [PyPI wheel](https://pypi.org/project/tensorflow-directml-plugin/).
+* [GitHub repo](https://github.com/microsoft/tensorflow-directml-plugin).
* For questions, feedback or to raise issues, please visit the
- [Issues page of `tensorflow-directml-plugin` on GitHub](https://github.com/microsoft/tensorflow-directml-plugin/issues){:.external}.
+ [Issues page of `tensorflow-directml-plugin` on GitHub](https://github.com/microsoft/tensorflow-directml-plugin/issues).
Intel® Extension for TensorFlow `PluggableDevice` for Linux and WSL:
* Works with TF 2.10 or later.
* [Getting started guide](https://intel.github.io/intel-extension-for-tensorflow/latest/get_started.html)
-* [PyPI wheel](https://pypi.org/project/intel-extension-for-tensorflow/){:.external}.
-* [GitHub repo](https://github.com/intel/intel-extension-for-tensorflow){:.external}.
+* [PyPI wheel](https://pypi.org/project/intel-extension-for-tensorflow/).
+* [GitHub repo](https://github.com/intel/intel-extension-for-tensorflow).
* For questions, feedback, or to raise issues, please visit the
- [Issues page of `intel-extension-for-tensorflow` on GitHub](https://github.com/intel/intel-extension-for-tensorflow/issues){:.external}.
+ [Issues page of `intel-extension-for-tensorflow` on GitHub](https://github.com/intel/intel-extension-for-tensorflow/issues).
diff --git a/site/en/install/lang_c.ipynb b/site/en/install/lang_c.ipynb
index cfff20db10b..240cfc29865 100644
--- a/site/en/install/lang_c.ipynb
+++ b/site/en/install/lang_c.ipynb
@@ -130,16 +130,23 @@
"
\n",
@@ -178,8 +185,8 @@
"outputs": [],
"source": [
"%%bash\n",
- "FILENAME=libtensorflow-cpu-linux-x86_64-2.15.0.tar.gz\n",
- "wget -q --no-check-certificate https://storage.googleapis.com/tensorflow/libtensorflow/${FILENAME}\n",
+ "FILENAME=libtensorflow-cpu-linux-x86_64.tar.gz\n",
+ "wget -q --no-check-certificate https://storage.googleapis.com/tensorflow/versions/2.17.0/${FILENAME}\n",
"sudo tar -C /usr/local -xzf ${FILENAME}"
]
},
@@ -348,7 +355,7 @@
"## Build from source\n",
"\n",
"TensorFlow is open source. Read\n",
- "[the instructions](https://github.com/tensorflow/tensorflow/blob/master/tensorflow/tools/lib_package/README.md){:.external}\n",
+ "[the instructions](https://github.com/tensorflow/tensorflow/blob/master/tensorflow/tools/lib_package/README.md)\n",
"to build TensorFlow's C library from source code."
]
}
diff --git a/site/en/install/lang_java_legacy.md b/site/en/install/lang_java_legacy.md
index 3a2e121cfc7..37341c36659 100644
--- a/site/en/install/lang_java_legacy.md
+++ b/site/en/install/lang_java_legacy.md
@@ -27,7 +27,7 @@ To use TensorFlow on Android see [TensorFlow Lite](https://tensorflow.org/lite)
## TensorFlow with Apache Maven
-To use TensorFlow with [Apache Maven](https://maven.apache.org){:.external},
+To use TensorFlow with [Apache Maven](https://maven.apache.org),
add the dependency to the project's `pom.xml` file:
```xml
@@ -167,7 +167,7 @@ system and processor support:
Note: On Windows, the native library (`tensorflow_jni.dll`) requires
`msvcp140.dll` at runtime. See the
[Windows build from source](./source_windows.md) guide to install the
-[Visual C++ 2019 Redistributable](https://visualstudio.microsoft.com/vs/){:.external}.
+[Visual C++ 2019 Redistributable](https://visualstudio.microsoft.com/vs/).
### Compile
@@ -203,5 +203,5 @@ Success: TensorFlow for Java is configured.
## Build from source
TensorFlow is open source. Read
-[the instructions](https://github.com/tensorflow/tensorflow/blob/master/tensorflow/java/README.md){:.external}
+[the instructions](https://github.com/tensorflow/tensorflow/blob/master/tensorflow/java/README.md)
to build TensorFlow's Java and native libraries from source code.
diff --git a/site/en/install/pip.md b/site/en/install/pip.md
index 4add60b11d7..2ac105e10d3 100644
--- a/site/en/install/pip.md
+++ b/site/en/install/pip.md
@@ -62,11 +62,11 @@ step-by-step instructions.
Note: TensorFlow with GPU access is supported for WSL2 on Windows 10 19044 or
higher. This corresponds to Windows 10 version 21H2, the November 2021
update. You can get the latest update from here:
- [Download Windows 10](https://www.microsoft.com/software-download/windows10){:.external}.
+ [Download Windows 10](https://www.microsoft.com/software-download/windows10).
For instructions, see
- [Install WSL2](https://docs.microsoft.com/windows/wsl/install){:.external}
+ [Install WSL2](https://docs.microsoft.com/windows/wsl/install)
and
- [NVIDIA’s setup docs](https://docs.nvidia.com/cuda/wsl-user-guide/index.html){:.external}
+ [NVIDIA’s setup docs](https://docs.nvidia.com/cuda/wsl-user-guide/index.html)
for CUDA in WSL.
```bash
@@ -108,14 +108,14 @@ step-by-step instructions.
## Hardware requirements
Note: TensorFlow binaries use
-[AVX instructions](https://en.wikipedia.org/wiki/Advanced_Vector_Extensions#CPUs_with_AVX){:.external}
+[AVX instructions](https://en.wikipedia.org/wiki/Advanced_Vector_Extensions#CPUs_with_AVX)
which may not run on older CPUs.
The following GPU-enabled devices are supported:
* NVIDIA® GPU card with CUDA® architectures 3.5, 5.0, 6.0, 7.0, 7.5, 8.0 and
higher. See the list of
- [CUDA®-enabled GPU cards](https://developer.nvidia.com/cuda-gpus){:.external}.
+ [CUDA®-enabled GPU cards](https://developer.nvidia.com/cuda-gpus).
* For GPUs with unsupported CUDA® architectures, or to avoid JIT compilation
from PTX, or to use different versions of the NVIDIA® libraries, see the
[Linux build from source](./source.md) guide.
@@ -123,7 +123,7 @@ The following GPU-enabled devices are supported:
architecture; therefore, TensorFlow fails to load on older GPUs when
`CUDA_FORCE_PTX_JIT=1` is set. (See
[Application Compatibility](https://docs.nvidia.com/cuda/cuda-c-programming-guide/index.html#application-compatibility)
- for details.) {:.external}
+ for details.)
Note: The error message "Status: device kernel image is invalid" indicates that
the TensorFlow package does not contain PTX for your architecture. You can
@@ -140,21 +140,22 @@ Note: GPU support is available for Ubuntu and Windows with CUDA®-enabled cards.
## Software requirements
-* Python 3.9–3.11
+* Python 3.9–3.12
* pip version 19.0 or higher for Linux (requires `manylinux2014` support) and
Windows. pip version 20.3 or higher for macOS.
* Windows Native Requires
- [Microsoft Visual C++ Redistributable for Visual Studio 2015, 2017 and 2019](https://support.microsoft.com/help/2977003/the-latest-supported-visual-c-downloads){:.external}
+ [Microsoft Visual C++ Redistributable for Visual Studio 2015, 2017 and 2019](https://support.microsoft.com/help/2977003/the-latest-supported-visual-c-downloads)
The following NVIDIA® software are only required for GPU support.
-* [NVIDIA® GPU drivers](https://www.nvidia.com/drivers){:.external}
- version 450.80.02 or higher.
-* [CUDA® Toolkit 11.8](https://developer.nvidia.com/cuda-toolkit-archive){:.external}.
-* [cuDNN SDK 8.6.0](https://developer.nvidia.com/cudnn){:.external}.
+* [NVIDIA® GPU drivers](https://www.nvidia.com/drivers)
+ * >= 525.60.13 for Linux
+ * >= 528.33 for WSL on Windows
+* [CUDA® Toolkit 12.3](https://developer.nvidia.com/cuda-toolkit-archive).
+* [cuDNN SDK 8.9.7](https://developer.nvidia.com/cudnn).
* *(Optional)*
- [TensorRT](https://docs.nvidia.com/deeplearning/tensorrt/archives/index.html#trt_7){:.external}
+ [TensorRT](https://docs.nvidia.com/deeplearning/tensorrt/archives/index.html#trt_7)
to improve latency and throughput for inference.
## Step-by-step instructions
@@ -165,7 +166,7 @@ The following NVIDIA® software are only required for GPU support.
* Ubuntu 16.04 or higher (64-bit)
- TensorFlow only officially support Ubuntu. However, the following
+ TensorFlow only officially supports Ubuntu. However, the following
instructions may also work for other Linux distros.
Note: Starting with TensorFlow `2.10`, Linux CPU-builds for Aarch64/ARM64
@@ -185,7 +186,7 @@ The following NVIDIA® software are only required for GPU support.
You can skip this section if you only run TensorFlow on the CPU.
Install the
- [NVIDIA GPU driver](https://www.nvidia.com/Download/index.aspx){:.external}
+ [NVIDIA GPU driver](https://www.nvidia.com/Download/index.aspx)
if you have not. You can use the following command to verify it is
installed.
@@ -316,25 +317,25 @@ The following NVIDIA® software are only required for GPU support.
with *Visual Studio 2019* but can be installed separately:
1. Go to the
- [Microsoft Visual C++ downloads](https://support.microsoft.com/help/2977003/the-latest-supported-visual-c-downloads){:.external}.
+ [Microsoft Visual C++ downloads](https://support.microsoft.com/help/2977003/the-latest-supported-visual-c-downloads).
2. Scroll down the page to the *Visual Studio 2015, 2017 and 2019* section.
3. Download and install the *Microsoft Visual C++ Redistributable for
Visual Studio 2015, 2017 and 2019* for your platform.
Make sure
- [long paths are enabled](https://superuser.com/questions/1119883/windows-10-enable-ntfs-long-paths-policy-option-missing){:.external}
+ [long paths are enabled](https://superuser.com/questions/1119883/windows-10-enable-ntfs-long-paths-policy-option-missing)
on Windows.
### 3. Install Miniconda
- [Miniconda](https://docs.conda.io/en/latest/miniconda.html){:.external}
+ [Miniconda](https://docs.conda.io/en/latest/miniconda.html)
is the recommended approach for installing TensorFlow with GPU support.
It creates a separate environment to avoid changing any installed
software in your system. This is also the easiest way to install the
required software especially for the GPU setup.
Download the
- [Miniconda Windows Installer](https://repo.anaconda.com/miniconda/Miniconda3-latest-Windows-x86_64.exe){:.external}.
+ [Miniconda Windows Installer](https://repo.anaconda.com/miniconda/Miniconda3-latest-Windows-x86_64.exe).
Double-click the downloaded file and follow the instructions on the screen.
### 4. Create a conda environment
@@ -359,7 +360,7 @@ The following NVIDIA® software are only required for GPU support.
You can skip this section if you only run TensorFlow on CPU.
First install
- [NVIDIA GPU driver](https://www.nvidia.com/Download/index.aspx){:.external}
+ [NVIDIA GPU driver](https://www.nvidia.com/Download/index.aspx)
if you have not.
Then install the CUDA, cuDNN with conda.
@@ -416,16 +417,16 @@ The following NVIDIA® software are only required for GPU support.
See the following documents to:
- * [Download the latest Windows 10 update](https://www.microsoft.com/software-download/windows10){:.external}.
- * [Install WSL2](https://docs.microsoft.com/windows/wsl/install){:.external}
- * [Setup NVIDIA® GPU support in WSL2](https://docs.nvidia.com/cuda/wsl-user-guide/index.html){:.external}
+ * [Download the latest Windows 10 update](https://www.microsoft.com/software-download/windows10).
+ * [Install WSL2](https://docs.microsoft.com/windows/wsl/install)
+ * [Setup NVIDIA® GPU support in WSL2](https://docs.nvidia.com/cuda/wsl-user-guide/index.html)
### 2. GPU setup
You can skip this section if you only run TensorFlow on the CPU.
Install the
- [NVIDIA GPU driver](https://www.nvidia.com/Download/index.aspx){:.external}
+ [NVIDIA GPU driver](https://www.nvidia.com/Download/index.aspx)
if you have not. You can use the following command to verify it is
installed.
@@ -478,58 +479,111 @@ The value you specify depends on your Python version.
Note: A `pip` version >19.0 is required to install the TensorFlow 2 `.whl`
@@ -60,7 +59,7 @@ file.
Clang is a C/C++/Objective-C compiler that is compiled in C++ based on LLVM. It
is the default compiler to build TensorFlow starting with TensorFlow 2.13. The
-current supported version is LLVM/Clang 16.
+current supported version is LLVM/Clang 17.
[LLVM Debian/Ubuntu nightly packages](https://apt.llvm.org) provide an automatic
installation script and packages for manual installation on Linux. Make sure you
@@ -68,50 +67,58 @@ run the following command if you manually add llvm apt repository to your
package sources:
+Now that `/usr/lib/llvm-17/bin/clang` is the actual path to clang in this case.
+
Alternatively, you can download and unpack the pre-built
-[Clang + LLVM 16](https://github.com/llvm/llvm-project/releases/tag/llvmorg-16.0.0).
+[Clang + LLVM 17](https://github.com/llvm/llvm-project/releases/tag/llvmorg-17.0.2).
-Below is an example of steps you can take to set up the downloaded
-Clang + LLVM 16 binaries:
+Below is an example of steps you can take to set up the downloaded Clang + LLVM
+17 binaries on Debian/Ubuntu operating systems:
-1. Change to the desired destination directory:
- ```cd ```
+1. Change to the desired destination directory: `cd `
-2. Load and extract an archive file...(suitable to your architecture):
+1. Load and extract an archive file...(suitable to your architecture):
-
- wget https://github.com/llvm/llvm-project/releases/download/llvmorg-16.0.0/clang+llvm-16.0.0-x86_64-linux-gnu-ubuntu-18.04.tar.xz
+ wget https://github.com/llvm/llvm-project/releases/download/llvmorg-17.0.2/clang+llvm-17.0.2-x86_64-linux-gnu-ubuntu-22.04.tar.xz
- tar -xvf clang+llvm-16.0.0-x86_64-linux-gnu-ubuntu-18.04.tar.xz
+ tar -xvf clang+llvm-17.0.2-x86_64-linux-gnu-ubuntu-22.04.tar.xz
-3. Check the obtained Clang + LLVM 16 binaries version:
+1. Copy the extracted contents (directories and files) to `/usr` (you may need
+ sudo permissions, and the correct directory may vary by distribution). This
+ effectively installs Clang and LLVM, and adds it to the path. You should not
+ have to replace anything, unless you have a previous installation, in which
+ case you should replace the files:
+
-4. Directory `/clang+llvm-16.0.0-x86_64-linux-gnu-ubuntu-18.04/bin/clang-16` is
- the actual path to your new clang. You can run the `./configure` script or
- manually set environment variables `CC` and `BAZEL_COMPILER` to this path.
+1. Now that `/usr/bin/clang` is the actual path to your new clang. You can run
+ the `./configure` script or manually set environment variables `CC` and
+ `BAZEL_COMPILER` to this path.
### Install GPU support (optional, Linux only)
There is *no* GPU support for macOS.
-Read the [GPU support](./gpu.md) guide to install the drivers and additional
+Read the [GPU support](./pip.md) guide to install the drivers and additional
software required to run TensorFlow on a GPU.
Note: It is easier to set up one of TensorFlow's GPU-enabled [Docker images](#docker_linux_builds).
### Download the TensorFlow source code
-Use [Git](https://git-scm.com/){:.external} to clone the
-[TensorFlow repository](https://github.com/tensorflow/tensorflow){:.external}:
+Use [Git](https://git-scm.com/) to clone the
+[TensorFlow repository](https://github.com/tensorflow/tensorflow):
git clone https://github.com/tensorflow/tensorflow.git
@@ -119,7 +126,7 @@ Use [Git](https://git-scm.com/){:.external} to clone the
The repo defaults to the `master` development branch. You can also check out a
-[release branch](https://github.com/tensorflow/tensorflow/releases){:.external}
+[release branch](https://github.com/tensorflow/tensorflow/releases)
to build:
@@ -204,7 +211,14 @@ Preconfigured Bazel build configs to DISABLE default on features:
#### GPU support
-For [GPU support](./gpu.md), set `cuda=Y` during configuration and specify the
+##### from v.2.18.0
+For [GPU support](./pip.md), set `cuda=Y` during configuration and specify the
+versions of CUDA and cuDNN if required. Bazel will download CUDA and CUDNN
+packages automatically or point to CUDA/CUDNN/NCCL redistributions on local file
+system if required.
+
+##### before v.2.18.0
+For [GPU support](./pip.md), set `cuda=Y` during configuration and specify the
versions of CUDA and cuDNN. If your system has multiple versions of CUDA or
cuDNN installed, explicitly set the version instead of relying on the default.
`./configure` creates symbolic links to your system's CUDA libraries—so if you
@@ -216,7 +230,7 @@ building.
For compilation optimization flags, the default (`-march=native`) optimizes the
generated code for your machine's CPU type. However, if building TensorFlow for
a different CPU type, consider a more specific optimization flag. Check the
-[GCC manual](https://gcc.gnu.org/onlinedocs/gcc-4.5.3/gcc/i386-and-x86_002d64-Options.html){:.external}
+[GCC manual](https://gcc.gnu.org/onlinedocs/gcc-4.5.3/gcc/i386-and-x86_002d64-Options.html)
for examples.
#### Preconfigured configurations
@@ -228,25 +242,12 @@ There are some preconfigured build configs available that can be added to the
[CONTRIBUTING.md](https://github.com/tensorflow/tensorflow/blob/master/CONTRIBUTING.md)
for details.
* `--config=mkl` —Support for the
- [Intel® MKL-DNN](https://github.com/intel/mkl-dnn){:.external}.
+ [Intel® MKL-DNN](https://github.com/intel/mkl-dnn).
* `--config=monolithic` —Configuration for a mostly static, monolithic build.
## Build and install the pip package
-The pip package is build in two steps. A `bazel build` commands creates a
-"package-builder" program. You then run the package-builder to create the
-package.
-
-### Build the package-builder
-Note: GPU support can be enabled with `cuda=Y` during the `./configure` stage.
-
-Use `bazel build` to create the TensorFlow 2.x package-builder:
-
-
-
#### Bazel build options
Refer to the Bazel
@@ -262,25 +263,34 @@ that complies with the manylinux2014 package standard.
### Build the package
-The `bazel build` command creates an executable named `build_pip_package`—this
-is the program that builds the `pip` package. Run the executable as shown
-below to build a `.whl` package in the `/tmp/tensorflow_pkg` directory.
+To build pip package, you need to specify `--repo_env=WHEEL_NAME` flag.
+depending on the provided name, package will be created, e.g:
-To build from a release branch:
+To build tensorflow CPU package:
+
-Although it is possible to build both CUDA and non-CUDA configurations under the
-same source tree, it's recommended to run `bazel clean` when switching between
-these two configurations in the same source tree.
+As a result, generated wheel will be located in
+
### Install the package
@@ -288,7 +298,7 @@ The filename of the generated `.whl` file depends on the TensorFlow version and
your platform. Use `pip install` to install the package, for example:
Success: TensorFlow is now installed.
@@ -300,7 +310,7 @@ TensorFlow's Docker development images are an easy way to set up an environment
to build Linux packages from source. These images already contain the source
code and dependencies required to build TensorFlow. Go to the TensorFlow
[Docker guide](./docker.md) for installation instructions and the
-[list of available image tags](https://hub.docker.com/r/tensorflow/tensorflow/tags/){:.external}.
+[list of available image tags](https://hub.docker.com/r/tensorflow/tensorflow/tags/).
### CPU-only
@@ -336,20 +346,17 @@ docker run -it -w /tensorflow -v /path/to/tensorflow:/tensorflow -v $
With the source tree set up, build the TensorFlow package within the container's
virtual environment:
-1. Optional: Configure the build—this prompts the user to answer build configuration
- questions.
-2. Build the tool used to create the *pip* package.
-3. Run the tool to create the *pip* package.
-4. Adjust the ownership permissions of the file for outside the container.
+1. Optional: Configure the build—this prompts the user to answer build
+ configuration questions.
+2. Build the *pip* package.
+3. Adjust the ownership permissions of the file for outside the container.
Install and verify the package within the container:
@@ -357,7 +364,7 @@ Install and verify the package within the container:
pip uninstall tensorflow # remove current version
-pip install /mnt/tensorflow-version-tags.whl
+pip install bazel-bin/tensorflow/tools/pip_package/wheel_house/tensorflow-version-tags.whlcd /tmp # don't import from source directorypython -c "import tensorflow as tf; print(tf.__version__)"
@@ -370,12 +377,15 @@ On your host machine, the TensorFlow *pip* package is in the current directory
### GPU support
+Note: Starting from Tensorflow v.2.18.0 the wheels can be built from
+source on a machine without GPUs and without NVIDIA driver installed.
+
Docker is the easiest way to build GPU support for TensorFlow since the *host*
machine only requires the
-[NVIDIA® driver](https://github.com/NVIDIA/nvidia-docker/wiki/Frequently-Asked-Questions#how-do-i-install-the-nvidia-driver){:.external}
+[NVIDIA® driver](https://github.com/NVIDIA/nvidia-docker/wiki/Frequently-Asked-Questions#how-do-i-install-the-nvidia-driver)
(the *NVIDIA® CUDA® Toolkit* doesn't have to be installed). Refer to the
-[GPU support guide](./gpu.md) and the TensorFlow [Docker guide](./docker.md) to
-set up [nvidia-docker](https://github.com/NVIDIA/nvidia-docker){:.external}
+[GPU support guide](./pip.md) and the TensorFlow [Docker guide](./docker.md) to
+set up [nvidia-docker](https://github.com/NVIDIA/nvidia-docker)
(Linux only).
The following example downloads the TensorFlow `:devel-gpu` image and uses
@@ -395,11 +405,9 @@ with GPU support:
Install and verify the package within the container and check for a GPU:
@@ -407,7 +415,7 @@ Install and verify the package within the container and check for a GPU:
pip uninstall tensorflow # remove current version
-pip install /mnt/tensorflow-version-tags.whl
+pip install bazel-bin/tensorflow/tools/pip_package/wheel_house/tensorflow-version-tags.whlcd /tmp # don't import from source directorypython -c "import tensorflow as tf; print(\"Num GPUs Available: \", len(tf.config.list_physical_devices('GPU')))"
@@ -424,6 +432,8 @@ Success: TensorFlow is now installed.
Version
Python version
Compiler
Build tools
+
tensorflow-2.17.0
3.9-3.12
Clang 17.0.6
Bazel 6.5.0
+
tensorflow-2.16.1
3.9-3.12
Clang 17.0.6
Bazel 6.5.0
tensorflow-2.15.0
3.9-3.11
Clang 16.0.0
Bazel 6.1.0
tensorflow-2.14.0
3.9-3.11
Clang 16.0.0
Bazel 6.1.0
tensorflow-2.13.0
3.8-3.11
Clang 16.0.0
Bazel 5.3.0
@@ -462,7 +472,9 @@ Success: TensorFlow is now installed.
Version
Python version
Compiler
Build tools
cuDNN
CUDA
-
tensorflow-2.15.0
3.9-3.11
Clang 16.0.0
Bazel 6.1.0
8.8
12.2
+
tensorflow-2.17.0
3.9-3.12
Clang 17.0.6
Bazel 6.5.0
8.9
12.3
+
tensorflow-2.16.1
3.9-3.12
Clang 17.0.6
Bazel 6.5.0
8.9
12.3
+
tensorflow-2.15.0
3.9-3.11
Clang 16.0.0
Bazel 6.1.0
8.9
12.2
tensorflow-2.14.0
3.9-3.11
Clang 16.0.0
Bazel 6.1.0
8.7
11.8
tensorflow-2.13.0
3.8-3.11
Clang 16.0.0
Bazel 5.3.0
8.6
11.8
tensorflow-2.12.0
3.8-3.11
GCC 9.3.1
Bazel 5.3.0
8.6
11.8
@@ -502,6 +514,7 @@ Success: TensorFlow is now installed.
Version
Python version
Compiler
Build tools
+
tensorflow-2.16.1
3.9-3.12
Clang from Xcode 13.6
Bazel 6.5.0
tensorflow-2.15.0
3.9-3.11
Clang from xcode 10.15
Bazel 6.1.0
tensorflow-2.14.0
3.9-3.11
Clang from xcode 10.15
Bazel 6.1.0
tensorflow-2.13.0
3.8-3.11
Clang from xcode 10.15
Bazel 5.3.0
diff --git a/site/en/install/source_windows.md b/site/en/install/source_windows.md
index 9cf33d0458b..68fbcbf4785 100644
--- a/site/en/install/source_windows.md
+++ b/site/en/install/source_windows.md
@@ -1,6 +1,6 @@
# Build from source on Windows
-Build a TensorFlow *pip* package from source and install it on Windows.
+Build a TensorFlow *pip* package from the source and install it on Windows.
Note: We already provide well-tested, pre-built
[TensorFlow packages](./pip.md) for Windows systems.
@@ -13,13 +13,14 @@ environment.
### Install Python and the TensorFlow package dependencies
Install a
-[Python 3.9+ 64-bit release for Windows](https://www.python.org/downloads/windows/){:.external}.
+[Python 3.9+ 64-bit release for Windows](https://www.python.org/downloads/windows/).
Select *pip* as an optional feature and add it to your `%PATH%` environmental
variable.
Install the TensorFlow *pip* package dependencies:
@@ -41,28 +42,41 @@ Add the location of the Bazel executable to your `%PATH%` environment variable.
### Install MSYS2
-[Install MSYS2](https://www.msys2.org/){:.external} for the bin tools needed to
+[Install MSYS2](https://www.msys2.org/) for the bin tools needed to
build TensorFlow. If MSYS2 is installed to `C:\msys64`, add
`C:\msys64\usr\bin` to your `%PATH%` environment variable. Then, using `cmd.exe`,
run:
-### Install Visual C++ Build Tools 2019
+Note: Clang will be the preferred compiler to build TensorFlow CPU wheels on the Windows Platform starting with TF 2.16.1 The currently supported version is LLVM/clang 17.0.6.
-Install the *Visual C++ build tools 2019*. This comes with *Visual Studio 2019*
+Note: To build with Clang on Windows, it is required to install both LLVM and Visual C++ Build tools as although Windows uses clang-cl.exe as the compiler, Visual C++ Build tools are needed to link to Visual C++ libraries
+
+### Install Visual C++ Build Tools 2022
+
+Install the *Visual C++ build tools 2022*. This comes with *Visual Studio Community 2022*
but can be installed separately:
1. Go to the
- [Visual Studio downloads](https://visualstudio.microsoft.com/downloads/){:.external},
-2. Select *Redistributables and Build Tools*,
+ [Visual Studio downloads](https://visualstudio.microsoft.com/downloads/),
+2. Select *Tools for Visual Studio or Other Tools, Framework and Redistributables*,
3. Download and install:
- - *Microsoft Visual C++ 2019 Redistributable*
- - *Microsoft Build Tools 2019*
+ - *Build Tools for Visual Studio 2022*
+ - *Microsoft Visual C++ Redistributables for Visual Studio 2022*
+
+Note: TensorFlow is tested against the *Visual Studio Community 2022*.
+
+### Install LLVM
+
+1. Go to the
+ [LLVM downloads](https://github.com/llvm/llvm-project/releases/),
+2. Download and install Windows-compatible LLVM in C:/Program Files/LLVM e.g., LLVM-17.0.6-win64.exe
-Note: TensorFlow is tested against the *Visual Studio 2019*.
### Install GPU support (optional)
@@ -73,8 +87,8 @@ Note: GPU support on native-Windows is only available for 2.10 or earlier versio
### Download the TensorFlow source code
-Use [Git](https://git-scm.com/){:.external} to clone the
-[TensorFlow repository](https://github.com/tensorflow/tensorflow){:.external}
+Use [Git](https://git-scm.com/) to clone the
+[TensorFlow repository](https://github.com/tensorflow/tensorflow)
(`git` is installed with MSYS2):
@@ -83,7 +97,7 @@ Use [Git](https://git-scm.com/){:.external} to clone the
The repo defaults to the `master` development branch. You can also check out a
-[release branch](https://github.com/tensorflow/tensorflow/releases){:.external}
+[release branch](https://github.com/tensorflow/tensorflow/releases)
to build:
@@ -94,31 +108,32 @@ Key Point: If you're having build problems on the latest development branch, try
a release branch that is known to work.
## Optional: Environmental Variable Set Up
-Run following commands before running build command to avoid issue with package creation:
-(If the below commands were set up while installing the packages, please ignore them). Run `set` check if all the paths were set correctly, run `echo %Environmental Variable%` e.g. `echo %BAZEL_VC%` to check path set up for a specific Environmental Variable
+Run the following commands before running the build command to avoid issues with package creation:
+(If the below commands were set up while installing the packages, please ignore them). Run `set` to check if all the paths were set correctly, run `echo %Environmental Variable%` e.g., `echo %BAZEL_VC%` to check the path set up for a specific Environmental Variable
Python path set up issue [tensorflow:issue#59943](https://github.com/tensorflow/tensorflow/issues/59943),[tensorflow:issue#9436](https://github.com/tensorflow/tensorflow/issues/9436),[tensorflow:issue#60083](https://github.com/tensorflow/tensorflow/issues/60083)
-set PATH=path/to/python # [e.g. (C:/Python310)]
-set PATH=path/to/python/Scripts # [e.g. (C:/Python310/Scripts)]
+set PATH=path/to/python;%PATH% # [e.g. (C:/Python311)]
+set PATH=path/to/python/Scripts;%PATH% # [e.g. (C:/Python311/Scripts)]
set PYTHON_BIN_PATH=path/to/python_virtualenv/Scripts/python.exe
set PYTHON_LIB_PATH=path/to/python virtualenv/lib/site-packages
set PYTHON_DIRECTORY=path/to/python_virtualenv/Scripts
-Bazel/MSVC path set up issue [tensorflow:issue#54578](https://github.com/tensorflow/tensorflow/issues/54578)
+Bazel/MSVC/CLANG path set up issue [tensorflow:issue#54578](https://github.com/tensorflow/tensorflow/issues/54578)
set BAZEL_SH=C:/msys64/usr/bin/bash.exe
-set BAZEL_VS=C:/Program Files(x86)/Microsoft Visual Studio/2019/BuildTools
-set BAZEL_VC=C:/Program Files(x86)/Microsoft Visual Studio/2019/BuildTools/VC
+set BAZEL_VS=C:/Program Files/Microsoft Visual Studio/2022/BuildTools
+set BAZEL_VC=C:/Program Files/Microsoft Visual Studio/2022/BuildTools/VC
+set Bazel_LLVM=C:/Program Files/LLVM (explicitly tell Bazel where LLVM is installed by BAZEL_LLVM, needed while using CLANG)
+set PATH=C:/Program Files/LLVM/bin;%PATH% (Optional, needed while using CLANG as Compiler)
-
## Optional: Configure the build
-TensorFlow builds are configured by the `.bazelrc` file in the respoitory's
+TensorFlow builds are configured by the `.bazelrc` file in the repository's
root directory. The `./configure` or `./configure.py` scripts can be used to
adjust common settings.
@@ -138,21 +153,27 @@ differ):
View sample configuration session
python ./configure.py
-You have bazel 5.3.0 installed.
-Please specify the location of python. [Default is C:\Python310\python.exe]:
+You have bazel 6.5.0 installed.
+Please specify the location of python. [Default is C:\Python311\python.exe]:
+
Found possible Python library paths:
-C:\Python310\lib\site-packages
-Please input the desired Python library path to use. Default is [C:\Python310\lib\site-packages]
+C:\Python311\lib\site-packages
+Please input the desired Python library path to use. Default is [C:\Python311\lib\site-packages]
Do you wish to build TensorFlow with ROCm support? [y/N]:
No ROCm support will be enabled for TensorFlow.
-
WARNING: Cannot build with CUDA support on Windows.
-Starting in TF 2.11, CUDA build is not supported for Windows. For using TensorFlow GPU on Windows, you will need to build/install TensorFlow in WSL2.
+Starting in TF 2.11, CUDA build is not supported for Windows. To use TensorFlow GPU on Windows, you will need to build/install TensorFlow in WSL2.
-Please specify optimization flags to use during compilation when bazel option "--config=opt" is specified [Default is /arch:AVX]:
+Do you want to use Clang to build TensorFlow? [Y/n]:
+Add "--config=win_clang" to compile TensorFlow with CLANG.
+Please specify the path to clang executable. [Default is C:\Program Files\LLVM\bin\clang.EXE]:
+
+You have Clang 17.0.6 installed.
+
+Please specify optimization flags to use during compilation when bazel option "--config=opt" is specified [Default is /arch:AVX]:
Would you like to override eigen strong inline for some C++ compilation to reduce the compilation time? [Y/n]:
Eigen strong inline overridden.
@@ -170,13 +191,12 @@ Preconfigured Bazel build configs. You can use any of the below by adding "--con
Preconfigured Bazel build configs to DISABLE default on features:
--config=nogcp # Disable GCP support.
--config=nonccl # Disable NVIDIA NCCL support.
-
## Build and install the pip package
-The pip package gets built in two steps. A `bazel build` commands creates a
+The pip package is built in two steps. A `bazel build` command creates a
"package-builder" program. You then run the package-builder to create the
package.
@@ -187,15 +207,23 @@ tensorflow:master repo has been updated to build 2.x by default.
`bazel build ` to create the TensorFlow package-builder.
#### GPU support
@@ -217,7 +245,7 @@ bazel clean --expunge
#### Bazel build options
-Use this option when building to avoid issue with package creation:
+Use this option when building to avoid issues with package creation:
[tensorflow:issue#22390](https://github.com/tensorflow/tensorflow/issues/22390)
@@ -236,33 +264,37 @@ to suppress nvcc warning messages.
### Build the package
-The `bazel build` command creates an executable named `build_pip_package`—this
-is the program that builds the `pip` package. For example, the following builds
-a `.whl` package in the `C:/tmp/tensorflow_pkg` directory:
+To build a pip package, you need to specify the --repo_env=WHEEL_NAME flag.
+Depending on the provided name, the package will be created. For example:
-
-bazel-bin\tensorflow\tools\pip_package\build_pip_package C:/tmp/tensorflow_pkg
+To build tensorflow CPU package:
+
-Although it is possible to build both CUDA and non-CUDA configs under the
-same source tree, we recommend running `bazel clean` when switching between
-these two configurations in the same source tree.
+To build nightly package, set `tf_nightly` instead of `tensorflow`, e.g.
+to build CPU nightly package:
+
### Install the package
The filename of the generated `.whl` file depends on the TensorFlow version and
-your platform. Use `pip3 install` to install the package, for example:
+your platform. Use `pip install` to install the package, for example:
-
Success: TensorFlow is now installed.
-
## Build using the MSYS shell
TensorFlow can also be built using the MSYS shell. Make the changes listed
@@ -309,6 +341,8 @@ Note: Starting in TF 2.11, CUDA build is not supported for Windows. For using Te
Loads into the provided '*inputs' pointer the starting address of an array of indices representing the tensors that are inputs to the subgraph that is associated with the provided 'opaque_context'.
Loads into the provided '*outputs' pointer the starting address of an array of indices representing the tensors that are outputs to the subgraph that is associated with the provided 'opaque_context'.
Loads into the provided '*variables' pointer the starting address of an array of indices representing the tensors that are variables to the subgraph that is associated with the provided 'opaque_context'.
Reports an error message formed by using the provided 'format' string in combination with the data provided via the unnamed arguments following the 'format' parameter ('...').
Resizes the provided 'tensor' that is associated with the provided 'context' so that the 'tensor's shape matches the dimensionality specified via the provided 'new_size' array.
Given an 'index_of_input', which must be in the range of [0, N), where N is the number of input tensors of the provided 'opaque_node', returns the (global) index of the tensor that holds the input.
Given an 'index_of_output', which must be in the range of [0, N), where N is the number of output tensors of the provided 'opaque_node', returns the (global) index of the tensor that holds the output.
Loads into the provided '*inputs' pointer the starting address of an array of indices representing the tensors that are inputs of the provided 'opaque_node'.
Loads into the provided '*outputs' pointer the starting address of an array of indices representing the tensors that are outputs of the provided 'opaque_node'.
Loads into the provided '*temporaries' pointer the starting address of an array of indices representing the temporary tensors associated with the provided 'opaque_node'.
Retrieves the corresponding TfLiteOpaqueContext of a subgraph given a subgraph index and switches to the delegate context for this subgraph. If an invalid subgraph index is given, then returns kTfLiteError.
+
NOTE: This function is expected to be paired with TfLiteOpaqueContextReleaseSubgraphContext() once the delegate preparation is done and/or the delegate context functions are no longer needed.
Adds an additional tensor and configures its properties based on the provided 'builder', preserving pre-existing Tensor entries.
+
If non-null, the value pointed to by 'new_tensor_index' will be set to the index of the new tensor. Returns 'kTfLiteOk' when the tensor has been added successfully. Returns 'kTfLiteError' in case of failure.
Loads the provided execution_plan associated with the provided opaque_context.
+
Returns kTfLiteOk if the execution_plan was successfully loaded. A return value different from kTfLiteOk indicates a failure and the execution_plan will be left in an unspecified state.
+
+
+
+
TfLiteOpaqueContextGetInputs
+
TFL_CAPI_EXPORT TfLiteStatus TfLiteOpaqueContextGetInputs(
+ const struct TfLiteOpaqueContext *opaque_context,
+ const int **inputs,
+ int *num_inputs
+)
+
+
Loads into the provided '*inputs' pointer the starting address of an array of indices representing the tensors that are inputs to the subgraph that is associated with the provided 'opaque_context'.
+
The length of the array is loaded into the provided 'num_inputs' pointer. Returns 'kTfLiteOk' in case of success. Any other return value indicates a failure and will leave 'inputs' and 'num_inputs' in an unspecified state. Calls to 'SetInputs' on the associated subgraph invalidate the loaded pointers.
Given the specified 'opaque_context' and 'node_index', load the caller's opaque '*node' and '*registration_external' pointer.
+
Return 'kTfLiteOk' if both the '*node' as well as the '*registration_external' have been loaded correctly. Any other return code indicates a failure and both '*node' as well as '*registration_external' will be in an unspecified state.
+
A caller can obtain a node's index by calling 'TfLiteOpaqueContextGetExecutionPlan', which provides an array of node indices, sorted in execution order. A node index might also come from the data structures passed to the delegate kernel's callback parameters, like the delegate parameters data structure passed to the 'init' callback that contains an array of node indices that are meant to be handled by the delegate kernel.
+
This function is expected to be called from within a delegate callback, like 'Prepare', or a delegate kernel callback (i.e., a callback registered with a 'TfLiteRegistrationExternal' object).
+
The loaded '*node' and '*registration_external' pointers will generally remain valid for the lifetime of the associated 'opaque_context', but can be invalidated through API calls where delegates get un-applied, like API calls that modify the model graph via a delegate, or if input tensors get re-sized.
Loads metadata of a TF Lite node's custom initialization data.
+
Specifically:
+
Loads into the supplied 'fd' the file descriptor of the file that stores the 'node's custom initialization data. This output parameter will be loaded if the TF Lite runtime has access to the file descriptor, though this is not always the case, e.g. if a client provides a tflite::Model directly to the TF Lite runtime. If 'fd' can be loaded then 'kTfLiteOk' will be returned, otherwise 'kTfLiteError' is returned.
+
Loads into the supplied 'custom_initial_data_offset_in_file' pointer the offset of the 'node's custom init data in the file associated with 'fd'. This output parameter will be set to -1 if the 'node' does not have custom init data set.
+
Loads into the supplied 'custom_initial_data_size' the size of the custom initialization data. This output parameter will be set to -1 if the 'node' does not have custom init data set.
+
+
Returns 'kTfLiteOk' when 'fd' has been loaded successfully and 'kTfLiteError' otherwise. Note that this means that 'kTfLiteOk' can be returned, even if the 'node' does not have custom init data set.
Returns modifiable access to the opaque tensor that corresponds to the specified index and is associated with the provided opaque_context.
+
This requires the index to be between 0 and N - 1, where N is the number of tensors in the model.
+
Typically the tensors associated with the context would be set during the initialization of the interpreter that the context belongs to, through a mechanism like the InterpreterBuilder, and remain unchanged throughout the lifetime of the interpreter. However, there are some circumstances in which the pointer may not remain valid throughout the lifetime of the interpreter, because calls to AddTensors on the interpreter invalidate the returned pointer.
+
The ownership of the tensor remains with the TFLite runtime, meaning the caller should not deallocate the pointer.
+
+
+
+
TfLiteOpaqueContextGetOutputs
+
TFL_CAPI_EXPORT TfLiteStatus TfLiteOpaqueContextGetOutputs(
+ const struct TfLiteOpaqueContext *opaque_context,
+ const int **outputs,
+ int *num_outputs
+)
+
+
Loads into the provided '*outputs' pointer the starting address of an array of indices representing the tensors that are outputs to the subgraph that is associated with the provided 'opaque_context'.
+
The length of the array is loaded into the provided 'num_outputs' pointer. Returns 'kTfLiteOk' in case of success. Any other return value indicates a failure and will leave 'outputs' and 'num_outputs' in an unspecified state. Calls to 'SetOutputs' on the associated subgraph invalidate the loaded pointers.
Populates the size in bytes of a provide 'type' into 'bytes'.
+
Returns 'kTfLiteOk' for valid types, and 'kTfLiteError' otherwise.
+
+
+
+
TfLiteOpaqueContextGetVariables
+
TFL_CAPI_EXPORT TfLiteStatus TfLiteOpaqueContextGetVariables(
+ const struct TfLiteOpaqueContext *opaque_context,
+ const int **variables,
+ int *num_variables
+)
+
+
Loads into the provided '*variables' pointer the starting address of an array of indices representing the tensors that are variables to the subgraph that is associated with the provided 'opaque_context'.
+
The length of the array is loaded into the provided 'num_variables' pointer. Returns 'kTfLiteOk' in case of success. Any other return value indicates a failure and will leave 'variables' and 'num_variables' in an unspecified state. Calls to 'SetVariables' on the associated subgraph invalidate the loaded pointers.
TFL_CAPI_EXPORT TfLiteStatus TfLiteOpaqueContextMarkSubgraphAsDelegationSkippable(
+ TfLiteOpaqueContext *opaque_context,
+ int subgraph_index
+)
+
+
Entry point for C API MarkSubgraphAsDelegationSkippable.
+
Marks the subgraph with the given index as "delegation-skippable". Returns kTfLiteOk if the given subgraph index is valid and is successfully marked as delegation-skippable, and an error status if the subgraph index is invalid. If a subgraph is delegation-skippable, then the subgraph will be handled by a specific TfLiteOpaqueDelegate that is already supposed to be aware of this condition, and therefore, TfLiteInterpreter can skip invoking ModifyGraphWithDelegate on this subgraph.
+
NOTE: This function is expected to be called only when the subgraph that subgraph_index is pointing to should be skipped by interpreter::ModifyGraphWithDelegate (e.g. the subgraph is part of the list of callee subgraphs of the same control flow node, and all of those callees are supported by the same delegate at once).
+
For example, this function can be used when the delegate is handling control flow ops such as while ops. For instance, a while op has a condition subgraph indexed at i and a body subgraph indexed at j. The op can be delegated when the following conditions hold:
+
The delegate supports while op
+
Both condition subgraph i and body subgraph j can be fully delegated to the delegate.
+
+
Then if the delegate decides to support the while node along with both body and condition subgraphs, it should mark subgraphs i and j skippable so that those two subgraphs won't be delegated to another delegate.
+
WARNING: It is the delegate's responsibility to define when to skip Subgraph::ModifyGraphWithDelegate, to check for any edge cases (i.e. multiple references to the subgraph that subgraph_index is pointing to), and to mark a subgraph as skippable by using this function.
Releases the corresponding TfLiteOpaqueContext by switching back to the TFLite kernel context for this specified subgraph.
+
NOTE: This function is expected to be used after TfLiteOpaqueContextAcquireSubgraphContext() once the delegate preparation is done and/or the delegate context functions are no longer needed.
Entry point for C API ReplaceNodeSubsetsWithDelegateKernels.
+
Replaces the specified nodes_to_replace that are associated with the provided opaque_context with delegate kernels. The provided registration_external represents the delegate kernel and will be used for each node subset that will be delegate to the provided opaque_delegate.
+
The TF Lite runtime will take ownership of the registration_external and will delete it when the associated opaque_context gets destroyed.
+
The ownership of the nodes_to_replace and the opaque_delegate remains with the caller.
Reports an error message formed by using the provided 'format' string in combination with the data provided via the unnamed arguments following the 'format' parameter ('...').
+
The intended usage and behavior is the same as with 'printf' with regards to how the data and the formatting string interact. E.g. 'TfLiteOpaqueContextReportError(opaque_context, "a=%d b=%d", a, b);'
+
The provided 'opaque_context' will be used for reporting the resulting error message.
+
Note that TF Lite clients can use macros like 'TF_LITE_OPAQUE_ENSURE' to check for certain conditions to be true, and print an error message if the condition does not hold. Direct usage of this function from application code should therefore be rare.
Same as TfLiteOpaqueContextReportError, but with the variable arguments passed via a va_list instead of directly.
+
Callers that receive an ellipsis and want to forward it to to the opaque context error reporting API can add the ellipsis content to a va_list and then call TfLiteOpaqueContextReportErrorVa. E.g.:
Resizes the provided 'tensor' that is associated with the provided 'context' so that the 'tensor's shape matches the dimensionality specified via the provided 'new_size' array.
+
Returns 'kTfLiteOk' in case of success. Any other return value indicates a failure and will leave the 'tensor' in an unspecified state. The TF Lite runtime takes ownership of the 'new_size' array, even in case of failure.
Returns the builtin data associated with the provided 'opaque_node'.
+
The builtin init data associated with a node would typically be set during the creation of the associated interpreter, through a mechanism like the interpreter builder that loads a TFLite model and initialises the interpreter's nodes accordingly. Under these conditions the returned address remains valid throughout the lifetime of the 'opaque_node'.
Loads into the provided '*init_data' pointer the address of the custom init data associated with the provided 'opaque_node'.
+
The length of data is loaded into the provided 'size' pointer. Returns 'kTfLiteOk' in case of success. Any other return value indicates a failure and will leave 'init_data' and 'size' in an unspecified state.
+
The custom init data associated with a node would typically be set during the creation of the associated interpreter, through a mechanism like the interpreter builder that loads a TFLite model and initialises the interpreter's nodes accordingly. Under these conditions the returned address remains valid throughout the lifetime of the 'opaque_node'.
TFL_CAPI_EXPORT int TfLiteOpaqueNodeGetInputTensorIndex(
+ const TfLiteOpaqueNode *opaque_node,
+ int index_of_input
+)
+
+
Given an 'index_of_input', which must be in the range of [0, N), where N is the number of input tensors of the provided 'opaque_node', returns the (global) index of the tensor that holds the input.
+
Returns -1 if 'index_of_input' is not within the [0, N) range.
TFL_CAPI_EXPORT int TfLiteOpaqueNodeGetOutputTensorIndex(
+ const TfLiteOpaqueNode *opaque_node,
+ int index_of_output
+)
+
+
Given an 'index_of_output', which must be in the range of [0, N), where N is the number of output tensors of the provided 'opaque_node', returns the (global) index of the tensor that holds the output.
+
Returns -1 if 'index_of_output' is not within the [0, N) range.
Returns opaque data provided by the node implementer.
+
The value returned from this function is the value that was returned from the init callback that was passed to TfLiteRegistrationExternalSetInit.
+
+
+
+
TfLiteOpaqueNodeInputs
+
TFL_CAPI_EXPORT TfLiteStatus TfLiteOpaqueNodeInputs(
+ const TfLiteOpaqueNode *opaque_node,
+ const int **inputs,
+ int *num_inputs
+)
+
+
Loads into the provided '*inputs' pointer the starting address of an array of indices representing the tensors that are inputs of the provided 'opaque_node'.
+
The length of the array is loaded into the provided 'num_inputs' pointer. Returns 'kTfLiteOk' in case of success. Any other return value indicates a failure and will leave 'inputs' and 'num_inputs' in an unspecified state.
+
The input tensors associated with a node would typically be set during the creation of the associated interpreter, through a mechanism like the interpreter builder that loads a TFLite model and initialises the interpreter's nodes accordingly. Under these conditions the loaded address remains valid throughout the lifetime of the 'opaque_node'.
+
+
+
+
TfLiteOpaqueNodeNumberOfInputs
+
TFL_CAPI_EXPORT int TfLiteOpaqueNodeNumberOfInputs(
+ const TfLiteOpaqueNode *opaque_node
+)
+
+
Gets the number of input tensors of the provided 'opaque_node'.
+
+
+
+
TfLiteOpaqueNodeNumberOfOutputs
+
TFL_CAPI_EXPORT int TfLiteOpaqueNodeNumberOfOutputs(
+ const TfLiteOpaqueNode *opaque_node
+)
+
+
Gets the number of output tensors of the provided 'opaque_node'.
+
+
+
+
TfLiteOpaqueNodeOutputs
+
TFL_CAPI_EXPORT TfLiteStatus TfLiteOpaqueNodeOutputs(
+ const TfLiteOpaqueNode *opaque_node,
+ const int **outputs,
+ int *num_outputs
+)
+
+
Loads into the provided '*outputs' pointer the starting address of an array of indices representing the tensors that are outputs of the provided 'opaque_node'.
+
The length of the array is loaded into the provided 'num_outputs' pointer. Returns 'kTfLiteOk' in case of success. Any other return value indicates a failure and will leave 'outputs' and 'num_outputs' in an unspecified state.
+
The output tensors associated with a node would typically be set during the creation of the associated interpreter, through a mechanism like the interpreter builder that loads a TFLite model and initialises the interpreter's nodes accordingly. Under these conditions the loaded address remains valid throughout the lifetime of the 'opaque_node'.
+
+
+
+
TfLiteOpaqueNodeTemporaries
+
TFL_CAPI_EXPORT TfLiteStatus TfLiteOpaqueNodeTemporaries(
+ const TfLiteOpaqueNode *opaque_node,
+ const int **temporaries,
+ int *num_temporaries
+)
+
+
Loads into the provided '*temporaries' pointer the starting address of an array of indices representing the temporary tensors associated with the provided 'opaque_node'.
+
The length of the array is loaded into the provided 'num_temporaries' pointer. Returns 'kTfLiteOk' in case of success. Any other return value indicates a failure and will leave 'temporaries' and 'num_temporaries' in an unspecified state.
+
The temporary tensors associated with a node would typically be set during the creation of the associated interpreter, through a mechanism like the interpreter builder that loads a TFLite model and initialises the interpreter's nodes accordingly. Under these conditions the loaded address remains valid throughout the lifetime of the 'opaque_node'.
Sets the allocation type of the provided 'builder' to the provided 'allocation_type'.
+
The 'allocation_type' must be one of the following: 'kTfLiteDynamic', 'kTfLiteArenaRw' or 'kTfLiteArenaRwPersistent'. If the provided 'allocation_type' is not one of those values then 'TfLiteOpaqueContextAddTensor' will return an error. Returns the address of the provided 'builder', so that builder calls can be chained together.
Loads into the provided 'num_dims' the number of dimensions that the tensor's signature has.
+
Returns 'kTfLiteOk' if 'num_dims' was successfully loaded. Any other return code indicates an error and 'num_dims' won't be loaded.
+
A tensor's dimension signature encodes shapes with unknown dimensions with -1. E.g. for a tensor with three dimensions, whose first dimension has an unknown size, and the second and third dimension have a size of 2, the dimension signature is [-1,2,2], and 'TfLiteOpaqueTensorGetNumDimsSignature' loads 3 into 'num_dims'. If the tensor does not have its dimension signature field set then 'num_dims' is set to -1.
Returns the operation step when the shape of a tensor is computed.
+
+
+
+
TfLiteOpaqueTensorGetString
+
TfLiteStatus TfLiteOpaqueTensorGetString(
+ const TfLiteOpaqueTensor *tensor,
+ int index,
+ const char **str,
+ int *len
+)
+
+
Stores the address of the n-th (denoted by the provided 'index') string contained in the provided 'tensor' in the provided '*str' pointer.
+
Stores the length of the string in the provided '*len' argument.
+
Returns 'kTfLiteOk' if '*str' and '*len' have been set successfully. Any other return value indicates a failure, which leaves '*str' and '*len' in an unspecified state.
+
The range of valid indices is defined by the half open interval [0, N), where N == TfLiteOpaqueTensorGetStringCount(tensor).
+
Note that 'str' is not guaranteed to be null-terminated. Also note that this function will not create a copy of the underlying string data. The data is owned by the 'tensor'.
+
+
+
+
TfLiteOpaqueTensorGetStringCount
+
int TfLiteOpaqueTensorGetStringCount(
+ const TfLiteOpaqueTensor *tensor
+)
+
+
Returns the number of strings stored in the provided 'tensor'.
+
Returns -1 in case of failure.
+
+
+
+
TfLiteOpaqueTensorIsVariable
+
TFL_CAPI_EXPORT int TfLiteOpaqueTensorIsVariable(
+ const TfLiteOpaqueTensor *opaque_tensor
+)
+
+
Returns 'non-zero' if the provided 'opaque_tensor' is a variable, and returns zero otherwise.
Writes the string pointed to by the provided 'str' pointer of length 'len' into the provided 'tensor'.
+
The string provided via 'str' is copied into the 'tensor'. Returns 'kTfLiteOk' in case of success. Any other return value indicates a failure.
+
Note that calling 'TfLiteOpaqueTensorWriteString' deallocates any previously stored data in the 'tensor'. E.g. suppose 't' denotes a 'TfLiteOpaqueTensor*', then calling 'TfLiteOpaqueTensorWriteString(t, "AB", 2)' followed by a call to 'TfLiteOpaqueTensorWriteString(t, "CD", 2)' will lead to 't' containing 'CD', not 'ABCD'.
+
'TfLiteOpaqueTensorWriteString' is a convenience function for the use case of writing a single string to a tensor and its effects are identical to calling 'TfLiteOpaqueTensorWriteStrings' with an array of a single string.
+
+
+
+
TfLiteOpaqueTensorWriteStrings
+
TfLiteStatus TfLiteOpaqueTensorWriteStrings(
+ TfLiteOpaqueTensor *tensor,
+ const char *const *str_array,
+ int str_array_len,
+ const int *str_n_len
+)
+
+
Writes the array of strings specified by 'str_array' into the specified 'tensor'.
+
The strings provided via the 'str_array' are being copied into the 'tensor'. Returns 'kTfLiteOk' in case of success. Any other return value indicates a failure.
+
The provided 'str_array_len' must denote the length of 'str_array' and 'str_n_len[i]' must denote the length of the i-th string.
+
The provided strings don't need to be null terminated and may contain embedded null characters. The amount of bytes copied into the 'tensor' is entirely determined by 'str_n_len[i]' and it is the caller's responsibility to set this value correctly to avoid undefined behavior.
+
Also note that calling 'TfLiteOpaqueTensorWriteStrings' deallocates any previously stored data in the 'tensor'.
This file declares types used by the pure C inference API defined in c_api.h, some of which are also used in the C++ and C kernel and interpreter APIs.
Note that new error status values may be added in future in order to indicate more fine-grained internal states, therefore, applications should not rely on status values being members of the enum.
Note that new error status values may be added in future in order to indicate more fine-grained internal states, therefore, applications should not rely on status values being members of the enum.
Note that new error status values may be added in future in order to indicate more fine-grained internal states, therefore, applications should not rely on status values being members of the enum.
+
+
+
Properties
+
+
+
+ kTfLiteApplicationError
+
+
+
Generally referring to an error in applying a delegate due to incompatibility between runtime and delegate, e.g., this error is returned when trying to apply a TF Lite delegate onto a model graph that's already immutable.
+
+
+
+
+ kTfLiteCancelled
+
+
+
Generally referring to invocation cancelled by the user.
+
See interpreter::Cancel.
+
+
+
+
+ kTfLiteDelegateDataNotFound
+
+
+
Generally referring to serialized delegate data not being found.
+
See tflite::delegates::Serialization.
+
+
+
+
+ kTfLiteDelegateDataReadError
+
+
+
Generally referring to data-reading issues in delegate serialization.
+
See tflite::delegates::Serialization.
+
+
+
+
+ kTfLiteDelegateDataWriteError
+
+
+
Generally referring to data-writing issues in delegate serialization.
+
See tflite::delegates::Serialization.
+
+
+
+
+ kTfLiteDelegateError
+
+
+
Generally referring to an error from a TfLiteDelegate itself.
+
+
+
+
+ kTfLiteError
+
+
+
Generally referring to an error in the runtime (i.e. interpreter)
+
+
+
+
+ kTfLiteOk
+
+
+
Success.
+
+
+
+
+ kTfLiteUnresolvedOps
+
+
+
Generally referring to issues when the TF Lite model has ops that cannot be resolved at runtime.
+
This could happen when the specific op is not registered or built with the TF Lite framework.
TfLiteOpaqueDelegateStruct: unconditionally opaque version of TfLiteDelegate; allows delegation of nodes to alternative backends.
+
This is an abstract type that is intended to have the same role as TfLiteDelegate, but without exposing the implementation details of how delegates are implemented.
+
WARNING: This is an experimental type and subject to change.
Will be deprecated in favor of TfLiteAffineQuantization. If per-layer quantization is specified this field will still be populated in addition to TfLiteAffineQuantization. Parameters for asymmetric quantization. Quantized values can be converted back to float using: real_value = scale * (quantized_value - zero_point)
Note that new error status values may be added in future in order to indicate more fine-grained internal states, therefore, applications should not rely on status values being members of the enum.
The API leans towards simplicity and uniformity instead of convenience, as most usage will be by language-specific wrappers. It provides largely the same set of functionality as that of the C++ TensorFlow Lite Interpreter API, but is useful for shared libraries where having a stable ABI boundary is important.
+
Conventions:
+
We use the prefix TfLite for everything in the API.
+
size_t is used to represent byte sizes of objects that are materialized in the address space of the calling process.
+
int is used as an index into arrays.
+
+
Usage:
+// Create the model and interpreter options.
+TfLiteModel* model = TfLiteModelCreateFromFile("/path/to/model.tflite");
+TfLiteInterpreterOptions* options = TfLiteInterpreterOptionsCreate();
+TfLiteInterpreterOptionsSetNumThreads(options, 2);
+
+
// Create the interpreter.
+TfLiteInterpreter* interpreter = TfLiteInterpreterCreate(model, options);
// Dispose of the model and interpreter objects.
+TfLiteInterpreterDelete(interpreter);
+TfLiteInterpreterOptionsDelete(options);
+TfLiteModelDelete(model);
+
Returns a pointer to a statically allocated string that is the version number of the TF Lite Extension APIs supported by the (potentially dynamically loaded) TF Lite Runtime library. The TF Lite "Extension APIs" are the APIs for extending TF Lite with custom ops and delegates. More specifically, this version number covers the (non-experimental) functionality documented in the following header files:
+
+
+
lite/c/c_api_opaque.h
+
lite/c/common.h
+
lite/c/builtin_op_data.h
+
lite/builtin_ops.h
+
+
+
This version number uses semantic versioning, and the return value should be in semver 2 format http://semver.org, starting with MAJOR.MINOR.PATCH, e.g. "2.14.0" or "2.15.0-rc2".
Returns a new interpreter using the provided model and options, or null on failure.
+
+
+
model must be a valid model instance. The caller retains ownership of the object, and may destroy it (via TfLiteModelDelete) immediately after creating the interpreter. However, if the TfLiteModel was allocated with TfLiteModelCreate, then the model_data buffer that was passed to TfLiteModelCreate must outlive the lifetime of the TfLiteInterpreter object that this function returns, and must not be modified during that time; and if the TfLiteModel was allocated with TfLiteModelCreateFromFile, then the contents of the model file must not be modified during the lifetime of the TfLiteInterpreter object that this function returns.
+
optional_options may be null. The caller retains ownership of the object, and can safely destroy it (via TfLiteInterpreterOptionsDelete) immediately after creating the interpreter.
(i) (recommended) using the Interpreter to initialize SignatureRunner(s) and then only using SignatureRunner APIs.
+
(ii) only using Interpreter APIs.
+
NOTE:
+
Only use one of the above options to run inference, i.e. avoid mixing both SignatureRunner APIs and Interpreter APIs to run inference as they share the same underlying data (e.g. updating an input tensor “A” retrieved using the Interpreter APIs will update the state of the input tensor “B” retrieved using SignatureRunner APIs, if they point to the same underlying tensor in the model; as it is not possible for a user to debug this by analyzing the code, it can lead to undesirable behavior).
+
The TfLiteSignatureRunner type is conditionally thread-safe, provided that no two threads attempt to simultaneously access two TfLiteSignatureRunner instances that point to the same underlying signature, or access a TfLiteSignatureRunner and its underlying TfLiteInterpreter, unless all such simultaneous accesses are reads (rather than writes).
+
The lifetime of a TfLiteSignatureRunner object ends when TfLiteSignatureRunnerDelete() is called on it (or when the lifetime of the underlying TfLiteInterpreter ends but you should call TfLiteSignatureRunnerDelete() before that happens in order to avoid resource leaks).
+
You can only apply delegates to the interpreter (via TfLiteInterpreterOptions) and not to a signature. Returns the number of signatures defined in the model.
Returns modifiable access to the tensor that corresponds to the specified index and is associated with the provided interpreter.
+
This requires the index to be between 0 and N - 1, where N is the number of tensors in the model.
+
Typically the tensors associated with the interpreter would be set during the interpreter initialization, through a mechanism like the InterpreterBuilder, and remain unchanged throughout the lifetime of the interpreter. However, there are some circumstances in which the pointer may not remain valid throughout the lifetime of the interpreter, because calls to AddTensors on the interpreter invalidate the returned pointer.
+
Note the difference between this function and TfLiteInterpreterGetInputTensor (or TfLiteInterpreterGetOutputTensor for that matter): TfLiteInterpreterGetTensor takes an index into the array of all tensors associated with the interpreter's model, whereas TfLiteInterpreterGetInputTensor takes an index into the array of input tensors.
+
The ownership of the tensor remains with the TFLite runtime, meaning the caller should not deallocate the pointer.
+
+
+
+
TfLiteInterpreterInputTensorIndices
+
TFL_CAPI_EXPORT const int * TfLiteInterpreterInputTensorIndices(
+ const TfLiteInterpreter *interpreter
+)
+
+
Returns a pointer to an array of input tensor indices.
+
The length of the array can be obtained via a call to TfLiteInterpreterGetInputTensorCount.
+
Typically the input tensors associated with an interpreter would be set during the initialization of the interpreter, through a mechanism like the InterpreterBuilder, and remain unchanged throughout the lifetime of the interpreter. However, there are some circumstances in which the pointer may not remain valid throughout the lifetime of the interpreter, because calls to SetInputs on the interpreter invalidate the returned pointer.
+
The ownership of the array remains with the TFLite runtime.
Before calling this function, the caller should first invoke TfLiteInterpreterAllocateTensors() and should also set the values for the input tensors. After successfully calling this function, the values for the output tensors will be set.
+
+If the (experimental!) delegate fallback option was enabled in the interpreter options, then the interpreter will automatically fall back to not using any delegates if execution with delegates fails. For details, see TfLiteInterpreterOptionsSetEnableDelegateFallback in c_api_experimental.h.
+
Returns one of the following status codes:
+
kTfLiteOk: Success. Output is valid.
+
kTfLiteDelegateError: Execution with delegates failed, due to a problem with the delegate(s). If fallback was not enabled, output is invalid. If fallback was enabled, this return value indicates that fallback succeeded, the output is valid, and all delegates previously applied to the interpreter have been undone.
+
kTfLiteApplicationError: Same as for kTfLiteDelegateError, except that the problem was not with the delegate itself, but rather was due to an incompatibility between the delegate(s) and the interpreter or model.
+
kTfLiteError: Unexpected/runtime failure. Output is invalid.
Adds a delegate to be applied during TfLiteInterpreter creation.
+
If delegate application fails, interpreter creation will also fail with an associated error logged.
+
+If you are NOT using "TensorFlow Lite in Play Services", and NOT building with TFLITE_WITH_STABLE_ABI or TFLITE_USE_OPAQUE_DELEGATE macros enabled, it is possible to pass a TfLiteDelegate* rather than a TfLiteOpaqueDelegate* to this function, since in those cases, TfLiteOpaqueDelegate is just a typedef alias for TfLiteDelegate. This is for compatibility with existing source code and existing delegates. For new delegates, it is recommended to use TfLiteOpaqueDelegate rather than TfLiteDelegate. (See TfLiteOpaqueDelegate in tensorflow/lite/core/c/c_api_types.h.)
Adds an op registration to be applied during TfLiteInterpreter creation.
+
The TfLiteRegistrationExternal object is needed to implement custom op of TFLite Interpreter via C API. Calling this function ensures that any TfLiteInterpreter created with the specified options can execute models that use the custom operator specified in registration. Please refer https://www.tensorflow.org/lite/guide/ops_custom for custom op support. This is an experimental API and subject to change.
Sets the number of CPU threads to use for the interpreter.
+
+
+
+
TfLiteInterpreterOutputTensorIndices
+
TFL_CAPI_EXPORT const int * TfLiteInterpreterOutputTensorIndices(
+ const TfLiteInterpreter *interpreter
+)
+
+
Returns a pointer to an array of output tensor indices.
+
The length of the array can be obtained via a call to TfLiteInterpreterGetOutputTensorCount.
+
Typically the output tensors associated with an interpreter would be set during the initialization of the interpreter, through a mechanism like the InterpreterBuilder, and remain unchanged throughout the lifetime of the interpreter. However, there are some circumstances in which the pointer may not remain valid throughout the lifetime of the interpreter, because calls to SetOutputs on the interpreter invalidate the returned pointer.
+
The ownership of the array remains with the TFLite runtime.
TFL_CAPI_EXPORT int TfLiteSchemaVersion(
+ void
+)
+
+
The supported TensorFlow Lite model file Schema version.
+
Returns the (major) version number of the Schema used for model files that is supported by the (potentially dynamically loaded) TensorFlow Lite Runtime.
+
Model files using schema versions different to this may not be supported by the current version of the TF Lite Runtime.
Before calling this function, the caller should first invoke TfLiteSignatureRunnerAllocateTensors() and should also set the values for the input tensors. After successfully calling this function, the values for the output tensors will be set.
Resizes the input tensor identified as input_name to be the dimensions specified by input_dims and input_dims_size.
+
Only unknown dimensions can be resized with this function. Unknown dimensions are indicated as -1 in the dims_signature attribute of a TfLiteTensor.
+
Returns status of failure or success. Note that this doesn't actually resize any existing buffers. A call to TfLiteSignatureRunnerAllocateTensors() is required to change the tensor input buffer.
Returns the parameters for asymmetric quantization.
+
The quantization parameters are only valid when the tensor type is kTfLiteUInt8 and the scale != 0. Quantized values can be converted back to float using: real_value = scale * (quantized_value - zero_point);
Returns a pointer to a statically allocated string that is the version number of the (potentially dynamically loaded) TF Lite Runtime library. TensorFlow Lite uses semantic versioning, and the return value should be in semver 2 format http://semver.org, starting with MAJOR.MINOR.PATCH, e.g. "2.12.0" or "2.13.0-rc2".
TfLiteRegistrationExternal is an external version of TfLiteRegistration for C API which doesn't use internal types (such as TfLiteContext) but only uses stable API types (such as TfLiteOpaqueContext).
A union of pointers that points to memory for a given tensor.
+
+
+
+
Enumerations
+
+
Anonymous Enum 0
+
Anonymous Enum 0
+
+
+
+
TfLiteAllocationStrategy
+
TfLiteAllocationStrategy
+
+
Memory allocation strategies.
+
TfLiteAllocationType values have been overloaded to mean more than their original intent. This enum should only be used to document the allocation strategy used by a tensor for it data.
+
+
+
Properties
+
+
+
+ kTfLiteAllocationStrategyArena
+
+
+
Data is mmaped.
+
+
+
+
+ kTfLiteAllocationStrategyMMap
+
+
+
No data is allocated.
+
+
+
+
+ kTfLiteAllocationStrategyMalloc
+
+
+
Handled by the arena.
+
+
+
+
+ kTfLiteAllocationStrategyNew
+
+
+
Uses malloc/free.
+
Uses new[]/delete[].
+
+
+
+
+
+
+
TfLiteAllocationType
+
TfLiteAllocationType
+
+
Memory allocation strategies.
+
+
+
kTfLiteMmapRo: Read-only memory-mapped data, or data externally allocated.
+
kTfLiteArenaRw: Arena allocated with no guarantees about persistence, and available during eval.
+
kTfLiteArenaRwPersistent: Arena allocated but persistent across eval, and only available during eval.
+
kTfLiteDynamic: Allocated during eval, or for string tensors.
+
kTfLitePersistentRo: Allocated and populated during prepare. This is useful for tensors that can be computed during prepare and treated as constant inputs for downstream ops (also in prepare).
+
kTfLiteCustom: Custom memory allocation provided by the user. See TfLiteCustomAllocation below.
+
kTfLiteVariantObject: Allocation is an arbitrary type-erased C++ object. Allocation and deallocation are done through new and delete.
+
+
+
+
+
+
TfLiteCustomAllocationFlags
+
TfLiteCustomAllocationFlags
+
+
The flags used in Interpreter::SetCustomAllocationForTensor.
+
Note that this is a bitmask, so the values should be 1, 2, 4, 8, ...etc.
+
+
+
Properties
+
+
+
+ kTfLiteCustomAllocationFlagsSkipAlignCheck
+
+
+
Skips checking whether allocation.data points to an aligned buffer as expected by the TFLite runtime.
+
NOTE: Setting this flag can cause crashes when calling Invoke(). Use with caution.
Note that this is a bitmask, so the values should be 1, 2, 4, 8, ...etc.
+
+
+
Properties
+
+
+
+ kTfLiteDelegateFlagsAllowDynamicTensors
+
+
+
The flag is set if the delegate can handle dynamic sized tensors.
+
For example, the output shape of a Resize op with non-constant shape can only be inferred when the op is invoked. In this case, the Delegate is responsible for calling SetTensorToDynamic to mark the tensor as a dynamic tensor, and calling ResizeTensor when invoking the op.
+
If the delegate isn't capable to handle dynamic tensors, this flag need to be set to false.
+
+
+
+
+ kTfLiteDelegateFlagsPerOperatorProfiling
+
+
+
This flag can be used by delegates to request per-operator profiling.
+
If a node is a delegate node, this flag will be checked before profiling. If set, then the node will not be profiled. The delegate will then add per operator information using Profiler::EventType::OPERATOR_INVOKE_EVENT and the results will appear in the operator-wise Profiling section and not in the Delegate internal section.
+
+
+
+
+ kTfLiteDelegateFlagsRequirePropagatedShapes
+
+
+
This flag can be used by delegates (that allow dynamic tensors) to ensure applicable tensor shapes are automatically propagated in the case of tensor resizing.
+
This means that non-dynamic (allocation_type != kTfLiteDynamic) I/O tensors of a delegate kernel will have correct shapes before its Prepare() method is called. The runtime leverages TFLite builtin ops in the original execution plan to propagate shapes.
+
A few points to note:
+
This requires kTfLiteDelegateFlagsAllowDynamicTensors. If that flag is false, this one is redundant since the delegate kernels are re-initialized every time tensors are resized.
+
Enabling this flag adds some overhead to AllocateTensors(), since extra work is required to prepare the original execution plan.
+
This flag requires that the original execution plan only have ops with valid registrations (and not 'dummy' custom ops like with Flex).
+
+
WARNING: This feature is experimental and subject to change.
+
+
+
+
+
+
+
TfLiteDimensionType
+
TfLiteDimensionType
+
+
Storage format of each dimension in a sparse tensor.
+
+
+
+
TfLiteExternalContextType
+
TfLiteExternalContextType
+
+
The list of external context types known to TF Lite.
+
This list exists solely to avoid conflicts and to ensure ops can share the external contexts they need. Access to the external contexts is controlled by one of the corresponding support files.
This allow an op to signal to the runtime that the same data pointer may be passed as an input and output without impacting the result. This does not mean that the memory can safely be reused, it is up to the runtime to determine this, e.g. if another op consumes the same input or not or if an input tensor has sufficient memory allocated to store the output data.
+
Setting these flags authorizes the runtime to set the data pointers of an input and output tensor to the same value. In such cases, the memory required by the output must be less than or equal to that required by the shared input, never greater. If kTfLiteInplaceOpDataUnmodified is set, then the runtime can share the same input tensor with multiple operator's outputs, provided that kTfLiteInplaceOpDataUnmodified is set for all of them. Otherwise, if an input tensor is consumed by multiple operators, it may only be shared with the operator which is the last to consume it.
+
Note that this is a bitmask, so the values should be 1, 2, 4, 8, ...etc.
Setting kTfLiteInplaceInputCanBeSharedWithCorrespondingOutput means that InputN may be shared with OutputN instead of with the first output.
+
This flag requires one or more of kTfLiteInplaceOpInputNShared to be set.
+
+
+
+
+ kTfLiteInplaceOpDataUnmodified
+
+
+
This indicates that an op's first output's data is identical to its first input's data, for example Reshape.
+
+
+
+
+ kTfLiteInplaceOpInput0Shared
+
+
+
kTfLiteInplaceOpInputNShared indicates that it is safe for an op to share InputN's data pointer with an output tensor.
+
If kTfLiteInplaceInputCanBeSharedWithCorrespondingOutput is set then kTfLiteInplaceOpInputNShared indicates that InputN may be shared with OutputN, otherwise kTfLiteInplaceOpInputNShared indicates that InputN may be shared with the first output.
+
Indicates that an op's first input may be shared with the first output tensor. kTfLiteInplaceInputCanBeSharedWithCorrespondingOutput has no impact on the behavior allowed by this flag.
+
+
+
+
+ kTfLiteInplaceOpInput1Shared
+
+
+
Indicates that an op's second input may be shared with the first output if kTfLiteInplaceInputCanBeSharedWithCorrespondingOutput is not set or second output if kTfLiteInplaceInputCanBeSharedWithCorrespondingOutput is set.
+
+
+
+
+ kTfLiteInplaceOpInput2Shared
+
+
+
Indicates that an op's third input may be shared with the first output if kTfLiteInplaceInputCanBeSharedWithCorrespondingOutput is not set or third output if kTfLiteInplaceInputCanBeSharedWithCorrespondingOutput is set.
+
+
+
+
+ kTfLiteInplaceOpMaxValue
+
+
+
Placeholder to ensure that enum can hold 64 bit values to accommodate future fields.
+
+
+
+
+ kTfLiteInplaceOpNone
+
+
+
The default value.
+
This indicates that the same data pointer cannot safely be passed as an op's input and output.
+
+
+
+
+
+
+
TfLiteQuantizationType
+
TfLiteQuantizationType
+
+
SupportedQuantizationTypes.
+
+
+
Properties
+
+
+
+ kTfLiteAffineQuantization
+
+
+
Affine quantization (with support for per-channel quantization).
Parameters for asymmetric quantization across a dimension (i.e per output channel quantization).
+
quantized_dimension specifies which dimension the scales and zero_points correspond to. For a particular value in quantized_dimension, quantized values can be converted back to float using: real_value = scale * (quantized_value - zero_point)
TfLiteAllocationType values have been overloaded to mean more than their original intent. This enum should only be used to document the allocation strategy used by a tensor for it data.
kTfLiteMmapRo: Read-only memory-mapped data, or data externally allocated.
+
kTfLiteArenaRw: Arena allocated with no guarantees about persistence, and available during eval.
+
kTfLiteArenaRwPersistent: Arena allocated but persistent across eval, and only available during eval.
+
kTfLiteDynamic: Allocated during eval, or for string tensors.
+
kTfLitePersistentRo: Allocated and populated during prepare. This is useful for tensors that can be computed during prepare and treated as constant inputs for downstream ops (also in prepare).
+
kTfLiteCustom: Custom memory allocation provided by the user. See TfLiteCustomAllocation below.
+
kTfLiteVariantObject: Allocation is an arbitrary type-erased C++ object. Allocation and deallocation are done through new and delete.
+
+
+
+
+
+
TfLiteBufferHandle
+
int TfLiteBufferHandle
+
+
The delegates should use zero or positive integers to represent handles.
TfLiteContext is a struct that is created by the TF Lite runtime and passed to the "methods" (C function pointers) in the TfLiteRegistration struct that are used to define custom ops and custom delegate kernels. It contains information and methods (C function pointers) that can be called by the code implementing a custom op or a custom delegate kernel. These methods provide access to the context in which that custom op or custom delegate kernel occurs, such as access to the input and output tensors for that op, as well as methods for allocating memory buffers and intermediate tensors, etc.
+
See also TfLiteOpaqueContext, which is an more ABI-stable equivalent.
Defines a custom memory allocation not owned by the runtime.
+
data should be aligned to kDefaultTensorAlignment defined in lite/util.h. (Currently 64 bytes) NOTE: See Interpreter::SetCustomAllocationForTensor for details on usage.
WARNING: This is an experimental interface that is subject to change.
+
Currently, TfLiteDelegateParams has to be allocated in a way that it's trivially destructable. It will be stored as builtin_data field in TfLiteNode of the delegate node.
+
See also the CreateDelegateParams function in interpreter.cc details.
An external context is a collection of information unrelated to the TF Lite framework, but useful to a subset of the ops.
+
TF Lite knows very little about the actual contexts, but it keeps a list of them, and is able to refresh them if configurations like the number of recommended threads change.
The list of external context types known to TF Lite.
+
This list exists solely to avoid conflicts and to ensure ops can share the external contexts they need. Access to the external contexts is controlled by one of the corresponding support files.
TfLiteOpaqueDelegateBuilder is used for constructing TfLiteOpaqueDelegate, see TfLiteOpaqueDelegateCreate below.
+
Note: This struct is not ABI stable.
+
For forward source compatibility TfLiteOpaqueDelegateBuilder objects should be brace-initialized, so that all fields (including any that might be added in the future) get zero-initialized. The purpose of each field is exactly the same as with TfLiteDelegate.
+
WARNING: This is an experimental interface that is subject to change.
WARNING: This is an experimental interface that is subject to change.
+
Currently, TfLiteOpaqueDelegateParams has to be allocated in a way that it's trivially destructable. It will be stored as builtin_data field in TfLiteNode of the delegate node.
+
See also the CreateOpaqueDelegateParams function in subgraph.cc details.
TfLiteRegistrationExternal is an external version of TfLiteRegistration for C API which doesn't use internal types (such as TfLiteContext) but only uses stable API types (such as TfLiteOpaqueContext).
+
The purpose of each field is the exactly the same as with TfLiteRegistration.
Old version of TfLiteRegistration to maintain binary backward compatibility.
+
The legacy registration type must be a POD struct type whose field types must be a prefix of the field types in TfLiteRegistration, and offset of the first field in TfLiteRegistration that is not present in the legacy registration type must be greater than or equal to the size of the legacy registration type.
+
WARNING: This structure is deprecated / not an official part of the API. It should be only used for binary backward compatibility.
Old version of TfLiteRegistration to maintain binary backward compatibility.
+
The legacy registration type must be a POD struct type whose field types must be a prefix of the field types in TfLiteRegistration, and offset of the first field in TfLiteRegistration that is not present in the legacy registration type must be greater than or equal to the size of the legacy registration type.
+
WARNING: This structure is deprecated / not an official part of the API. It should be only used for binary backward compatibility.
Old version of TfLiteRegistration to maintain binary backward compatibility.
+
The legacy registration type must be a POD struct type whose field types must be a prefix of the field types in TfLiteRegistration, and offset of the first field in TfLiteRegistration that is not present in the legacy registration type must be greater than or equal to the size of the legacy registration type.
+
WARNING: This structure is deprecated / not an official part of the API. It should be only used for binary backward compatibility.
Creates an opaque delegate and returns its address.
+
The opaque delegate will behave according to the provided opaque_delegate_builder. The lifetime of the objects pointed to by any of the fields within the opaque_delegate_builder must outlive the returned TfLiteOpaqueDelegate and any TfLiteInterpreter, TfLiteInterpreterOptions, tflite::Interpreter, or tflite::InterpreterBuilder that the delegate is added to. The returned address should be passed to TfLiteOpaqueDelegateDelete for deletion. If opaque_delegate_builder is a null pointer, then a null pointer will be returned.
The delegate has been constructed via a TfLiteOpaqueDelegateBuilder, but the data field of the TfLiteOpaqueDelegateBuilder is null.The data_ field of delegate will be returned if the opaque_delegate_builder field is null.
Function does nothing if either src or dst is passed as nullptr and return kTfLiteOk. Returns kTfLiteError if src and dst doesn't have matching data size. Note function copies contents, so it won't create new data pointer or change allocation type. All Tensor related properties will be copied from src to dst like quantization, sparsity, ...
Returns the operation steop when the shape of a tensor is computed.
+
Some operations can precompute the shape of their results before the evaluation step. This makes the shape available earlier for subsequent operations.
Change the size of the memory block owned by tensor to num_bytes.
+
Tensors with allocation types other than kTfLiteDynamic will be ignored and a kTfLiteOk will be returned. tensor's internal data buffer will be assigned a pointer which can safely be passed to free or realloc if num_bytes is zero. Tensor data will be unchanged in the range from the start of the region up to the minimum of the old and new sizes. In the case of NULL tensor, or an error allocating new memory, returns kTfLiteError.
Change the size of the memory block owned by tensor to num_bytes.
+
Tensors with allocation types other than kTfLiteDynamic will be ignored and a kTfLiteOk will be returned. tensor's internal data buffer will be assigned a pointer which can safely be passed to free or realloc if num_bytes is zero. If preserve_data is true, tensor data will be unchanged in the range from the start of the region up to the minimum of the old and new sizes. In the case of NULL tensor, or an error allocating new memory, returns kTfLiteError.
+
+
+
+
TfLiteTypeGetName
+
const char * TfLiteTypeGetName(
+ TfLiteType type
+)
+
+
Return the name of a given type, for error reporting purposes.
Type of delegate creation function used to allocate and construct a delegate.
+
The tflite_settings parameter passed to the delegate creation function should be a pointer to a FlatBuffer table object of type tflite::TFLiteSettings. We use const void * here rather than const tflite::TFLiteSettings* since this is a C API so we don't want to directly reference C++ types such as tflite::TFLiteSettings. But note that this address should point to the 'parsed' FlatBuffer object, not the raw byte buffer. (Note that 'parsing' FlatBuffers is very cheap, it's just an offset load.)
+
If you are using the FlatBuffers C API, then you can alternatively pass in a value of type tflite_TFLiteSettings_table_t, which is a typedef for const struct tflite_TFLiteSettings_table* that is the corresponding type for the 'parsed' FlatBuffer object in the FlatBuffers C API.
+
Ownership of the tflite_settings flatbuffer remains with the caller. The caller of a delegate creation function may end the lifetime of the tflite_settings FlatBuffer immediately after the call to the function. So the delegate creation function should ensure that any settings that the delegate may need to reference later, after the delegate has been constructed, are copied from the FlatBuffer into storage owned by the delegate.
This header file is for the delegate plugin for GPU.
+
Summary
+
For the C++ delegate plugin interface, the GPU delegate plugin is added to the DelegatePluginRegistry by the side effect of a constructor for a static object, so there's no public API needed for this plugin, other than the API of tflite::delegates::DelegatePluginRegistrys, which is declared in delegate_registry.h.
+
But to provide a C API to access the GPU delegate plugin, we do expose some functions, which are declared below.
the com.google.android.gms.tflite.java.TfLiteNative.initialize(Context context) or com.google.android.gms.tflite.java.TfLiteNative.initialize(Context context, TfLiteInitializationOptions options) methods defined in the Java API.
Checks whether the TFLite API has been initialized, throwing a Java exception otherwise.
+
+
+
+
Details
+
+
+
+
Parameters
+
+
+
+
+
+ env
+
+
+
The JNIEnv for the current thread (which has to be attached to the JVM).
+
+
+
+
+
+
+
+
+ Returns
+
+
+
Whether or not the TFLite API has been initialized. If this method returns false, no other JNI method should be called until the pending exception has been handled (typically by returning to Java).
+
+
+
+
+
+
+
+
GmsTfLiteErrorCodeVersionTooNew
+
bool GmsTfLiteErrorCodeVersionTooNew(
+ int error_code
+)
+
+
Returns true if the error code indicates the TFLite ABI version is too new.
+
In this case, the client should be updated to a newer version.
+
To avoid this error, make sure that your app is built against the latest version of the TFLite in Google Play Services client library code.
+
If TFLite is important for the functionality of the app, then we recommend that the calling code notify the user in this case. Suggested actions for the user could include:
bool GmsTfLiteErrorCodeVersionTooOld(
+ int error_code
+)
+
+
Returns true if the error code indicates that the TFLite ABI version is too old.
+
In this case, the TFLite in Google Play Services module should be updated to a newer version.
+
If TFLite is important for the functionality of the app, then we recommend that the calling code notify the user in this case. Suggested actions for the user could include:
+
Make sure your device is connected to the internet, and
int GmsTfLiteInitialize(
+ JNIEnv *env,
+ jobjecthandle
+)
+
+
Initialize TFLite with a handle acquired from Google Play Services API.
+
+This method (along with GmsTfLiteInitializeOrThrow()) can be called multiple times with the same handle; attempting to initialize with a different handle (without a call to GmsTfLiteShutdown() in between) will fail.
+
+
+
Details
+
+
+
+
Parameters
+
+
+
+
+
+ env
+
+
+
The JNIEnv for the current thread (which has to be attached to the JVM).
+
+
+
+
+ handle
+
+
+
An InternalNativeInitializationHandle object acquired through the Google Play Services API.
+
+
+
+
+
+
+
+
+ Returns
+
+
+
0 on success, or a non-zero error code on failure. The error codes are implementation-specific, but error conditions that clients may need to deal with can be tested using the GmsTfLiteErrorCodeVersionTooOld() and GmsTfLiteErrorCodeVersionTooNew() functions. Clients may also wish to log the specific error code for ease of debugging.
Initialize TFLite with a handle acquired from Google Play Services API, throwing a Java exception on failure.
+
+This method (along with GmsTfLiteInitialize()) can be called multiple times with the same handle; attempting to initialize with a different handle (without a call to GmsTfLiteShutdown() in between) will fail.
+
+
+
Details
+
+
+
+
Parameters
+
+
+
+
+
+ env
+
+
+
The JNIEnv for the current thread (which has to be attached to the JVM).
+
+
+
+
+ handle
+
+
+
An InternalNativeInitializationHandle object acquired through the Google Play Services API.
+
+
+
+
+
+
+
+
+ Returns
+
+
+
Whether or not initialization was successful. If this method returns false, no other JNI method should be called until the pending exception has been handled (typically by returning to Java).
+
+
+
+
+
+
+
+
GmsTfLiteShutdown
+
void GmsTfLiteShutdown(
+ void
+)
+
+
Resets the TFLite API.
+
After this method is called, the TFLite API will be unusable until a subsequent call to GmsTfLiteInitialize() or GmsTfLiteInitializeOrThrow(). This can be used to switch to a different version of the TFLite library.
This header file is for the delegate plugin for XNNPACK.
+
Summary
+
For the C++ delegate plugin interface, the XNNPACK delegate plugin is added to the DelegatePluginRegistry by the side effect of a constructor for a static object, so there's no public API needed for this plugin, other than the API of tflite::delegates::DelegatePluginRegistry, which is declared in delegate_registry.h.
+
But to provide a C API to access the XNNPACK delegate plugin, we do expose some functions, which are declared below.
This file declares types used by the pure C inference API defined in c_api.h, some of which are also used in the C++ and C kernel and interpreter APIs.
Parameters for asymmetric quantization across a dimension (i.e per output channel quantization).
+
Summary
+
quantized_dimension specifies which dimension the scales and zero_points correspond to. For a particular value in quantized_dimension, quantized values can be converted back to float using: real_value = scale * (quantized_value - zero_point)
TfLiteContext is a struct that is created by the TF Lite runtime and passed to the "methods" (C function pointers) in the TfLiteRegistration struct that are used to define custom ops and custom delegate kernels. It contains information and methods (C function pointers) that can be called by the code implementing a custom op or a custom delegate kernel. These methods provide access to the context in which that custom op or custom delegate kernel occurs, such as access to the input and output tensors for that op, as well as methods for allocating memory buffers and intermediate tensors, etc.
+
See also TfLiteOpaqueContext, which is an more ABI-stable equivalent.
+
+
+
+
Public attributes
+
+
+
+
+ AcquireSubgraphContext)(struct TfLiteContext *context, int subgraph_index, struct TfLiteContext **acquired_context)
+
Retrieves the corresponding TfLiteContext of a subgraph that the given subgraph_index points to and switches to the delegate context for that subgraph.
+
+
+
+
+ AddTensors)(struct TfLiteContext *, int tensors_to_add, int *first_new_tensor_index)
+
TfLiteStatus(* TfLiteContext::AcquireSubgraphContext)(struct TfLiteContext *context, int subgraph_index, struct TfLiteContext **acquired_context)
+
+
Retrieves the corresponding TfLiteContext of a subgraph that the given subgraph_index points to and switches to the delegate context for that subgraph.
+
If an invalid subgraph index is given, returns kTfLiteError.
+
NOTE: This function is expected to be paired with ReleaseSubgraphContext() once the delegate preparation is done and/or the delegate context functions are no longer needed.
+
WARNING: This is an experimental interface that is subject to change.
+
+
+
+
AddTensors
+
TfLiteStatus(* TfLiteContext::AddTensors)(struct TfLiteContext *, int tensors_to_add, int *first_new_tensor_index)
The execution plan contains a list of the node indices in execution order.
+
execution_plan->size is the current number of nodes. And, execution_plan->data[0] is the first node that needs to be run. TfLiteDelegates can traverse the current execution plan by iterating through each member of this array and using GetNodeAndRegistration() to access details about a node. i.e.
Note: the memory pointed by '*execution_plan is OWNED by TfLite runtime. Future calls to GetExecutionPlan invalidates earlier outputs. The following code snippet shows the issue of such an invocation pattern. After calling CheckNode, subsequent access to plan_1st is undefined.
Retrieves named metadata buffer from the TFLite model.
+
Returns kTfLiteOk if metadata is successfully obtained from the flatbuffer Model: that is, there exists a metadata entry with given name string. (see TFLite's schema.fbs). The corresponding buffer information is populated in ptr & bytes. The data from ptr is valid for the lifetime of the Interpreter.
+
WARNING: This is an experimental interface that is subject to change.
NOTE: The context owns the memory referenced by partition_params_array. It will be cleared with another call to PreviewDelegatePartitioning, or after TfLiteDelegateParams::Prepare returns.
+
WARNING: This is an experimental interface that is subject to change.
+
+
+
+
ReleaseSubgraphContext
+
TfLiteStatus(* TfLiteContext::ReleaseSubgraphContext)(struct TfLiteContext *context, int subgraph_index)
+
+
Releases the subgraph context by switching back to the TFLite kernel context for the subgraph that the given subgraph_index points to.
+
NOTE: This function is expected to be used after AcquireSubgraphContext() once the delegate preparation is done and/or the delegate context functions are no longer needed.
+
WARNING: This is an experimental interface that is subject to change.
Request that an error be reported with format string msg.
+
+
+
+
RequestScratchBufferInArena
+
TfLiteStatus(* TfLiteContext::RequestScratchBufferInArena)(struct TfLiteContext *ctx, size_t bytes, int *buffer_idx)
+
+
Request a scratch buffer in the arena through static memory planning.
+
This method is only available in Prepare stage and the buffer is allocated by the interpreter between Prepare and Eval stage. In Eval stage, GetScratchBuffer API can be used to fetch the address.
+
WARNING: This is an experimental interface that is subject to change.
Updates dimensions on the tensor. NOTE: ResizeTensor takes ownership of newSize.
+
+
+
+
ResizeTensorExplicit
+
TfLiteStatus(* TfLiteContext::ResizeTensorExplicit)(struct TfLiteContext *ctx, TfLiteTensor *tensor, int dims, const int *shape)
+
+
Resize the memory pointer of the tensor.
+
This method behaves the same as ResizeTensor, except that it makes a copy of the shape array internally so the shape array could be deallocated right afterwards.
+
WARNING: This is an experimental interface that is subject to change.
Defines a custom memory allocation not owned by the runtime.
+
Summary
+
data should be aligned to kDefaultTensorAlignment defined in lite/util.h. (Currently 64 bytes) NOTE: See Interpreter::SetCustomAllocationForTensor for details on usage.
WARNING: This is an experimental interface that is subject to change.
+
Summary
+
Currently, TfLiteDelegateParams has to be allocated in a way that it's trivially destructable. It will be stored as builtin_data field in TfLiteNode of the delegate node.
+
See also the CreateDelegateParams function in interpreter.cc details.
Copy the data from delegate buffer handle into raw memory of the given tensor.
+
Note that the delegate is allowed to allocate the raw bytes as long as it follows the rules for kTfLiteDynamic tensors, in which case this cannot be null.
Note: This only frees the handle, but this doesn't release the underlying resource (e.g. textures). The resources are either owned by application layer or the delegate. This can be null if the delegate doesn't use its own buffer.
This prepare is called, giving the delegate a view of the current graph through TfLiteContext*. It typically will look at the nodes and call ReplaceNodeSubsetsWithDelegateKernels() to ask the TensorFlow lite runtime to create macro-nodes to represent delegated subgraphs of the original graph.
+
+
+
+
data_
+
void * TfLiteDelegate::data_
+
+
Data that delegate needs to identify itself.
+
This data is owned by the delegate. The delegate is owned in the user code, so the delegate is responsible for deallocating this when it is destroyed.
+
+
+
+
flags
+
int64_t TfLiteDelegate::flags
+
+
Bitmask flags. See the comments in TfLiteDelegateFlags.
The opaque delegate builder associated with this object.
+
If set then the TF Lite runtime will give precedence to this field. E.g. instead of invoking Prepare via the function pointer inside the TfLiteDelegate object, the runtime will first check if the corresponding function pointer inside opaque_delegate_builder is set and if so invoke that.
+
If this field is non-null, then the Prepare field (of the TfLiteDelegate) should be null.
An external context is a collection of information unrelated to the TF Lite framework, but useful to a subset of the ops.
+
Summary
+
TF Lite knows very little about the actual contexts, but it keeps a list of them, and is able to refresh them if configurations like the number of recommended threads change.
TfLiteOpaqueDelegateBuilder is used for constructing TfLiteOpaqueDelegate, see TfLiteOpaqueDelegateCreate below.
+
Summary
+
Note: This struct is not ABI stable.
+
For forward source compatibility TfLiteOpaqueDelegateBuilder objects should be brace-initialized, so that all fields (including any that might be added in the future) get zero-initialized. The purpose of each field is exactly the same as with TfLiteDelegate.
+
WARNING: This is an experimental interface that is subject to change.
Copies the data from delegate buffer handle into raw memory of the given tensor.
+
Note that the delegate is allowed to allocate the raw bytes as long as it follows the rules for kTfLiteDynamic tensors, in which case this cannot be null.
Note: This only frees the handle, but this doesn't release the underlying resource (e.g. textures). The resources are either owned by application layer or the delegate. This can be null if the delegate doesn't use its own buffer.
This prepare is called, giving the delegate a view of the current graph through TfLiteContext*. It typically will look at the nodes and call ReplaceNodeSubsetsWithDelegateKernels() to ask the TensorFlow lite runtime to create macro-nodes to represent delegated subgraphs of the original graph.
+
+
+
+
data
+
void * TfLiteOpaqueDelegateBuilder::data
+
+
Data that delegate needs to identify itself.
+
This data is owned by the delegate. The delegate is owned in the user code, so the delegate is responsible for deallocating this when it is destroyed.
+
+
+
+
flags
+
int64_t TfLiteOpaqueDelegateBuilder::flags
+
+
Bitmask flags. See the comments in TfLiteDelegateFlags.
WARNING: This is an experimental interface that is subject to change.
+
Summary
+
Currently, TfLiteOpaqueDelegateParams has to be allocated in a way that it's trivially destructable. It will be stored as builtin_data field in TfLiteNode of the delegate node.
+
See also the CreateOpaqueDelegateParams function in subgraph.cc details.
Will be deprecated in favor of TfLiteAffineQuantization. If per-layer quantization is specified this field will still be populated in addition to TfLiteAffineQuantization. Parameters for asymmetric quantization. Quantized values can be converted back to float using: real_value = scale * (quantized_value - zero_point)
If the async_kernel field is nullptr, it means the operation described by this TfLiteRegistration object does not support asynchronous execution. Otherwise, the function that the field points to should only be called for delegate kernel nodes, i.e. node should be a delegate kernel node created by applying a delegate. If the function returns nullptr, that means that the underlying delegate does not support asynchronous execution for this node.
+
+
+
+
builtin_code
+
int32_t TfLiteRegistration::builtin_code
+
+
Builtin codes.
+
If this kernel refers to a builtin this is the code of the builtin. This is so we can do marshaling to other frameworks like NN API.
+
Note: It is the responsibility of the registration binder to set this properly.
+
+
+
+
custom_name
+
const char * TfLiteRegistration::custom_name
+
+
Custom op name.
+
If the op is a builtin, this will be null.
+
Note: It is the responsibility of the registration binder to set this properly.
+
WARNING: This is an experimental interface that is subject to change.
profiling_string is called during summarization of profiling information in order to group executions together.
+
Providing a value here will cause a given op to appear multiple times is the profiling report. This is particularly useful for custom ops that can perform significantly different calculations depending on their user-data.
Since we can't use internal types (such as TfLiteContext) for C API to maintain ABI stability. C API user will provide TfLiteRegistrationExternal to implement custom ops. We keep it inside of TfLiteRegistration and use it to route callbacks properly.
+
+
+
+
version
+
int TfLiteRegistration::version
+
+
The version of the op.
+
Note: It is the responsibility of the registration binder to set this properly.
An integer buffer handle that can be handled by delegate.
+
The value is valid only when delegate is not null.
+
WARNING: This is an experimental interface that is subject to change.
+
+
+
+
bytes
+
size_t TfLiteTensor::bytes
+
+
The number of bytes required to store the data of this Tensor.
+
I.e. (bytes of each element) * dims[0] * ... * dims[n-1]. For example, if type is kTfLiteFloat32 and dims = {3, 2} then bytes = sizeof(float) * 3 * 2 = 4 * 3 * 2 = 24.
The appropriate type should be used for a typed tensor based on type.
+
+
+
+
data_is_stale
+
bool TfLiteTensor::data_is_stale
+
+
If the delegate uses its own buffer (e.g.
+
GPU memory), the delegate is responsible to set data_is_stale to true. delegate->CopyFromBufferHandle can be called to copy the data from delegate buffer.
+
WARNING: This is an experimental interface that is subject to change.
Encodes shapes with unknown dimensions with -1. This field is only populated when unknown dimensions exist in a read-write tensor (i.e. an input or output tensor). (e.g. dims contains [1, 1, 1, 3] and dims_signature contains [1, -1, -1, 3]). If no unknown dimensions exist then dims_signature is either null, or set to an empty array. Note that this field only exists when TF_LITE_STATIC_MEMORY is not defined.
Allocation(
+ ErrorReporter *error_reporter,
+ Type type
+)
+
+
+
diff --git a/site/en/lite/api_docs/cc/class/tflite/error-reporter.html b/site/en/lite/api_docs/cc/class/tflite/error-reporter.html
new file mode 100644
index 00000000000..6d9e58bfa87
--- /dev/null
+++ b/site/en/lite/api_docs/cc/class/tflite/error-reporter.html
@@ -0,0 +1,95 @@
+
+
+
+
+tflite::ErrorReporter Class Reference
+
+
+
tflite::ErrorReporter
This is an abstract class.
#include <error_reporter.h>
A functor that reports error to supporting system.
Summary
Invoked similar to printf.
Usage: ErrorReporter foo; foo.Report("test %d", 5); or va_list args; foo.Report("test %d", args); // where args is va_list
Subclass ErrorReporter to provide another reporting destination. For example, if you have a GUI program, you might redirect to a buffer that drives a GUI error log box.
The additional void* parameter is unused. This method is for compatibility with macros that takes TfLiteContext, like TF_LITE_ENSURE and related macros.
+
+
+
~ErrorReporter
+
virtual ~ErrorReporter()=default
+
+
+
diff --git a/site/en/lite/api_docs/cc/class/tflite/file-copy-allocation.html b/site/en/lite/api_docs/cc/class/tflite/file-copy-allocation.html
new file mode 100644
index 00000000000..e625112b1d2
--- /dev/null
+++ b/site/en/lite/api_docs/cc/class/tflite/file-copy-allocation.html
@@ -0,0 +1,89 @@
+
+
+
+
+tflite::FileCopyAllocation Class Reference
+
+
+
Builds a model directly from a flatbuffer pointer Caller retains ownership of the buffer and should keep it alive until the returned object is destroyed.
Ownership of the allocation is passed to the model, but the caller retains ownership of error_reporter and must ensure its lifetime is longer than the FlatBufferModel instance. Returns a nullptr in case of failure (e.g., the allocation is invalid).
Caller retains ownership of the buffer and should keep it alive until the returned object is destroyed. Caller also retains ownership of error_reporter and must ensure its lifetime is longer than the FlatBufferModel instance. Returns a nullptr in case of failure. NOTE: this does NOT validate the buffer so it should NOT be called on invalid/untrusted input. Use VerifyAndBuildFromBuffer in that case
Caller retains ownership of error_reporter and must ensure its lifetime is longer than the FlatBufferModel instance. Returns a nullptr in case of failure.
Builds a model directly from a flatbuffer pointer Caller retains ownership of the buffer and should keep it alive until the returned object is destroyed.
+
Caller retains ownership of error_reporter and must ensure its lifetime is longer than the FlatBufferModel instance. Returns a nullptr in case of failure.
Verifies whether the content of the allocation is legit, then builds a model based on the provided allocation.
+
The extra_verifier argument is an additional optional verifier for the buffer. By default, we always check with tflite::VerifyModelBuffer. If extra_verifier is supplied, the buffer is checked against the extra_verifier after the check against tflite::VerifyModelBuilder. Ownership of the allocation is passed to the model, but the caller retains ownership of error_reporter and must ensure its lifetime is longer than the FlatBufferModel instance. Returns a nullptr in case of failure.
Verifies whether the content of the buffer is legit, then builds a model based on the pre-loaded flatbuffer.
+
The extra_verifier argument is an additional optional verifier for the buffer. By default, we always check with tflite::VerifyModelBuffer. If extra_verifier is supplied, the buffer is checked against the extra_verifier after the check against tflite::VerifyModelBuilder. The caller retains ownership of the buffer and should keep it alive until the returned object is destroyed. Caller retains ownership of error_reporter and must ensure its lifetime is longer than the FlatBufferModel instance. Returns a nullptr in case of failure.
Verifies whether the content of the file is legit, then builds a model based on the file.
+
The extra_verifier argument is an additional optional verifier for the file contents. By default, we always check with tflite::VerifyModelBuffer. If extra_verifier is supplied, the file contents is also checked against the extra_verifier after the check against tflite::VerifyModelBuilder. Caller retains ownership of error_reporter and must ensure its lifetime is longer than the FlatBufferModel instance. Returns a nullptr in case of failure.
+
+
+
Public functions
+
+
CheckModelIdentifier
+
bool CheckModelIdentifier() const
+
+
Returns true if the model identifier is correct (otherwise false and reports an error).
Any delegates added with AddDelegate will be applied to the Interpreter generated by operator(), in the order that they were added.
+
(The delegate parameter passed to AddDelegate should be non-null, otherwise an error will be reported, and the call to AddDelegate will have no other effect.) The lifetime of the delegate must be at least as long as the lifetime of any Interpreter generated by this InterpreterBuilder.
Builds an interpreter given only the raw flatbuffer Model object (instead of a FlatBufferModel).
+
Mostly used for testing. If error_reporter is null, then DefaultErrorReporter() is used. options object is copied during construction. So caller can release it
The capacity headroom of tensors_ vector before calling ops' prepare and invoke function.
+
In these functions, it's guaranteed allocating up to kTensorsCapacityHeadroom more tensors won't invalidate pointers to existing tensors.
+
+
+
+
kTensorsReservedCapacity
+
constexpr int kTensorsReservedCapacity = 128
+
+
+
Friend classes
+
+
tflite::impl::InterpreterBuilder
+
friend class tflite::impl::InterpreterBuilder
+
+
+
Public functions
+
+
AddProfiler
+
void AddProfiler(
+ Profiler *profiler
+)
+
+
\warning This is an experimental API and subject to change.
+
\n Adds the profiler to tracing execution. The caller retains ownership of the profiler and must ensure its validity. nullptr profiler will be ignored.
\warning This is an experimental API and subject to change.
+
\n Adds the profiler to tracing execution. Transfers ownership of the profiler to the interpreter. nullptr profiler will be ignored.
+
+
+
+
AllocateTensors
+
TfLiteStatus AllocateTensors()
+
+
Update allocations for all tensors.
+
This will redim dependent tensors using the input tensor dimensionality as given. This is relatively expensive. This must be called after the interpreter has been created and before running inference (and accessing tensor buffers), and must be called again if (and only if) an input tensor is resized. Returns status of success or failure. Will fail if any of the ops in the model (other than those which were rewritten by delegates, if any) are not supported by the Interpreter's OpResolver.
\warning This is an experimental API and subject to change.
+
\n Apply InterpreterOptions which tunes behavior of the interpreter.
+
+
+
+
Cancel
+
TfLiteStatus Cancel()
+
+
\warning This is an experimental API and subject to change.
+
\n Attempts to cancel in flight invocation if any. This will not affect Invokes that happends after the cancellation. Non blocking. Thread safe. Returns kTfLiteError if cancellation is not enabled, otherwise returns kTfLiteOk.
+
+
+
+
EnsureTensorDataIsReadable
+
TfLiteStatus EnsureTensorDataIsReadable(
+ int tensor_index
+)
+
+
\warning This is an experimental API and subject to change.
+
\n Ensure the data in tensor.data is readable. In case delegate is used, it might require to copy the data from delegate buffer to raw memory.
+
+
+
+
GetAllowFp16PrecisionForFp32
+
bool GetAllowFp16PrecisionForFp32() const
+
+
\warning Experimental interface, subject to change.
\warning Experimental interface, subject to change.
+
\n Returns a pointer to the AsyncSignatureRunner instance to run the part of the graph identified by a SignatureDef. The nullptr is returned if the given signature key is not valid. The async delegate should be applied before calling this function.
\warning Experimental interface, subject to change.
+
\n Returns a pointer to the SignatureRunner instance to run the part of the graph identified by a SignatureDef. The nullptr is returned if the given signature key is not valid. If you need to specify delegates, you have to do that before calling this function. This function will additionally apply default delegates. Thus, applying delegates after that might lead to undesirable behaviors. Note, the pointed instance has lifetime same as the Interpreter object and the SignatureRunner class is not thread-safe.
+
+
+
+
GetSubgraphIndexFromSignature
+
int GetSubgraphIndexFromSignature(
+ const char *signature_key
+) const
+
+
\warning Experimental interface, subject to change.
+
\n Return the subgraph index that corresponds to a SignatureDef, defined by 'signature_key'. If invalid name passed, -1 will be returned.
Invoke the interpreter (run the whole graph in dependency order).
+
NOTE: It is possible that the interpreter is not in a ready state to evaluate (i.e. if a ResizeTensor() has been performed without an AllocateTensors(). Returns status of success or failure.
Allow a delegate to look at the graph and modify the graph to handle parts of the graph themselves.
+
After this is called, the graph may contain new nodes that replace 1 more nodes. 'delegate' must outlive the interpreter. Returns one of the following status codes:
+
kTfLiteOk: Success.
+
kTfLiteDelegateError: Delegation failed due to an error in the delegate, or the delegate parameter was null. The Interpreter has been restored to its pre-delegation state. NOTE: This undoes all delegates previously applied to the Interpreter.
+
kTfLiteApplicationError : Delegation failed to be applied due to the incompatibility with the TfLite runtime, e.g., the model graph is already immutable when applying the delegate. However, the interpreter could still be invoked.
+
kTfLiteUnresolvedOps: Delegation failed because the model has an operator that cannot be resolved. This can happen when the op is not registered or built with the TF Lite framework.
+
kTfLiteError: Unexpected/runtime failure. \n \warning This is an experimental API and subject to change. \n
TfLiteDelegate is a C structure, so it has no virtual destructor. The default deleter of the unique_ptr does not know how to delete C++ objects deriving from TfLiteDelegate.
Retrieve an operator's description of its work, for profiling purposes.
+
+
+
+
ReleaseNonPersistentMemory
+
TfLiteStatus ReleaseNonPersistentMemory()
+
+
\warning Experimental interface, subject to change.
+
\n This releases memory held by non-persistent tensors. It does NOT re-perform memory planning. AllocateTensors needs to be called before next invocation.
+
+
+
+
ResetVariableTensors
+
TfLiteStatus ResetVariableTensors()
+
+
\warning This is an experimental API and subject to change.
+
\n Reset all variable tensors to the default value. If a variable tensor doesn't have a buffer, reset it to zero. TODO(b/115961645): Implement - If a variable tensor has a buffer, reset it to the value of the buffer.
+
+
+
+
ResizeInputTensor
+
TfLiteStatus ResizeInputTensor(
+ int tensor_index,
+ const std::vector< int > & dims
+)
+
+
Change the dimensionality of a given tensor.
+
Note, this is only acceptable for tensor indices that are inputs or variables. Returns status of failure or success. Note that this doesn't actually resize any existing buffers. A call to AllocateTensors() is required to change the tensor input buffer.
+
+
+
+
ResizeInputTensorStrict
+
TfLiteStatus ResizeInputTensorStrict(
+ int tensor_index,
+ const std::vector< int > & dims
+)
+
+
Change the dimensionality of a given tensor.
+
This is only acceptable for tensor indices that are inputs or variables. Only unknown dimensions can be resized with this function. Unknown dimensions are indicated as -1 in the dims_signature attribute of a TfLiteTensor. Returns status of failure or success. Note that this doesn't actually resize any existing buffers. A call to AllocateTensors() is required to change the tensor input buffer.
\warning This is an experimental API and subject to change.
+
\n Set if buffer handle output is allowed.
+
When using hardware delegation, Interpreter will make the data of output tensors available in tensor->data by default. If the application can consume the buffer handle directly (e.g. reading output from OpenGL texture), it can set this flag to false, so Interpreter won't copy the data from buffer handle to CPU memory.
Allow float16 precision for FP32 calculation when possible.
+
Default: not allow.
+
WARNING: This API is deprecated: prefer controlling this via delegate options, e.g. `tflite::StatefulNnApiDelegate::Options::allow_fp16' or TfLiteGpuDelegateOptionsV2::is_precision_loss_allowed. This method will be removed in a future release.
\warning This is an experimental API and subject to change.
+
\n Set the delegate buffer handle to a tensor. It can be called in the following cases:
+
Set the buffer handle to a tensor that's not being written by a delegate. For example, feeding an OpenGL texture as the input of the inference graph.
+
Set the buffer handle to a tensor that uses the same delegate. For example, set an OpenGL texture as the output of inference, while the node which produces output is an OpenGL delegate node.
\warning This is an experimental API and subject to change.
+
\n Sets the cancellation function pointer in order to cancel a request in the middle of a call to Invoke(). The interpreter queries this function during inference, between op invocations; when it returns true, the interpreter will abort execution and return kTfLiteError. The data parameter contains any data used by the cancellation function, and if non-null, remains owned by the caller.
Assigns (or reassigns) a custom memory allocation for the given tensor.
+
flags is a bitmask, see TfLiteCustomAllocationFlags. The runtime does NOT take ownership of the underlying memory.
+
NOTE: User needs to call AllocateTensors() after this. Invalid/insufficient buffers will cause an error during AllocateTensors or Invoke (in case of dynamic shapes in the graph).
+
Parameters should satisfy the following conditions:
+
tensor->allocation_type == kTfLiteArenaRw or kTfLiteArenaRwPersistent In general, this is true for I/O tensors & variable tensors.
+
allocation->data has the appropriate permissions for runtime access (Read-only for inputs, Read-Write for others), and outlives Interpreter.
+
allocation->bytes >= tensor->bytes. This condition is checked again if any tensors are resized.
+
allocation->data should be aligned to kDefaultTensorAlignment defined in lite/util.h. (Currently 64 bytes) This check is skipped if kTfLiteCustomAllocationFlagsSkipAlignCheck is set through flags. \warning This is an experimental API and subject to change. \n
Set the number of threads available to the interpreter.
+
NOTE: num_threads should be >= -1. Setting num_threads to 0 has the effect to disable multithreading, which is equivalent to setting num_threads to 1. If set to the value -1, the number of threads used will be implementation-defined and platform-dependent.
+
As TfLite interpreter could internally apply a TfLite delegate by default (i.e. XNNPACK), the number of threads that are available to the default delegate should be set via InterpreterBuilder APIs as follows:
WARNING: This API is deprecated: prefer using InterpreterBuilder::SetNumThreads, as documented above.
+
+
+
+
SetProfiler
+
void SetProfiler(
+ Profiler *profiler
+)
+
+
\warning This is an experimental API and subject to change.
+
\n Sets the profiler to tracing execution. The caller retains ownership of the profiler and must ensure its validity. Previously registered profilers will be unregistered. If profiler is nullptr, all previously installed profilers will be removed.
\warning This is an experimental API and subject to change.
+
\n Same as SetProfiler except this interpreter takes ownership of the provided profiler. Previously registered profilers will be unregistered. If profiler is nullptr, all previously installed profilers will be removed.
\warning Experimental interface, subject to change.
+
\n Returns the mapping of inputs to tensor index in the signature specified through 'signature_key'. If invalid name passed, an empty list will be returned.
\warning Experimental interface, subject to change.
+
\n Returns the mapping of outputs to tensor index in the signature specified through 'signature_key'. If invalid name passed, an empty list will be returned.
+
+
+
+
tensor
+
TfLiteTensor * tensor(
+ int tensor_index
+)
+
+
Get a mutable tensor data structure.
+
+
+
+
tensor
+
const TfLiteTensor * tensor(
+ int tensor_index
+) const
+
+
Get an immutable tensor data structure.
+
+
+
+
tensors_size
+
size_t tensors_size() const
+
+
Return the number of tensors in the model.
+
+
+
+
typed_input_tensor
+
T * typed_input_tensor(
+ int index
+)
+
+
Return a mutable pointer into the data of a given input tensor.
+
The given index must be between 0 and inputs().size().
+
+
+
+
typed_input_tensor
+
const T * typed_input_tensor(
+ int index
+) const
+
+
Return an immutable pointer into the data of a given input tensor.
+
The given index must be between 0 and inputs().size().
+
+
+
+
typed_output_tensor
+
T * typed_output_tensor(
+ int index
+)
+
+
Return a mutable pointer into the data of a given output tensor.
+
The given index must be between 0 and outputs().size().
+
+
+
+
typed_output_tensor
+
const T * typed_output_tensor(
+ int index
+) const
+
+
Return an immutable pointer into the data of a given output tensor.
+
The given index must be between 0 and outputs().size().
+
+
+
+
typed_tensor
+
T * typed_tensor(
+ int tensor_index
+)
+
+
Perform a checked cast to the appropriate tensor type (mutable pointer version).
+
+
+
+
typed_tensor
+
const T * typed_tensor(
+ int tensor_index
+) const
+
+
Perform a checked cast to the appropriate tensor type (immutable pointer version).
+
+
+
+
variables
+
const std::vector< int > & variables() const
+
+
Read only access to list of variable tensors.
+
+
+
+
~Interpreter
+
~Interpreter()
+
+
+
+
diff --git a/site/en/lite/api_docs/cc/class/tflite/m-m-a-p-allocation.html b/site/en/lite/api_docs/cc/class/tflite/m-m-a-p-allocation.html
new file mode 100644
index 00000000000..ad1e1c8be3e
--- /dev/null
+++ b/site/en/lite/api_docs/cc/class/tflite/m-m-a-p-allocation.html
@@ -0,0 +1,286 @@
+
+
+
+
+tflite::MMAPAllocation Class Reference
+
+
+
tflite::MMAPAllocation
#include <allocation.h>
Note that not all platforms support MMAP-based allocation.
Registers all operator versions supported by another MutableOpResolver.
+
Replaces any previous registrations for the same operator versions, except that registrations made with AddBuiltin or AddCustom always take precedence over registrations made with ChainOpResolver.
+
+
+
AddBuiltin
+
void AddBuiltin(
+ tflite::BuiltinOperator op,
+ const TfLiteRegistration *registration,
+ int version
+)
+
+
Registers the specified version of the specified builtin operator op.
+
Replaces any previous registration for the same operator version.
+
+
+
AddBuiltin
+
void AddBuiltin(
+ tflite::BuiltinOperator op,
+ const TfLiteRegistration *registration,
+ int min_version,
+ int max_version
+)
+
+
Registers the specified version range (versions min_version to max_version, inclusive) of the specified builtin operator op.
+
Replaces any previous registration for the same operator version.
+
+
+
AddCustom
+
void AddCustom(
+ const char *name,
+ const TfLiteRegistration *registration,
+ int version
+)
+
+
Registers the specified version of the specified builtin operator op.
+
Replaces any previous registration for the same operator version.
+
+
+
AddCustom
+
void AddCustom(
+ const char *name,
+ const TfLiteRegistration *registration,
+ int min_version,
+ int max_version
+)
+
+
Registers the specified version range (versions min_version to max_version, inclusive) of the specified custom operator name.
+
Replaces any previous registration for the same operator version.
+
+
+
FindOp
+
virtual const TfLiteRegistration * FindOp(
+ tflite::BuiltinOperator op,
+ int version
+) const override
+
+
Finds the op registration for a builtin operator by enum code.
+
+
+
FindOp
+
virtual const TfLiteRegistration * FindOp(
+ const char *op,
+ int version
+) const override
+
+
Finds the op registration of a custom operator by op name.
+
+
+
GetDelegateCreators
+
virtual OpResolver::TfLiteDelegateCreators GetDelegateCreators() const final
+
+
+
GetOpaqueDelegateCreators
+
virtual OpResolver::TfLiteOpaqueDelegateCreators GetOpaqueDelegateCreators() const final
Registers all operator versions supported by another OpResolver, except any already registered in this MutableOpResolver.
+
other must point to an OpResolver whose lifetime is at least as long as the lifetime of the MutableOpResolver pointed to by this. The OpResolver pointed to by other should not be modified during the lifetime of this MutableOpResolver.
+
+
+
diff --git a/site/en/lite/api_docs/cc/class/tflite/op-resolver.html b/site/en/lite/api_docs/cc/class/tflite/op-resolver.html
new file mode 100644
index 00000000000..25f35a2af28
--- /dev/null
+++ b/site/en/lite/api_docs/cc/class/tflite/op-resolver.html
@@ -0,0 +1,193 @@
+
+
+
+
+tflite::OpResolver Class Reference
+
+
+
tflite::OpResolver
This is an abstract class.
#include <op_resolver.h>
Abstract interface that returns TfLiteRegistrations given op codes or custom op names.
Summary
This is the mechanism that ops being referenced in the flatbuffer model are mapped to executable function pointers (TfLiteRegistrations).
This provides a few C++ helpers that are useful for manipulating C structures in C++.
+
Main abstraction controlling the tflite interpreter. Do NOT include this file directly, instead include third_party/tensorflow/lite/interpreter.h See third_party/tensorflow/lite/c/common.h for the API for defining operations (TfLiteRegistration).
+
Provides functionality to construct an interpreter for a model.
+
WARNING: Users of TensorFlow Lite should not include this file directly, but should instead include "third_party/tensorflow/lite/interpreter_builder.h". Only the TensorFlow Lite implementation itself should include this file directly.
+
Deserialization infrastructure for tflite. Provides functionality to go from a serialized tflite model in flatbuffer format to an in-memory representation of the model.
+
WARNING: Users of TensorFlow Lite should not include this file directly, but should instead include "third_party/tensorflow/lite/model_builder.h". Only the TensorFlow Lite implementation itself should include this file directly.
An interpreter for a graph of nodes that input and output from tensors.
+
Each node of the graph processes a set of input tensors and produces a set of output Tensors. All inputs/output tensors are referenced by index.
+
Usage:
+
+
+// Create model from file. Note that the model instance must outlive the
+// interpreter instance.
+auto model = tflite::FlatBufferModel::BuildFromFile(...);
+if (model == nullptr) {
+ // Return error.
+}
+// Create an Interpreter with an InterpreterBuilder.
+std::unique_ptr interpreter;
+tflite::ops::builtin::BuiltinOpResolver resolver;
+if (InterpreterBuilder(*model, resolver)(&interpreter) != kTfLiteOk) {
+ // Return failure.
+}
+if (interpreter->AllocateTensors() != kTfLiteOk) {
+ // Return failure.
+}
+
+
+
auto input = interpreter->typed_tensor(0);
+for (int i = 0; i < input_size; i++) {
+ input[i] = ...; interpreter->Invoke();
+
+
+
Note: For nearly all practical use cases, one should not directly construct an Interpreter object, but rather use the InterpreterBuilder.
+
\warning This class is not thread-safe. The client is responsible for ensuring serialized interaction to avoid data races and undefined behavior.
+
+
+
+
InterpreterBuilder
+
impl::InterpreterBuilder InterpreterBuilder
+
+
Build an interpreter capable of interpreting model.
+
+
+
model: A model whose lifetime must be at least as long as any interpreter(s) created by the builder. In principle multiple interpreters can be made from a single model.
+
op_resolver: An instance that implements the OpResolver interface, which maps custom op names and builtin op codes to op registrations. The lifetime of the provided op_resolver object must be at least as long as the InterpreterBuilder; unlike model and error_reporter, the op_resolver does not need to exist for the duration of any created Interpreter objects.
+
error_reporter: a functor that is called to report errors that handles printf var arg semantics. The lifetime of the error_reporter object must be greater than or equal to the Interpreter created by operator().
+
options_experimental: Options that can change behavior of interpreter. WARNING: this parameter is an experimental API and is subject to change.
+
+
+
Returns a kTfLiteOk when successful and sets interpreter to a valid Interpreter. Note: The user must ensure the lifetime of the model (and error reporter, if provided) is at least as long as interpreter's lifetime, and a single model instance may safely be used with multiple interpreters.
An RAII object that represents a read-only tflite model, copied from disk, or mmapped.
+
Summary
+
This uses flatbuffers as the serialization format.
+
NOTE: The current API requires that a FlatBufferModel instance be kept alive by the client as long as it is in use by any dependent Interpreter instances. As the FlatBufferModel instance is effectively immutable after creation, the client may safely use a single model with multiple dependent Interpreter instances, even across multiple threads (though note that each Interpreter instance is not thread-safe).
+
+
+using namespace tflite;
+StderrReporter error_reporter;
+auto model = FlatBufferModel::BuildFromFile("interesting_model.tflite",
+ &error_reporter);
+MyOpResolver resolver; // You need to subclass OpResolver to provide
+ // implementations.
+InterpreterBuilder builder(*model, resolver);
+std::unique_ptr interpreter;
+if(builder(&interpreter) == kTfLiteOk) {
+ .. run model inference with interpreter
+}
+
+
+
OpResolver must be defined to provide your kernel implementations to the interpreter. This is environment specific and may consist of just the builtin ops, or some custom operators you defined to extend tflite.
Indicates that this object (class, method, etc) should be retained and not renamed when
+ generating the SDK, but should be allowed to be stripped or renamed in end developer apps.
Wrapper for a native TensorFlow Lite Delegate.
+
+
If a delegate implementation holds additional resources or memory that should be explicitly
+ freed, then best practice is to add a close() method to the implementation and have the
+ client call that explicitly when the delegate instance is no longer in use. While this approach
+ technically allows sharing of a single delegate instance across multiple interpreter instances,
+ the delegate implementation must explicitly support this.
+
Returns a native handle to the TensorFlow Lite delegate implementation.
+
+
+
+
+
+
+
+
Inherited Methods
+
+
+ From interface
+ java.io.Closeable
+
+
+
+
+
+
+
+
+ abstract
+
+
+
+
+ void
+
+
+
+close()
+
+
+
+
+
+
+
+
+
+
+ From interface
+ java.lang.AutoCloseable
+
+
+
+
+
+
+
+
+ abstract
+
+
+
+
+ void
+
+
+
+close()
+
+
+
+
+
+
+
+
+
+
+
+
+
+
+
+
+
+
+
+
+
+
+
+
Public Methods
+
+
+
+
+ public
+
+
+
+
+ void
+
+close
+()
+
+
+
+
+
+
Closes the delegate and releases any resources associated with it.
+
+
In contrast to the method declared in the base Closeable interface, this method
+ does not throw checked exceptions.
+
+
+
+
+
+
+
+ public
+
+
+ abstract
+
+ long
+
+getNativeHandle
+()
+
+
+
+
+
+
Returns a native handle to the TensorFlow Lite delegate implementation.
+
+
Note: The Java Delegate maintains ownership of the native delegate instance, and
+ must ensure its existence for the duration of usage with any InterpreterApi instance.
+
+
Note: the native delegate instance may not be created until the delegate has been attached
+ to an interpreter, so this method should not be called until after an interpreter has been
+ constructed with this delegate.
+
+
Returns
+
The native delegate handle. In C/C++, this should be a pointer to
+ 'TfLiteOpaqueDelegate'.
+
Note for developers implementing this interface: Currently TF Lite in Google Play Services
+ does not support external (developer-provided) delegates. Correspondingly, implementations of
+ this method can expect to be called with RuntimeFlavor.APPLICATION.
+
Advanced: Set if buffer handle output is allowed.
+
+
When a Delegate supports hardware acceleration, the interpreter will make the data
+ of output tensors available in the CPU-allocated tensor buffers by default. If the client can
+ consume the buffer handle directly (e.g. reading output from OpenGL texture), it can set this
+ flag to false, avoiding the copy of data to the CPU buffer. The delegate documentation should
+ indicate whether this is supported and how it can be used.
+
+
WARNING: This is an experimental interface that is subject to change.
+
Advanced: Set if the interpreter is able to be cancelled.
+
+
Interpreters may have an experimental API setCancelled(boolean).
+ If this interpreter is cancellable and such a method is invoked, a cancellation flag will be
+ set to true. The interpreter will check the flag between Op invocations, and if it's true, the interpreter will stop execution. The interpreter will remain a cancelled state
+ until explicitly "uncancelled" by setCancelled(false).
+
Sets the number of threads to be used for ops that support multi-threading.
+
+
numThreads should be >= -1. Setting numThreads to 0 has the effect
+ of disabling multithreading, which is equivalent to setting numThreads to 1. If
+ unspecified, or set to the value -1, the number of threads used will be
+ implementation-defined and platform-dependent.
+
Driver class to drive model inference with TensorFlow Lite.
+
+
Note: If you don't need access to any of the "experimental" API features below, prefer to use
+ InterpreterApi and InterpreterFactory rather than using Interpreter directly.
+
+
A Interpreter encapsulates a pre-trained TensorFlow Lite model, in which operations
+ are executed for model inference.
+
+
For example, if a model takes only one input and returns only one output:
+
+
String[] input = {"foo", "bar"}; // Input tensor shape is [2].
+ String[][] output = new String[3][2]; // Output tensor shape is [3, 2].
+ try (Interpreter interpreter = new Interpreter(file_of_a_tensorflowlite_model)) {
+ interpreter.runForMultipleInputsOutputs(input, output);
+ }
+
+
Note that there's a distinction between shape [] and shape[1]. For scalar string tensor
+ outputs:
+
+
String[] input = {"foo"}; // Input tensor shape is [1].
+ ByteBuffer outputBuffer = ByteBuffer.allocate(OUTPUT_BYTES_SIZE); // Output tensor shape is [].
+ try (Interpreter interpreter = new Interpreter(file_of_a_tensorflowlite_model)) {
+ interpreter.runForMultipleInputsOutputs(input, outputBuffer);
+ }
+ byte[] outputBytes = new byte[outputBuffer.remaining()];
+ outputBuffer.get(outputBytes);
+ // Below, the `charset` can be StandardCharsets.UTF_8.
+ String output = new String(outputBytes, charset);
+
+
Orders of inputs and outputs are determined when converting TensorFlow model to TensorFlowLite
+ model with Toco, as are the default shapes of the inputs.
+
+
When inputs are provided as (multi-dimensional) arrays, the corresponding input tensor(s) will
+ be implicitly resized according to that array's shape. When inputs are provided as Buffer
+ types, no implicit resizing is done; the caller must ensure that the Buffer byte size
+ either matches that of the corresponding tensor, or that they first resize the tensor via resizeInput(int, int[]). Tensor shape and type information can be obtained via the Tensor class, available via getInputTensor(int) and getOutputTensor(int).
+
+
WARNING:Interpreter instances are not thread-safe. A Interpreter
+ owns resources that must be explicitly freed by invoking close()
+
The TFLite library is built against NDK API 19. It may work for Android API levels below 19,
+ but is not guaranteed.
+
Initializes an Interpreter with a ByteBuffer of a model file.
+
+
The ByteBuffer should not be modified after the construction of a Interpreter. The
+ ByteBuffer can be either a MappedByteBuffer that memory-maps a model file, or a
+ direct ByteBuffer of nativeOrder() that contains the bytes content of a model.
Initializes an Interpreter with a ByteBuffer of a model file and a set of
+ custom Interpreter.Options.
+
+
The ByteBuffer should not be modified after the construction of an Interpreter. The ByteBuffer can be either a MappedByteBuffer that memory-maps
+ a model file, or a direct ByteBuffer of nativeOrder() that contains the bytes content
+ of a model.
Explicitly updates allocations for all tensors, if necessary.
+
+
This will propagate shapes and memory allocations for dependent tensors using the input
+ tensor shape(s) as given.
+
+
Note: This call is *purely optional*. Tensor allocation will occur automatically during
+ execution if any input tensors have been resized. This call is most useful in determining the
+ shapes for any output tensors before executing the graph, e.g.,
+
+
Gets the Tensor associated with the provided output index.
+
+
Note: Output tensor details (e.g., shape) may not be fully populated until after inference
+ is executed. If you need updated details *before* running inference (e.g., after resizing an
+ input tensor, which may invalidate output tensor shapes), use allocateTensors() to
+ explicitly trigger allocation and shape propagation. Note that, for graphs with output shapes
+ that are dependent on input *values*, the output shape may not be fully determined until
+ running inference.
+
+
Parameters
+
+
+
outputIndex
+
+
+
+
+
+
+
+
+
+
+ public
+
+
+
+
+ int
+
+getOutputTensorCount
+()
+
Gets the Tensor associated with the provided output name in specific signature method.
+
+
Note: Output tensor details (e.g., shape) may not be fully populated until after inference
+ is executed. If you need updated details *before* running inference (e.g., after resizing an
+ input tensor, which may invalidate output tensor shapes), use allocateTensors() to
+ explicitly trigger allocation and shape propagation. Note that, for graphs with output shapes
+ that are dependent on input *values*, the output shape may not be fully determined until
+ running inference.
+
+
WARNING: This is an experimental API and subject to change.
+
+
Parameters
+
+
+
outputName
+
Output name in the signature.
+
+
+
signatureKey
+
Signature key identifying the SignatureDef, can be null if the model has
+ one signature.
Resizes idx-th input of the native model to the given dims.
+
+
When `strict` is True, only unknown dimensions can be resized. Unknown dimensions are
+ indicated as `-1` in the array returned by `Tensor.shapeSignature()`.
Runs model inference if the model takes only one input, and provides only one output.
+
+
Warning: The API is more efficient if a Buffer (preferably direct, but not required)
+ is used as the input/output data type. Please consider using Buffer to feed and fetch
+ primitive data for better performance. The following concrete Buffer types are
+ supported:
+
+
+
ByteBuffer - compatible with any underlying primitive Tensor type.
+
FloatBuffer - compatible with float Tensors.
+
IntBuffer - compatible with int32 Tensors.
+
LongBuffer - compatible with int64 Tensors.
+
+
+ Note that boolean types are only supported as arrays, not Buffers, or as scalar inputs.
+
+
Parameters
+
+
+
input
+
an array or multidimensional array, or a Buffer of primitive types
+ including int, float, long, and byte. Buffer is the preferred way to pass large
+ input data for primitive types, whereas string types require using the (multi-dimensional)
+ array input path. When a Buffer is used, its content should remain unchanged until
+ model inference is done, and the caller must ensure that the Buffer is at the
+ appropriate read position. A null value is allowed only if the caller is using a
+ Delegate that allows buffer handle interop, and such a buffer has been bound to the
+ input Tensor.
+
+
+
output
+
a multidimensional array of output data, or a Buffer of primitive types
+ including int, float, long, and byte. When a Buffer is used, the caller must ensure
+ that it is set the appropriate write position. A null value is allowed, and is useful for
+ certain cases, e.g., if the caller is using a Delegate that allows buffer handle
+ interop, and such a buffer has been bound to the output Tensor (see also Interpreter.Options#setAllowBufferHandleOutput(boolean)),
+ or if the graph has dynamically shaped outputs and the caller must query the output Tensor shape after inference has been invoked, fetching the data directly from the output
+ tensor (via Tensor.asReadOnlyBuffer()).
Runs model inference if the model takes multiple inputs, or returns multiple outputs.
+
+
Warning: The API is more efficient if Buffers (preferably direct, but not required)
+ are used as the input/output data types. Please consider using Buffer to feed and fetch
+ primitive data for better performance. The following concrete Buffer types are
+ supported:
+
+
+
ByteBuffer - compatible with any underlying primitive Tensor type.
+
FloatBuffer - compatible with float Tensors.
+
IntBuffer - compatible with int32 Tensors.
+
LongBuffer - compatible with int64 Tensors.
+
+
+ Note that boolean types are only supported as arrays, not Buffers, or as scalar inputs.
+
+
Note: null values for invididual elements of inputs and outputs is
+ allowed only if the caller is using a Delegate that allows buffer handle interop, and
+ such a buffer has been bound to the corresponding input or output Tensor(s).
+
+
Parameters
+
+
+
inputs
+
an array of input data. The inputs should be in the same order as inputs of the
+ model. Each input can be an array or multidimensional array, or a Buffer of
+ primitive types including int, float, long, and byte. Buffer is the preferred way
+ to pass large input data, whereas string types require using the (multi-dimensional) array
+ input path. When Buffer is used, its content should remain unchanged until model
+ inference is done, and the caller must ensure that the Buffer is at the appropriate
+ read position.
+
+
+
outputs
+
a map mapping output indices to multidimensional arrays of output data or Buffers of primitive types including int, float, long, and byte. It only needs to keep
+ entries for the outputs to be used. When a Buffer is used, the caller must ensure
+ that it is set the appropriate write position. The map may be empty for cases where either
+ buffer handles are used for output tensor data, or cases where the outputs are dynamically
+ shaped and the caller must query the output Tensor shape after inference has been
+ invoked, fetching the data directly from the output tensor (via Tensor.asReadOnlyBuffer()).
Same as runSignature(Map, Map, String) but doesn't require passing a signatureKey,
+ assuming the model has one SignatureDef. If the model has more than one SignatureDef it will
+ throw an exception.
+
+
WARNING: This is an experimental API and subject to change.
+
Runs model inference based on SignatureDef provided through signatureKey.
+
+
See run(Object, Object) for more details on the allowed input and output
+ data types.
+
+
WARNING: This is an experimental API and subject to change.
+
+
Parameters
+
+
+
inputs
+
A map from input name in the SignatureDef to an input object.
+
+
+
outputs
+
A map from output name in SignatureDef to output data. This may be empty if the
+ caller wishes to query the Tensor data directly after inference (e.g., if the
+ output shape is dynamic, or output buffer handles are used).
Advanced: Interrupts inference in the middle of a call to run(Object, Object).
+
+
A cancellation flag will be set to true when this function gets called. The interpreter will
+ check the flag between Op invocations, and if it's true, the interpreter will stop
+ execution. The interpreter will remain a cancelled state until explicitly "uncancelled" by
+ setCancelled(false).
+
+
WARNING: This is an experimental API and subject to change.
+
+
Parameters
+
+
+
cancelled
+
true to cancel inference in a best-effort way; false to
+ resume.
+ public static final enum
+ InterpreterApi.Options.TfLiteRuntime
+
+
+
+
+
Enum to represent where to get the TensorFlow Lite runtime implementation from.
+
+
The difference between this class and the RuntimeFlavor class: This class specifies a
+ preference which runtime to use, whereas RuntimeFlavor specifies which exact
+ runtime is being used.
+
+
+ public
+ static
+ final
+
+
+ InterpreterApi.Options.TfLiteRuntime
+
+
+FROM_APPLICATION_ONLY
+
+
+
+
+
Use a TF Lite runtime implementation that is linked into the application. If there is no
+ suitable TF Lite runtime implementation linked into the application, then attempting to
+ create an InterpreterApi instance with this TfLiteRuntime setting will throw an
+ IllegalStateException exception (even if the OS or system services could provide a TF Lite
+ runtime implementation).
+
+
This is the default setting. This setting is also appropriate for apps that must run on
+ systems that don't provide a TF Lite runtime implementation.
+
+
+
+
+
+
+
+ public
+ static
+ final
+
+
+ InterpreterApi.Options.TfLiteRuntime
+
+
+FROM_SYSTEM_ONLY
+
+
+
+
+
Use a TF Lite runtime implementation provided by the OS or system services. This will be
+ obtained from a system library / shared object / service, such as Google Play Services. It
+ may be newer than the version linked into the application (if any). If there is no suitable
+ TF Lite runtime implementation provided by the system, then attempting to create an
+ InterpreterApi instance with this TfLiteRuntime setting will throw an IllegalStateException
+ exception (even if there is a TF Lite runtime implementation linked into the application).
+
+
This setting is appropriate for code that will use a system-provided TF Lite runtime,
+ which can reduce app binary size and can be updated more frequently.
+
+
+
+
+
+
+
+ public
+ static
+ final
+
+
+ InterpreterApi.Options.TfLiteRuntime
+
+
+PREFER_SYSTEM_OVER_APPLICATION
+
+
+
+
+
Use a system-provided TF Lite runtime implementation, if any, otherwise use the TF Lite
+ runtime implementation linked into the application, if any. If no suitable TF Lite runtime
+ can be found in any location, then attempting to create an InterpreterApi instance with
+ this TFLiteRuntime setting will throw an IllegalStateException. If there is both a suitable
+ TF Lite runtime linked into the application and also a suitable TF Lite runtime provided by
+ the system, the one provided by the system will be used.
+
+
This setting is suitable for use in code that doesn't care where the TF Lite runtime is
+ coming from (e.g. middleware layers).
+
Returns the list of delegates intended to be applied during interpreter creation that have
+ been registered via addDelegate.
+
+
+
+
+
+
+
+ public
+
+
+
+
+ int
+
+getNumThreads
+()
+
+
+
+
+
+
Returns the number of threads to be used for ops that support multi-threading.
+
+
numThreads should be >= -1. Values of 0 (or 1) disable multithreading.
+ Default value is -1: the number of threads used will be implementation-defined and
+ platform-dependent.
+
Advanced: Returns whether the interpreter is able to be cancelled.
+
+
Interpreters may have an experimental API setCancelled(boolean).
+ If this interpreter is cancellable and such a method is invoked, a cancellation flag will be
+ set to true. The interpreter will check the flag between Op invocations, and if it's true, the interpreter will stop execution. The interpreter will remain a cancelled state
+ until explicitly "uncancelled" by setCancelled(false).
+
Advanced: Set if the interpreter is able to be cancelled.
+
+
Interpreters may have an experimental API setCancelled(boolean).
+ If this interpreter is cancellable and such a method is invoked, a cancellation flag will be
+ set to true. The interpreter will check the flag between Op invocations, and if it's true, the interpreter will stop execution. The interpreter will remain a cancelled state
+ until explicitly "uncancelled" by setCancelled(false).
+
Sets the number of threads to be used for ops that support multi-threading.
+
+
numThreads should be >= -1. Setting numThreads to 0 has the effect
+ of disabling multithreading, which is equivalent to setting numThreads to 1. If
+ unspecified, or set to the value -1, the number of threads used will be
+ implementation-defined and platform-dependent.
+
String[] input = {"foo", "bar"}; // Input tensor shape is [2].
+ String[][] output = new String[3][2]; // Output tensor shape is [3, 2].
+ try (InterpreterApi interpreter =
+ new InterpreterApi.create(file_of_a_tensorflowlite_model)) {
+ interpreter.runForMultipleInputsOutputs(input, output);
+ }
+
+
Note that there's a distinction between shape [] and shape[1]. For scalar string tensor
+ outputs:
+
+
String[] input = {"foo"}; // Input tensor shape is [1].
+ ByteBuffer outputBuffer = ByteBuffer.allocate(OUTPUT_BYTES_SIZE); // Output tensor shape is [].
+ try (Interpreter interpreter = new Interpreter(file_of_a_tensorflowlite_model)) {
+ interpreter.runForMultipleInputsOutputs(input, outputBuffer);
+ }
+ byte[] outputBytes = new byte[outputBuffer.remaining()];
+ outputBuffer.get(outputBytes);
+ // Below, the `charset` can be StandardCharsets.UTF_8.
+ String output = new String(outputBytes, charset);
+
+
Orders of inputs and outputs are determined when converting TensorFlow model to TensorFlowLite
+ model with Toco, as are the default shapes of the inputs.
+
+
When inputs are provided as (multi-dimensional) arrays, the corresponding input tensor(s) will
+ be implicitly resized according to that array's shape. When inputs are provided as Buffer types, no implicit resizing is done; the caller must ensure that the Buffer byte size either matches that of the corresponding tensor, or that they first
+ resize the tensor via resizeInput(int, int[]). Tensor shape and type information can be
+ obtained via the Tensor class, available via getInputTensor(int) and getOutputTensor(int).
+
+
WARNING:InterpreterApi instances are not thread-safe.
+
+
WARNING:An InterpreterApi instance owns resources that must be
+ explicitly freed by invoking close()
+
The TFLite library is built against NDK API 19. It may work for Android API levels below 19,
+ but is not guaranteed.
+
Explicitly updates allocations for all tensors, if necessary.
+
+
This will propagate shapes and memory allocations for dependent tensors using the input
+ tensor shape(s) as given.
+
+
Note: This call is *purely optional*. Tensor allocation will occur automatically during
+ execution if any input tensors have been resized. This call is most useful in determining the
+ shapes for any output tensors before executing the graph, e.g.,
+
+
Constructs an InterpreterApi instance, using the specified model and options. The model
+ will be read from a ByteBuffer.
+
+
Parameters
+
+
+
byteBuffer
+
A pre-trained TF Lite model, in binary serialized form. The ByteBuffer should
+ not be modified after the construction of an InterpreterApi instance. The ByteBuffer can be either a MappedByteBuffer that memory-maps a model file, or a
+ direct ByteBuffer of nativeOrder() that contains the bytes content of a model.
+
+
+
options
+
A set of options for customizing interpreter behavior.
Gets the Tensor associated with the provided output index.
+
+
Note: Output tensor details (e.g., shape) may not be fully populated until after inference
+ is executed. If you need updated details *before* running inference (e.g., after resizing an
+ input tensor, which may invalidate output tensor shapes), use allocateTensors() to
+ explicitly trigger allocation and shape propagation. Note that, for graphs with output shapes
+ that are dependent on input *values*, the output shape may not be fully determined until
+ running inference.
Resizes idx-th input of the native model to the given dims.
+
+
When `strict` is True, only unknown dimensions can be resized. Unknown dimensions are
+ indicated as `-1` in the array returned by `Tensor.shapeSignature()`.
if idx is negative or is not smaller than the number
+ of model inputs; or if error occurs when resizing the idx-th input. Additionally, the error
+ occurs when attempting to resize a tensor with fixed dimensions when `strict` is True.
+
Runs model inference if the model takes only one input, and provides only one output.
+
+
Warning: The API is more efficient if a Buffer (preferably direct, but not required)
+ is used as the input/output data type. Please consider using Buffer to feed and fetch
+ primitive data for better performance. The following concrete Buffer types are
+ supported:
+
+
+
ByteBuffer - compatible with any underlying primitive Tensor type.
+
FloatBuffer - compatible with float Tensors.
+
IntBuffer - compatible with int32 Tensors.
+
LongBuffer - compatible with int64 Tensors.
+
+
+ Note that boolean types are only supported as arrays, not Buffers, or as scalar inputs.
+
+
Parameters
+
+
+
input
+
an array or multidimensional array, or a Buffer of primitive types
+ including int, float, long, and byte. Buffer is the preferred way to pass large
+ input data for primitive types, whereas string types require using the (multi-dimensional)
+ array input path. When a Buffer is used, its content should remain unchanged until
+ model inference is done, and the caller must ensure that the Buffer is at the
+ appropriate read position. A null value is allowed only if the caller is using a
+ Delegate that allows buffer handle interop, and such a buffer has been bound to the
+ input Tensor.
+
+
+
output
+
a multidimensional array of output data, or a Buffer of primitive types
+ including int, float, long, and byte. When a Buffer is used, the caller must ensure
+ that it is set the appropriate write position. A null value is allowed, and is useful for
+ certain cases, e.g., if the caller is using a Delegate that allows buffer handle
+ interop, and such a buffer has been bound to the output Tensor (see also Interpreter.Options#setAllowBufferHandleOutput(boolean)),
+ or if the graph has dynamically shaped outputs and the caller must query the output Tensor shape after inference has been invoked, fetching the data directly from the output
+ tensor (via Tensor.asReadOnlyBuffer()).
Runs model inference if the model takes multiple inputs, or returns multiple outputs.
+
+
Warning: The API is more efficient if Buffers (preferably direct, but not required)
+ are used as the input/output data types. Please consider using Buffer to feed and fetch
+ primitive data for better performance. The following concrete Buffer types are
+ supported:
+
+
+
ByteBuffer - compatible with any underlying primitive Tensor type.
+
FloatBuffer - compatible with float Tensors.
+
IntBuffer - compatible with int32 Tensors.
+
LongBuffer - compatible with int64 Tensors.
+
+
+ Note that boolean types are only supported as arrays, not Buffers, or as scalar inputs.
+
+
Note: null values for invididual elements of inputs and outputs is
+ allowed only if the caller is using a Delegate that allows buffer handle interop, and
+ such a buffer has been bound to the corresponding input or output Tensor(s).
+
+
Parameters
+
+
+
inputs
+
an array of input data. The inputs should be in the same order as inputs of the
+ model. Each input can be an array or multidimensional array, or a Buffer of
+ primitive types including int, float, long, and byte. Buffer is the preferred way
+ to pass large input data, whereas string types require using the (multi-dimensional) array
+ input path. When Buffer is used, its content should remain unchanged until model
+ inference is done, and the caller must ensure that the Buffer is at the appropriate
+ read position.
+
+
+
outputs
+
a map mapping output indices to multidimensional arrays of output data or Buffers of primitive types including int, float, long, and byte. It only needs to keep
+ entries for the outputs to be used. When a Buffer is used, the caller must ensure
+ that it is set the appropriate write position. The map may be empty for cases where either
+ buffer handles are used for output tensor data, or cases where the outputs are dynamically
+ shaped and the caller must query the output Tensor shape after inference has been
+ invoked, fetching the data directly from the output tensor (via Tensor.asReadOnlyBuffer()).
Constructs an InterpreterApi instance, using the specified model and options. The model
+ will be read from a ByteBuffer.
+
+
Parameters
+
+
+
byteBuffer
+
A pre-trained TF Lite model, in binary serialized form. The ByteBuffer should
+ not be modified after the construction of an InterpreterApi instance. The ByteBuffer can be either a MappedByteBuffer that memory-maps a model file, or a
+ direct ByteBuffer of nativeOrder() that contains the bytes content of a model.
+
+
+
options
+
A set of options for customizing interpreter behavior.
Represents a TFLite runtime. In contrast to InterpreterApi.Options.TfLiteRuntime, this enum represents the
+ actual runtime that is being used, whereas the latter represents a preference for which runtime
+ should be used.
+
A typed multi-dimensional array used in Tensorflow Lite.
+
+
The native handle of a Tensor is managed by NativeInterpreterWrapper, and does
+ not needed to be closed by the client. However, once the NativeInterpreterWrapper has
+ been closed, the tensor handle will be invalidated.
+
Returns a read-only ByteBuffer view of the tensor data.
+
+
In general, this method is most useful for obtaining a read-only view of output tensor data,
+ *after* inference has been executed (e.g., via InterpreterApi.run(Object, Object)). In
+ particular, some graphs have dynamically shaped outputs, which can make feeding a predefined
+ output buffer to the interpreter awkward. Example usage:
+
+
interpreter.run(input, null);
+ ByteBuffer outputBuffer = interpreter.getOutputTensor(0).asReadOnlyBuffer();
+ // Copy or read from outputBuffer.
+
WARNING: If the tensor has not yet been allocated, e.g., before inference has been executed,
+ the result is undefined. Note that the underlying tensor pointer may also change when the
+ tensor is invalidated in any way (e.g., if inference is executed, or the graph is resized), so
+ it is *not* safe to hold a reference to the returned buffer beyond immediate use directly
+ following inference. Example *bad* usage:
+
+
ByteBuffer outputBuffer = interpreter.getOutputTensor(0).asReadOnlyBuffer();
+ interpreter.run(input, null);
+ // Copy or read from outputBuffer (which may now be invalid).
Returns the original shape of the Tensor,
+ i.e., the sizes of each dimension - before any resizing was performed. Unknown dimensions are
+ designated with a value of -1.
+
+
Returns
+
an array where the i-th element is the size of the i-th dimension of the tensor.
+
+ public interface
+ ValidatedAccelerationConfig
+
+
+
+
+
Interface specifying validated acceleration configuration. Developers should not implement this
+ interface directly as it is only supported through the Acceleration service SDK.
+
The GPU delegate is not supported on all Android devices, due to differences in available
+ OpenGL versions, driver features, and device resources. This class provides information on
+ whether the GPU delegate is suitable for the current device.
+
+
This API is experimental and subject to change.
+
+
WARNING: the compatibilityList is constructed from testing done on a limited set of
+ models. You should plan to verify that your own model(s) work.
+
+
Example usage:
+
+
Interpreter.Options options = new Interpreter.Options();
+ try (CompatibilityList compatibilityList = new CompatibilityList()) {
+ if (compatibilityList.isDelegateSupportedOnThisDevice()) {
+ GpuDelegate.Options delegateOptions = compatibilityList.getBestOptionsForThisDevice();
+ gpuDelegate = new GpuDelegate(delegateOptions):
+ options.addDelegate(gpuDelegate);
+ }
+ }
+ Interpreter interpreter = new Interpreter(modelBuffer, options);
+
Note: When calling Interpreter.Options.addDelegate() and Interpreter.run(),
+ the caller must have an EGLContext in the current thread and Interpreter.run() must be called from the same EGLContext. If an EGLContext does
+ not exist, the delegate will internally create one, but then the developer must ensure that
+ Interpreter.run() is always called from the same thread in which Interpreter.Options.addDelegate() was called.
+
User is expected to call this method explicitly.
+
+
+
+
+
+
+
+ public
+
+
+
+
+ long
+
+getNativeHandle
+()
+
+
+
+
+
+
Returns a native handle to the TensorFlow Lite delegate implementation.
+
+
Note: The Java Delegate maintains ownership of the native delegate instance, and
+ must ensure its existence for the duration of usage with any InterpreterApi instance.
+
+
Note: the native delegate instance may not be created until the delegate has been attached
+ to an interpreter, so this method should not be called until after an interpreter has been
+ constructed with this delegate.
+
+
Returns
+
The native delegate handle. In C/C++, this should be a pointer to
+ 'TfLiteOpaqueDelegate'.
+
When `true` (default), the GPU may quantify tensors, downcast
+ values, process in FP16. When `false`, computations are carried out in 32-bit floating
+ point.
+
Enables serialization on the delegate. Note non-null serializationDir and modelToken are required for serialization.
+
+
WARNING: This is an experimental API and subject to change.
+
+
Parameters
+
+
+
serializationDir
+
The directory to use for storing data. Caller is responsible to
+ ensure the model is not stored in a public directory. It's recommended to use Context.getCodeCacheDir() to provide a private location for the
+ application on Android.
+
+
+
modelToken
+
The token to be used to identify the model. Caller is responsible to ensure
+ the token is unique to the model graph and data.
+
Note for developers implementing this interface: Currently TF Lite in Google Play Services
+ does not support external (developer-provided) delegates. Correspondingly, implementations of
+ this method can expect to be called with RuntimeFlavor.APPLICATION.
+
+ public static abstract class
+ TensorAudio.TensorAudioFormat
+
+
+
+
+
Wraps a few constants describing the format of the incoming audio samples, namely number of
+ channels and the sample rate. By default, channels is set to 1.
+
Defines a ring buffer and some utility functions to prepare the input audio samples.
+
+
It maintains a Ring Buffer to hold
+ input audio data. Clients could feed input audio data via `load` methods and access the
+ aggregated audio samples via `getTensorBuffer` method.
+
+
number of captured audio values whose size is channelCount * sampleCount. If
+ there was no new data in the AudioRecord or an error occurred, this method will return 0.
Loads labels from the label file into a list of strings.
+
+
A legal label file is the plain text file whose contents are split into lines, and each line
+ is an individual value. The file should be in assets of the context.
+
+
Parameters
+
+
+
context
+
The context holds assets.
+
+
+
filePath
+
The path of the label file, relative with assets directory.
Loads labels from the label file into a list of strings.
+
+
A legal label file is the plain text file whose contents are split into lines, and each line
+ is an individual value. The empty lines will be ignored. The file should be in assets of the
+ context.
+
+
Parameters
+
+
+
context
+
The context holds assets.
+
+
+
filePath
+
The path of the label file, relative with assets directory.
+
+
+
cs
+
Charset to use when decoding content of label file.
Loads a vocabulary file (a single-column text file) into a list of strings.
+
+
A vocabulary file is a single-column plain text file whose contents are split into lines,
+ and each line is an individual value. The file should be in assets of the context.
+
+
Parameters
+
+
+
context
+
The context holds assets.
+
+
+
filePath
+
The path of the vocabulary file, relative with assets directory.
Loads vocabulary from an input stream of an opened vocabulary file (which is a single-column
+ text file).
+
+
A vocabulary file is a single-column plain text file whose contents are split into lines,
+ and each line is an individual value. The file should be in assets of the context.
TensorProcessor is a helper class for preprocessing and postprocessing tensors. It could
+ transform a TensorBuffer to another by executing a chain of TensorOperator.
+
+
Dequantizes a TensorBuffer with given zeroPoint and scale.
+
+
Note: The data type of output tensor is always FLOAT32 except when the DequantizeOp is
+ created effectively as an identity Op such as setting zeroPoint to 0 and scale to
+ 1 (in this case, the output tensor is the same instance as input).
+
+
If both zeroPoint and scale are 0, the DequantizeOp will be bypassed,
+ which is equivalent to setting zeroPoint to 0 and scale to 1. This can be useful
+ when passing in the quantization parameters that are extracted directly from the TFLite model
+ flatbuffer. If the tensor is not quantized, both zeroPoint and scale will be read
+ as 0.
+
Initializes a NormalizeOp. When being called, it creates a new TensorBuffer, which
+ satisfies:
+
+
+ output = (input - mean) / stddev
+
+
In the following two cases, reset mean to 0 and stddev to 1 to bypass the
+ normalization.
+ 1. Both mean and {code stddev} are 0.
+ 2. mean is 0 and {stddev} is Infinity.
+
+
Note: If mean is set to 0 and stddev is set to 1, no computation will
+ happen, and original input will be directly returned in execution.
+
+
Note: The returned TensorBuffer is always a DataType.FLOAT32 tensor at
+ present, except when the input is a DataType.UINT8 tensor, mean is set to 0 and
+ stddev is set to 1, so that the original DataType.UINT8 tensor is returned.
Initializes a NormalizeOp. When being called, it creates a new TensorBuffer, which
+ satisfies:
+
+
+ // Pseudo code. [...][i] means a certain element whose channel id is i.
+ output[...][i] = (input[...][i] - mean[i]) / stddev[i]
+
+
Note: If all values in mean are set to 0 and all stddev are set to 1, no
+ computation will happen, and original input will be directly returned in execution.
+
+
Note: The returned TensorBuffer is always a DataType.FLOAT32 tensor at
+ present, except that the input is a DataType.UINT8 tensor, all mean are set to
+ 0 and all stddev are set to 1.
+
+
Parameters
+
+
+
mean
+
the mean values to be subtracted first for each channel.
+
+
+
stddev
+
the standard deviation values to divide then for each channel.
Quantizes a TensorBuffer with given zeroPoint and scale.
+
+
Note: QuantizeOp does not cast output to UINT8, but only performs the quantization
+ math on top of input. The data type of output tensor is always FLOAT32 except that the Op
+ is effectively an identity Op (in this case, the output tensor is the same instance as the
+ input). To connect with quantized model, a CastOp is probably needed.
+
+
If both zeroPoint and scale are 0, the QuantizeOp will be bypassed,
+ which is equivalent to setting zeroPoint to 0 and scale to 1. This can be useful
+ when passing in the quantization parameters that are extracted directly from the TFLite model
+ flatbuffer. If the tensor is not quantized, both zeroPoint and scale will be read
+ as 0.
+
+
+ public
+ static
+ final
+
+
+ BoundingBoxUtil.Type
+
+
+BOUNDARIES
+
+
+
+
+
Represents the bounding box by using the combination of boundaries, {left, top, right,
+ bottom}. The default order is {left, top, right, bottom}. Other orders can be indicated by an
+ index array.
+
+
+
+
+
+
+
+ public
+ static
+ final
+
+
+ BoundingBoxUtil.Type
+
+
+CENTER
+
+
+
+
+
Represents the bounding box by using the center of the box, width and height. The default
+ order is {center_x, center_y, width, height}. Other orders can be indicated by an index
+ array.
+
+
+
+
+
+
+
+ public
+ static
+ final
+
+
+ BoundingBoxUtil.Type
+
+
+UPPER_LEFT
+
+
+
+
+
Represents the bounding box by using the upper_left corner, width and height. The default
+ order is {upper_left_x, upper_left_y, width, height}. Other orders can be indicated by an
+ index array.
+
Helper class for converting values that represents bounding boxes into rectangles.
+
+
The class provides a static function to create bounding boxes as RectF from different types of configurations.
+
+
Generally, a bounding box could be represented by 4 float values, but the values could be
+ interpreted in many ways. We now support 3 BoundingBoxUtil.Type of configurations, and the order of
+ elements in each type is configurable as well.
+
Creates a list of bounding boxes from a TensorBuffer which represents bounding boxes.
+
+
Parameters
+
+
+
tensor
+
holds the data representing some boxes.
+
+
+
valueIndex
+
denotes the order of the elements defined in each bounding box type. An empty
+ index array represent the default order of each bounding box type. For example, to denote
+ the default order of BOUNDARIES, {left, top, right, bottom}, the index should be {0, 1, 2,
+ 3}. To denote the order {left, right, top, bottom}, the order should be {0, 2, 1, 3}.
+
The index array can be applied to all bounding box types to adjust the order of their
+ corresponding underlying elements.
+
+
+
boundingBoxAxis
+
specifies the index of the dimension that represents bounding box. The
+ size of that dimension is required to be 4. Index here starts from 0. For example, if the
+ tensor has shape 4x10, the axis for bounding boxes is likely to be 0. Negative axis is also
+ supported: -1 gives the last axis and -2 gives the second, .etc. theFor shape 10x4, the
+ axis is likely to be 1 (or -1, equivalently).
A list of bounding boxes that the tensor represents. All dimensions except
+ boundingBoxAxis will be collapsed with order kept. For example, given tensor with shape {1, 4, 10, 2} and boundingBoxAxis = 1, The result will be a list
+ of 20 bounding boxes.
+
+ public
+ static
+ final
+
+
+ ColorSpaceType
+
+
+GRAYSCALE
+
+
+
+
+
Each pixel is a single element representing only the amount of light.
+
+
+
+
+
+
+ public
+ static
+ final
+
+
+ ColorSpaceType
+
+
+NV12
+
+
+
+
+
YUV420sp format, encoded as "YYYYYYYY UVUV".
+
+
+
+
+
+
+ public
+ static
+ final
+
+
+ ColorSpaceType
+
+
+NV21
+
+
+
+
+
YUV420sp format, encoded as "YYYYYYYY VUVU", the standard picture format on Android Camera1
+ preview.
+
+
+
+
+
+
+
+ public
+ static
+ final
+
+
+ ColorSpaceType
+
+
+RGB
+
+
+
+
+
Each pixel has red, green, and blue color components.
+
+
+
+
+
+
+ public
+ static
+ final
+
+
+ ColorSpaceType
+
+
+YUV_420_888
+
+
+
+
+
YUV420 format corresponding to ImageFormat.YUV_420_888. The actual
+ encoding format (i.e. NV12 / Nv21 / YV12 / YV21) depends on the implementation of the image.
+
+
ImageProcessor is a helper class for preprocessing and postprocessing TensorImage. It
+ could transform a TensorImage to another by executing a chain of ImageOperator.
+
+
WARNING: Instances of an ImageProcessor are not thread-safe with updateNumberOfRotations(int). Updating the number of rotations and then processing images (using
+ SequentialProcessor.process(T)) must be protected from concurrent access. It is recommended to create separate
+ ImageProcessor instances for each thread. If multiple threads access a ImageProcessor concurrently, it must be synchronized externally.
WARNING:this method is not thread-safe. Updating the number of rotations and
+ then processing images (using SequentialProcessor.process(T)) must be protected from concurrent access with
+ additional synchronization.
+
+ public
+
+
+
+ synchronized
+ void
+
+updateNumberOfRotations
+(int k, int occurrence)
+
+
+
+
+
+
Updates the number of rotations for the Rot90Op specified by occurrence in this
+ ImageProcessor.
+
+
WARNING:this method is not thread-safe. Updating the number of rotations and
+ then processing images (using SequentialProcessor.process(T)) must be protected from concurrent access with
+ additional synchronization.
+
+
Parameters
+
+
+
k
+
the number of rotations
+
+
+
occurrence
+
the index of perticular Rot90Op in this ImageProcessor. For
+ example, if the second Rot90Op needs to be updated, occurrence should be
+ set to 1.
IMPORTANT: The returned TensorImage shares storage with mlImage, so do not
+ modify the contained object in the TensorImage, as MlImage expects its
+ contained data are immutable. Also, callers should use MlImage#getInternal()#acquire()
+ and MlImage#release() to avoid the mlImage being released unexpectedly.
TensorImage is the wrapper class for Image object. When using image processing utils in
+ TFLite.support library, it's common to convert image objects in variant types to TensorImage at
+ first.
+
+
At present, only RGB images are supported, and the A channel is always ignored.
+
+
Details of data storage: a TensorImage object may have 2 potential sources of truth: a
+ Bitmap or a TensorBuffer. TensorImage maintains the
+ state and only converts one to the other when needed. A typical use case of TensorImage
+ is to first load a Bitmap image, then process it using ImageProcessor, and finally get the underlying ByteBuffer of the TensorBuffer
+ and feed it into the TFLite interpreter.
+
+
IMPORTANT: to achieve the best performance, TensorImage avoids copying data whenever
+ it's possible. Therefore, it doesn't own its data. Callers should not modify data objects those
+ are passed to load(Bitmap) or load(TensorBuffer, ColorSpaceType).
+
+
IMPORTANT: all methods are not proved thread-safe.
Note: the shape of a TensorImage is not fixed. It can be adjusted to the shape of
+ the image being loaded to this TensorImage.
+
+
Parameters
+
+
+
dataType
+
the expected data type of the resulting TensorBuffer. The type is
+ always fixed during the lifetime of the TensorImage. To convert the data type, use
+ createFrom(TensorImage, DataType) to create a copy and convert data type at the
+ same time.
Numeric casting and clamping will be applied if the stored data is not uint8.
+
+
Note that, the reliable way to get pixels from an ALPHA_8 Bitmap is to use copyPixelsToBuffer. Bitmap methods such as, `setPixels()` and `getPixels` do not work.
+
+
Important: it's only a reference. DO NOT MODIFY. We don't create a copy here for performance
+ concern, but if modification is necessary, please make a copy.
+
+
Returns
+
a reference to a Bitmap in ARGB_8888 config ("A"
+ channel is always opaque) or in ALPHA_8, depending on the ColorSpaceType of
+ this TensorBuffer.
Returns a ByteBuffer representation of this TensorImage with the expected data
+ type.
+
+
Numeric casting and clamping will be applied if the stored data is different from the data
+ type of the TensorImage.
+
+
Important: it's only a reference. DO NOT MODIFY. We don't create a copy here for performance
+ concern, but if modification is necessary, please make a copy.
+
+
It's essentially a short cut for getTensorBuffer().getBuffer().
+
+
Returns
+
a reference to a ByteBuffer which holds the image data
This method only works when the TensorImage is backed by an Image, meaning you need to first load an Image through
+ load(Image).
+
+
Important: it's only a reference. DO NOT MODIFY. We don't create a copy here for performance
+ concern, but if modification is necessary, please make a copy.
+
+
Returns
+
a reference to a Bitmap in ARGB_8888 config ("A"
+ channel is always opaque) or in ALPHA_8, depending on the ColorSpaceType of
+ this TensorBuffer.
Returns a TensorBuffer representation of this TensorImage with the expected
+ data type.
+
+
Numeric casting and clamping will be applied if the stored data is different from the data
+ type of the TensorImage.
+
+
Important: it's only a reference. DO NOT MODIFY. We don't create a copy here for performance
+ concern, but if modification is necessary, please make a copy.
+
+
Returns
+
a reference to a TensorBuffer which holds the image data
Note: if the data type of buffer does not match that of this TensorImage,
+ numeric casting and clamping will be applied when calling getTensorBuffer() and getBuffer().
+
+
Parameters
+
+
+
buffer
+
the TensorBuffer to be loaded. Its shape should be either (h, w, 3) or
+ (1, h, w, 3) for RGB images, and either (h, w) or (1, h, w) for GRAYSCALE images
Important: when loading a bitmap, DO NOT MODIFY the bitmap from the caller side anymore. The
+ TensorImage object will rely on the bitmap. It will probably modify the bitmap as well.
+ In this method, we perform a zero-copy approach for that bitmap, by simply holding its
+ reference. Use bitmap.copy(bitmap.getConfig(), true) to create a copy if necessary.
+
+
Note: to get the best performance, please load images in the same shape to avoid memory
+ re-allocation.
Loads an int array as RGB pixels into this TensorImage, representing the pixels inside.
+
+
Note: numeric casting and clamping will be applied to convert the values into the data type
+ of this TensorImage when calling getTensorBuffer() and getBuffer().
+
+
Parameters
+
+
+
pixels
+
the RGB pixels representing the image
+
+
+
shape
+
the shape of the image, should either in form (h, w, 3), or in form (1, h, w, 3)
Note: if the data type of buffer does not match that of this TensorImage,
+ numeric casting and clamping will be applied when calling getTensorBuffer() and getBuffer().
The shape of the TensorBuffer will not be used to determine image height and width.
+ Set image properties through ImageProperties.
+
+
Note: if the data type of buffer does not match that of this TensorImage,
+ numeric casting and clamping will be applied when calling getTensorBuffer() and getBuffer().
As a computation unit for processing images, it could resize image to predefined size.
+
+
It will not stretch or compress the content of image. However, to fit the new size, it crops
+ or pads pixels. When it crops image, it performs a center-crop; when it pads pixels, it performs
+ a zero-padding.
Applies an operation on a T object, returning a T object.
+
+
+
+
+
+
+
+
+
+
+
+
+
+
+
+
+
+
+
+
Public Constructors
+
+
+
+
+ public
+
+
+
+
+
+
+Rot90Op
+()
+
+
+
+
+
+
Creates a Rot90 Op which will rotate image by 90 degree counter-clockwise.
+
+
+
+
+
+
+ public
+
+
+
+
+
+
+Rot90Op
+(int k)
+
+
+
+
+
+
Creates a Rot90 Op which will rotate image by 90 degree for k times counter-clockwise.
+
+
Parameters
+
+
+
k
+
The number of times the image is rotated by 90 degrees. If it's positive, the image
+ will be rotated counter-clockwise. If it's negative, the op will rotate image clockwise.
+
The conversion is based on OpenCV RGB to GRAY conversion
+ https://docs.opencv.org/master/de/d25/imgproc_color_conversions.html#color_convert_rgb_gray
+
Category is a util class, contains a label, its display name, a float value as score, and the
+ index of the label in the corresponding label file. Typically it's used as result of
+ classification tasks.
+
the display name of the label, which may be translated for different
+ locales. For exmaple, a label, "apple", may be translated into Spanish for display purpose,
+ so that the displayName is "manzana".
+
+
+
score
+
the probability score of this label category
+
+
+
index
+
the index of the label in the corresponding label file
+
Maps an int value tensor to a list of string labels. It takes an array of strings as the
+ dictionary. Example: if the given tensor is [3, 1, 0], and given labels is ["background",
+ "apple", "banana", "cherry", "date"], the result will be ["date", "banana", "apple"].
+
+
Parameters
+
+
+
tensorBuffer
+
A tensor with index values. The values should be non-negative integers, and
+ each value x will be converted to labels[x + offset]. If the tensor is
+ given as a float TensorBuffer, values will be cast to integers. All values that are
+ out of bound will map to empty string.
+
+
+
labels
+
A list of strings, used as a dictionary to look up. The index of the array
+ element will be used as the key. To get better performance, use an object that implements
+ RandomAccess, such as ArrayList.
+
+
+
offset
+
The offset value when look up int values in the labels.
TensorLabel is an util wrapper for TensorBuffers with meaningful labels on an axis.
+
+
For example, an image classification model may have an output tensor with shape as {1, 10},
+ where 1 is the batch size and 10 is the number of categories. In fact, on the 2nd axis, we could
+ label each sub-tensor with the name or description of each corresponding category. TensorLabel could help converting the plain Tensor in TensorBuffer into a map from
+ predefined labels to sub-tensors. In this case, if provided 10 labels for the 2nd axis, TensorLabel could convert the original {1, 10} Tensor to a 10 element map, each value of which
+ is Tensor in shape {} (scalar). Usage example:
+
+
+ TensorBuffer outputTensor = ...;
+ List<String> labels = FileUtil.loadLabels(context, labelFilePath);
+ // labels the first axis with size greater than one
+ TensorLabel labeled = new TensorLabel(labels, outputTensor);
+ // If each sub-tensor has effectively size 1, we can directly get a float value
+ Map<String, Float> probabilities = labeled.getMapWithFloatValue();
+ // Or get sub-tensors, when each sub-tensor has elements more than 1
+ Map<String, TensorBuffer> subTensors = labeled.getMapWithTensorBuffer();
+
+
Note: currently we only support tensor-to-map conversion for the first label with size greater
+ than 1.
Creates a TensorLabel object which is able to label on the axes of multi-dimensional tensors.
+
+
Parameters
+
+
+
axisLabels
+
A map, whose key is axis id (starting from 0) and value is corresponding
+ labels. Note: The size of labels should be same with the size of the tensor on that axis.
if any key in axisLabels is out of range (compared to
+ the shape of tensorBuffer, or any value (labels) has different size with the tensorBuffer on the given dimension.
+
Creates a TensorLabel object which is able to label on one axis of multi-dimensional tensors.
+
+
Note: The labels are applied on the first axis whose size is larger than 1. For example, if
+ the shape of the tensor is [1, 10, 3], the labels will be applied on axis 1 (id starting from
+ 0), and size of axisLabels should be 10 as well.
+
+
Parameters
+
+
+
axisLabels
+
A list of labels, whose size should be same with the size of the tensor on
+ the to-be-labeled axis.
The axis of label should be effectively the last axis (which means every sub tensor
+ specified by this axis should have a flat size of 1), so that each labelled sub tensor could be
+ converted into a float value score. Example: A TensorLabel with shape {2, 5, 3}
+ and axis 2 is valid. If axis is 1 or 0, it cannot be converted into a Category.
+
+
Gets a map that maps label to float. Only allow the mapping on the first axis with size greater
+ than 1, and the axis should be effectively the last axis (which means every sub tensor
+ specified by this axis should have a flat size of 1).
+
+
Gets the map with a pair of the label and the corresponding TensorBuffer. Only allow the
+ mapping on the first axis with size greater than 1 currently.
+
Some models contain a TFLite Metadata Flatbuffer, which records more information about what
+ the model does and how to interprete the model. TFLite Metadata Flatbuffer can be generated using
+ the TFLite
+ Metadata schema file.
+
It is allowed to pass in a model FlatBuffer without TFLite metadata. However, invoking methods
+ that read from TFLite metadata will cause runtime errors.
+
+
Similarly, it is allowed to pass in a model FlatBuffer without associated files. However,
+ invoking methods that read the associated files will cause runtime errors.
+
+
Returns true if the minimum parser version required by the given metadata flatbuffer
+ precedes or equals to the version of the metadata parser that this MetadataExtractor library is
+ relying on.
Returns true if the model has metadata. Otherwise, returns false.
+
+
+
+
+
+
+ public
+
+ final
+
+
+ boolean
+
+isMinimumParserVersionSatisfied
+()
+
+
+
+
+
+
Returns true if the minimum parser version required by the given metadata flatbuffer
+ precedes or equals to the version of the metadata parser that this MetadataExtractor library is
+ relying on. All fields in the metadata can be parsed correctly with this metadata extractor
+ library in this case. Otherwise, it returns false.
+
+
For example, assume the underlying metadata parser version is 1.14.1,
+
+
+
it returns true, if the required minimum parser version is the same or older,
+ such as 1.14.1 or 1.14.0. Null version precedes all numeric versions,
+ because some metadata flatbuffers are generated before the first versioned release;
+
it returns false, if the required minimum parser version is newer, such as 1.14.2.
+
+
+ public
+ static
+ final
+
+
+ String
+
+VERSION
+
+
+
+
+
The version of the metadata parser that this metadata extractor library is depending on. The
+ value should match the value of "Schema Semantic version" in metadata_schema.fbs.
+
Runs model inference on multiple inputs, and returns multiple outputs.
+
+
Parameters
+
+
+
inputs
+
an array of input data. The inputs should be in the same order as inputs of the
+ model. Each input can be an array or multidimensional array, or a ByteBuffer of primitive types including int, float, long, and byte. ByteBuffer is the preferred way to pass large input data, whereas string types
+ require using the (multi-dimensional) array input path. When ByteBuffer is
+ used, its content should remain unchanged until model inference is done.
+
+
+
outputs
+
a map mapping output indices to multidimensional arrays of output data or ByteBuffers of primitive types including int, float, long, and byte. It only
+ needs to keep entries for the outputs to be used.
+
Dynamic TensorBuffers will reallocate memory when loading arrays or data buffers of
+ different buffer sizes. Here are some examples:
+
+
+ // Creating a float dynamic TensorBuffer:
+ TensorBuffer tensorBuffer = TensorBuffer.createDynamic(DataType.FLOAT32);
+ // Loading a float array:
+ float[] arr1 = new float[] {1, 2, 3};
+ tensorBuffer.loadArray(arr, new int[] {arr1.length});
+ // loading another float array:
+ float[] arr2 = new float[] {1, 2, 3, 4, 5};
+ tensorBuffer.loadArray(arr, new int[] {arr2.length});
+ // loading a third float array with the same size as arr2, assuming shape doesn't change:
+ float[] arr3 = new float[] {5, 4, 3, 2, 1};
+ tensorBuffer.loadArray(arr);
+ // loading a forth float array with different size as arr3 and omitting the shape will result
+ // in error:
+ float[] arr4 = new float[] {3, 2, 1};
+ tensorBuffer.loadArray(arr); // Error: The size of byte buffer and the shape do not match.
+
Returns a float array of the values stored in this buffer. If the buffer is of different types
+ than float, the values will be converted into float. For example, values in TensorBufferUint8 will be converted from uint8 to float.
+
Returns a float value at a given index. If the buffer is of different types than float, the
+ value will be converted into float. For example, when reading a value from TensorBufferUint8, the value will be first read out as uint8, and then will be converted from
+ uint8 to float.
+
+
+ For example, a TensorBuffer with shape {2, 3} that represents the following array,
+ [[0.0f, 1.0f, 2.0f], [3.0f, 4.0f, 5.0f]].
+
+ The fourth element (whose value is 3.0f) in the TensorBuffer can be retrieved by:
+ float v = tensorBuffer.getFloatValue(3);
+
Returns an int array of the values stored in this buffer. If the buffer is of different type
+ than int, the values will be converted into int, and loss of precision may apply. For example,
+ getting an int array from a TensorBufferFloat with values {400.32f, 23.04f}, the output
+ is {400, 23}.
+
+
+
+
+
+
+
+ public
+
+
+ abstract
+
+ int
+
+getIntValue
+(int absIndex)
+
+
+
+
+
+
Returns an int value at a given index. If the buffer is of different types than int, the value
+ will be converted into int. For example, when reading a value from TensorBufferFloat,
+ the value will be first read out as float, and then will be converted from float to int. Loss
+ of precision may apply.
+
+
+ For example, a TensorBuffer with shape {2, 3} that represents the following array,
+ [[0.0f, 1.0f, 2.0f], [3.0f, 4.0f, 5.0f]].
+
+ The fourth element (whose value is 3.0f) in the TensorBuffer can be retrieved by:
+ int v = tensorBuffer.getIntValue(3);
+ Note that v is converted from 3.0f to 3 as a result of type conversion.
+
+
+
Parameters
+
+
+
absIndex
+
The absolute index of the value to be read.
+
+
+
+
+
+
+
+
+
+
+ public
+
+
+
+
+ int[]
+
+getShape
+()
+
+
+
+
+
+
Gets the current shape. (returning a copy here to avoid unexpected modification.)
Loads an int array into this buffer with specific shape. If the buffer is of different types
+ than int, the values will be converted into the buffer's type before being loaded into the
+ buffer, and loss of precision may apply. For example, loading an int array with values {400,
+ -23} into a TensorBufferUint8 , the values will be clamped to [0, 255] and then be
+ casted to uint8 by {255, 0}.
Loads a float array into this buffer with specific shape. If the buffer is of different types
+ than float, the values will be converted into the buffer's type before being loaded into the
+ buffer, and loss of precision may apply. For example, loading a float array into a TensorBufferUint8 with values {400.32f, -23.04f}, the values will be clamped to [0, 255] and
+ then be casted to uint8 by {255, 0}.
Loads a float array into this buffer. If the buffer is of different types than float, the
+ values will be converted into the buffer's type before being loaded into the buffer, and loss
+ of precision may apply. For example, loading a float array into a TensorBufferUint8
+ with values {400.32f, -23.04f}, the values will be clamped to [0, 255] and then be casted to
+ uint8 by {255, 0}.
+
+
Using this method assumes that the shape of src is the same as the shape of this
+ TensorBuffer. Thus the size of buffer (src.length) should always match
+ the flat size of this TensorBuffer, for both fixed-size and dynamic TensorBuffer. Use loadArray(float[], int[]) if src has a different shape.
Loads an int array into this buffer. If the buffer is of different types than int, the values
+ will be converted into the buffer's type before being loaded into the buffer, and loss of
+ precision may apply. For example, loading an int array with values {400, -23} into a TensorBufferUint8 , the values will be clamped to [0, 255] and then be casted to uint8 by
+ {255, 0}.
+
+
Using this method assumes that the shape of src is the same as the shape of this
+ TensorBuffer. Thus the size of buffer (src.length) should always match
+ the flat size of this TensorBuffer, for both fixed-size and dynamic TensorBuffer. Use loadArray(int[], int[]) if src has a different shape.
Loads a byte buffer into this TensorBuffer. Buffer size must match the flat size of
+ this TensorBuffer.
+
+
Using this method assumes that the shape of buffer is the same as the shape of this
+ TensorBuffer. Thus the size of buffer (buffer.limit()) should always
+ match the flat size of this TensorBuffer, for both fixed-size and dynamic TensorBuffer. Use loadBuffer(ByteBuffer, int[]) if buffer has a different
+ shape.
+
+
Important: The loaded buffer is a reference. DO NOT MODIFY. We don't create a copy here for
+ performance concern, but if modification is necessary, please make a copy.
+
+
For the best performance, always load a direct ByteBuffer or a ByteBuffer
+ backed by an array.
+
+
If the buffer is read-only, we adopt a copy-on-write strategy for performance.
Loads a byte buffer into this TensorBuffer with specific shape.
+
+
Important: The loaded buffer is a reference. DO NOT MODIFY. We don't create a copy here for
+ performance concern, but if modification is necessary, please make a copy.
+
+
For the best performance, always load a direct ByteBuffer or a ByteBuffer
+ backed by an array.
Returns a float array of the values stored in this buffer. If the buffer is of different types
+ than float, the values will be converted into float. For example, values in TensorBufferUint8 will be converted from uint8 to float.
+
Returns a float value at a given index. If the buffer is of different types than float, the
+ value will be converted into float. For example, when reading a value from TensorBufferUint8, the value will be first read out as uint8, and then will be converted from
+ uint8 to float.
+
+
+ For example, a TensorBuffer with shape {2, 3} that represents the following array,
+ [[0.0f, 1.0f, 2.0f], [3.0f, 4.0f, 5.0f]].
+
+ The fourth element (whose value is 3.0f) in the TensorBuffer can be retrieved by:
+ float v = tensorBuffer.getFloatValue(3);
+
+
+
Parameters
+
+
+
absIndex
+
The absolute index of the value to be read.
+
+
+
+
+
+
+
+
+
+
+ public
+
+
+
+
+ int[]
+
+getIntArray
+()
+
+
+
+
+
+
Returns an int array of the values stored in this buffer. If the buffer is of different type
+ than int, the values will be converted into int, and loss of precision may apply. For example,
+ getting an int array from a TensorBufferFloat with values {400.32f, 23.04f}, the output
+ is {400, 23}.
+
+
+
+
+
+
+
+ public
+
+
+
+
+ int
+
+getIntValue
+(int absIndex)
+
+
+
+
+
+
Returns an int value at a given index. If the buffer is of different types than int, the value
+ will be converted into int. For example, when reading a value from TensorBufferFloat,
+ the value will be first read out as float, and then will be converted from float to int. Loss
+ of precision may apply.
+
+
+ For example, a TensorBuffer with shape {2, 3} that represents the following array,
+ [[0.0f, 1.0f, 2.0f], [3.0f, 4.0f, 5.0f]].
+
+ The fourth element (whose value is 3.0f) in the TensorBuffer can be retrieved by:
+ int v = tensorBuffer.getIntValue(3);
+ Note that v is converted from 3.0f to 3 as a result of type conversion.
+
+
+
Parameters
+
+
+
absIndex
+
The absolute index of the value to be read.
+
+
+
+
+
+
+
+
+
+
+ public
+
+
+
+
+ int
+
+getTypeSize
+()
+
+
+
+
+
+
Returns the number of bytes of a single element in the array. For example, a float buffer will
+ return 4, and a byte buffer will return 1.
+
Loads an int array into this buffer with specific shape. If the buffer is of different types
+ than int, the values will be converted into the buffer's type before being loaded into the
+ buffer, and loss of precision may apply. For example, loading an int array with values {400,
+ -23} into a TensorBufferUint8 , the values will be clamped to [0, 255] and then be
+ casted to uint8 by {255, 0}.
Loads a float array into this buffer with specific shape. If the buffer is of different types
+ than float, the values will be converted into the buffer's type before being loaded into the
+ buffer, and loss of precision may apply. For example, loading a float array into a TensorBufferUint8 with values {400.32f, -23.04f}, the values will be clamped to [0, 255] and
+ then be casted to uint8 by {255, 0}.
Returns a float array of the values stored in this buffer. If the buffer is of different types
+ than float, the values will be converted into float. For example, values in TensorBufferUint8 will be converted from uint8 to float.
+
Returns a float value at a given index. If the buffer is of different types than float, the
+ value will be converted into float. For example, when reading a value from TensorBufferUint8, the value will be first read out as uint8, and then will be converted from
+ uint8 to float.
+
+
+ For example, a TensorBuffer with shape {2, 3} that represents the following array,
+ [[0.0f, 1.0f, 2.0f], [3.0f, 4.0f, 5.0f]].
+
+ The fourth element (whose value is 3.0f) in the TensorBuffer can be retrieved by:
+ float v = tensorBuffer.getFloatValue(3);
+
+
+
Parameters
+
+
+
index
+
The absolute index of the value to be read.
+
+
+
+
+
+
+
+
+
+
+ public
+
+
+
+
+ int[]
+
+getIntArray
+()
+
+
+
+
+
+
Returns an int array of the values stored in this buffer. If the buffer is of different type
+ than int, the values will be converted into int, and loss of precision may apply. For example,
+ getting an int array from a TensorBufferFloat with values {400.32f, 23.04f}, the output
+ is {400, 23}.
+
+
+
+
+
+
+
+ public
+
+
+
+
+ int
+
+getIntValue
+(int index)
+
+
+
+
+
+
Returns an int value at a given index. If the buffer is of different types than int, the value
+ will be converted into int. For example, when reading a value from TensorBufferFloat,
+ the value will be first read out as float, and then will be converted from float to int. Loss
+ of precision may apply.
+
+
+ For example, a TensorBuffer with shape {2, 3} that represents the following array,
+ [[0.0f, 1.0f, 2.0f], [3.0f, 4.0f, 5.0f]].
+
+ The fourth element (whose value is 3.0f) in the TensorBuffer can be retrieved by:
+ int v = tensorBuffer.getIntValue(3);
+ Note that v is converted from 3.0f to 3 as a result of type conversion.
+
+
+
Parameters
+
+
+
index
+
The absolute index of the value to be read.
+
+
+
+
+
+
+
+
+
+
+ public
+
+
+
+
+ int
+
+getTypeSize
+()
+
+
+
+
+
+
Returns the number of bytes of a single element in the array. For example, a float buffer will
+ return 4, and a byte buffer will return 1.
+
Loads an int array into this buffer with specific shape. If the buffer is of different types
+ than int, the values will be converted into the buffer's type before being loaded into the
+ buffer, and loss of precision may apply. For example, loading an int array with values {400,
+ -23} into a TensorBufferUint8 , the values will be clamped to [0, 255] and then be
+ casted to uint8 by {255, 0}.
Loads a float array into this buffer with specific shape. If the buffer is of different types
+ than float, the values will be converted into the buffer's type before being loaded into the
+ buffer, and loss of precision may apply. For example, loading a float array into a TensorBufferUint8 with values {400.32f, -23.04f}, the values will be clamped to [0, 255] and
+ then be casted to uint8 by {255, 0}.
If non-empty, classifications whose label is not in this set will be filtered out.
+ Duplicate or unknown labels are ignored. Mutually exclusive with labelDenyList.
+
If non-empty, classifications whose label is in this set will be filtered out. Duplicate
+ or unknown labels are ignored. Mutually exclusive with labelAllowList.
+
Performs actual classification on the provided audio tensor.
+
+
Parameters
+
+
+
tensor
+
a TensorAudio containing the input audio clip in float with values
+ between [-1, 1). The tensor argument should have the same flat size as the TFLite
+ model's input tensor. It's recommended to create tensor using createInputTensorAudio method.
Creates an AudioRecord instance to record audio stream. The returned
+ AudioRecord instance is initialized and client needs to call AudioRecord.startRecordingnull method to start recording.
The classification results of one head in a multihead (a.k.a. multi-output) AudioClassifier. A multihead AudioClassifier can perform classification for multiple
+ purposes, such as a fine grained classifier to distinguish different bird sounds.
+
+ public static final enum
+ ImageProcessingOptions.Orientation
+
+
+
+
+
Orientation type that follows EXIF specification.
+
+
The name of each enum value defines the position of the 0th row and the 0th column of the
+ image content. See the EXIF orientation
+ documentation for details.
+
+ public abstract class
+ ImageProcessingOptions
+
+
+
+
+
Options to configure the image processing pipeline, which operates before inference.
+
+
The Task Library Vision API performs image preprocessing on the input image over the region of
+ interest, so that it fits model requirements (e.g. upright 224x224 RGB) and populate the
+ corresponding input tensor. This is performed by (in this order):
+
+
+
cropping the frame buffer to the region of interest (which, in most cases, just covers the
+ entire input image),
+
resizing it (with bilinear interpolation, aspect-ratio *not* preserved) to the dimensions
+ of the model input tensor,
+
converting it to the colorspace of the input tensor (i.e. RGB, which is the only supported
+ colorspace for now),
+
IMPORTANT: as a consequence of cropping occurring first, the provided region of interest is
+ expressed in the unrotated frame of reference coordinates system, i.e. in [0,
+ TensorImage.getWidth()) x [0, TensorImage.getHeight()), which are the dimensions of the
+ underlying image data before any orientation gets applied. If the region is out of these bounds,
+ the inference method, such as ImageClassifier.classify(MlImage), will return error.
+
Initializes the TFLite Tasks Audio API. TFLite Tasks Audio API methods should only be called
+ after the task returned by this method has successfully completed.
+
+
This method returns a Task<Void>, so you should wait for the task to be completed,
+ but the return value of the Task is irrelevant.
+
Initializes the TFLite Tasks Audio API with the specified options. TFLite Tasks Audio API
+ methods should only be called after the task returned by this method has successfully
+ completed.
+
+
This method returns a Task<Void>, so you should wait for the task to be completed,
+ but the return value of the Task is irrelevant.
+
Initializes the TFLite Tasks Text API. TFLite Tasks Text API methods should only be called
+ after the task returned by this method has successfully completed.
+
+
This method returns a Task<Void>, so you should wait for the task to be completed,
+ but the return value of the Task is irrelevant.
+
Initializes the TFLite Tasks Text API with the specified options. TFLite Tasks Text API methods
+ should only be called after the task returned by this method has successfully completed.
+
+
This method returns a Task<Void>, so you should wait for the task to be completed,
+ but the return value of the Task is irrelevant.
+
Sets whether to normalize the embedding feature vector with L2 norm. Defaults to false.
+
+
Use this option only if the model does not already contain a native L2_NORMALIZATION
+ TFLite Op. In most cases, this is already the case and L2 norm is thus achieved through
+ TFLite inference.
+
Sets whether the embedding should be quantized to bytes via scalar quantization. Defaults to
+ false.
+
+
Embeddings are implicitly assumed to be unit-norm and therefore any dimension is
+ guaranteed to have a value in [-1.0, 1.0]. Use the l2_normalize option if this is not
+ the case.
+
Classifier API for NLClassification tasks with Bert models, categorizes string into different
+ classes. The API expects a Bert based TFLite model with metadata populated.
+
+
The metadata should contain the following information:
+
+
+
1 input_process_unit for Wordpiece/Sentencepiece Tokenizer.
+
3 input tensors with names "ids", "mask" and "segment_ids".
+
1 output tensor of type float32[1, 2], with a optionally attached label file. If a label
+ file is attached, the file should be a plain text file with one label per line, the number
+ of labels should match the number of categories the model outputs.
+
Set the index of the input text tensor among all input tensors, if the model has multiple
+ inputs. Only the input tensor specified will be used for inference; other input tensors
+ will be ignored. Dafualt to 0.
+
+
See the section, Configure the input/output tensors for NLClassifier, for more details.
+
Set the name of the input text tensor, if the model has multiple inputs. Only the input
+ tensor specified will be used for inference; other input tensors will be ignored. Dafualt
+ to "INPUT".
+
+
See the section, Configure the input/output tensors for NLClassifier, for more details.
+
Set the name of the output label tensor, if the model has multiple outputs. Dafualt to
+ "OUTPUT_LABEL".
+
+
See the section, Configure the input/output tensors for NLClassifier, for more details.
+
+
By default, label file should be packed with the output score tensor through Model
+ Metadata. See the MetadataWriter
+ for NLClassifier. NLClassifier reads and parses labels from the label file
+ automatically. However, some models may output a specific label tensor instead. In this
+ case, NLClassifier reads labels from the output label tensor.
+
output scores for each class, if type is one of the Int types, dequantize it, if it
+ is Bool type, convert the values to 0.0 and 1.0 respectively.
+
can have an optional associated file in metadata for labels, the file should be a
+ plain text file with one label per line, the number of labels should match the number
+ of categories the model outputs. Output label tensor: optional (kTfLiteString) -
+ output classname for each class, should be of the same length with scores. If this
+ tensor is not present, the API uses score indices as classnames. - will be ignored if
+ output score tensor already has an associated label file.
+
output classname for each class, should be of the same length with scores. If this
+ tensor is not present, the API uses score indices as classnames.
+
will be ignored if output score tensor already has an associated labe file.
+
+
+
By default the API tries to find the input/output tensors with default configurations in
+ NLClassifier.NLClassifierOptions, with tensor name prioritized over tensor index. The option is
+ configurable for different TFLite models.
+
Returns the most possible answers on a given question for QA models (BERT, Albert, etc.).
+
+
The API expects a Bert based TFLite model with metadata containing the following information:
+
+
+
input_process_units for Wordpiece/Sentencepiece Tokenizer - Wordpiece Tokenizer can be used
+ for a MobileBert model,
+ Sentencepiece Tokenizer Tokenizer can be used for an Albert model.
+
3 input tensors with names "ids", "mask" and "segment_ids".
+
2 output tensors with names "end_logits" and "start_logits".
+
The API expects a TFLite model with optional, but strongly recommended, TFLite Model Metadata..
+
+
The API expects a TFLite model with metadata populated. The metadata should contain the
+ following information:
+
+
+
For Bert based TFLite model:
+
+
3 input tensors of type kTfLiteString with names "ids", "mask" and "segment_ids".
+
input_process_units for Wordpiece/Sentencepiece Tokenizer
+
exactly one output tensor of type kTfLiteFloat32
+
+
For Regex based TFLite model:
+
+
1 input tensor.
+
input_process_units for RegexTokenizer Tokenizer
+
exactly one output tensor of type kTfLiteFloat32
+
+
For Universal Sentence Encoder based TFLite model:
+
+
3 input tensors with names "inp_text", "res_context" and "res_text"
+
2 output tensors with names "query_encoding" and "response_encoding" of type
+ kTfLiteFloat32
+
+
+
TODO(b/180502532): add pointer to example model.
+
+
TODO(b/222671076): add factory create methods without options, such as `createFromFile`, once
+ the single file format (index file packed in the model) is supported.
+
The classification results of one head in a multihead (a.k.a. multi-output) ImageClassifier. A multihead ImageClassifier can perform classification for multiple
+ purposes, such as a fine grained classifier to describe apparel items (e.g. color, material,
+ type, etc.).
+
+ This method is deprecated. use BaseOptions to configure number of threads instead. This method
+ will override the number of threads configured from BaseOptions.
+
If non-empty, classifications whose label is not in this set will be filtered out.
+ Duplicate or unknown labels are ignored. Mutually exclusive with labelDenyList.
+
If non-empty, classifications whose label is in this set will be filtered out. Duplicate
+ or unknown labels are ignored. Mutually exclusive with labelAllowList.
+
+
+ This method is deprecated. use BaseOptions to configure number of threads instead. This method
+ will override the number of threads configured from BaseOptions.
+
+
+
Sets the number of threads to be used for TFLite ops that support multi-threading when
+ running inference with CPU. Defaults to -1.
+
+
numThreads should be greater than 0 or equal to -1. Setting numThreads to -1 has the
+ effect to let TFLite runtime set the value.
+ This method is deprecated. use BaseOptions to configure number of threads instead. This method
+ will override the number of threads configured from BaseOptions.
+
Sets the maximum number of top-scored detection results to return.
+
+
If < 0, all available results will be returned. If 0, an invalid argument error is
+ returned. Note that models may intrinsically be limited to returning a maximum number of
+ results N: if the provided value here is above N, only N results will be returned. Defaults
+ to -1.
+
+ This method is deprecated. use BaseOptions to configure number of threads instead. This method
+ will override the number of threads configured from BaseOptions.
+
+
+
Sets the number of threads to be used for TFLite ops that support multi-threading when
+ running inference with CPU. Defaults to -1.
+
+
numThreads should be greater than 0 or equal to -1. Setting numThreads to -1 has the
+ effect to let TFLite runtime set the value.
image input of size [batch x height x width x channels].
+
batch inference is not supported (batch is required to be 1).
+
only RGB inputs are supported (channels is required to be 3).
+
if type is kTfLiteFloat32, NormalizationOptions are required to be attached
+ to the metadata for input normalization.
+
+
Output tensor (kTfLiteUInt8/kTfLiteFloat32)
+
+
N components corresponding to the N dimensions of the returned
+ feature vector for this output layer.
+
Either 2 or 4 dimensions, i.e. [1 x N] or [1 x 1 x 1 x N].
+
+
+
TODO(b/180502532): add pointer to example model.
+
+
TODO(b/222671076): add factory create methods without options, such as `createFromFile`, once
+ the single file format (index file packed in the model) is supported.
+
the color components for the label. The Color instatnce is supported on Android
+ API level 26 and above. For API level lower than 26, use create(String, String, int). See Android
+ Color instances. for more details.
+
+
+
+
+
+
+
+
+
+
+ public
+ static
+
+
+
+ ColoredLabel
+
+create
+(String label, String displayName, int argb)
+
+
+
+
+
+
Creates a ColoredLabel object with an ARGB color int.
+
+
Parameters
+
+
+
label
+
the label string, as provided in the label map packed in the TFLite Model
+ Metadata.
Gets the Color instance of the underlying color.
+
+
The Color instatnce is supported on Android API level 26 and above. For API level lower than
+ 26, use getArgb(). See Android
+ Color instances. for more details.
+
+ This method is deprecated. use BaseOptions to configure number of threads instead. This method
+ will override the number of threads configured from BaseOptions.
+
+
+ This method is deprecated. use BaseOptions to configure number of threads instead. This method
+ will override the number of threads configured from BaseOptions.
+
+
+
Sets the number of threads to be used for TFLite ops that support multi-threading when
+ running inference with CPU. Defaults to -1.
+
+
numThreads should be greater than 0 or equal to -1. Setting numThreads to -1 has the
+ effect to let TFLite runtime set the value.
tensor of size [batch x mask_height x mask_width x num_classes], where batch is required to be 1, mask_width and mask_height are the
+ dimensions of the segmentation masks produced by the model, and num_classes
+ is the number of classes supported by the model.
+
optional (but recommended) label map(s) can be attached as AssociatedFile-s with type
+ TENSOR_AXIS_LABELS, containing one label per line. The first such AssociatedFile (if
+ any) is used to fill the class name, i.e. ColoredLabel.getlabel() of the
+ results. The display name, i.e. ColoredLabel.getDisplayName(), is filled from
+ the AssociatedFile (if any) whose locale matches the `display_names_locale` field of
+ the `ImageSegmenterOptions` used at creation time ("en" by default, i.e. English). If
+ none of these are available, only the `index` field of the results will be filled.
+
a UINT8 TensorImage object that represents an RGB or YUV image
+
+
+
+
+
Returns
+
results of performing image segmentation. Note that at the time, a single Segmentation element is expected to be returned. The result is stored in a List
+ for later extension to e.g. instance segmentation models, which may return one segmentation
+ per object.
Performs actual segmentation on the provided MlImage.
+
+
Parameters
+
+
+
image
+
an MlImage to segment.
+
+
+
+
+
Returns
+
results of performing image segmentation. Note that at the time, a single Segmentation element is expected to be returned. The result is stored in a List
+ for later extension to e.g. instance segmentation models, which may return one segmentation
+ per object.
a UINT8 TensorImage object that represents an RGB or YUV image
+
+
+
options
+
the options configure how to preprocess the image
+
+
+
+
+
Returns
+
results of performing image segmentation. Note that at the time, a single Segmentation element is expected to be returned. The result is stored in a List
+ for later extension to e.g. instance segmentation models, which may return one segmentation
+ per object.
the options configure how to preprocess the image.
+
+
+
+
+
Returns
+
results of performing image segmentation. Note that at the time, a single Segmentation element is expected to be returned. The result is stored in a List
+ for later extension to e.g. instance segmentation models, which may return one segmentation
+ per object.
+
+
+
+Public API for tf.lite namespace.
+
+
+
+## Modules
+
+[`experimental`](../tf/lite/experimental) module: Public API for tf.lite.experimental namespace.
+
+## Classes
+
+[`class Interpreter`](../tf/lite/Interpreter): Interpreter interface for running TensorFlow Lite models.
+
+[`class OpsSet`](../tf/lite/OpsSet): Enum class defining the sets of ops available to generate TFLite models.
+
+[`class Optimize`](../tf/lite/Optimize): Enum defining the optimizations to apply when generating a tflite model.
+
+[`class RepresentativeDataset`](../tf/lite/RepresentativeDataset): Representative dataset used to optimize the model.
+
+[`class TFLiteConverter`](../tf/lite/TFLiteConverter): Converts a TensorFlow model into TensorFlow Lite model.
+
+[`class TargetSpec`](../tf/lite/TargetSpec): Specification of target device used to optimize the model.
diff --git a/site/en/lite/api_docs/python/tf/lite/Interpreter.md b/site/en/lite/api_docs/python/tf/lite/Interpreter.md
new file mode 100644
index 00000000000..671ec34e5ae
--- /dev/null
+++ b/site/en/lite/api_docs/python/tf/lite/Interpreter.md
@@ -0,0 +1,778 @@
+page_type: reference
+description: Interpreter interface for running TensorFlow Lite models.
+
+
+
+
+
+
+
+
+
+Models obtained from `TfLiteConverter` can be run in Python with
+`Interpreter`.
+
+As an example, lets generate a simple Keras model and convert it to TFLite
+(`TfLiteConverter` also supports other input formats with `from_saved_model`
+and `from_concrete_function`)
+
+
+
+
+`tflite_model` can be saved to a file and loaded later, or directly into the
+`Interpreter`. Since TensorFlow Lite pre-plans tensor allocations to optimize
+inference, the user needs to call `allocate_tensors()` before any inference.
+
+
+interpreter = tf.lite.Interpreter(model_content=tflite_model)
+interpreter.allocate_tensors() # Needed before execution!
+
+
+
+#### Sample execution:
+
+
+output = interpreter.get_output_details()[0] # Model has single output.
+input = interpreter.get_input_details()[0] # Model has single input.
+input_data = tf.constant(1., shape=[1, 1])
+interpreter.set_tensor(input['index'], input_data)
+interpreter.invoke()
+interpreter.get_tensor(output['index']).shape
+(1, 1)
+
+
+
+Use `get_signature_runner()` for a more user-friendly inference API.
+
+
+
+
+
Args
+
+
+
+`model_path`
+
+
+Path to TF-Lite Flatbuffer file.
+
+
+
+`model_content`
+
+
+Content of model.
+
+
+
+`experimental_delegates`
+
+
+Experimental. Subject to change. List of
+[TfLiteDelegate](https://www.tensorflow.org/lite/performance/delegates)
+ objects returned by lite.load_delegate().
+
+
+
+`num_threads`
+
+
+Sets the number of threads used by the interpreter and
+available to CPU kernels. If not set, the interpreter will use an
+implementation-dependent default number of threads. Currently, only a
+subset of kernels, such as conv, support multi-threading. num_threads
+should be >= -1. Setting num_threads to 0 has the effect to disable
+multithreading, which is equivalent to setting num_threads to 1. If set
+to the value -1, the number of threads used will be
+implementation-defined and platform-dependent.
+
+
+
+`experimental_op_resolver_type`
+
+
+The op resolver used by the interpreter. It
+must be an instance of OpResolverType. By default, we use the built-in
+op resolver which corresponds to tflite::ops::builtin::BuiltinOpResolver
+in C++.
+
+
+
+`experimental_preserve_all_tensors`
+
+
+If true, then intermediate tensors used
+during computation are preserved for inspection, and if the passed op
+resolver type is AUTO or BUILTIN, the type will be changed to
+BUILTIN_WITHOUT_DEFAULT_DELEGATES so that no Tensorflow Lite default
+delegates are applied. If false, getting intermediate tensors could
+result in undefined values or None, especially when the graph is
+successfully modified by the Tensorflow Lite default delegate.
+
+A list in which each item is a dictionary with details about
+an input tensor. Each dictionary contains the following fields
+that describe the tensor:
+
++ `name`: The tensor name.
++ `index`: The tensor index in the interpreter.
++ `shape`: The shape of the tensor.
++ `shape_signature`: Same as `shape` for models with known/fixed shapes.
+ If any dimension sizes are unknown, they are indicated with `-1`.
+
++ `dtype`: The numpy data type (such as `np.int32` or `np.uint8`).
++ `quantization`: Deprecated, use `quantization_parameters`. This field
+ only works for per-tensor quantization, whereas
+ `quantization_parameters` works in all cases.
++ `quantization_parameters`: A dictionary of parameters used to quantize
+ the tensor:
+ ~ `scales`: List of scales (one if per-tensor quantization).
+ ~ `zero_points`: List of zero_points (one if per-tensor quantization).
+ ~ `quantized_dimension`: Specifies the dimension of per-axis
+ quantization, in the case of multiple scales/zero_points.
++ `sparsity_parameters`: A dictionary of parameters used to encode a
+ sparse tensor. This is empty if the tensor is dense.
+
+A list in which each item is a dictionary with details about
+an output tensor. The dictionary contains the same fields as
+described for `get_input_details()`.
+
+
+Gets list of SignatureDefs in the model.
+
+Example,
+
+```
+signatures = interpreter.get_signature_list()
+print(signatures)
+
+# {
+# 'add': {'inputs': ['x', 'y'], 'outputs': ['output_0']}
+# }
+
+Then using the names in the signature list you can get a callable from
+get_signature_runner().
+```
+
+
+
+
+
Returns
+
+
+A list of SignatureDef details in a dictionary structure.
+It is keyed on the SignatureDef method name, and the value holds
+dictionary of inputs and outputs.
+
+
+Gets callable for inference of specific SignatureDef.
+
+Example usage,
+
+```
+interpreter = tf.lite.Interpreter(model_content=tflite_model)
+interpreter.allocate_tensors()
+fn = interpreter.get_signature_runner('div_with_remainder')
+output = fn(x=np.array([3]), y=np.array([2]))
+print(output)
+# {
+# 'quotient': array([1.], dtype=float32)
+# 'remainder': array([1.], dtype=float32)
+# }
+```
+
+None can be passed for signature_key if the model has a single Signature
+only.
+
+All names used are this specific SignatureDef names.
+
+
+
+
+
+
Args
+
+
+
+`signature_key`
+
+
+Signature key for the SignatureDef, it can be None if and
+only if the model has a single SignatureDef. Default value is None.
+
+
+
+
+
+
+
+
+
+
Returns
+
+
+This returns a callable that can run inference for SignatureDef defined
+by argument 'signature_key'.
+The callable will take key arguments corresponding to the arguments of the
+SignatureDef, that should have numpy values.
+The callable will returns dictionary that maps from output names to numpy
+values of the computed results.
+
+
+Gets the value of the output tensor (get a copy).
+
+If you wish to avoid the copy, use `tensor()`. This function cannot be used
+to read intermediate results.
+
+
+
+
+
Args
+
+
+
+`tensor_index`
+
+
+Tensor index of tensor to get. This value can be gotten from
+the 'index' field in get_output_details.
+
+
+
+`subgraph_index`
+
+
+Index of the subgraph to fetch the tensor. Default value
+is 0, which means to fetch from the primary subgraph.
+
+
+Gets tensor details for every tensor with valid tensor details.
+
+Tensors where required information about the tensor is not found are not
+added to the list. This includes temporary tensors without a name.
+
+
+
+
+
Returns
+
+
+A list of dictionaries containing tensor information.
+
+
+Invoke the interpreter.
+
+Be sure to set the input sizes, allocate tensors and fill values before
+calling this. Also, note that this function releases the GIL so heavy
+computation can be done in the background while the Python interpreter
+continues. No other function on this object should be called while the
+invoke() call has not finished.
+
+
+
+
+
Raises
+
+
+
+`ValueError`
+
+
+When the underlying interpreter fails raise ValueError.
+
+Tensor index of input to set. This value can be gotten from
+the 'index' field in get_input_details.
+
+
+
+`tensor_size`
+
+
+The tensor_shape to resize the input to.
+
+
+
+`strict`
+
+
+Only unknown dimensions can be resized when `strict` is True.
+Unknown dimensions are indicated as `-1` in the `shape_signature`
+attribute of a given tensor. (default False)
+
+
+
+
+
+
+
+
+
+
Raises
+
+
+
+`ValueError`
+
+
+If the interpreter could not resize the input tensor.
+
+
+Sets the value of the input tensor.
+
+Note this copies data in `value`.
+
+If you want to avoid copying, you can use the `tensor()` function to get a
+numpy buffer pointing to the input buffer in the tflite interpreter.
+
+
+
+
+
Args
+
+
+
+`tensor_index`
+
+
+Tensor index of tensor to set. This value can be gotten from
+the 'index' field in get_input_details.
+
+
+Returns function that gives a numpy view of the current tensor buffer.
+
+This allows reading and writing to this tensors w/o copies. This more
+closely mirrors the C++ Interpreter class interface's tensor() member, hence
+the name. Be careful to not hold these output references through calls
+to `allocate_tensors()` and `invoke()`. This function cannot be used to read
+intermediate results.
+
+#### Usage:
+
+
+
+```
+interpreter.allocate_tensors()
+input = interpreter.tensor(interpreter.get_input_details()[0]["index"])
+output = interpreter.tensor(interpreter.get_output_details()[0]["index"])
+for i in range(10):
+ input().fill(3.)
+ interpreter.invoke()
+ print("inference %s" % output())
+```
+
+Notice how this function avoids making a numpy array directly. This is
+because it is important to not hold actual numpy views to the data longer
+than necessary. If you do, then the interpreter can no longer be invoked,
+because it is possible the interpreter would resize and invalidate the
+referenced tensors. The NumPy API doesn't allow any mutability of the
+the underlying buffers.
+
+#### WRONG:
+
+
+
+```
+input = interpreter.tensor(interpreter.get_input_details()[0]["index"])()
+output = interpreter.tensor(interpreter.get_output_details()[0]["index"])()
+interpreter.allocate_tensors() # This will throw RuntimeError
+for i in range(10):
+ input.fill(3.)
+ interpreter.invoke() # this will throw RuntimeError since input,output
+```
+
+
+
+
+
Args
+
+
+
+`tensor_index`
+
+
+Tensor index of tensor to get. This value can be gotten from
+the 'index' field in get_output_details.
+
+
+
+
+
+
+
+
+
+
Returns
+
+
+A function that can return a new numpy array pointing to the internal
+TFLite tensor state at any point. It is safe to hold the function forever,
+but it is not safe to hold the numpy array forever.
+
+
+
+
diff --git a/site/en/lite/api_docs/python/tf/lite/OpsSet.md b/site/en/lite/api_docs/python/tf/lite/OpsSet.md
new file mode 100644
index 00000000000..fd0a22dca45
--- /dev/null
+++ b/site/en/lite/api_docs/python/tf/lite/OpsSet.md
@@ -0,0 +1,75 @@
+page_type: reference
+description: Enum class defining the sets of ops available to generate TFLite models.
+
+
+
+
+
+
+
+
+
+
+Enum defining the optimizations to apply when generating a tflite model.
+
+
+
+DEFAULT
+ The default optimization strategy that enables post-training quantization.
+ The type of post-training quantization that will be used is dependent on
+ the other converter options supplied. Refer to the
+ [documentation](/lite/performance/post_training_quantization) for further
+ information on the types available and how to use them.
+
+OPTIMIZE_FOR_SIZE
+ Deprecated. Does the same as DEFAULT.
+
+OPTIMIZE_FOR_LATENCY
+ Deprecated. Does the same as DEFAULT.
+
+EXPERIMENTAL_SPARSITY
+ Experimental flag, subject to change.
+
+ Enable optimization by taking advantage of the sparse model weights
+ trained with pruning.
+
+ The converter will inspect the sparsity pattern of the model weights and
+ do its best to improve size and latency.
+ The flag can be used alone to optimize float32 models with sparse weights.
+ It can also be used together with the DEFAULT optimization mode to
+ optimize quantized models with sparse weights.
+
+
+
+
+
+
+
Class Variables
+
+
+
+DEFAULT
+
+
+``
+
+
+
+EXPERIMENTAL_SPARSITY
+
+
+``
+
+
+
+OPTIMIZE_FOR_LATENCY
+
+
+``
+
+
+
+OPTIMIZE_FOR_SIZE
+
+
+``
+
+
+
diff --git a/site/en/lite/api_docs/python/tf/lite/RepresentativeDataset.md b/site/en/lite/api_docs/python/tf/lite/RepresentativeDataset.md
new file mode 100644
index 00000000000..d6b693e2a58
--- /dev/null
+++ b/site/en/lite/api_docs/python/tf/lite/RepresentativeDataset.md
@@ -0,0 +1,65 @@
+page_type: reference
+description: Representative dataset used to optimize the model.
+
+
+
+
+
+
+
+
+
+
+Representative dataset used to optimize the model.
+
+
+tf.lite.RepresentativeDataset(
+ input_gen
+)
+
+
+
+
+
+
+This is a generator function that provides a small dataset to calibrate or
+estimate the range, i.e, (min, max) of all floating-point arrays in the model
+(such as model input, activation outputs of intermediate layers, and model
+output) for quantization. Usually, this is a small subset of a few hundred
+samples randomly chosen, in no particular order, from the training or
+evaluation dataset.
+
+
+
+
+
Args
+
+
+
+`input_gen`
+
+
+A generator function that generates input samples for the
+model and has the same order, type and shape as the inputs to the model.
+Usually, this is a small subset of a few hundred samples randomly
+chosen, in no particular order, from the training or evaluation dataset.
+
+
+
diff --git a/site/en/lite/api_docs/python/tf/lite/TFLiteConverter.md b/site/en/lite/api_docs/python/tf/lite/TFLiteConverter.md
new file mode 100644
index 00000000000..337210ecf0d
--- /dev/null
+++ b/site/en/lite/api_docs/python/tf/lite/TFLiteConverter.md
@@ -0,0 +1,535 @@
+page_type: reference
+description: Converts a TensorFlow model into TensorFlow Lite model.
+
+
+
+
+
+
+
+
+
+
+#### Example usage:
+
+
+
+```python
+# Converting a SavedModel to a TensorFlow Lite model.
+ converter = tf.lite.TFLiteConverter.from_saved_model(saved_model_dir)
+ tflite_model = converter.convert()
+
+# Converting a tf.Keras model to a TensorFlow Lite model.
+converter = tf.lite.TFLiteConverter.from_keras_model(model)
+tflite_model = converter.convert()
+
+# Converting ConcreteFunctions to a TensorFlow Lite model.
+converter = tf.lite.TFLiteConverter.from_concrete_functions([func], model)
+tflite_model = converter.convert()
+
+# Converting a Jax model to a TensorFlow Lite model.
+converter = tf.lite.TFLiteConverter.experimental_from_jax([func], [[
+ ('input1', input1), ('input2', input2)]])
+tflite_model = converter.convert()
+```
+
+
+
+
+
Args
+
+
+
+`funcs`
+
+
+List of TensorFlow ConcreteFunctions. The list should not contain
+duplicate elements.
+
+
+
+`trackable_obj`
+
+
+tf.AutoTrackable object associated with `funcs`. A
+reference to this object needs to be maintained so that Variables do not
+get garbage collected since functions have a weak reference to
+Variables. This is only required when the tf.AutoTrackable object is not
+maintained by the user (e.g. `from_saved_model`).
+
+
+
+
+
+
+
+
+
+
+
+
Attributes
+
+
+
+`optimizations`
+
+
+Experimental flag, subject to change. Set of optimizations to
+apply. e.g {tf.lite.Optimize.DEFAULT}. (default None, must be None or a
+set of values of type tf.lite.Optimize)
+
+
+
+`representative_dataset`
+
+
+A generator function used for integer quantization
+where each generated sample has the same order, type and shape as the
+inputs to the model. Usually, this is a small subset of a few hundred
+samples randomly chosen, in no particular order, from the training or
+evaluation dataset. This is an optional attribute, but required for full
+integer quantization, i.e, if tf.int8 is the only supported type in
+`target_spec.supported_types`. Refer to tf.lite.RepresentativeDataset.
+(default None)
+
+
+
+`target_spec`
+
+
+Experimental flag, subject to change. Specifications of target
+device, including supported ops set, supported types and a set of user's
+defined TensorFlow operators required in the TensorFlow Lite runtime.
+Refer to tf.lite.TargetSpec.
+
+
+
+`inference_input_type`
+
+
+Data type of the input layer. Note that integer types
+(tf.int8 and tf.uint8) are currently only supported for post training
+integer quantization and quantization aware training. (default tf.float32,
+must be in {tf.float32, tf.int8, tf.uint8})
+
+
+
+`inference_output_type`
+
+
+Data type of the output layer. Note that integer
+types (tf.int8 and tf.uint8) are currently only supported for post
+training integer quantization and quantization aware training. (default
+tf.float32, must be in {tf.float32, tf.int8, tf.uint8})
+
+
+
+`allow_custom_ops`
+
+
+Boolean indicating whether to allow custom operations.
+When False, any unknown operation is an error. When True, custom ops are
+created for any op that is unknown. The developer needs to provide these
+to the TensorFlow Lite runtime with a custom resolver. (default False)
+
+
+
+`exclude_conversion_metadata`
+
+
+Whether not to embed the conversion metadata
+into the converted model. (default False)
+
+Experimental flag, subject to change. Enables
+MLIR-based quantization conversion instead of Flatbuffer-based conversion.
+(default True)
+
+
+
+`experimental_enable_resource_variables`
+
+
+Experimental flag, subject to
+change. Enables
+[resource variables](https://tensorflow.org/guide/migrate/tf1_vs_tf2#resourcevariables_instead_of_referencevariables)
+to be converted by this converter. This is only allowed if the
+from_saved_model interface is used. (default True)
+
+
+Creates a TFLiteConverter object from ConcreteFunctions.
+
+
+
+
+
+
Args
+
+
+
+`funcs`
+
+
+List of TensorFlow ConcreteFunctions. The list should not contain
+duplicate elements. Currently converter can only convert a single
+ConcreteFunction. Converting multiple functions is under development.
+
+
+
+`trackable_obj`
+
+
+ An `AutoTrackable` object (typically `tf.module`)
+associated with `funcs`. A reference to this object needs to be
+maintained so that Variables do not get garbage collected since
+functions have a weak reference to Variables.
+
+
+Creates a TFLiteConverter object from a SavedModel directory.
+
+
+
+
+
+
Args
+
+
+
+`saved_model_dir`
+
+
+SavedModel directory to convert.
+
+
+
+`signature_keys`
+
+
+List of keys identifying SignatureDef containing inputs
+and outputs. Elements should not be duplicated. By default the
+`signatures` attribute of the MetaGraphdef is used. (default
+saved_model.signatures)
+
+
+
+`tags`
+
+
+Set of tags identifying the MetaGraphDef within the SavedModel to
+analyze. All tags in the tag set must be present. (default
+{tf.saved_model.SERVING} or {'serve'})
+
+
+
+
+
+
+
+
+
+
Returns
+
+
+TFLiteConverter object.
+
+
+
+
+
+
+
+
+
+
+
Raises
+
+
+Invalid signature keys.
+
+
+
+
diff --git a/site/en/lite/api_docs/python/tf/lite/TargetSpec.md b/site/en/lite/api_docs/python/tf/lite/TargetSpec.md
new file mode 100644
index 00000000000..e7798cedb24
--- /dev/null
+++ b/site/en/lite/api_docs/python/tf/lite/TargetSpec.md
@@ -0,0 +1,113 @@
+page_type: reference
+description: Specification of target device used to optimize the model.
+
+
+
+
+
+
+
+Experimental flag, subject to change. Set of tf.lite.OpsSet
+options, where each option represents a set of operators supported by the
+target device. (default {tf.lite.OpsSet.TFLITE_BUILTINS}))
+
+
+
+`supported_types`
+
+
+Set of tf.dtypes.DType data types supported on the target
+device. If initialized, optimization might be driven by the smallest type
+in this set. (default set())
+
+
+
+`experimental_select_user_tf_ops`
+
+
+Experimental flag, subject to change. Set
+of user's TensorFlow operators' names that are required in the TensorFlow
+Lite runtime. These ops will be exported as select TensorFlow ops in the
+model (in conjunction with the tf.lite.OpsSet.SELECT_TF_OPS flag). This is
+an advanced feature that should only be used if the client is using TF ops
+that may not be linked in by default with the TF ops that are provided
+when using the SELECT_TF_OPS path. The client is responsible for linking
+these ops into the target runtime.
+
+
+
+`experimental_supported_backends`
+
+
+Experimental flag, subject to change.
+Set containing names of supported backends. Currently only "GPU" is
+supported, more options will be available later.
+
+
+
+
+Public API for tf.lite.experimental namespace.
+
+
+
+## Modules
+
+[`authoring`](../../tf/lite/experimental/authoring) module: Public API for tf.lite.experimental.authoring namespace.
+
+## Classes
+
+[`class Analyzer`](../../tf/lite/experimental/Analyzer): Provides a collection of TFLite model analyzer tools.
+
+[`class OpResolverType`](../../tf/lite/experimental/OpResolverType): Different types of op resolvers for Tensorflow Lite.
+
+[`class QuantizationDebugOptions`](../../tf/lite/experimental/QuantizationDebugOptions): Debug options to set up a given QuantizationDebugger.
+
+[`class QuantizationDebugger`](../../tf/lite/experimental/QuantizationDebugger): Debugger for Quantized TensorFlow Lite debug mode models.
+
+## Functions
+
+[`load_delegate(...)`](../../tf/lite/experimental/load_delegate): Returns loaded Delegate object.
diff --git a/site/en/lite/api_docs/python/tf/lite/experimental/Analyzer.md b/site/en/lite/api_docs/python/tf/lite/experimental/Analyzer.md
new file mode 100644
index 00000000000..328ad057cd6
--- /dev/null
+++ b/site/en/lite/api_docs/python/tf/lite/experimental/Analyzer.md
@@ -0,0 +1,149 @@
+page_type: reference
+description: Provides a collection of TFLite model analyzer tools.
+
+
+
+
+
+
+
+
+Analyzes the given tflite_model with dumping model structure.
+
+This tool provides a way to understand users' TFLite flatbuffer model by
+dumping internal graph structure. It also provides additional features
+like checking GPU delegate compatibility.
+
+Warning: Experimental interface, subject to change.
+ The output format is not guaranteed to stay stable, so don't
+ write scripts to this.
+
+
+
+
+
Args
+
+
+
+`model_path`
+
+
+TFLite flatbuffer model path.
+
+
+
+`model_content`
+
+
+TFLite flatbuffer model object.
+
+
+
+`gpu_compatibility`
+
+
+Whether to check GPU delegate compatibility.
+
+
+
+`**kwargs`
+
+
+Experimental keyword arguments to analyze API.
+
+
+
+
+
+
+
+
+
+
Returns
+
+
+Print analyzed report via console output.
+
+
+
+
diff --git a/site/en/lite/api_docs/python/tf/lite/experimental/OpResolverType.md b/site/en/lite/api_docs/python/tf/lite/experimental/OpResolverType.md
new file mode 100644
index 00000000000..03f8bae1590
--- /dev/null
+++ b/site/en/lite/api_docs/python/tf/lite/experimental/OpResolverType.md
@@ -0,0 +1,85 @@
+page_type: reference
+description: Different types of op resolvers for Tensorflow Lite.
+
+
+
+
+
+
+
+
+
+
+Different types of op resolvers for Tensorflow Lite.
+
+
+
+* `AUTO`: Indicates the op resolver that is chosen by default in TfLite
+ Python, which is the "BUILTIN" as described below.
+* `BUILTIN`: Indicates the op resolver for built-in ops with optimized kernel
+ implementation.
+* `BUILTIN_REF`: Indicates the op resolver for built-in ops with reference
+ kernel implementation. It's generally used for testing and debugging.
+* `BUILTIN_WITHOUT_DEFAULT_DELEGATES`: Indicates the op resolver for
+ built-in ops with optimized kernel implementation, but it will disable
+ the application of default TfLite delegates (like the XNNPACK delegate) to
+ the model graph. Generally this should not be used unless there are issues
+ with the default configuration.
+
+
+
+
+
+
+
Class Variables
+
+
+
+AUTO
+
+
+``
+
+
+
+BUILTIN
+
+
+``
+
+
+
+BUILTIN_REF
+
+
+``
+
+
+
+BUILTIN_WITHOUT_DEFAULT_DELEGATES
+
+
+``
+
+
+
diff --git a/site/en/lite/api_docs/python/tf/lite/experimental/QuantizationDebugOptions.md b/site/en/lite/api_docs/python/tf/lite/experimental/QuantizationDebugOptions.md
new file mode 100644
index 00000000000..5743c638c18
--- /dev/null
+++ b/site/en/lite/api_docs/python/tf/lite/experimental/QuantizationDebugOptions.md
@@ -0,0 +1,149 @@
+page_type: reference
+description: Debug options to set up a given QuantizationDebugger.
+
+
+
+
+
+
+
+a dict to specify layer debug functions
+{function_name_str: function} where the function accepts result of
+ NumericVerify Op, which is value difference between float and
+ dequantized op results. The function returns single scalar value.
+
+
+
+`model_debug_metrics`
+
+
+a dict to specify model debug functions
+{function_name_str: function} where the function accepts outputs from
+ two models, and returns single scalar value for a metric. (e.g.
+ accuracy, IoU)
+
+
+
+`layer_direct_compare_metrics`
+
+
+a dict to specify layer debug functions
+{function_name_str: function}. The signature is different from that of
+ `layer_debug_metrics`, and this one gets passed (original float value,
+ original quantized value, scale, zero point). The function's
+ implementation is responsible for correctly dequantize the quantized
+ value to compare. Use this one when comparing diff is not enough.
+ (Note) quantized value is passed as int8, so cast to int32 is needed.
+
+
+
+`denylisted_ops`
+
+
+a list of op names which is expected to be removed from
+quantization.
+
+
+
+`denylisted_nodes`
+
+
+a list of op's output tensor names to be removed from
+quantization.
+
+
+
+`fully_quantize`
+
+
+Bool indicating whether to fully quantize the model.
+Besides model body, the input/output will be quantized as well.
+Corresponding to mlir_quantize's fully_quantize parameter.
+
+
+
+
+
+
+
+
+
+
Raises
+
+
+
+`ValueError`
+
+
+when there are duplicate keys
+
+
+
diff --git a/site/en/lite/api_docs/python/tf/lite/experimental/QuantizationDebugger.md b/site/en/lite/api_docs/python/tf/lite/experimental/QuantizationDebugger.md
new file mode 100644
index 00000000000..a64c7d59ee9
--- /dev/null
+++ b/site/en/lite/api_docs/python/tf/lite/experimental/QuantizationDebugger.md
@@ -0,0 +1,302 @@
+page_type: reference
+description: Debugger for Quantized TensorFlow Lite debug mode models.
+
+
+
+
+
+
+
+
+
+This can run the TensorFlow Lite converted models equipped with debug ops and
+collect debug information. This debugger calculates statistics from
+user-defined post-processing functions as well as default ones.
+
+
+
+
+
Args
+
+
+
+`quant_debug_model_path`
+
+
+Path to the quantized debug TFLite model file.
+
+
+
+`quant_debug_model_content`
+
+
+Content of the quantized debug TFLite model.
+
+
+
+`float_model_path`
+
+
+Path to float TFLite model file.
+
+
+
+`float_model_content`
+
+
+Content of the float TFLite model.
+
+
+
+`debug_dataset`
+
+
+a factory function that returns dataset generator which is
+used to generate input samples (list of np.ndarray) for the model. The
+generated elements must have same types and shape as inputs to the
+model.
+
+
+
+`debug_options`
+
+
+Debug options to debug the given model.
+
+
+
+`converter`
+
+
+Optional, use converter instead of quantized model.
+
+
+Returns an instrumented quantized model.
+
+Convert the quantized model with the initialized converter and
+return bytes for model. The model will be instrumented with numeric
+verification operations and should only be used for debugging.
+
+
+
+
+Returns a non-instrumented quantized model.
+
+Convert the quantized model with the initialized converter and
+return bytes for nondebug model. The model will not be instrumented with
+numeric verification operations.
+
+
+
+
+
+
+
+
+
+#### Example usage:
+
+
+
+```
+import tensorflow as tf
+
+try:
+ delegate = tf.lite.experimental.load_delegate('delegate.so')
+except ValueError:
+ // Fallback to CPU
+
+if delegate:
+ interpreter = tf.lite.Interpreter(
+ model_path='model.tflite',
+ experimental_delegates=[delegate])
+else:
+ interpreter = tf.lite.Interpreter(model_path='model.tflite')
+```
+
+This is typically used to leverage EdgeTPU for running TensorFlow Lite models.
+For more information see: https://coral.ai/docs/edgetpu/tflite-python/
+
+
+
+
+
Args
+
+
+
+`library`
+
+
+Name of shared library containing the
+[TfLiteDelegate](https://www.tensorflow.org/lite/performance/delegates).
+
+
+
+`options`
+
+
+Dictionary of options that are required to load the delegate. All
+keys and values in the dictionary should be convertible to str. Consult
+the documentation of the specific delegate for required and legal options.
+(default None)
+
+
+
+
+
+
+
+
+
+
Returns
+
+
+Delegate object.
+
+
+
+
+
+
+
+
+
+
+
Raises
+
+
+
+`ValueError`
+
+
+Delegate failed to load.
+
+
+
+`RuntimeError`
+
+
+If delegate loading is used on unsupported platform.
+
+
+
diff --git a/site/en/lite/api_docs/python/tflite_model_maker.md b/site/en/lite/api_docs/python/tflite_model_maker.md
new file mode 100644
index 00000000000..38c8da298a8
--- /dev/null
+++ b/site/en/lite/api_docs/python/tflite_model_maker.md
@@ -0,0 +1,94 @@
+page_type: reference
+description: Public APIs for TFLite Model Maker, a transfer learning library to train custom TFLite models.
+
+
+
+
+
+
+
+
+
+
+Public APIs for TFLite Model Maker, a transfer learning library to train custom TFLite models.
+
+
+You can install the package with
+
+```bash
+pip install tflite-model-maker
+```
+
+Typical usage of Model Maker is to create a model in a few lines of code, e.g.:
+
+```python
+# Load input data specific to an on-device ML app.
+data = DataLoader.from_folder('flower_photos/')
+train_data, test_data = data.split(0.9)
+
+# Customize the TensorFlow model.
+model = image_classifier.create(train_data)
+
+# Evaluate the model.
+accuracy = model.evaluate(test_data)
+
+# Export to Tensorflow Lite model and label file in `export_dir`.
+model.export(export_dir='/tmp/')
+```
+
+For more details, please refer to our guide:
+https://www.tensorflow.org/lite/guide/model_maker
+
+## Modules
+
+[`audio_classifier`](./tflite_model_maker/audio_classifier) module: APIs to train an audio classification model.
+
+[`config`](./tflite_model_maker/config) module: APIs for the config of TFLite Model Maker.
+
+[`image_classifier`](./tflite_model_maker/image_classifier) module: APIs to train an image classification model.
+
+[`model_spec`](./tflite_model_maker/model_spec) module: APIs for the model spec of TFLite Model Maker.
+
+[`object_detector`](./tflite_model_maker/object_detector) module: APIs to train an object detection model.
+
+[`question_answer`](./tflite_model_maker/question_answer) module: APIs to train a model that can answer questions based on a predefined text.
+
+[`recommendation`](./tflite_model_maker/recommendation) module: APIs to train an on-device recommendation model.
+
+[`searcher`](./tflite_model_maker/searcher) module: APIs to create the searcher model.
+
+[`text_classifier`](./tflite_model_maker/text_classifier) module: APIs to train a text classification model.
+
+
+
+
+
+A instance of audio_dataloader.DataLoader class.
+
+
+
+`model_spec`
+
+
+Specification for the model.
+
+
+
+`validation_data`
+
+
+Validation DataLoader. If None, skips validation process.
+
+
+
+`batch_size`
+
+
+Number of samples per training step. If `use_hub_library` is
+False, it represents the base learning rate when train batch size is 256
+and it's linear to the batch size.
+
+
+
+`epochs`
+
+
+Number of epochs for training.
+
+
+
+`model_dir`
+
+
+The location of the model checkpoint files.
+
+
+
+`do_train`
+
+
+Whether to run training.
+
+
+
+`train_whole_model`
+
+
+Boolean. By default, only the classification head is
+trained. When True, the base model is also trained.
+
+
+Converts the retrained model to tflite format and saves it.
+
+This method overrides the default `CustomModel._export_tflite` method, and
+include the pre-processing in the exported TFLite library since support
+library can't handle audio tasks yet.
+
+
+
+
+
Args
+
+
+
+`model`
+
+
+An instance of the keras classification model to be exported.
+
+
+
+`tflite_filepath`
+
+
+File path to save tflite model.
+
+
+
+`with_metadata`
+
+
+Whether the output tflite model contains metadata.
+
+
+
+`export_metadata_json_file`
+
+
+Whether to export metadata in json file. If
+True, export the metadata in the same directory as tflite model.Used
+only if `with_metadata` is True.
+
+
+
+`index_to_label`
+
+
+A list that map from index to label class name.
+
+A tf.data.Dataset object that contains a potentially large set of
+elements, where each element is a pair of (input_data, target). The
+`input_data` means the raw input data, like an image, a text etc., while
+the `target` means some ground truth of the raw input data, such as the
+classification label of the image etc.
+
+
+
+`size`
+
+
+The size of the dataset. tf.data.Dataset donesn't support a function
+to get the length directly since it's lazy-loaded and may be infinite.
+
+
+
+
+
+
+
+
+
+
+
+
Attributes
+
+
+
+`num_classes`
+
+
+
+
+
+
+`size`
+
+
+Returns the size of the dataset.
+
+Note that this function may return None becuase the exact size of the
+dataset isn't a necessary parameter to create an instance of this class,
+and tf.data.Dataset donesn't support a function to get the length directly
+since it's lazy-loaded and may be infinite.
+In most cases, however, when an instance of this class is created by helper
+functions like 'from_folder', the size of the dataset will be preprocessed,
+and this function can return an int representing the size of the dataset.
+
+
+Load ESC50 style audio samples.
+
+ESC50 file structure is expalined in https://github.com/karolpiczak/ESC-50
+Audio files should be put in `${data_path}/audio`
+Metadata file should be put in `${data_path}/meta/esc50.csv`
+
+Note that instead of relying on the `target` field in the CSV, a new
+`index_to_label` mapping is created based on the alphabet order of the
+available categories.
+
+
+
+
+
Args
+
+
+
+`spec`
+
+
+An instance of audio_spec.YAMNet
+
+
+
+`data_path`
+
+
+A string, location of the ESC50 dataset. It should contain at
+
+
+
+`folds`
+
+
+A integer list of selected folds. If empty, all folds will be
+selected.
+
+
+
+`categories`
+
+
+A string list of selected categories. If empty, all categories
+will be selected.
+
+
+
+`shuffle`
+
+
+boolean, if True, random shuffle data.
+
+
+
+`cache`
+
+
+str or boolean. When set to True, intermediate results will be
+cached in ram. When set to a file path in string, intermediate results
+will be cached in this file. Please note that, once file based cache is
+created, changes to the input data will have no effects until the cache
+file is removed or the filename is changed. More details can be found at
+https://www.tensorflow.org/api_docs/python/tf/data/Dataset#cache
+
+
+
+
+
+
+
+
+
+
Returns
+
+
+An instance of AudioDataLoader containing audio samples and labels.
+
+
+Load audio files from a data_path.
+
+- The root `data_path` folder contains a number of folders. The name for
+each folder is the name of the audio class.
+
+- Within each folder, there are a number of .wav files. Each .wav file
+corresponds to an example. Each .wav file is mono (single-channel) and has
+the typical 16 bit pulse-code modulation (PCM) encoding.
+
+- .wav files will be resampled to `spec.target_sample_rate` then fed into
+`spec.preprocess_ds` for split and other operations. Normally long wav files
+will be framed into multiple clips. And wav files shorter than a certain
+threshold will be ignored.
+
+
+
+
+
Args
+
+
+
+`spec`
+
+
+instance of `audio_spec.BaseSpec`.
+
+
+
+`data_path`
+
+
+string, location to the audio files.
+
+
+
+`categories`
+
+
+A string list of selected categories. If empty, all categories
+will be selected.
+
+
+
+`shuffle`
+
+
+boolean, if True, random shuffle data.
+
+
+
+`cache`
+
+
+str or boolean. When set to True, intermediate results will be
+cached in ram. When set to a file path in string, intermediate results
+will be cached in this file. Please note that, once file based cache is
+created, changes to the input data will have no effects until the cache
+file is removed or the filename is changed. More details can be found at
+https://www.tensorflow.org/api_docs/python/tf/data/Dataset#cache
+
+
+
+
+
+
+
+
+
+
Returns
+
+
+`AudioDataLoader` containing audio spectrogram (or any data type generated
+by `spec.preprocess_ds`) and labels.
+
+
+Generate a shared and batched tf.data.Dataset for training/evaluation.
+
+
+
+
+
+
Args
+
+
+
+`batch_size`
+
+
+A integer, the returned dataset will be batched by this size.
+
+
+
+`is_training`
+
+
+A boolean, when True, the returned dataset will be optionally
+shuffled. Data augmentation, if exists, will also be applied to the
+returned dataset.
+
+
+
+`shuffle`
+
+
+A boolean, when True, the returned dataset will be shuffled to
+create randomness during model training. Only applies when `is_training`
+is set to True.
+
+
+
+`input_pipeline_context`
+
+
+A InputContext instance, used to shared dataset
+among multiple workers when distribution strategy is used.
+
+
+
+`preprocess`
+
+
+Not in use.
+
+
+
+`drop_remainder`
+
+
+boolean, whether the finaly batch drops remainder.
+
+
+
+
+
+
+
+
+
+
Returns
+
+
+A TF dataset ready to be consumed by Keras model.
+
+
+Returns the number of audio files in the DataLoader.
+
+Note that one audio file could be framed (mostly via a sliding window of
+fixed size) into None or multiple audio clips during training and
+evaluation.
diff --git a/site/en/lite/api_docs/python/tflite_model_maker/audio_classifier/YamNetSpec.md b/site/en/lite/api_docs/python/tflite_model_maker/audio_classifier/YamNetSpec.md
new file mode 100644
index 00000000000..61e4e699b48
--- /dev/null
+++ b/site/en/lite/api_docs/python/tflite_model_maker/audio_classifier/YamNetSpec.md
@@ -0,0 +1,317 @@
+page_type: reference
+description: Model good at detecting environmental sounds, using YAMNet embedding.
+
+
+
+
+
+
+
+The location to save the model checkpoint files.
+
+
+
+`strategy`
+
+
+An instance of TF distribute strategy. If none, it will use the
+default strategy (either SingleDeviceStrategy or the current scoped
+strategy.
+
+
+
+`yamnet_model_handle`
+
+
+Path of the TFHub model for retrining.
+
+
+
+`frame_length`
+
+
+The number of samples in each audio frame. If the audio file
+is shorter than `frame_length`, then the audio file will be ignored.
+
+
+
+`frame_step`
+
+
+The number of samples between two audio frames. This value
+should be smaller than `frame_length`, otherwise some samples will be
+ignored.
+
+
+
+`keep_yamnet_and_custom_heads`
+
+
+Boolean, decides if the final TFLite model
+contains both YAMNet and custom trained classification heads. When set
+to False, only the trained custom head will be preserved.
+
+
+Converts the retrained model to tflite format and saves it.
+
+This method overrides the default `CustomModel._export_tflite` method, and
+include the spectrom extraction in the model.
+
+The exported model has input shape (1, number of wav samples)
+
+
+
+
+
Args
+
+
+
+`model`
+
+
+An instance of the keras classification model to be exported.
+
+
+
+`tflite_filepath`
+
+
+File path to save tflite model.
+
+
+
+`with_metadata`
+
+
+Whether the output tflite model contains metadata.
+
+
+
+`export_metadata_json_file`
+
+
+Whether to export metadata in json file. If
+True, export the metadata in the same directory as tflite model. Used
+only if `with_metadata` is True.
+
+
+
+`index_to_label`
+
+
+A list that map from index to label class name.
+
+A instance of audio_dataloader.DataLoader class.
+
+
+
+`model_spec`
+
+
+Specification for the model.
+
+
+
+`validation_data`
+
+
+Validation DataLoader. If None, skips validation process.
+
+
+
+`batch_size`
+
+
+Number of samples per training step. If `use_hub_library` is
+False, it represents the base learning rate when train batch size is 256
+and it's linear to the batch size.
+
+
+
+`epochs`
+
+
+Number of epochs for training.
+
+
+
+`model_dir`
+
+
+The location of the model checkpoint files.
+
+
+
+`do_train`
+
+
+Whether to run training.
+
+
+
+`train_whole_model`
+
+
+Boolean. By default, only the classification head is
+trained. When True, the base model is also trained.
+
+
+
+
+
+
+
+
+
+
Returns
+
+
+An instance based on AudioClassifier.
+
+
+
+
diff --git a/site/en/lite/api_docs/python/tflite_model_maker/config.md b/site/en/lite/api_docs/python/tflite_model_maker/config.md
new file mode 100644
index 00000000000..a6edc8226fc
--- /dev/null
+++ b/site/en/lite/api_docs/python/tflite_model_maker/config.md
@@ -0,0 +1,37 @@
+page_type: reference
+description: APIs for the config of TFLite Model Maker.
+
+
+
+
+
+
+
+A list of optimizations to apply when converting the model.
+If not set, use `[Optimize.DEFAULT]` by default.
+
+
+
+`representative_data`
+
+
+A DataLoader holding representative data for
+post-training quantization.
+
+
+
+`quantization_steps`
+
+
+Number of post-training quantization calibration steps
+to run.
+
+
+
+`inference_input_type`
+
+
+Target data type of real-number input arrays. Allows
+for a different type for input arrays. Defaults to None. If set, must be
+be `{tf.float32, tf.uint8, tf.int8}`.
+
+
+
+`inference_output_type`
+
+
+Target data type of real-number output arrays.
+Allows for a different type for output arrays. Defaults to None. If set,
+must be `{tf.float32, tf.uint8, tf.int8}`.
+
+
+
+`supported_ops`
+
+
+Set of OpsSet options supported by the device. Used to Set
+converter.target_spec.supported_ops.
+
+
+
+`supported_types`
+
+
+List of types for constant values on the target device.
+Supported values are types exported by lite.constants. Frequently, an
+optimization choice is driven by the most compact (i.e. smallest) type
+in this list (default [constants.FLOAT]).
+
+
+
+
+APIs to train an image classification model.
+
+
+
+#### Task guide:
+
+
+https://www.tensorflow.org/lite/tutorials/model_maker_image_classification
+
+## Classes
+
+[`class DataLoader`](../tflite_model_maker/image_classifier/DataLoader): DataLoader for image classifier.
+
+[`class ImageClassifier`](../tflite_model_maker/image_classifier/ImageClassifier): ImageClassifier class for inference and exporting to tflite.
+
+[`class ModelSpec`](../tflite_model_maker/image_classifier/ModelSpec): A specification of image model.
+
+## Functions
+
+[`EfficientNetLite0Spec(...)`](../tflite_model_maker/image_classifier/EfficientNetLite0Spec): Creates EfficientNet-Lite0 model spec. See also: tflite_model_maker.image_classifier.ModelSpec.
+
+[`EfficientNetLite1Spec(...)`](../tflite_model_maker/image_classifier/EfficientNetLite1Spec): Creates EfficientNet-Lite1 model spec. See also: tflite_model_maker.image_classifier.ModelSpec.
+
+[`EfficientNetLite2Spec(...)`](../tflite_model_maker/image_classifier/EfficientNetLite2Spec): Creates EfficientNet-Lite2 model spec. See also: tflite_model_maker.image_classifier.ModelSpec.
+
+[`EfficientNetLite3Spec(...)`](../tflite_model_maker/image_classifier/EfficientNetLite3Spec): Creates EfficientNet-Lite3 model spec. See also: tflite_model_maker.image_classifier.ModelSpec.
+
+[`EfficientNetLite4Spec(...)`](../tflite_model_maker/image_classifier/EfficientNetLite4Spec): Creates EfficientNet-Lite4 model spec. See also: tflite_model_maker.image_classifier.ModelSpec.
+
+[`MobileNetV2Spec(...)`](../tflite_model_maker/image_classifier/MobileNetV2Spec): Creates MobileNet v2 model spec. See also: tflite_model_maker.image_classifier.ModelSpec.
+
+[`Resnet50Spec(...)`](../tflite_model_maker/image_classifier/Resnet50Spec): Creates ResNet 50 model spec. See also: tflite_model_maker.image_classifier.ModelSpec.
+
+[`create(...)`](../tflite_model_maker/image_classifier/create): Loads data and retrains the model based on data for image classification.
diff --git a/site/en/lite/api_docs/python/tflite_model_maker/image_classifier/DataLoader.md b/site/en/lite/api_docs/python/tflite_model_maker/image_classifier/DataLoader.md
new file mode 100644
index 00000000000..9d0fdf9a722
--- /dev/null
+++ b/site/en/lite/api_docs/python/tflite_model_maker/image_classifier/DataLoader.md
@@ -0,0 +1,338 @@
+page_type: reference
+description: DataLoader for image classifier.
+
+
+
+
+
+
+
+A tf.data.Dataset object that contains a potentially large set of
+elements, where each element is a pair of (input_data, target). The
+`input_data` means the raw input data, like an image, a text etc., while
+the `target` means some ground truth of the raw input data, such as the
+classification label of the image etc.
+
+
+
+`size`
+
+
+The size of the dataset. tf.data.Dataset donesn't support a function
+to get the length directly since it's lazy-loaded and may be infinite.
+
+
+
+
+
+
+
+
+
+
+
+
Attributes
+
+
+
+`num_classes`
+
+
+
+
+
+
+`size`
+
+
+Returns the size of the dataset.
+
+Note that this function may return None becuase the exact size of the
+dataset isn't a necessary parameter to create an instance of this class,
+and tf.data.Dataset donesn't support a function to get the length directly
+since it's lazy-loaded and may be infinite.
+In most cases, however, when an instance of this class is created by helper
+functions like 'from_folder', the size of the dataset will be preprocessed,
+and this function can return an int representing the size of the dataset.
+
+A list that map from index to label class name.
+
+
+
+`shuffle`
+
+
+Whether the data should be shuffled.
+
+
+
+`hparams`
+
+
+A namedtuple of hyperparameters. This function expects
+.dropout_rate: The fraction of the input units to drop, used in dropout
+ layer.
+.do_fine_tuning: If true, the Hub module is trained together with the
+ classification layer on top.
+
+
+
+`use_augmentation`
+
+
+Use data augmentation for preprocessing.
+
+
+
+`representative_data`
+
+
+ Representative dataset for full integer
+quantization. Used when converting the keras model to the TFLite model
+with full integer quantization.
+
+
+Loads data and retrains the model based on data for image classification.
+
+
+
+
+
+
Args
+
+
+
+`train_data`
+
+
+Training data.
+
+
+
+`model_spec`
+
+
+Specification for the model.
+
+
+
+`validation_data`
+
+
+Validation data. If None, skips validation process.
+
+
+
+`batch_size`
+
+
+Number of samples per training step. If `use_hub_library` is
+False, it represents the base learning rate when train batch size is 256
+and it's linear to the batch size.
+
+
+
+`epochs`
+
+
+Number of epochs for training.
+
+
+
+`steps_per_epoch`
+
+
+Integer or None. Total number of steps (batches of
+samples) before declaring one epoch finished and starting the next
+epoch. If `steps_per_epoch` is None, the epoch will run until the input
+dataset is exhausted.
+
+
+
+`train_whole_model`
+
+
+If true, the Hub module is trained together with the
+classification layer on top. Otherwise, only train the top
+classification layer.
+
+
+
+`dropout_rate`
+
+
+The rate for dropout.
+
+
+
+`learning_rate`
+
+
+Base learning rate when train batch size is 256. Linear to
+the batch size.
+
+
+
+`momentum`
+
+
+a Python float forwarded to the optimizer. Only used when
+`use_hub_library` is True.
+
+
+
+`shuffle`
+
+
+Whether the data should be shuffled.
+
+
+
+`use_augmentation`
+
+
+Use data augmentation for preprocessing.
+
+
+
+`use_hub_library`
+
+
+Use `make_image_classifier_lib` from tensorflow hub to
+retrain the model.
+
+
+
+`warmup_steps`
+
+
+Number of warmup steps for warmup schedule on learning rate.
+If None, the default warmup_steps is used which is the total training
+steps in two epochs. Only used when `use_hub_library` is False.
+
+
+
+`model_dir`
+
+
+The location of the model checkpoint files. Only used when
+`use_hub_library` is False.
+
+Validation data. If None, skips validation process.
+
+
+
+`hparams`
+
+
+An instance of hub_lib.HParams or
+train_image_classifier_lib.HParams. Anamedtuple of hyperparameters.
+
+
+
+`steps_per_epoch`
+
+
+Integer or None. Total number of steps (batches of
+samples) before declaring one epoch finished and starting the next
+epoch. If 'steps_per_epoch' is None, the epoch will run until the input
+dataset is exhausted.
+
+
+
+
+
+
+
+
+
+
Returns
+
+
+The tf.keras.callbacks.History object returned by tf.keras.Model.fit*().
+
+
+
+
+
+
+
+
+
+
+
+
+
+
+
Class Variables
+
+
+
+ALLOWED_EXPORT_FORMAT
+
+
+`(,
+ ,
+ ,
+ )`
+
+
+
+DEFAULT_EXPORT_FORMAT
+
+
+`(, )`
+
+
+
diff --git a/site/en/lite/api_docs/python/tflite_model_maker/image_classifier/MobileNetV2Spec.md b/site/en/lite/api_docs/python/tflite_model_maker/image_classifier/MobileNetV2Spec.md
new file mode 100644
index 00000000000..cecedecaf73
--- /dev/null
+++ b/site/en/lite/api_docs/python/tflite_model_maker/image_classifier/MobileNetV2Spec.md
@@ -0,0 +1,76 @@
+page_type: reference
+description: Creates MobileNet v2 model spec. See also: tflite_model_maker.image_classifier.ModelSpec.
+
+
+
+
+
+
+
+list of int, input image shape. Default: [224, 224].
+
+
+
+`name`
+
+
+str, model spec name.
+
+
+
diff --git a/site/en/lite/api_docs/python/tflite_model_maker/image_classifier/create.md b/site/en/lite/api_docs/python/tflite_model_maker/image_classifier/create.md
new file mode 100644
index 00000000000..b4440fc9f56
--- /dev/null
+++ b/site/en/lite/api_docs/python/tflite_model_maker/image_classifier/create.md
@@ -0,0 +1,223 @@
+page_type: reference
+description: Loads data and retrains the model based on data for image classification.
+
+
+
+
+
+
+
+Validation data. If None, skips validation process.
+
+
+
+`batch_size`
+
+
+Number of samples per training step. If `use_hub_library` is
+False, it represents the base learning rate when train batch size is 256
+and it's linear to the batch size.
+
+
+
+`epochs`
+
+
+Number of epochs for training.
+
+
+
+`steps_per_epoch`
+
+
+Integer or None. Total number of steps (batches of
+samples) before declaring one epoch finished and starting the next
+epoch. If `steps_per_epoch` is None, the epoch will run until the input
+dataset is exhausted.
+
+
+
+`train_whole_model`
+
+
+If true, the Hub module is trained together with the
+classification layer on top. Otherwise, only train the top
+classification layer.
+
+
+
+`dropout_rate`
+
+
+The rate for dropout.
+
+
+
+`learning_rate`
+
+
+Base learning rate when train batch size is 256. Linear to
+the batch size.
+
+
+
+`momentum`
+
+
+a Python float forwarded to the optimizer. Only used when
+`use_hub_library` is True.
+
+
+
+`shuffle`
+
+
+Whether the data should be shuffled.
+
+
+
+`use_augmentation`
+
+
+Use data augmentation for preprocessing.
+
+
+
+`use_hub_library`
+
+
+Use `make_image_classifier_lib` from tensorflow hub to
+retrain the model.
+
+
+
+`warmup_steps`
+
+
+Number of warmup steps for warmup schedule on learning rate.
+If None, the default warmup_steps is used which is the total training
+steps in two epochs. Only used when `use_hub_library` is False.
+
+
+
+`model_dir`
+
+
+The location of the model checkpoint files. Only used when
+`use_hub_library` is False.
+
+
+
+`do_train`
+
+
+Whether to run training.
+
+
+
+
+
+
+
+
+
+
Returns
+
+
+An instance based on ImageClassifier.
+
+
+
+
diff --git a/site/en/lite/api_docs/python/tflite_model_maker/model_spec.md b/site/en/lite/api_docs/python/tflite_model_maker/model_spec.md
new file mode 100644
index 00000000000..c1a46677d2a
--- /dev/null
+++ b/site/en/lite/api_docs/python/tflite_model_maker/model_spec.md
@@ -0,0 +1,103 @@
+page_type: reference
+description: APIs for the model spec of TFLite Model Maker.
+
+
+
+
+
+
+
+
+
+
+APIs for the model spec of TFLite Model Maker.
+
+
+
+## Functions
+
+[`get(...)`](../tflite_model_maker/model_spec/get): Gets model spec by name or instance, and init with args and kwarges.
+
+
+
+
+
diff --git a/site/en/lite/api_docs/python/tflite_model_maker/model_spec/get.md b/site/en/lite/api_docs/python/tflite_model_maker/model_spec/get.md
new file mode 100644
index 00000000000..a517d63b253
--- /dev/null
+++ b/site/en/lite/api_docs/python/tflite_model_maker/model_spec/get.md
@@ -0,0 +1,61 @@
+page_type: reference
+description: Gets model spec by name or instance, and init with args and kwarges.
+
+
+
+
+
+
+
+
+
+
+APIs to train an object detection model.
+
+
+
+## Classes
+
+[`class DataLoader`](../tflite_model_maker/object_detector/DataLoader): DataLoader for object detector.
+
+[`class EfficientDetSpec`](../tflite_model_maker/object_detector/EfficientDetSpec): A specification of the EfficientDet model.
+
+[`class ObjectDetector`](../tflite_model_maker/object_detector/ObjectDetector): ObjectDetector class for inference and exporting to tflite.
+
+## Functions
+
+[`EfficientDetLite0Spec(...)`](../tflite_model_maker/object_detector/EfficientDetLite0Spec): Creates EfficientDet-Lite0 model spec. See also: tflite_model_maker.object_detector.EfficientDetSpec.
+
+[`EfficientDetLite1Spec(...)`](../tflite_model_maker/object_detector/EfficientDetLite1Spec): Creates EfficientDet-Lite1 model spec. See also: tflite_model_maker.object_detector.EfficientDetSpec.
+
+[`EfficientDetLite2Spec(...)`](../tflite_model_maker/object_detector/EfficientDetLite2Spec): Creates EfficientDet-Lite2 model spec. See also: tflite_model_maker.object_detector.EfficientDetSpec.
+
+[`EfficientDetLite3Spec(...)`](../tflite_model_maker/object_detector/EfficientDetLite3Spec): Creates EfficientDet-Lite3 model spec. See also: tflite_model_maker.object_detector.EfficientDetSpec.
+
+[`EfficientDetLite4Spec(...)`](../tflite_model_maker/object_detector/EfficientDetLite4Spec): Creates EfficientDet-Lite4 model spec. See also: tflite_model_maker.object_detector.EfficientDetSpec.
+
+[`create(...)`](../tflite_model_maker/object_detector/create): Loads data and train the model for object detection.
diff --git a/site/en/lite/api_docs/python/tflite_model_maker/object_detector/DataLoader.md b/site/en/lite/api_docs/python/tflite_model_maker/object_detector/DataLoader.md
new file mode 100644
index 00000000000..0c20e2c3f74
--- /dev/null
+++ b/site/en/lite/api_docs/python/tflite_model_maker/object_detector/DataLoader.md
@@ -0,0 +1,527 @@
+page_type: reference
+description: DataLoader for object detector.
+
+
+
+
+
+
+
+Glob for tfrecord files. e.g. "/tmp/coco*.tfrecord".
+
+
+
+`size`
+
+
+The size of the dataset.
+
+
+
+`label_map`
+
+
+Variable shows mapping label integers ids to string label
+names. 0 is the reserved key for `background` and doesn't need to be
+included in label_map. Label names can't be duplicated. Supported
+formats are:
+
+1. Dict, map label integers ids to string label names, such as {1:
+ 'person', 2: 'notperson'}. 2. List, a list of label names such as
+ ['person', 'notperson'] which is
+ the same as setting label_map={1: 'person', 2: 'notperson'}.
+3. String, name for certain dataset. Accepted values are: 'coco', 'voc'
+ and 'waymo'. 4. String, yaml filename that stores label_map.
+
+
+
+`annotations_json_file`
+
+
+JSON with COCO data format containing golden
+bounding boxes. Used for validation. If None, use the ground truth from
+the dataloader. Refer to
+https://towardsdatascience.com/coco-data-format-for-object-detection-a4c5eaf518c5
+ for the description of COCO data format.
+
+
+
+
+
+
+
+
+
+
+
+
Attributes
+
+
+
+`size`
+
+
+Returns the size of the dataset.
+
+Note that this function may return None becuase the exact size of the
+dataset isn't a necessary parameter to create an instance of this class,
+and tf.data.Dataset donesn't support a function to get the length directly
+since it's lazy-loaded and may be infinite.
+In most cases, however, when an instance of this class is created by helper
+functions like 'from_folder', the size of the dataset will be preprocessed,
+and this function can return an int representing the size of the dataset.
+
+Path to directory that store raw images. If None, the image
+path in the csv file is the path to Google Cloud Storage or the absolute
+path in the local machine.
+
+
+
+`delimiter`
+
+
+Character used to separate fields.
+
+
+
+`quotechar`
+
+
+Character used to quote fields containing special characters.
+
+
+
+`num_shards`
+
+
+Number of shards for output file.
+
+
+
+`max_num_images`
+
+
+Max number of imags to process.
+
+
+
+`cache_dir`
+
+
+The cache directory to save TFRecord, metadata and json file.
+When cache_dir is None, a temporary folder will be created and will not
+be removed automatically after training which makes it can be used
+later.
+
+
+
+`cache_prefix_filename`
+
+
+The cache prefix filename. If None, will
+automatically generate it based on `filename`.
+
+
+
+
+
+
+
+
+
+
Returns
+
+
+train_data, validation_data, test_data which are ObjectDetectorDataLoader
+objects. Can be None if without such data.
+
+Variable shows mapping label integers ids to string label
+names. 0 is the reserved key for `background`. Label names can't be
+duplicated. Supported format: 1. Dict, map label integers ids to string
+ label names, e.g.
+ {1: 'person', 2: 'notperson'}. 2. List, a list of label names. e.g.
+ ['person', 'notperson'] which is
+ the same as setting label_map={1: 'person', 2: 'notperson'}.
+
+3. String, name for certain dataset. Accepted values are: 'coco', 'voc'
+ and 'waymo'. 4. String, yaml filename that stores label_map.
+
+
+
+`annotation_filenames`
+
+
+Collection of annotation filenames (strings) to be
+loaded. For instance, if there're 3 annotation files [0.xml, 1.xml,
+2.xml] in `annotations_dir`, setting annotation_filenames=['0', '1']
+makes this method only load [0.xml, 1.xml].
+
+
+
+`ignore_difficult_instances`
+
+
+Whether to ignore difficult instances.
+`difficult` can be set inside `object` item in the annotation xml file.
+
+
+
+`num_shards`
+
+
+Number of shards for output file.
+
+
+
+`max_num_images`
+
+
+Max number of imags to process.
+
+
+
+`cache_dir`
+
+
+The cache directory to save TFRecord, metadata and json file.
+When cache_dir is not set, a temporary folder will be created and will
+not be removed automatically after training which makes it can be used
+later.
+
+
+
+`cache_prefix_filename`
+
+
+The cache prefix filename. If not set, will
+automatically generate it based on `image_dir`, `annotations_dir` and
+`annotation_filenames`.
+
+Hyperparameters used to overwrite default configuration. Can be
+
+1) Dict, contains parameter names and values; 2) String, Comma separated
+k=v pairs of hyperparameters; 3) String, yaml filename which's a module
+containing attributes to use as hyperparameters.
+
+
+
+`model_dir`
+
+
+The location to save the model checkpoint files.
+
+
+
+`epochs`
+
+
+Default training epochs.
+
+
+
+`batch_size`
+
+
+Training & Evaluation batch size.
+
+
+
+`steps_per_execution`
+
+
+Number of steps per training execution.
+
+
+
+`moving_average_decay`
+
+
+Float. The decay to use for maintaining moving
+averages of the trained parameters.
+
+
+
+`var_freeze_expr`
+
+
+Expression to freeze variables.
+
+
+
+`tflite_max_detections`
+
+
+The max number of output detections in the TFLite
+model.
+
+
+
+`strategy`
+
+
+ A string specifying which distribution strategy to use.
+Accepted values are 'tpu', 'gpus', None. tpu' means to use TPUStrategy.
+'gpus' mean to use MirroredStrategy for multi-gpus. If None, use TF
+default with OneDeviceStrategy.
+
+
+
+`tpu`
+
+
+The Cloud TPU to use for training. This should be either the name
+used when creating the Cloud TPU, or a grpc://ip.address.of.tpu:8470
+ url.
+
+
+
+`gcp_project`
+
+
+Project name for the Cloud TPU-enabled project. If not
+specified, we will attempt to automatically detect the GCE project from
+metadata.
+
+
+
+`tpu_zone`
+
+
+GCE zone where the Cloud TPU is located in. If not specified, we
+will attempt to automatically detect the GCE project from metadata.
+
+
+
+`use_xla`
+
+
+Use XLA even if strategy is not tpu. If strategy is tpu, always
+use XLA, and this flag has no effect.
+
+
+
+`profile`
+
+
+Enable profile mode.
+
+
+
+`debug`
+
+
+Enable debug mode.
+
+
+
+`tf_random_seed`
+
+
+Fixed random seed for deterministic execution across runs
+for debugging.
+
+Hyperparameters used to overwrite default configuration. Can be
+
+1) Dict, contains parameter names and values; 2) String, Comma separated
+k=v pairs of hyperparameters; 3) String, yaml filename which's a module
+containing attributes to use as hyperparameters.
+
+
+
+`model_dir`
+
+
+The location to save the model checkpoint files.
+
+
+
+`epochs`
+
+
+Default training epochs.
+
+
+
+`batch_size`
+
+
+Training & Evaluation batch size.
+
+
+
+`steps_per_execution`
+
+
+Number of steps per training execution.
+
+
+
+`moving_average_decay`
+
+
+Float. The decay to use for maintaining moving
+averages of the trained parameters.
+
+
+
+`var_freeze_expr`
+
+
+Expression to freeze variables.
+
+
+
+`tflite_max_detections`
+
+
+The max number of output detections in the TFLite
+model.
+
+
+
+`strategy`
+
+
+ A string specifying which distribution strategy to use.
+Accepted values are 'tpu', 'gpus', None. tpu' means to use TPUStrategy.
+'gpus' mean to use MirroredStrategy for multi-gpus. If None, use TF
+default with OneDeviceStrategy.
+
+
+
+`tpu`
+
+
+The Cloud TPU to use for training. This should be either the name
+used when creating the Cloud TPU, or a grpc://ip.address.of.tpu:8470
+ url.
+
+
+
+`gcp_project`
+
+
+Project name for the Cloud TPU-enabled project. If not
+specified, we will attempt to automatically detect the GCE project from
+metadata.
+
+
+
+`tpu_zone`
+
+
+GCE zone where the Cloud TPU is located in. If not specified, we
+will attempt to automatically detect the GCE project from metadata.
+
+
+
+`use_xla`
+
+
+Use XLA even if strategy is not tpu. If strategy is tpu, always
+use XLA, and this flag has no effect.
+
+
+
+`profile`
+
+
+Enable profile mode.
+
+
+
+`debug`
+
+
+Enable debug mode.
+
+
+
+`tf_random_seed`
+
+
+Fixed random seed for deterministic execution across runs
+for debugging.
+
+Hyperparameters used to overwrite default configuration. Can be
+
+1) Dict, contains parameter names and values; 2) String, Comma separated
+k=v pairs of hyperparameters; 3) String, yaml filename which's a module
+containing attributes to use as hyperparameters.
+
+
+
+`model_dir`
+
+
+The location to save the model checkpoint files.
+
+
+
+`epochs`
+
+
+Default training epochs.
+
+
+
+`batch_size`
+
+
+Training & Evaluation batch size.
+
+
+
+`steps_per_execution`
+
+
+Number of steps per training execution.
+
+
+
+`moving_average_decay`
+
+
+Float. The decay to use for maintaining moving
+averages of the trained parameters.
+
+
+
+`var_freeze_expr`
+
+
+Expression to freeze variables.
+
+
+
+`tflite_max_detections`
+
+
+The max number of output detections in the TFLite
+model.
+
+
+
+`strategy`
+
+
+ A string specifying which distribution strategy to use.
+Accepted values are 'tpu', 'gpus', None. tpu' means to use TPUStrategy.
+'gpus' mean to use MirroredStrategy for multi-gpus. If None, use TF
+default with OneDeviceStrategy.
+
+
+
+`tpu`
+
+
+The Cloud TPU to use for training. This should be either the name
+used when creating the Cloud TPU, or a grpc://ip.address.of.tpu:8470
+ url.
+
+
+
+`gcp_project`
+
+
+Project name for the Cloud TPU-enabled project. If not
+specified, we will attempt to automatically detect the GCE project from
+metadata.
+
+
+
+`tpu_zone`
+
+
+GCE zone where the Cloud TPU is located in. If not specified, we
+will attempt to automatically detect the GCE project from metadata.
+
+
+
+`use_xla`
+
+
+Use XLA even if strategy is not tpu. If strategy is tpu, always
+use XLA, and this flag has no effect.
+
+
+
+`profile`
+
+
+Enable profile mode.
+
+
+
+`debug`
+
+
+Enable debug mode.
+
+
+
+`tf_random_seed`
+
+
+Fixed random seed for deterministic execution across runs
+for debugging.
+
+Hyperparameters used to overwrite default configuration. Can be
+
+1) Dict, contains parameter names and values; 2) String, Comma separated
+k=v pairs of hyperparameters; 3) String, yaml filename which's a module
+containing attributes to use as hyperparameters.
+
+
+
+`model_dir`
+
+
+The location to save the model checkpoint files.
+
+
+
+`epochs`
+
+
+Default training epochs.
+
+
+
+`batch_size`
+
+
+Training & Evaluation batch size.
+
+
+
+`steps_per_execution`
+
+
+Number of steps per training execution.
+
+
+
+`moving_average_decay`
+
+
+Float. The decay to use for maintaining moving
+averages of the trained parameters.
+
+
+
+`var_freeze_expr`
+
+
+Expression to freeze variables.
+
+
+
+`tflite_max_detections`
+
+
+The max number of output detections in the TFLite
+model.
+
+
+
+`strategy`
+
+
+ A string specifying which distribution strategy to use.
+Accepted values are 'tpu', 'gpus', None. tpu' means to use TPUStrategy.
+'gpus' mean to use MirroredStrategy for multi-gpus. If None, use TF
+default with OneDeviceStrategy.
+
+
+
+`tpu`
+
+
+The Cloud TPU to use for training. This should be either the name
+used when creating the Cloud TPU, or a grpc://ip.address.of.tpu:8470
+ url.
+
+
+
+`gcp_project`
+
+
+Project name for the Cloud TPU-enabled project. If not
+specified, we will attempt to automatically detect the GCE project from
+metadata.
+
+
+
+`tpu_zone`
+
+
+GCE zone where the Cloud TPU is located in. If not specified, we
+will attempt to automatically detect the GCE project from metadata.
+
+
+
+`use_xla`
+
+
+Use XLA even if strategy is not tpu. If strategy is tpu, always
+use XLA, and this flag has no effect.
+
+
+
+`profile`
+
+
+Enable profile mode.
+
+
+
+`debug`
+
+
+Enable debug mode.
+
+
+
+`tf_random_seed`
+
+
+Fixed random seed for deterministic execution across runs
+for debugging.
+
+Hyperparameters used to overwrite default configuration. Can be
+
+1) Dict, contains parameter names and values; 2) String, Comma separated
+k=v pairs of hyperparameters; 3) String, yaml filename which's a module
+containing attributes to use as hyperparameters.
+
+
+
+`model_dir`
+
+
+The location to save the model checkpoint files.
+
+
+
+`epochs`
+
+
+Default training epochs.
+
+
+
+`batch_size`
+
+
+Training & Evaluation batch size.
+
+
+
+`steps_per_execution`
+
+
+Number of steps per training execution.
+
+
+
+`moving_average_decay`
+
+
+Float. The decay to use for maintaining moving
+averages of the trained parameters.
+
+
+
+`var_freeze_expr`
+
+
+Expression to freeze variables.
+
+
+
+`tflite_max_detections`
+
+
+The max number of output detections in the TFLite
+model.
+
+
+
+`strategy`
+
+
+ A string specifying which distribution strategy to use.
+Accepted values are 'tpu', 'gpus', None. tpu' means to use TPUStrategy.
+'gpus' mean to use MirroredStrategy for multi-gpus. If None, use TF
+default with OneDeviceStrategy.
+
+
+
+`tpu`
+
+
+The Cloud TPU to use for training. This should be either the name
+used when creating the Cloud TPU, or a grpc://ip.address.of.tpu:8470
+ url.
+
+
+
+`gcp_project`
+
+
+Project name for the Cloud TPU-enabled project. If not
+specified, we will attempt to automatically detect the GCE project from
+metadata.
+
+
+
+`tpu_zone`
+
+
+GCE zone where the Cloud TPU is located in. If not specified, we
+will attempt to automatically detect the GCE project from metadata.
+
+
+
+`use_xla`
+
+
+Use XLA even if strategy is not tpu. If strategy is tpu, always
+use XLA, and this flag has no effect.
+
+
+
+`profile`
+
+
+Enable profile mode.
+
+
+
+`debug`
+
+
+Enable debug mode.
+
+
+
+`tf_random_seed`
+
+
+Fixed random seed for deterministic execution across runs
+for debugging.
+
+Hyperparameters used to overwrite default configuration. Can be
+
+1) Dict, contains parameter names and values; 2) String, Comma separated
+k=v pairs of hyperparameters; 3) String, yaml filename which's a module
+containing attributes to use as hyperparameters.
+
+
+
+`model_dir`
+
+
+The location to save the model checkpoint files.
+
+
+
+`epochs`
+
+
+Default training epochs.
+
+
+
+`batch_size`
+
+
+Training & Evaluation batch size.
+
+
+
+`steps_per_execution`
+
+
+Number of steps per training execution.
+
+
+
+`moving_average_decay`
+
+
+Float. The decay to use for maintaining moving
+averages of the trained parameters.
+
+
+
+`var_freeze_expr`
+
+
+Expression to freeze variables.
+
+
+
+`tflite_max_detections`
+
+
+The max number of output detections in the TFLite
+model.
+
+
+
+`strategy`
+
+
+ A string specifying which distribution strategy to use.
+Accepted values are 'tpu', 'gpus', None. tpu' means to use TPUStrategy.
+'gpus' mean to use MirroredStrategy for multi-gpus. If None, use TF
+default with OneDeviceStrategy.
+
+
+
+`tpu`
+
+
+The Cloud TPU to use for training. This should be either the name
+used when creating the Cloud TPU, or a grpc://ip.address.of.tpu:8470
+ url.
+
+
+
+`gcp_project`
+
+
+Project name for the Cloud TPU-enabled project. If not
+specified, we will attempt to automatically detect the GCE project from
+metadata.
+
+
+
+`tpu_zone`
+
+
+GCE zone where the Cloud TPU is located in. If not specified, we
+will attempt to automatically detect the GCE project from metadata.
+
+
+
+`use_xla`
+
+
+Use XLA even if strategy is not tpu. If strategy is tpu, always
+use XLA, and this flag has no effect.
+
+
+
+`profile`
+
+
+Enable profile mode.
+
+
+
+`debug`
+
+
+Enable debug mode.
+
+
+
+`tf_random_seed`
+
+
+Fixed random seed for deterministic execution across runs
+for debugging.
+
+
+Converts the retrained model to tflite format and saves it.
+
+The exported TFLite model has the following inputs & outputs:
+One input:
+ image: a float32 tensor of shape[1, height, width, 3] containing the
+ normalized input image. `self.config.image_size` is [height, width].
+
+
+
+
+
Four Outputs
+
+
+
+`detection_boxes`
+
+
+a float32 tensor of shape [1, num_boxes, 4] with box
+locations.
+
+
+
+`detection_classes`
+
+
+a float32 tensor of shape [1, num_boxes] with class
+indices.
+
+
+
+`detection_scores`
+
+
+a float32 tensor of shape [1, num_boxes] with class
+scores.
+
+
+
+`num_boxes`
+
+
+a float32 tensor of size 1 containing the number of detected
+boxes.
+
+
+
+
+
+
+
+
+
+
Args
+
+
+
+`model`
+
+
+The EfficientDetNet model used for training which doesn't have pre
+and post processing.
+
+ Dict, map label integer ids to string label names such as {1:
+'person', 2: 'notperson'}. 0 is the reserved key for `background` and
+ doesn't need to be included in `label_map`. Label names can't be
+ duplicated.
+
+
+
+`representative_data`
+
+
+ Representative dataset for full integer
+quantization. Used when converting the keras model to the TFLite model
+with full interger quantization.
+
+
+Loads data and train the model for object detection.
+
+
+
+
+
+
Args
+
+
+
+`train_data`
+
+
+Training data.
+
+
+
+`model_spec`
+
+
+Specification for the model.
+
+
+
+`validation_data`
+
+
+Validation data. If None, skips validation process.
+
+
+
+`epochs`
+
+
+Number of epochs for training.
+
+
+
+`batch_size`
+
+
+Batch size for training.
+
+
+
+`train_whole_model`
+
+
+Boolean, False by default. If true, train the whole
+model. Otherwise, only train the layers that are not match
+`model_spec.config.var_freeze_expr`.
+
+Validation data. If None, skips validation process.
+
+
+
+`epochs`
+
+
+Number of epochs for training.
+
+
+
+`batch_size`
+
+
+Batch size for training.
+
+
+
+`train_whole_model`
+
+
+Boolean, False by default. If true, train the whole
+model. Otherwise, only train the layers that are not match
+`model_spec.config.var_freeze_expr`.
+
+
+
+`do_train`
+
+
+Whether to run training.
+
+
+
+
+
+
+
+
+
+
Returns
+
+
+An instance based on ObjectDetector.
+
+
+
+
diff --git a/site/en/lite/api_docs/python/tflite_model_maker/question_answer.md b/site/en/lite/api_docs/python/tflite_model_maker/question_answer.md
new file mode 100644
index 00000000000..0dbc9caeb69
--- /dev/null
+++ b/site/en/lite/api_docs/python/tflite_model_maker/question_answer.md
@@ -0,0 +1,52 @@
+page_type: reference
+description: APIs to train a model that can answer questions based on a predefined text.
+
+
+
+
+
+
+
+
+
+
+APIs to train a model that can answer questions based on a predefined text.
+
+
+
+#### Task guide:
+
+
+https://www.tensorflow.org/lite/tutorials/model_maker_question_answer
+
+## Classes
+
+[`class BertQaSpec`](../tflite_model_maker/question_answer/BertQaSpec): A specification of BERT model for question answering.
+
+[`class DataLoader`](../tflite_model_maker/question_answer/DataLoader): DataLoader for question answering.
+
+[`class QuestionAnswer`](../tflite_model_maker/question_answer/QuestionAnswer): QuestionAnswer class for inference and exporting to tflite.
+
+## Functions
+
+[`MobileBertQaSpec(...)`](../tflite_model_maker/question_answer/MobileBertQaSpec): Creates MobileBert model spec for the question answer task. See also: tflite_model_maker.question_answer.BertQaSpec.
+
+[`MobileBertQaSquadSpec(...)`](../tflite_model_maker/question_answer/MobileBertQaSquadSpec): Creates MobileBert model spec that's already retrained on SQuAD1.1 for the question answer task. See also: tflite_model_maker.question_answer.BertQaSpec.
+
+[`create(...)`](../tflite_model_maker/question_answer/create): Loads data and train the model for question answer.
diff --git a/site/en/lite/api_docs/python/tflite_model_maker/question_answer/BertQaSpec.md b/site/en/lite/api_docs/python/tflite_model_maker/question_answer/BertQaSpec.md
new file mode 100644
index 00000000000..d19c210628a
--- /dev/null
+++ b/site/en/lite/api_docs/python/tflite_model_maker/question_answer/BertQaSpec.md
@@ -0,0 +1,629 @@
+page_type: reference
+description: A specification of BERT model for question answering.
+
+
+
+
+
+
+
+The stride when we do a sliding window approach to take chunks
+of the documents.
+
+
+
+`dropout_rate`
+
+
+The rate for dropout.
+
+
+
+`initializer_range`
+
+
+The stdev of the truncated_normal_initializer for
+initializing all weight matrices.
+
+
+
+`learning_rate`
+
+
+The initial learning rate for Adam.
+
+
+
+`distribution_strategy`
+
+
+ A string specifying which distribution strategy to
+use. Accepted values are 'off', 'one_device', 'mirrored',
+'parameter_server', 'multi_worker_mirrored', and 'tpu' -- case
+insensitive. 'off' means not to use Distribution Strategy; 'tpu' means
+to use TPUStrategy using `tpu_address`.
+
+
+
+`num_gpus`
+
+
+How many GPUs to use at each worker with the
+DistributionStrategies API. The default is -1, which means utilize all
+available GPUs.
+
+
+
+`tpu`
+
+
+TPU address to connect to.
+
+
+
+`trainable`
+
+
+boolean, whether pretrain layer is trainable.
+
+
+
+`predict_batch_size`
+
+
+Batch size for prediction.
+
+
+
+`do_lower_case`
+
+
+boolean, whether to lower case the input text. Should be
+True for uncased models and False for cased models.
+
+
+
+`is_tf2`
+
+
+boolean, whether the hub module is in TensorFlow 2.x format.
+
+
+
+`tflite_input_name`
+
+
+Dict, input names for the TFLite model.
+
+
+
+`tflite_output_name`
+
+
+Dict, output names for the TFLite model.
+
+
+
+`init_from_squad_model`
+
+
+boolean, whether to initialize from the model that
+is already retrained on Squad 1.1.
+
+tf.data.Dataset, training data to be fed in
+tf.keras.Model.fit().
+
+
+
+`epochs`
+
+
+Integer, training epochs.
+
+
+
+`steps_per_epoch`
+
+
+Integer or None. Total number of steps (batches of
+samples) before declaring one epoch finished and starting the next
+epoch. If `steps_per_epoch` is None, the epoch will run until the input
+dataset is exhausted.
+
+
+
+`**kwargs`
+
+
+Other parameters used in the tf.keras.Model.fit().
+
+
+
+
+
+
+
+
+
+
Returns
+
+
+tf.keras.Model, the keras model that's already trained.
+
+A tf.data.Dataset object that contains a potentially large set of
+elements, where each element is a pair of (input_data, target). The
+`input_data` means the raw input data, like an image, a text etc., while
+the `target` means some ground truth of the raw input data, such as the
+classification label of the image etc.
+
+
+
+`size`
+
+
+The size of the dataset. tf.data.Dataset donesn't support a function
+to get the length directly since it's lazy-loaded and may be infinite.
+
+
+
+
+
+
+
+
+
+
+
+
Attributes
+
+
+
+`size`
+
+
+Returns the size of the dataset.
+
+Note that this function may return None becuase the exact size of the
+dataset isn't a necessary parameter to create an instance of this class,
+and tf.data.Dataset donesn't support a function to get the length directly
+since it's lazy-loaded and may be infinite.
+In most cases, however, when an instance of this class is created by helper
+functions like 'from_folder', the size of the dataset will be preprocessed,
+and this function can return an int representing the size of the dataset.
+
+The stride when we do a sliding window approach to take chunks
+of the documents.
+
+
+
+`dropout_rate`
+
+
+The rate for dropout.
+
+
+
+`initializer_range`
+
+
+The stdev of the truncated_normal_initializer for
+initializing all weight matrices.
+
+
+
+`learning_rate`
+
+
+The initial learning rate for Adam.
+
+
+
+`distribution_strategy`
+
+
+ A string specifying which distribution strategy to
+use. Accepted values are 'off', 'one_device', 'mirrored',
+'parameter_server', 'multi_worker_mirrored', and 'tpu' -- case
+insensitive. 'off' means not to use Distribution Strategy; 'tpu' means
+to use TPUStrategy using `tpu_address`.
+
+
+
+`num_gpus`
+
+
+How many GPUs to use at each worker with the
+DistributionStrategies API. The default is -1, which means utilize all
+available GPUs.
+
+
+
+`tpu`
+
+
+TPU address to connect to.
+
+
+
+`trainable`
+
+
+boolean, whether pretrain layer is trainable.
+
+
+
+`predict_batch_size`
+
+
+Batch size for prediction.
+
+
+
+`do_lower_case`
+
+
+boolean, whether to lower case the input text. Should be
+True for uncased models and False for cased models.
+
+
+
+`is_tf2`
+
+
+boolean, whether the hub module is in TensorFlow 2.x format.
+
+
+
+`tflite_input_name`
+
+
+Dict, input names for the TFLite model.
+
+
+
+`tflite_output_name`
+
+
+Dict, output names for the TFLite model.
+
+
+
+`init_from_squad_model`
+
+
+boolean, whether to initialize from the model that
+is already retrained on Squad 1.1.
+
+
+
+`default_batch_size`
+
+
+Default batch size for training.
+
+
+
+`name`
+
+
+Name of the object.
+
+
+
diff --git a/site/en/lite/api_docs/python/tflite_model_maker/question_answer/MobileBertQaSquadSpec.md b/site/en/lite/api_docs/python/tflite_model_maker/question_answer/MobileBertQaSquadSpec.md
new file mode 100644
index 00000000000..8bacef70a75
--- /dev/null
+++ b/site/en/lite/api_docs/python/tflite_model_maker/question_answer/MobileBertQaSquadSpec.md
@@ -0,0 +1,214 @@
+page_type: reference
+description: Creates MobileBert model spec that's already retrained on SQuAD1.1 for the question answer task. See also: tflite_model_maker.question_answer.BertQaSpec.
+
+
+
+
+
+
+
+The stride when we do a sliding window approach to take chunks
+of the documents.
+
+
+
+`dropout_rate`
+
+
+The rate for dropout.
+
+
+
+`initializer_range`
+
+
+The stdev of the truncated_normal_initializer for
+initializing all weight matrices.
+
+
+
+`learning_rate`
+
+
+The initial learning rate for Adam.
+
+
+
+`distribution_strategy`
+
+
+ A string specifying which distribution strategy to
+use. Accepted values are 'off', 'one_device', 'mirrored',
+'parameter_server', 'multi_worker_mirrored', and 'tpu' -- case
+insensitive. 'off' means not to use Distribution Strategy; 'tpu' means
+to use TPUStrategy using `tpu_address`.
+
+
+
+`num_gpus`
+
+
+How many GPUs to use at each worker with the
+DistributionStrategies API. The default is -1, which means utilize all
+available GPUs.
+
+
+
+`tpu`
+
+
+TPU address to connect to.
+
+
+
+`trainable`
+
+
+boolean, whether pretrain layer is trainable.
+
+
+
+`predict_batch_size`
+
+
+Batch size for prediction.
+
+
+
+`do_lower_case`
+
+
+boolean, whether to lower case the input text. Should be
+True for uncased models and False for cased models.
+
+
+
+`is_tf2`
+
+
+boolean, whether the hub module is in TensorFlow 2.x format.
+
+
+
+`tflite_input_name`
+
+
+Dict, input names for the TFLite model.
+
+
+
+`tflite_output_name`
+
+
+Dict, output names for the TFLite model.
+
+
+
+`init_from_squad_model`
+
+
+boolean, whether to initialize from the model that
+is already retrained on Squad 1.1.
+
+
+
+`default_batch_size`
+
+
+Default batch size for training.
+
+
+
+`name`
+
+
+Name of the object.
+
+
+
diff --git a/site/en/lite/api_docs/python/tflite_model_maker/question_answer/QuestionAnswer.md b/site/en/lite/api_docs/python/tflite_model_maker/question_answer/QuestionAnswer.md
new file mode 100644
index 00000000000..ad720c2913f
--- /dev/null
+++ b/site/en/lite/api_docs/python/tflite_model_maker/question_answer/QuestionAnswer.md
@@ -0,0 +1,514 @@
+page_type: reference
+description: QuestionAnswer class for inference and exporting to tflite.
+
+
+
+
+
+
+
+
+Loads data and train the model for question answer.
+
+
+
+
+
+
Args
+
+
+
+`train_data`
+
+
+Training data.
+
+
+
+`model_spec`
+
+
+Specification for the model.
+
+
+
+`batch_size`
+
+
+Batch size for training.
+
+
+
+`epochs`
+
+
+Number of epochs for training.
+
+
+
+`steps_per_epoch`
+
+
+Integer or None. Total number of steps (batches of
+samples) before declaring one epoch finished and starting the next
+epoch. If `steps_per_epoch` is None, the epoch will run until the input
+dataset is exhausted.
+
+Integer or None. Total number of steps (batches of
+samples) before declaring one epoch finished and starting the next
+epoch. If `steps_per_epoch` is None, the epoch will run until the input
+dataset is exhausted.
+
+
+
+`shuffle`
+
+
+Whether the data should be shuffled.
+
+
+
+`do_train`
+
+
+Whether to run training.
+
+
+
+
+
+
+
+
+
+
Returns
+
+
+An instance based on QuestionAnswer.
+
+
+
+
diff --git a/site/en/lite/api_docs/python/tflite_model_maker/recommendation.md b/site/en/lite/api_docs/python/tflite_model_maker/recommendation.md
new file mode 100644
index 00000000000..31625b28563
--- /dev/null
+++ b/site/en/lite/api_docs/python/tflite_model_maker/recommendation.md
@@ -0,0 +1,52 @@
+page_type: reference
+description: APIs to train an on-device recommendation model.
+
+
+
+
+
+
+
+list of dict, each vocab item is described above.
+
+
+
+
+
+
+
+
+
+
+
+
Attributes
+
+
+
+`size`
+
+
+Returns the size of the dataset.
+
+Note that this function may return None becuase the exact size of the
+dataset isn't a necessary parameter to create an instance of this class,
+and tf.data.Dataset donesn't support a function to get the length directly
+since it's lazy-loaded and may be infinite.
+In most cases, however, when an instance of this class is created by helper
+functions like 'from_folder', the size of the dataset will be preprocessed,
+and this function can return an int representing the size of the dataset.
+
+
+Generates data loader from movielens dataset.
+
+The method downloads and prepares dataset, then generates for train/eval.
+
+For `movielens` data format, see:
+
+- function `_generate_fake_data` in `recommendation_testutil.py`
+- Or, zip file: http://files.grouplens.org/datasets/movielens/ml-1m.zip
+
+
+
+
+
Args
+
+
+
+`data_dir`
+
+
+str, path to dataset containing (unzipped) text data.
+
+
+
+`data_tag`
+
+
+str, specify dataset in {'train', 'test'}.
+
+
+
+`input_spec`
+
+
+InputSpec, specify data format for input and embedding.
+
+
+
+`generated_examples_dir`
+
+
+str, path to generate preprocessed examples.
+(default: same as data_dir)
+
+
+
+`min_timeline_length`
+
+
+int, min timeline length to split train/eval set.
+
+
+
+`max_context_length`
+
+
+int, max context length as one input.
+
+
+
+`max_context_movie_genre_length`
+
+
+int, max context length of movie genre as
+one input.
+
+@classmethod
+get_num_classes(
+ meta
+) -> int
+
+
+Gets number of classes.
+
+0 is reserved. Number of classes is Max Id + 1, e.g., if Max Id = 100,
+then classes are [0, 100], that is 101 classes in total.
+
+
+
+
+Loads vocab from file.
+
+The vocab file should be json format of: a list of list[size=4], where the 4
+elements are ordered as:
+ [id=int, title=str, genres=str joined with '|', count=int]
+It is generated when preparing movielens dataset.
+
+
+
+
+
Args
+
+
+
+`vocab_file`
+
+
+str, path to vocab file.
+
+
+
+
+
+
+
+
+
+
Returns
+
+
+
+`vocab`
+
+
+an OrderedDict maps id to item. Each item represents a movie
+{
+ 'id': int,
+ 'title': str,
+ 'genres': list[str],
+ 'count': int,
+}
+
+Embedding dataset used to build on-device ScaNN index file. The
+dataset shape should be (dataset_size, embedding_dim). If None,
+`dataset` will be generated from raw input data later.
+
+
+
+`metadata`
+
+
+ The metadata for each data in the dataset. The length of
+`metadata` should be same as `dataset` and passed in the same order as
+`dataset`. If `dataset` is set, `metadata` should be set as well.
+
+
+
+
+
+
+
+
+
+
+
+
Attributes
+
+
+
+`dataset`
+
+
+Gets the dataset.
+
+Due to performance consideration, we don't return a copy, but the returned
+`self._dataset` should never be changed.
+
+
+
+`embedder_path`
+
+
+Gets the path to the TFLite Embedder model file.
+
+
+Appends the dataset.
+
+Don't check if embedders from the two data loader are the same in this
+function. Users are responsible to keep the embedder identical.
+
+
+
+
+
Args
+
+
+
+`data_loader`
+
+
+The data loader in which the data will be appended.
+
+
+Appends the dataset.
+
+Don't check if embedders from the two data loader are the same in this
+function. Users are responsible to keep the embedder identical.
+
+
+
+
+
Args
+
+
+
+`data_loader`
+
+
+The data loader in which the data will be appended.
+
+
+Creates DataLoader for the Image Searcher task.
+
+
+
+
+
+
Args
+
+
+
+`image_embedder_path`
+
+
+Path to the ".tflite" image embedder model.
+
+
+
+`metadata_type`
+
+
+Type of MetadataLoader to load metadata for each input
+image based on image path. By default, load the file name as metadata
+for each input image.
+
+
+
+`l2_normalize`
+
+
+Whether to normalize the returned feature vector with L2
+norm. Use this option only if the model does not already contain a
+native L2_NORMALIZATION TF Lite Op. In most cases, this is already the
+case and L2 norm is thus achieved through TF Lite inference.
+
+
+
+
+
+
+
+
+
+
Returns
+
+
+DataLoader object created for the Image Searcher task.
+
+
+Loads image data from folder.
+
+Users can load images from different folders one by one. For instance,
+
+```
+# Creates data_loader instance.
+data_loader = image_searcher_dataloader.DataLoader.create(tflite_path)
+
+# Loads images, first from `image_path1` and secondly from `image_path2`.
+data_loader.load_from_folder(image_path1)
+data_loader.load_from_folder(image_path2)
+```
+
+
+
+
+
Args
+
+
+
+`path`
+
+
+image directory to be loaded.
+
+
+
+`mode`
+
+
+mode in which the file is opened, Used when metadata_type is
+FROM_DAT_FILE. Only 'r' and 'rb' are supported. 'r' means opening for
+reading, 'rb' means opening for reading binary.
+
+
+
+ScaNN
+(https://ai.googleblog.com/2020/07/announcing-scann-efficient-vector.html) is
+a highly efficient and scalable vector nearest neighbor retrieval
+library from Google Research. We use ScaNN to build the on-device search
+index, and do on-device retrieval with a simplified implementation.
+
+
+
+
+
+
+
+
Attributes
+
+
+
+`distance_measure`
+
+
+How to compute the distance. Allowed values are
+'dot_product' and 'squared_l2'. Please note that when distance is
+'dot_product', we actually compute the negative dot product between query
+and database vectors, to preserve the notion that "smaller is closer".
+
+
+
+`tree`
+
+
+Configure partitioning. If not set, no partitioning is performed.
+
+
+
+`score_ah`
+
+
+Configure asymmetric hashing. Must defined this or
+`score_brute_force`.
+
+
+
+`score_brute_force`
+
+
+Configure bruce force. Must defined this or `score_ah`.
+
+
+
+In ScaNN we use PQ to compress the database embeddings, but not the query
+embedding. We called it Asymmetric Hashing. See
+https://research.google/pubs/pub41694/
+
+
+
+
+
+
+
Attributes
+
+
+
+`dimensions_per_block`
+
+
+How many dimensions in each PQ block. If the embedding
+vector dimensionality is a multiple of this value, there will be
+`number_of_dimensions / dimensions_per_block` PQ blocks. Otherwise, the
+last block will be the remainder. For example, if a vector has 12
+dimensions, and `dimensions_per_block` is 2, then there will be 6
+2-dimension blocks. However, if the vector has 13 dimensions and
+`dimensions_per_block` is still 2, there will be 6 2-dimension blocks and
+one 1-dimension block.
+
+
+
+`anisotropic_quantization_threshold`
+
+
+If this value is set, we will penalize
+the quantization error that's parallel to the original vector differently
+than the orthogonal error. A generally recommended value for this
+parameter would be 0.2. For more details, please look at ScaNN's 2020 ICML
+paper https://arxiv.org/abs/1908.10396 and the Google AI Blog post
+https://ai.googleblog.com/2020/07/announcing-scann-efficient-vector.html
+
+
+
+`training_sample_size`
+
+
+How many database points to sample for training the
+K-Means for PQ centers. A good starting value would be 100k or the whole
+dataset if it's smaller than that.
+
+
+
+`training_iterations`
+
+
+How many iterations to run K-Means for PQ.
+
+
+
+
+
+
+## Methods
+
+
__eq__
+
+
+__eq__(
+ other
+)
+
+
+
+
+
+
+
+
+
+
+
+
+
Class Variables
+
+
+
+anisotropic_quantization_threshold
+
+
+`nan`
+
+
+
+training_iterations
+
+
+`10`
+
+
+
+training_sample_size
+
+
+`100000`
+
+
+
diff --git a/site/en/lite/api_docs/python/tflite_model_maker/searcher/ScoreBruteForce.md b/site/en/lite/api_docs/python/tflite_model_maker/searcher/ScoreBruteForce.md
new file mode 100644
index 00000000000..2f33925577f
--- /dev/null
+++ b/site/en/lite/api_docs/python/tflite_model_maker/searcher/ScoreBruteForce.md
@@ -0,0 +1,52 @@
+page_type: reference
+description: Bruce force in-partition scoring configuration.
+
+
+
+
+
+
+
+The cache directory to save serialized ScaNN and/or the tflite
+model. When cache_dir is not set, a temporary folder will be created and
+will **not** be removed automatically which makes it can be used later.
+
+
+Appends the dataset.
+
+Don't check if embedders from the two data loader are the same in this
+function. Users are responsible to keep the embedder identical.
+
+
+
+
+
Args
+
+
+
+`data_loader`
+
+
+The data loader in which the data will be appended.
+
+
+Creates DataLoader for the Text Searcher task.
+
+
+
+
+
+
Args
+
+
+
+`text_embedder_path`
+
+
+Path to the ".tflite" text embedder model. case and L2
+norm is thus achieved through TF Lite inference.
+
+
+
+`l2_normalize`
+
+
+Whether to normalize the returned feature vector with L2
+norm. Use this option only if the model does not already contain a
+native L2_NORMALIZATION TF Lite Op. In most cases, this is already the
+case and L2 norm is thus achieved through TF Lite inference.
+
+
+
+
+
+
+
+
+
+
Returns
+
+
+DataLoader object created for the Text Searcher task.
+
+
+Loads text data from csv file that includes a "header" line with titles.
+
+Users can load text from different csv files one by one. For instance,
+
+```
+# Creates data_loader instance.
+data_loader = text_searcher_dataloader.DataLoader.create(tflite_path)
+
+# Loads text, first from `text_path1` and secondly from `text_path2`.
+data_loader.load_from_csv(
+ text_path1, text_column='text', metadata_column='metadata')
+data_loader.load_from_csv(
+ text_path2, text_column='text', metadata_column='metadata')
+```
+
+
+
+
+
Args
+
+
+
+`path`
+
+
+Text csv file path to be loaded.
+
+
+
+`text_column`
+
+
+Column name for input text.
+
+
+
+`metadata_column`
+
+
+Column name for user metadata associated with each input
+text.
+
+
+
+`delimiter`
+
+
+Character used to separate fields.
+
+
+
+`quotechar`
+
+
+Character used to quote fields containing special characters.
+
+
+
+In ScaNN, we use single layer K-Means tree to partition the database (index)
+as a way to reduce search space.
+
+
+
+
+
+
+
Attributes
+
+
+
+`num_leaves`
+
+
+How many leaves (partitions) to have on the K-Means tree. In
+general, a good starting point would be the square root of the database
+size.
+
+
+
+`num_leaves_to_search`
+
+
+During inference ScaNN will compare the query vector
+against all the partition centroids and select the closest
+`num_leaves_to_search` ones to search in. The more leaves to search, the
+better the retrieval quality, and higher computational cost.
+
+
+
+`training_sample_size`
+
+
+How many database embeddings to sample for the K-Means
+training. Generally, you want to use a large enough sample of the database
+to train K-Means so that it's representative enough. However, large sample
+can also lead to longer training time. A good starting value would be
+100k, or the whole dataset if it's smaller than that.
+
+
+
+`min_partition_size`
+
+
+Smallest allowable cluster size. Any clusters smaller
+than this will be removed, and its data points will be merged with other
+clusters. Recommended to be 1/10 of average cluster size (size of database
+divided by `num_leaves`)
+
+
+
+`training_iterations`
+
+
+How many itrations to train K-Means.
+
+
+
+`spherical`
+
+
+If true, L2 normalize the K-Means centroids.
+
+
+
+`quantize_centroids`
+
+
+If true, quantize centroids to int8.
+
+
+
+`random_init`
+
+
+If true, use random init. Otherwise use K-Means++.
+
+
+
+
+
+
+## Methods
+
+
__eq__
+
+
+__eq__(
+ other
+)
+
+
+
+
+
+
+
+
+
+
+
+
+
Class Variables
+
+
+
+min_partition_size
+
+
+`50`
+
+
+
+quantize_centroids
+
+
+`False`
+
+
+
+random_init
+
+
+`True`
+
+
+
+spherical
+
+
+`False`
+
+
+
+training_iterations
+
+
+`12`
+
+
+
+training_sample_size
+
+
+`100000`
+
+
+
diff --git a/site/en/lite/api_docs/python/tflite_model_maker/text_classifier.md b/site/en/lite/api_docs/python/tflite_model_maker/text_classifier.md
new file mode 100644
index 00000000000..a29756c82f5
--- /dev/null
+++ b/site/en/lite/api_docs/python/tflite_model_maker/text_classifier.md
@@ -0,0 +1,52 @@
+page_type: reference
+description: APIs to train a text classification model.
+
+
+
+
+
+
+
+
+
+
+APIs to train a text classification model.
+
+
+
+#### Task guide:
+
+
+https://www.tensorflow.org/lite/tutorials/model_maker_text_classification
+
+## Classes
+
+[`class AverageWordVecSpec`](../tflite_model_maker/text_classifier/AverageWordVecSpec): A specification of averaging word vector model.
+
+[`class BertClassifierSpec`](../tflite_model_maker/text_classifier/BertClassifierSpec): A specification of BERT model for text classification.
+
+[`class DataLoader`](../tflite_model_maker/text_classifier/DataLoader): DataLoader for text classifier.
+
+[`class TextClassifier`](../tflite_model_maker/text_classifier/TextClassifier): TextClassifier class for inference and exporting to tflite.
+
+## Functions
+
+[`MobileBertClassifierSpec(...)`](../tflite_model_maker/text_classifier/MobileBertClassifierSpec): Creates MobileBert model spec for the text classification task. See also: tflite_model_maker.text_classifier.BertClassifierSpec.
+
+[`create(...)`](../tflite_model_maker/text_classifier/create): Loads data and train the model for test classification.
diff --git a/site/en/lite/api_docs/python/tflite_model_maker/text_classifier/AverageWordVecSpec.md b/site/en/lite/api_docs/python/tflite_model_maker/text_classifier/AverageWordVecSpec.md
new file mode 100644
index 00000000000..a8741b8983f
--- /dev/null
+++ b/site/en/lite/api_docs/python/tflite_model_maker/text_classifier/AverageWordVecSpec.md
@@ -0,0 +1,359 @@
+page_type: reference
+description: A specification of averaging word vector model.
+
+
+
+
+
+
+
+The stdev of the truncated_normal_initializer for
+initializing all weight matrices.
+
+
+
+`learning_rate`
+
+
+The initial learning rate for Adam.
+
+
+
+`distribution_strategy`
+
+
+ A string specifying which distribution strategy to
+use. Accepted values are 'off', 'one_device', 'mirrored',
+'parameter_server', 'multi_worker_mirrored', and 'tpu' -- case
+insensitive. 'off' means not to use Distribution Strategy; 'tpu' means
+to use TPUStrategy using `tpu_address`.
+
+
+
+`num_gpus`
+
+
+How many GPUs to use at each worker with the
+DistributionStrategies API. The default is -1, which means utilize all
+available GPUs.
+
+
+
+`tpu`
+
+
+TPU address to connect to.
+
+
+
+`trainable`
+
+
+boolean, whether pretrain layer is trainable.
+
+
+
+`do_lower_case`
+
+
+boolean, whether to lower case the input text. Should be
+True for uncased models and False for cased models.
+
+
+
+`is_tf2`
+
+
+boolean, whether the hub module is in TensorFlow 2.x format.
+
+
+
+`name`
+
+
+The name of the object.
+
+
+
+`tflite_input_name`
+
+
+Dict, input names for the TFLite model.
+
+
+
+`default_batch_size`
+
+
+Default batch size for training.
+
+
+
+`index_to_label`
+
+
+List of labels in the training data. e.g. ['neg', 'pos'].
+
+
+Creates classifier and runs the classifier training.
+
+
+
+
+
+
Args
+
+
+
+`train_ds`
+
+
+tf.data.Dataset, training data to be fed in
+tf.keras.Model.fit().
+
+
+
+`validation_ds`
+
+
+tf.data.Dataset, validation data to be fed in
+tf.keras.Model.fit().
+
+
+
+`epochs`
+
+
+Integer, training epochs.
+
+
+
+`steps_per_epoch`
+
+
+Integer or None. Total number of steps (batches of
+samples) before declaring one epoch finished and starting the next
+epoch. If `steps_per_epoch` is None, the epoch will run until the input
+dataset is exhausted.
+
+
+
+`num_classes`
+
+
+Interger, number of classes.
+
+
+
+`**kwargs`
+
+
+Other parameters used in the tf.keras.Model.fit().
+
+
+
+
+
+
+
+
+
+
Returns
+
+
+tf.keras.Model, the keras model that's already trained.
+
+A tf.data.Dataset object that contains a potentially large set of
+elements, where each element is a pair of (input_data, target). The
+`input_data` means the raw input data, like an image, a text etc., while
+the `target` means some ground truth of the raw input data, such as the
+classification label of the image etc.
+
+
+
+`size`
+
+
+The size of the dataset. tf.data.Dataset donesn't support a function
+to get the length directly since it's lazy-loaded and may be infinite.
+
+
+
+
+
+
+
+
+
+
+
+
Attributes
+
+
+
+`num_classes`
+
+
+
+
+
+
+`size`
+
+
+Returns the size of the dataset.
+
+Note that this function may return None becuase the exact size of the
+dataset isn't a necessary parameter to create an instance of this class,
+and tf.data.Dataset donesn't support a function to get the length directly
+since it's lazy-loaded and may be infinite.
+In most cases, however, when an instance of this class is created by helper
+functions like 'from_folder', the size of the dataset will be preprocessed,
+and this function can return an int representing the size of the dataset.
+
+
+Loads text with labels and preproecess text according to `model_spec`.
+
+Assume the text data of the same label are in the same subdirectory. each
+file is one text.
+
+
+
+
+
Args
+
+
+
+`filename`
+
+
+Name of the file.
+
+
+
+`model_spec`
+
+
+Specification for the model.
+
+
+
+`is_training`
+
+
+Whether the loaded data is for training or not.
+
+
+
+`class_labels`
+
+
+Class labels that should be considered. Name of the
+subdirectory not in `class_labels` will be ignored. If None, all the
+subdirectories will be considered.
+
+
+
+`shuffle`
+
+
+boolean, if shuffle, random shuffle data.
+
+
+
+`cache_dir`
+
+
+The cache directory to save preprocessed data. If None,
+generates a temporary directory to cache preprocessed data.
+
+
+
+
+
+
+
+
+
+
Returns
+
+
+TextDataset containing text, labels and other related info.
+
+The stdev of the truncated_normal_initializer for
+initializing all weight matrices.
+
+
+
+`learning_rate`
+
+
+The initial learning rate for Adam.
+
+
+
+`distribution_strategy`
+
+
+ A string specifying which distribution strategy to
+use. Accepted values are 'off', 'one_device', 'mirrored',
+'parameter_server', 'multi_worker_mirrored', and 'tpu' -- case
+insensitive. 'off' means not to use Distribution Strategy; 'tpu' means
+to use TPUStrategy using `tpu_address`.
+
+
+
+`num_gpus`
+
+
+How many GPUs to use at each worker with the
+DistributionStrategies API. The default is -1, which means utilize all
+available GPUs.
+
+
+
+`tpu`
+
+
+TPU address to connect to.
+
+
+
+`trainable`
+
+
+boolean, whether pretrain layer is trainable.
+
+
+
+`do_lower_case`
+
+
+boolean, whether to lower case the input text. Should be
+True for uncased models and False for cased models.
+
+
+
+`is_tf2`
+
+
+boolean, whether the hub module is in TensorFlow 2.x format.
+
+
+
+`name`
+
+
+The name of the object.
+
+
+
+`tflite_input_name`
+
+
+Dict, input names for the TFLite model.
+
+
+
+`default_batch_size`
+
+
+Default batch size for training.
+
+
+
+`index_to_label`
+
+
+List of labels in the training data. e.g. ['neg', 'pos'].
+
+
+
diff --git a/site/en/lite/api_docs/python/tflite_model_maker/text_classifier/TextClassifier.md b/site/en/lite/api_docs/python/tflite_model_maker/text_classifier/TextClassifier.md
new file mode 100644
index 00000000000..9766b8c6876
--- /dev/null
+++ b/site/en/lite/api_docs/python/tflite_model_maker/text_classifier/TextClassifier.md
@@ -0,0 +1,535 @@
+page_type: reference
+description: TextClassifier class for inference and exporting to tflite.
+
+
+
+
+
+
+
+
+Loads data and train the model for test classification.
+
+
+
+
+
+
Args
+
+
+
+`train_data`
+
+
+Training data.
+
+
+
+`model_spec`
+
+
+Specification for the model.
+
+
+
+`validation_data`
+
+
+Validation data. If None, skips validation process.
+
+
+
+`batch_size`
+
+
+Batch size for training.
+
+
+
+`epochs`
+
+
+Number of epochs for training.
+
+
+
+`steps_per_epoch`
+
+
+Integer or None. Total number of steps (batches of
+samples) before declaring one epoch finished and starting the next
+epoch. If `steps_per_epoch` is None, the epoch will run until the input
+dataset is exhausted.
+
+
+Feeds the training data for training.
+
+
+
+
+
+
+
+
+
+
Class Variables
+
+
+
+ALLOWED_EXPORT_FORMAT
+
+
+`(,
+ ,
+ ,
+ ,
+ )`
+
+
+
+DEFAULT_EXPORT_FORMAT
+
+
+`(,
+ ,
+ )`
+
+
+
diff --git a/site/en/lite/api_docs/python/tflite_model_maker/text_classifier/create.md b/site/en/lite/api_docs/python/tflite_model_maker/text_classifier/create.md
new file mode 100644
index 00000000000..d121b0fd078
--- /dev/null
+++ b/site/en/lite/api_docs/python/tflite_model_maker/text_classifier/create.md
@@ -0,0 +1,148 @@
+page_type: reference
+description: Loads data and train the model for test classification.
+
+
+
+
+
+
+
+Validation data. If None, skips validation process.
+
+
+
+`batch_size`
+
+
+Batch size for training.
+
+
+
+`epochs`
+
+
+Number of epochs for training.
+
+
+
+`steps_per_epoch`
+
+
+Integer or None. Total number of steps (batches of
+samples) before declaring one epoch finished and starting the next
+epoch. If `steps_per_epoch` is None, the epoch will run until the input
+dataset is exhausted.
+
+
+
+`shuffle`
+
+
+Whether the data should be shuffled.
+
+
+
+`do_train`
+
+
+Whether to run training.
+
+
+
+
+
+
+
+
+
+
Returns
+
+
+An instance based on TextClassifier.
+
+
+
+
diff --git a/site/en/lite/api_docs/python/tflite_support.md b/site/en/lite/api_docs/python/tflite_support.md
new file mode 100644
index 00000000000..bcd1c4691a5
--- /dev/null
+++ b/site/en/lite/api_docs/python/tflite_support.md
@@ -0,0 +1,54 @@
+page_type: reference
+description: The TensorFlow Lite Support Library.
+
+
+
+
+
+
+
+
+
+
+TensorFlow Lite metadata tools.
+
+
+
+## Classes
+
+[`class MetadataDisplayer`](../tflite_support/metadata/MetadataDisplayer): Displays metadata and associated file info in human-readable format.
+
+[`class MetadataPopulator`](../tflite_support/metadata/MetadataPopulator): Packs metadata and associated files into TensorFlow Lite model file.
+
+## Functions
+
+[`convert_to_json(...)`](../tflite_support/metadata/convert_to_json): Converts the metadata into a json string.
+
+[`get_metadata_buffer(...)`](../tflite_support/metadata/get_metadata_buffer): Returns the metadata in the model file as a buffer.
+
+[`get_path_to_datafile(...)`](../tflite_support/metadata/get_path_to_datafile): Gets the path to the specified file in the data dependencies.
diff --git a/site/en/lite/api_docs/python/tflite_support/metadata/MetadataDisplayer.md b/site/en/lite/api_docs/python/tflite_support/metadata/MetadataDisplayer.md
new file mode 100644
index 00000000000..60746e88b83
--- /dev/null
+++ b/site/en/lite/api_docs/python/tflite_support/metadata/MetadataDisplayer.md
@@ -0,0 +1,300 @@
+page_type: reference
+description: Displays metadata and associated file info in human-readable format.
+
+
+
+
+
+
+
+
+
+
+
+
+MetadataPopulator can be used to populate metadata and model associated files
+into a model file or a model buffer (in bytearray). It can also help to
+inspect list of files that have been packed into the model or are supposed to
+be packed into the model.
+
+The metadata file (or buffer) should be generated based on the metadata
+schema:
+third_party/tensorflow/lite/schema/metadata_schema.fbs
+
+#### Example usage:
+
+
+Populate matadata and label file into an image classifier model.
+
+First, based on metadata_schema.fbs, generate the metadata for this image
+classifer model using Flatbuffers API. Attach the label file onto the ouput
+tensor (the tensor of probabilities) in the metadata.
+
+Then, pack the metadata and label file into the model as follows.
+
+ ```python
+ # Populating a metadata file (or a metadta buffer) and associated files to
+ a model file:
+ populator = MetadataPopulator.with_model_file(model_file)
+ # For metadata buffer (bytearray read from the metadata file), use:
+ # populator.load_metadata_buffer(metadata_buf)
+ populator.load_metadata_file(metadata_file)
+ populator.load_associated_files([label.txt])
+ # For associated file buffer (bytearray read from the file), use:
+ # populator.load_associated_file_buffers({"label.txt": b"file content"})
+ populator.populate()
+
+ # Populating a metadata file (or a metadta buffer) and associated files to
+ a model buffer:
+ populator = MetadataPopulator.with_model_buffer(model_buf)
+ populator.load_metadata_file(metadata_file)
+ populator.load_associated_files([label.txt])
+ populator.populate()
+ # Writing the updated model buffer into a file.
+ updated_model_buf = populator.get_model_buffer()
+ with open("updated_model.tflite", "wb") as f:
+ f.write(updated_model_buf)
+
+ # Transferring metadata and associated files from another TFLite model:
+ populator = MetadataPopulator.with_model_buffer(model_buf)
+ populator_dst.load_metadata_and_associated_files(src_model_buf)
+ populator_dst.populate()
+ updated_model_buf = populator.get_model_buffer()
+ with open("updated_model.tflite", "wb") as f:
+ f.write(updated_model_buf)
+ ```
+
+Note that existing metadata buffer (if applied) will be overridden by the new
+metadata buffer.
+
+
+
+
+
Args
+
+
+
+`model_file`
+
+
+valid path to a TensorFlow Lite model file.
+
+
+
+
+
+
+
+
+
+
Raises
+
+
+
+`IOError`
+
+
+File not found.
+
+
+
+`ValueError`
+
+
+the model does not have the expected flatbuffer identifer.
+
+
+Gets a list of associated files recorded in metadata of the model file.
+
+Associated files may be attached to a model, a subgraph, or an input/output
+tensor.
+
+
+
+
+Loads the associated file buffers (in bytearray) to be populated.
+
+
+
+
+
+
Args
+
+
+
+`associated_files`
+
+
+a dictionary of associated file names and corresponding
+file buffers, such as {"file.txt": b"file content"}. If pass in file
+ paths for the file name, only the basename will be populated.
+
+error occured when parsing the metadata schema file.
+
+
+
diff --git a/site/en/lite/api_docs/python/tflite_support/metadata/get_metadata_buffer.md b/site/en/lite/api_docs/python/tflite_support/metadata/get_metadata_buffer.md
new file mode 100644
index 00000000000..a737a5d56e8
--- /dev/null
+++ b/site/en/lite/api_docs/python/tflite_support/metadata/get_metadata_buffer.md
@@ -0,0 +1,70 @@
+page_type: reference
+description: Returns the metadata in the model file as a buffer.
+
+
+
+
+
+
+
+Metadata buffer. Returns `None` if the model does not have metadata.
+
+
+
+
diff --git a/site/en/lite/api_docs/python/tflite_support/metadata/get_path_to_datafile.md b/site/en/lite/api_docs/python/tflite_support/metadata/get_path_to_datafile.md
new file mode 100644
index 00000000000..e5b29803b04
--- /dev/null
+++ b/site/en/lite/api_docs/python/tflite_support/metadata/get_path_to_datafile.md
@@ -0,0 +1,75 @@
+page_type: reference
+description: Gets the path to the specified file in the data dependencies.
+
+
+
+
+
+
+
+
+
+
+
+
+The path is relative to the file calling the function.
+
+It's a simple replacement of
+"tensorflow.python.platform.resource_loader.get_path_to_datafile".
+
+
+
+
+
Args
+
+
+
+`path`
+
+
+a string resource path relative to the calling file.
+
+
+
+
+
+
+
+
+
+
Returns
+
+
+The path to the specified file present in the data attribute of py_test
+or py_binary.
+
+
+
+
+TF Lite Metadata Writer API.
+
+
+This module provides interfaces for writing metadata for common model types
+supported by the task library, such as:
+
+ * Image classification
+ * Object detection
+ * Image segmentation
+ * (Bert) Natural language classification
+ * Audio classification
+
+It is provided as part of the `tflite-support` package:
+
+```
+pip install tflite-support
+```
+
+Learn more about this API in the [metadata writer
+tutorial](https://www.tensorflow.org/lite/convert/metadata_writer_tutorial).
+
+## Modules
+
+[`audio_classifier`](../tflite_support/metadata_writers/audio_classifier) module: Writes metadata and label file to the audio classifier models.
+
+[`bert_nl_classifier`](../tflite_support/metadata_writers/bert_nl_classifier) module: Writes metadata and label file to the Bert NL classifier models.
+
+[`image_classifier`](../tflite_support/metadata_writers/image_classifier) module: Writes metadata and label file to the image classifier models.
+
+[`image_segmenter`](../tflite_support/metadata_writers/image_segmenter) module: Writes metadata and label file to the image segmenter models.
+
+[`metadata_info`](../tflite_support/metadata_writers/metadata_info) module: Helper classes for common model metadata information.
+
+[`nl_classifier`](../tflite_support/metadata_writers/nl_classifier) module: Writes metadata and label file to the NL classifier models.
+
+[`object_detector`](../tflite_support/metadata_writers/object_detector) module: Writes metadata and label file to the object detector models.
+
+[`writer_utils`](../tflite_support/metadata_writers/writer_utils) module: Helper methods for writing metadata into TFLite models.
diff --git a/site/en/lite/api_docs/python/tflite_support/metadata_writers/audio_classifier.md b/site/en/lite/api_docs/python/tflite_support/metadata_writers/audio_classifier.md
new file mode 100644
index 00000000000..a16506d8ae8
--- /dev/null
+++ b/site/en/lite/api_docs/python/tflite_support/metadata_writers/audio_classifier.md
@@ -0,0 +1,43 @@
+page_type: reference
+description: Writes metadata and label file to the audio classifier models.
+
+
+
+
+
+
+
+
+Creates mandatory metadata for TFLite Support inference.
+
+The parameters required in this method are mandatory when using TFLite
+Support features, such as Task library and Codegen tool (Android Studio ML
+Binding). Other metadata fields will be set to default. If other fields need
+to be filled, use the method `create_from_metadata_info` to edit them.
+
+
+
+
+
Args
+
+
+
+`model_buffer`
+
+
+valid buffer of the model file.
+
+
+
+`sample_rate`
+
+
+the sample rate in Hz when the audio was captured.
+
+
+
+`channels`
+
+
+the channel count of the audio.
+
+
+
+`label_file_paths`
+
+
+paths to the label files [1] in the classification
+tensor. Pass in an empty list if the model does not have any label file.
+
+
+
+`score_calibration_md`
+
+
+information of the score calibration operation [2]
+ in the classification tensor. Optional if the model does not use score
+ calibration.
+[1]:
+ https://github.com/tensorflow/tflite-support/blob/b80289c4cd1224d0e1836c7654e82f070f9eefaa/tensorflow_lite_support/metadata/metadata_schema.fbs#L95
+[2]:
+ https://github.com/tensorflow/tflite-support/blob/5e0cdf5460788c481f5cd18aab8728ec36cf9733/tensorflow_lite_support/metadata/metadata_schema.fbs#L434
+
+
+Creates a MetadataWriter instance for multihead models.
+
+
+
+
+
+
Args
+
+
+
+`model_buffer`
+
+
+valid buffer of the model file.
+
+
+
+`general_md`
+
+
+general information about the model. If not specified, default
+general metadata will be generated.
+
+
+
+`input_md`
+
+
+input audio tensor informaton. If not specified, default input
+metadata will be generated.
+
+
+
+`output_md_list`
+
+
+information of each output tensor head. If not specified,
+ default metadata will be generated for each output tensor. If
+ `tensor_name` in each `ClassificationTensorMd` instance is not
+ specified, elements in `output_md_list` need to have one-to-one mapping
+ with the output tensors [1] in the TFLite model.
+[1]:
+ https://github.com/tensorflow/tflite-support/blob/b2a509716a2d71dfff706468680a729cc1604cff/tensorflow_lite_support/metadata/metadata_schema.fbs#L605-L612
+
+
+Gets the generated JSON metadata string before populated into model.
+
+This method returns the metadata buffer before populated into the model.
+More fields could be filled by MetadataPopulator, such as
+min_parser_version. Use get_populated_metadata_json() if you want to get the
+final metadata string.
+
+
+
+
+
Returns
+
+
+The generated JSON metadata string before populated into model.
+
+
+Gets the generated JSON metadata string after populated into model.
+
+More fields could be filled by MetadataPopulator, such as
+min_parser_version. Use get_metadata_json() if you want to get the
+original metadata string.
+
+
+
+
+
Returns
+
+
+The generated JSON metadata string after populated into model.
+
+
+Gets the generated JSON metadata string before populated into model.
+
+This method returns the metadata buffer before populated into the model.
+More fields could be filled by MetadataPopulator, such as
+min_parser_version. Use get_populated_metadata_json() if you want to get the
+final metadata string.
+
+
+
+
+
Returns
+
+
+The generated JSON metadata string before populated into model.
+
+
+Gets the generated JSON metadata string after populated into model.
+
+More fields could be filled by MetadataPopulator, such as
+min_parser_version. Use get_metadata_json() if you want to get the
+original metadata string.
+
+
+
+
+
Returns
+
+
+The generated JSON metadata string after populated into model.
+
+
+Creates mandatory metadata for TFLite Support inference.
+
+The parameters required in this method are mandatory when using TFLite
+Support features, such as Task library and Codegen tool (Android Studio ML
+Binding). Other metadata fields will be set to default. If other fields need
+to be filled, use the method `create_from_metadata_info` to edit them.
+
+`ids_name`, `mask_name`, and `segment_name` correspond to the Tensor.name
+in the TFLite schema, which help to determine the tensor order when
+populating metadata. The default values come from Model Maker.
+
+
+
+
+
Args
+
+
+
+`model_buffer`
+
+
+valid buffer of the model file.
+
+
+
+`tokenizer_md`
+
+
+information of the tokenizer used to process the input
+string, if any. Supported tokenziers are: `BertTokenizer` [1] and
+ `SentencePieceTokenizer` [2]. If the tokenizer is `RegexTokenizer`
+ [3], refer to nl_classifier.MetadataWriter.
+[1]:
+https://github.com/tensorflow/tflite-support/blob/b80289c4cd1224d0e1836c7654e82f070f9eefaa/tensorflow_lite_support/metadata/metadata_schema.fbs#L436
+[2]:
+https://github.com/tensorflow/tflite-support/blob/b80289c4cd1224d0e1836c7654e82f070f9eefaa/tensorflow_lite_support/metadata/metadata_schema.fbs#L473
+[3]:
+https://github.com/tensorflow/tflite-support/blob/b80289c4cd1224d0e1836c7654e82f070f9eefaa/tensorflow_lite_support/metadata/metadata_schema.fbs#L475
+
+
+
+`label_file_paths`
+
+
+paths to the label files [4] in the classification
+tensor. Pass in an empty list if the model does not have any label file.
+[4]:
+https://github.com/tensorflow/tflite-support/blob/b80289c4cd1224d0e1836c7654e82f070f9eefaa/tensorflow_lite_support/metadata/metadata_schema.fbs#L95
+
+
+
+`ids_name`
+
+
+name of the ids tensor, which represents the tokenized ids of
+the input text.
+
+
+
+`mask_name`
+
+
+name of the mask tensor, which represents the mask with 1 for
+real tokens and 0 for padding tokens.
+
+
+
+`segment_name`
+
+
+name of the segment ids tensor, where `0` stands for the
+first sequence, and `1` stands for the second sequence if exists.
+
+
+Gets the generated JSON metadata string before populated into model.
+
+This method returns the metadata buffer before populated into the model.
+More fields could be filled by MetadataPopulator, such as
+min_parser_version. Use get_populated_metadata_json() if you want to get the
+final metadata string.
+
+
+
+
+
Returns
+
+
+The generated JSON metadata string before populated into model.
+
+
+Gets the generated JSON metadata string after populated into model.
+
+More fields could be filled by MetadataPopulator, such as
+min_parser_version. Use get_metadata_json() if you want to get the
+original metadata string.
+
+
+
+
+
Returns
+
+
+The generated JSON metadata string after populated into model.
+
+
+Creates mandatory metadata for TFLite Support inference.
+
+The parameters required in this method are mandatory when using TFLite
+Support features, such as Task library and Codegen tool (Android Studio ML
+Binding). Other metadata fields will be set to default. If other fields need
+to be filled, use the method `create_from_metadata_info` to edit them.
+
+
+
+
+
Args
+
+
+
+`model_buffer`
+
+
+valid buffer of the model file.
+
+
+
+`input_norm_mean`
+
+
+the mean value used in the input tensor normalization
+[1].
+
+
+
+`input_norm_std`
+
+
+the std value used in the input tensor normalizarion [1].
+
+
+
+`label_file_paths`
+
+
+paths to the label files [2] in the classification
+tensor. Pass in an empty list if the model does not have any label file.
+
+
+
+`score_calibration_md`
+
+
+information of the score calibration operation [3]
+ in the classification tensor. Optional if the model does not use score
+ calibration.
+[1]:
+ https://www.tensorflow.org/lite/convert/metadata#normalization_and_quantization_parameters
+[2]:
+ https://github.com/tensorflow/tflite-support/blob/b80289c4cd1224d0e1836c7654e82f070f9eefaa/tensorflow_lite_support/metadata/metadata_schema.fbs#L95
+[3]:
+ https://github.com/tensorflow/tflite-support/blob/5e0cdf5460788c481f5cd18aab8728ec36cf9733/tensorflow_lite_support/metadata/metadata_schema.fbs#L434
+
+
+Gets the generated JSON metadata string before populated into model.
+
+This method returns the metadata buffer before populated into the model.
+More fields could be filled by MetadataPopulator, such as
+min_parser_version. Use get_populated_metadata_json() if you want to get the
+final metadata string.
+
+
+
+
+
Returns
+
+
+The generated JSON metadata string before populated into model.
+
+
+Gets the generated JSON metadata string after populated into model.
+
+More fields could be filled by MetadataPopulator, such as
+min_parser_version. Use get_metadata_json() if you want to get the
+original metadata string.
+
+
+
+
+
Returns
+
+
+The generated JSON metadata string after populated into model.
+
+
+Creates mandatory metadata for TFLite Support inference.
+
+The parameters required in this method are mandatory when using TFLite
+Support features, such as Task library and Codegen tool (Android Studio ML
+Binding). Other metadata fields will be set to default. If other fields need
+to be filled, use the method `create_from_metadata_info` to edit them.
+
+
+
+
+
Args
+
+
+
+`model_buffer`
+
+
+valid buffer of the model file.
+
+
+
+`input_norm_mean`
+
+
+the mean value used in the input tensor normalization
+[1].
+
+
+
+`input_norm_std`
+
+
+the std value used in the input tensor normalizarion [1].
+
+
+
+`label_file_paths`
+
+
+paths to the label files [2] in the category tensor.
+ Pass in an empty list If the model does not have any label file.
+[1]:
+ https://www.tensorflow.org/lite/convert/metadata#normalization_and_quantization_parameters
+[2]:
+ https://github.com/tensorflow/tflite-support/blob/b80289c4cd1224d0e1836c7654e82f070f9eefaa/tensorflow_lite_support/metadata/metadata_schema.fbs#L108
+
+
+Creates MetadataWriter based on general/input/outputs information.
+
+
+
+
+
+
Args
+
+
+
+`model_buffer`
+
+
+valid buffer of the model file.
+
+
+
+`general_md`
+
+
+general information about the model.
+
+
+
+`input_md`
+
+
+input image tensor informaton.
+
+
+
+`output_md`
+
+
+output segmentation mask tensor informaton. This tensor is a
+multidimensional array of [1 x mask_height x mask_width x num_classes],
+where mask_width and mask_height are the dimensions of the segmentation
+masks produced by the model, and num_classes is the number of classes
+supported by the model.
+
+
+Gets the generated JSON metadata string before populated into model.
+
+This method returns the metadata buffer before populated into the model.
+More fields could be filled by MetadataPopulator, such as
+min_parser_version. Use get_populated_metadata_json() if you want to get the
+final metadata string.
+
+
+
+
+
Returns
+
+
+The generated JSON metadata string before populated into model.
+
+
+Gets the generated JSON metadata string after populated into model.
+
+More fields could be filled by MetadataPopulator, such as
+min_parser_version. Use get_metadata_json() if you want to get the
+original metadata string.
+
+
+
+
+
Returns
+
+
+The generated JSON metadata string after populated into model.
+
+
+
+
+
+
+## Modules
+
+[`writer_utils`](../../tflite_support/metadata_writers/writer_utils) module: Helper methods for writing metadata into TFLite models.
+
+## Classes
+
+[`class AssociatedFileMd`](../../tflite_support/metadata_writers/metadata_info/AssociatedFileMd): A container for common associated file metadata information.
+
+[`class BertInputTensorsMd`](../../tflite_support/metadata_writers/metadata_info/BertInputTensorsMd): A container for the input tensor metadata information of Bert models.
+
+[`class BertTokenizerMd`](../../tflite_support/metadata_writers/metadata_info/BertTokenizerMd): A container for the Bert tokenizer [1] metadata information.
+
+[`class CategoryTensorMd`](../../tflite_support/metadata_writers/metadata_info/CategoryTensorMd): A container for the category tensor metadata information.
+
+[`class ClassificationTensorMd`](../../tflite_support/metadata_writers/metadata_info/ClassificationTensorMd): A container for the classification tensor metadata information.
+
+[`class GeneralMd`](../../tflite_support/metadata_writers/metadata_info/GeneralMd): A container for common metadata information of a model.
+
+[`class InputAudioTensorMd`](../../tflite_support/metadata_writers/metadata_info/InputAudioTensorMd): A container for the input audio tensor metadata information.
+
+[`class InputImageTensorMd`](../../tflite_support/metadata_writers/metadata_info/InputImageTensorMd): A container for input image tensor metadata information.
+
+[`class InputTextTensorMd`](../../tflite_support/metadata_writers/metadata_info/InputTextTensorMd): A container for the input text tensor metadata information.
+
+[`class LabelFileMd`](../../tflite_support/metadata_writers/metadata_info/LabelFileMd): A container for label file metadata information.
+
+[`class RegexTokenizerMd`](../../tflite_support/metadata_writers/metadata_info/RegexTokenizerMd): A container for the Regex tokenizer [1] metadata information.
+
+[`class ScoreCalibrationMd`](../../tflite_support/metadata_writers/metadata_info/ScoreCalibrationMd): A container for score calibration [1] metadata information.
+
+[`class SentencePieceTokenizerMd`](../../tflite_support/metadata_writers/metadata_info/SentencePieceTokenizerMd): A container for the sentence piece tokenizer [1] metadata information.
+
+[`class TensorMd`](../../tflite_support/metadata_writers/metadata_info/TensorMd): A container for common tensor metadata information.
diff --git a/site/en/lite/api_docs/python/tflite_support/metadata_writers/metadata_info/AssociatedFileMd.md b/site/en/lite/api_docs/python/tflite_support/metadata_writers/metadata_info/AssociatedFileMd.md
new file mode 100644
index 00000000000..7b2562555b3
--- /dev/null
+++ b/site/en/lite/api_docs/python/tflite_support/metadata_writers/metadata_info/AssociatedFileMd.md
@@ -0,0 +1,122 @@
+page_type: reference
+description: A container for common associated file metadata information.
+
+
+
+
+
+
+
+name of the ids tensor, which represents the tokenized ids of
+the input text.
+
+
+
+`mask_name`
+
+
+name of the mask tensor, which represents the mask with 1 for
+real tokens and 0 for padding tokens.
+
+
+
+`segment_name`
+
+
+name of the segment ids tensor, where `0` stands for the
+first sequence, and `1` stands for the second sequence if exists.
+
+
+
+`ids_md`
+
+
+input ids tensor informaton.
+
+
+
+`mask_md`
+
+
+input mask tensor informaton.
+
+
+
+`segment_ids_md`
+
+
+input segment tensor informaton.
+
+
+
+`tokenizer_md`
+
+
+information of the tokenizer used to process the input
+string, if any. Supported tokenziers are: `BertTokenizer` [1] and
+ `SentencePieceTokenizer` [2]. If the tokenizer is `RegexTokenizer`
+ [3], refer to nl_classifier.MetadataWriter.
+[1]:
+https://github.com/tensorflow/tflite-support/blob/b80289c4cd1224d0e1836c7654e82f070f9eefaa/tensorflow_lite_support/metadata/metadata_schema.fbs#L436
+[2]:
+https://github.com/tensorflow/tflite-support/blob/b80289c4cd1224d0e1836c7654e82f070f9eefaa/tensorflow_lite_support/metadata/metadata_schema.fbs#L473
+[3]:
+https://github.com/tensorflow/tflite-support/blob/b80289c4cd1224d0e1836c7654e82f070f9eefaa/tensorflow_lite_support/metadata/metadata_schema.fbs#L475
+
+
+Gets the associated files that are packed in the tokenizer.
diff --git a/site/en/lite/api_docs/python/tflite_support/metadata_writers/metadata_info/BertTokenizerMd.md b/site/en/lite/api_docs/python/tflite_support/metadata_writers/metadata_info/BertTokenizerMd.md
new file mode 100644
index 00000000000..08fc00e35c7
--- /dev/null
+++ b/site/en/lite/api_docs/python/tflite_support/metadata_writers/metadata_info/BertTokenizerMd.md
@@ -0,0 +1,94 @@
+page_type: reference
+description: A container for the Bert tokenizer [1] metadata information.
+
+
+
+
+
+
+
+information of the label files [1] in the category tensor.
+[1]:
+ https://github.com/tensorflow/tflite-support/blob/b80289c4cd1224d0e1836c7654e82f070f9eefaa/tensorflow_lite_support/metadata/metadata_schema.fbs#L108
+
+information of the label files [1] in the classification
+tensor.
+
+
+
+`tensor_type`
+
+
+data type of the tensor.
+
+
+
+`score_calibration_md`
+
+
+information of the score calibration files operation
+[2] in the classification tensor.
+
+
+
+`tensor_name`
+
+
+name of the corresponding tensor [3] in the TFLite model. It
+ is used to locate the corresponding classification tensor and decide the
+ order of the tensor metadata [4] when populating model metadata.
+[1]:
+ https://github.com/tensorflow/tflite-support/blob/b80289c4cd1224d0e1836c7654e82f070f9eefaa/tensorflow_lite_support/metadata/metadata_schema.fbs#L95
+[2]:
+ https://github.com/tensorflow/tflite-support/blob/5e0cdf5460788c481f5cd18aab8728ec36cf9733/tensorflow_lite_support/metadata/metadata_schema.fbs#L434
+[3]:
+ https://github.com/tensorflow/tensorflow/blob/cb67fef35567298b40ac166b0581cd8ad68e5a3a/tensorflow/lite/schema/schema.fbs#L1129-L1136
+[4]:
+ https://github.com/tensorflow/tflite-support/blob/b2a509716a2d71dfff706468680a729cc1604cff/tensorflow_lite_support/metadata/metadata_schema.fbs#L595-L612
+
+
+
+
+
+
+
+
+
+
+
+
Attributes
+
+
+
+`label_files`
+
+
+information of the label files [1] in the classification
+tensor.
+
+
+
+`score_calibration_md`
+
+
+information of the score calibration operation [2] in
+ the classification tensor.
+[1]:
+ https://github.com/tensorflow/tflite-support/blob/b80289c4cd1224d0e1836c7654e82f070f9eefaa/tensorflow_lite_support/metadata/metadata_schema.fbs#L95
+[2]:
+ https://github.com/tensorflow/tflite-support/blob/5e0cdf5460788c481f5cd18aab8728ec36cf9733/tensorflow_lite_support/metadata/metadata_schema.fbs#L434
+
+
+Creates the classification tensor metadata based on the information.
diff --git a/site/en/lite/api_docs/python/tflite_support/metadata_writers/metadata_info/GeneralMd.md b/site/en/lite/api_docs/python/tflite_support/metadata_writers/metadata_info/GeneralMd.md
new file mode 100644
index 00000000000..3bbeaa58bf1
--- /dev/null
+++ b/site/en/lite/api_docs/python/tflite_support/metadata_writers/metadata_info/GeneralMd.md
@@ -0,0 +1,126 @@
+page_type: reference
+description: A container for common metadata information of a model.
+
+
+
+
+
+
+
+the mean value used in tensor normalization [1].
+
+
+
+`norm_std`
+
+
+the std value used in the tensor normalization [1]. norm_mean
+and norm_std must have the same dimension.
+
+
+
+`color_space_type`
+
+
+the color space type of the input image [2].
+
+
+
+`tensor_type`
+
+
+data type of the tensor.
+[1]:
+ https://www.tensorflow.org/lite/convert/metadata#normalization_and_quantization_parameters
+[2]:
+https://github.com/tensorflow/tflite-support/blob/b80289c4cd1224d0e1836c7654e82f070f9eefaa/tensorflow_lite_support/metadata/metadata_schema.fbs#L172
+
+
+
+
+
+
+
+
+
+
Raises
+
+
+
+`ValueError`
+
+
+if norm_mean and norm_std have different dimensions.
+
+
+
+
+
+
+
+
+
+
+
+
Attributes
+
+
+
+`norm_mean`
+
+
+the mean value used in tensor normalization [1].
+
+
+
+`norm_std`
+
+
+the std value used in the tensor normalization [1]. norm_mean and
+norm_std must have the same dimension.
+
+
+
+`color_space_type`
+
+
+the color space type of the input image [2].
+[1]:
+ https://www.tensorflow.org/lite/convert/metadata#normalization_and_quantization_parameters
+[2]:
+ https://github.com/tensorflow/tflite-support/blob/b80289c4cd1224d0e1836c7654e82f070f9eefaa/tensorflow_lite_support/metadata/metadata_schema.fbs#L172
+
+information of the tokenizer in the input text tensor, if
+any. Only `RegexTokenizer` [1] is currenly supported. If the tokenizer
+is `BertTokenizer` [2] or `SentencePieceTokenizer` [3], refer to
+bert_nl_classifier.MetadataWriter.
+[1]:
+https://github.com/tensorflow/tflite-support/blob/b80289c4cd1224d0e1836c7654e82f070f9eefaa/tensorflow_lite_support/metadata/metadata_schema.fbs#L475
+[2]:
+https://github.com/tensorflow/tflite-support/blob/b80289c4cd1224d0e1836c7654e82f070f9eefaa/tensorflow_lite_support/metadata/metadata_schema.fbs#L436
+[3]:
+https://github.com/tensorflow/tflite-support/blob/b80289c4cd1224d0e1836c7654e82f070f9eefaa/tensorflow_lite_support/metadata/metadata_schema.fbs#L473
+
+
+
+
+
+
+
+
+
+
+
+
Attributes
+
+
+
+`tokenizer_md`
+
+
+information of the tokenizer in the input text tensor, if any.
+
+locale of the label file [1].
+[1]:
+https://github.com/tensorflow/tflite-support/blob/b80289c4cd1224d0e1836c7654e82f070f9eefaa/tensorflow_lite_support/metadata/metadata_schema.fbs#L154
+
+information of the associated files in the tensor.
+
+
+
+`tensor_name`
+
+
+name of the corresponding tensor [1] in the TFLite model. It is
+ used to locate the corresponding tensor and decide the order of the tensor
+ metadata [2] when populating model metadata.
+[1]:
+ https://github.com/tensorflow/tensorflow/blob/cb67fef35567298b40ac166b0581cd8ad68e5a3a/tensorflow/lite/schema/schema.fbs#L1129-L1136
+[2]:
+ https://github.com/tensorflow/tflite-support/blob/b2a509716a2d71dfff706468680a729cc1604cff/tensorflow_lite_support/metadata/metadata_schema.fbs#L595-L612
+
+
+Creates mandatory metadata for TFLite Support inference.
+
+The parameters required in this method are mandatory when using TFLite
+Support features, such as Task library and Codegen tool (Android Studio ML
+Binding). Other metadata fields will be set to default. If other fields need
+to be filled, use the method `create_from_metadata_info` to edit them.
+
+
+
+
+
Args
+
+
+
+`model_buffer`
+
+
+valid buffer of the model file.
+
+
+
+`tokenizer_md`
+
+
+information of the tokenizer used to process the input
+string, if any. Only `RegexTokenizer` [1] is currently supported. If the
+tokenizer is `BertTokenizer` [2] or `SentencePieceTokenizer` [3], refer
+to bert_nl_classifier.MetadataWriter.
+[1]:
+https://github.com/tensorflow/tflite-support/blob/b80289c4cd1224d0e1836c7654e82f070f9eefaa/tensorflow_lite_support/metadata/metadata_schema.fbs#L475
+[2]:
+https://github.com/tensorflow/tflite-support/blob/b80289c4cd1224d0e1836c7654e82f070f9eefaa/tensorflow_lite_support/metadata/metadata_schema.fbs#L436
+[3]:
+https://github.com/tensorflow/tflite-support/blob/b80289c4cd1224d0e1836c7654e82f070f9eefaa/tensorflow_lite_support/metadata/metadata_schema.fbs#L473
+
+
+
+`label_file_paths`
+
+
+paths to the label files [4] in the classification
+tensor. Pass in an empty list if the model does not have any label
+file.
+[4]:
+https://github.com/tensorflow/tflite-support/blob/b80289c4cd1224d0e1836c7654e82f070f9eefaa/tensorflow_lite_support/metadata/metadata_schema.fbs#L95
+
+
+Gets the generated JSON metadata string before populated into model.
+
+This method returns the metadata buffer before populated into the model.
+More fields could be filled by MetadataPopulator, such as
+min_parser_version. Use get_populated_metadata_json() if you want to get the
+final metadata string.
+
+
+
+
+
Returns
+
+
+The generated JSON metadata string before populated into model.
+
+
+Gets the generated JSON metadata string after populated into model.
+
+More fields could be filled by MetadataPopulator, such as
+min_parser_version. Use get_metadata_json() if you want to get the
+original metadata string.
+
+
+
+
+
Returns
+
+
+The generated JSON metadata string after populated into model.
+
+
+Creates mandatory metadata for TFLite Support inference.
+
+The parameters required in this method are mandatory when using TFLite
+Support features, such as Task library and Codegen tool (Android Studio ML
+Binding). Other metadata fields will be set to default. If other fields need
+to be filled, use the method `create_from_metadata_info` to edit them.
+
+
+
+
+
Args
+
+
+
+`model_buffer`
+
+
+valid buffer of the model file.
+
+
+
+`input_norm_mean`
+
+
+the mean value used in the input tensor normalization
+[1].
+
+
+
+`input_norm_std`
+
+
+the std value used in the input tensor normalizarion [1].
+
+
+
+`label_file_paths`
+
+
+paths to the label files [2] in the category tensor.
+Pass in an empty list, If the model does not have any label file.
+
+
+
+`score_calibration_md`
+
+
+information of the score calibration operation [3]
+ in the classification tensor. Optional if the model does not use score
+ calibration.
+[1]:
+ https://www.tensorflow.org/lite/convert/metadata#normalization_and_quantization_parameters
+[2]:
+ https://github.com/tensorflow/tflite-support/blob/b80289c4cd1224d0e1836c7654e82f070f9eefaa/tensorflow_lite_support/metadata/metadata_schema.fbs#L108
+[3]:
+ https://github.com/tensorflow/tflite-support/blob/5e0cdf5460788c481f5cd18aab8728ec36cf9733/tensorflow_lite_support/metadata/metadata_schema.fbs#L434
+
+
+Creates MetadataWriter based on general/input/outputs information.
+
+
+
+
+
+
Args
+
+
+
+`model_buffer`
+
+
+valid buffer of the model file.
+
+
+
+`general_md`
+
+
+general information about the model.
+
+
+
+`input_md`
+
+
+input image tensor informaton.
+
+
+
+`output_location_md`
+
+
+output location tensor informaton. The location tensor
+is a multidimensional array of [N][4] floating point values between 0
+and 1, the inner arrays representing bounding boxes in the form [top,
+left, bottom, right].
+
+
+
+`output_category_md`
+
+
+output category tensor information. The category
+tensor is an array of N integers (output as floating point values) each
+indicating the index of a class label from the labels file.
+
+
+
+`output_score_md`
+
+
+output score tensor information. The score tensor is an
+array of N floating point values between 0 and 1 representing
+probability that a class was detected. Use ClassificationTensorMd to
+calibrate score.
+
+
+
+`output_number_md`
+
+
+output number of detections tensor information. This
+tensor is an integer value of N.
+
+
+Gets the generated JSON metadata string before populated into model.
+
+This method returns the metadata buffer before populated into the model.
+More fields could be filled by MetadataPopulator, such as
+min_parser_version. Use get_populated_metadata_json() if you want to get the
+final metadata string.
+
+
+
+
+
Returns
+
+
+The generated JSON metadata string before populated into model.
+
+
+Gets the generated JSON metadata string after populated into model.
+
+More fields could be filled by MetadataPopulator, such as
+min_parser_version. Use get_metadata_json() if you want to get the
+original metadata string.
+
+
+
+
+
Returns
+
+
+The generated JSON metadata string after populated into model.
+
+
+
+
+
+
+## Functions
+
+[`compute_flat_size(...)`](../../tflite_support/metadata_writers/writer_utils/compute_flat_size): Computes the flat size (number of elements) of tensor shape.
+
+[`get_input_tensor_names(...)`](../../tflite_support/metadata_writers/writer_utils/get_input_tensor_names): Gets a list of the input tensor names.
+
+[`get_input_tensor_shape(...)`](../../tflite_support/metadata_writers/writer_utils/get_input_tensor_shape): Gets the shape of the specified input tensor.
+
+[`get_input_tensor_types(...)`](../../tflite_support/metadata_writers/writer_utils/get_input_tensor_types): Gets a list of the input tensor types.
+
+[`get_output_tensor_names(...)`](../../tflite_support/metadata_writers/writer_utils/get_output_tensor_names): Gets a list of the output tensor names.
+
+[`get_output_tensor_types(...)`](../../tflite_support/metadata_writers/writer_utils/get_output_tensor_types): Gets a list of the output tensor types.
+
+[`get_tokenizer_associated_files(...)`](../../tflite_support/metadata_writers/writer_utils/get_tokenizer_associated_files): Gets a list of associated files packed in the tokenzier_options.
+
+[`load_file(...)`](../../tflite_support/metadata_writers/writer_utils/load_file): Loads file from the file path.
+
+[`save_file(...)`](../../tflite_support/metadata_writers/writer_utils/save_file): Loads file from the file path.
diff --git a/site/en/lite/api_docs/python/tflite_support/metadata_writers/writer_utils/compute_flat_size.md b/site/en/lite/api_docs/python/tflite_support/metadata_writers/writer_utils/compute_flat_size.md
new file mode 100644
index 00000000000..a9b8b2e45da
--- /dev/null
+++ b/site/en/lite/api_docs/python/tflite_support/metadata_writers/writer_utils/compute_flat_size.md
@@ -0,0 +1,78 @@
+page_type: reference
+description: Computes the flat size (number of elements) of tensor shape.
+
+
+
+
+
+
+
+
+
+
+The TensorFlow Lite Task Library.
+
+
+TensorFlow Lite Task Library contains a set of powerful and easy-to-use
+task-specific libraries for app developers to create ML experiences with
+TensorFlow Lite. It provides optimized out-of-box model interfaces for popular
+machine learning tasks, such as image and text classification. The model
+interfaces are specifically designed for each task to achieve the best
+performance and usability.
+
+Read more in the [Task Library Guide](
+https://tensorflow.org/lite/inference_with_metadata/task_library/overview).
+
+## Modules
+
+[`audio`](../tflite_support/task/audio) module: TensorFlow Lite Task Library Audio APIs.
+
+[`core`](../tflite_support/task/core) module: TensorFlow Lite Task Library's core module.
+
+[`processor`](../tflite_support/task/processor) module: TensorFlow Lite Task Library's processor module.
+
+[`text`](../tflite_support/task/text) module: TensorFlow Lite Task Library Text APIs.
+
+[`vision`](../tflite_support/task/vision) module: TensorFlow Lite Task Library Vision APIs.
diff --git a/site/en/lite/api_docs/python/tflite_support/task/audio.md b/site/en/lite/api_docs/python/tflite_support/task/audio.md
new file mode 100644
index 00000000000..0506d322d9a
--- /dev/null
+++ b/site/en/lite/api_docs/python/tflite_support/task/audio.md
@@ -0,0 +1,48 @@
+page_type: reference
+description: TensorFlow Lite Task Library Audio APIs.
+
+
+
+
+
+
+
+
+
+
+TensorFlow Lite Task Library Audio APIs.
+
+
+This module provides interface to run TensorFlow Lite audio models.
+
+## Classes
+
+[`class AudioClassifier`](../../tflite_support/task/audio/AudioClassifier): Class that performs classification on audio.
+
+[`class AudioClassifierOptions`](../../tflite_support/task/audio/AudioClassifierOptions): Options for the audio classifier task.
+
+[`class AudioEmbedder`](../../tflite_support/task/audio/AudioEmbedder): Class that performs dense feature vector extraction on audio.
+
+[`class AudioEmbedderOptions`](../../tflite_support/task/audio/AudioEmbedderOptions): Options for the audio embedder task.
+
+[`class AudioFormat`](../../tflite_support/task/audio/AudioFormat)
+
+[`class AudioRecord`](../../tflite_support/task/audio/AudioRecord): A class to record audio in a streaming basis.
+
+[`class TensorAudio`](../../tflite_support/task/audio/TensorAudio): A wrapper class to store the input audio.
diff --git a/site/en/lite/api_docs/python/tflite_support/task/audio/AudioClassifier.md b/site/en/lite/api_docs/python/tflite_support/task/audio/AudioClassifier.md
new file mode 100644
index 00000000000..0c5159acd2b
--- /dev/null
+++ b/site/en/lite/api_docs/python/tflite_support/task/audio/AudioClassifier.md
@@ -0,0 +1,331 @@
+page_type: reference
+description: Class that performs classification on audio.
+
+
+
+
+
+
+
diff --git a/site/en/lite/api_docs/python/tflite_support/task/audio/AudioRecord.md b/site/en/lite/api_docs/python/tflite_support/task/audio/AudioRecord.md
new file mode 100644
index 00000000000..02a0449e6a6
--- /dev/null
+++ b/site/en/lite/api_docs/python/tflite_support/task/audio/AudioRecord.md
@@ -0,0 +1,223 @@
+page_type: reference
+description: A class to record audio in a streaming basis.
+
+
+
+
+
+
+
+
+Creates `TensorAudio` object from the WAV file.
+
+
+
+
+
+
Args
+
+
+
+`file_name`
+
+
+WAV file name.
+
+
+
+`sample_count`
+
+
+The number of samples to read from the WAV file. This value
+should match with the input size of the TensorFlow Lite audio model that
+will consume the created TensorAudio object. If the WAV file contains
+more samples than sample_count, only the samples at the beginning of the
+WAV file will be loaded.
+
+
+
+`offset`
+
+
+An optional offset for allowing the user to skip a certain number
+samples at the beginning.
+
+
+
+
+
+
+
+
+
+
Returns
+
+
+`TensorAudio` object.
+
+
+
+
+
+
+
+
+
+
+
Raises
+
+
+
+`ValueError`
+
+
+If an input parameter, such as the audio file, is invalid.
+
+
+
+
+
+
+Represents external files used by the Task APIs (e.g. TF Lite FlatBuffer or
+plain-text labels file). The files can be specified by one of the following
+two ways:
+
+(1) file contents loaded in `file_content`.
+(2) file path in `file_name`.
+
+If more than one field of these fields is provided, they are used in this
+precedence order.
+
+
+
+
+
+
+
Attributes
+
+
+
+`file_name`
+
+
+Path to the index.
+
+
+
+`file_content`
+
+
+The index file contents as bytes.
+
+
+
+`num_threads`
+
+
+Number of thread, the default value is -1 which means
+Interpreter will decide what is the most appropriate `num_threads`.
+
+
+
+`use_coral`
+
+
+If true, inference will be delegated to a connected Coral Edge
+TPU device.
+
+
+
+
+TensorFlow Lite Task Library's processor module.
+
+
+This module contains classes related to the pre-processing and post-processing
+steps of the Task Library.
+
+## Classes
+
+[`class BertCluAnnotationOptions`](../../tflite_support/task/processor/BertCluAnnotationOptions): Options for Bert CLU Annotator processor.
+
+[`class BoundingBox`](../../tflite_support/task/processor/BoundingBox): An integer bounding box, axis aligned.
+
+[`class CategoricalSlot`](../../tflite_support/task/processor/CategoricalSlot): Represents a categorical slot whose values are within a finite set.
+
+[`class Category`](../../tflite_support/task/processor/Category): A classification category.
+
+[`class ClassificationOptions`](../../tflite_support/task/processor/ClassificationOptions): Options for classification processor.
+
+[`class ClassificationResult`](../../tflite_support/task/processor/ClassificationResult): Contains one set of results per classifier head.
+
+[`class Classifications`](../../tflite_support/task/processor/Classifications): List of predicted classes (aka labels) for a given classifier head.
+
+[`class CluRequest`](../../tflite_support/task/processor/CluRequest): The input to CLU (Conversational Language Understanding).
+
+[`class CluResponse`](../../tflite_support/task/processor/CluResponse): The output of CLU.
+
+[`class ColoredLabel`](../../tflite_support/task/processor/ColoredLabel): Defines a label associated with an RGB color, for display purposes.
+
+[`class ConfidenceMask`](../../tflite_support/task/processor/ConfidenceMask): 2D-array representing the confidence mask in row major order.
+
+[`class Detection`](../../tflite_support/task/processor/Detection): Represents one detected object in the object detector's results.
+
+[`class DetectionOptions`](../../tflite_support/task/processor/DetectionOptions): Options for object detection processor.
+
+[`class DetectionResult`](../../tflite_support/task/processor/DetectionResult): Represents the list of detected objects.
+
+[`class Embedding`](../../tflite_support/task/processor/Embedding): Result produced by one of the embedder model output layers.
+
+[`class EmbeddingOptions`](../../tflite_support/task/processor/EmbeddingOptions): Options for embedding processor.
+
+[`class EmbeddingResult`](../../tflite_support/task/processor/EmbeddingResult): Embeddings produced by the Embedder.
+
+[`class FeatureVector`](../../tflite_support/task/processor/FeatureVector): A dense feature vector.
+
+[`class Mention`](../../tflite_support/task/processor/Mention): A single mention result.
+
+[`class MentionedSlot`](../../tflite_support/task/processor/MentionedSlot): Non-categorical slot whose values are open text extracted from the input text.
+
+[`class NearestNeighbor`](../../tflite_support/task/processor/NearestNeighbor): A single nearest neighbor.
+
+[`class OutputType`](../../tflite_support/task/processor/OutputType): An enumeration.
+
+[`class Pos`](../../tflite_support/task/processor/Pos): Position information of the answer relative to context.
+
+[`class QaAnswer`](../../tflite_support/task/processor/QaAnswer): Represents the Answer to BertQuestionAnswerer.
+
+[`class QuestionAnswererResult`](../../tflite_support/task/processor/QuestionAnswererResult): The list of probable answers generated by BertQuestionAnswerer.
+
+[`class SearchOptions`](../../tflite_support/task/processor/SearchOptions): Options for search processor.
+
+[`class SearchResult`](../../tflite_support/task/processor/SearchResult): Results from a search as a list of nearest neigbors.
+
+[`class Segmentation`](../../tflite_support/task/processor/Segmentation): Represents one Segmentation object in the image segmenter's results.
+
+[`class SegmentationOptions`](../../tflite_support/task/processor/SegmentationOptions): Options for segmentation processor.
+
+[`class SegmentationResult`](../../tflite_support/task/processor/SegmentationResult): Results of performing image segmentation.
diff --git a/site/en/lite/api_docs/python/tflite_support/task/processor/BertCluAnnotationOptions.md b/site/en/lite/api_docs/python/tflite_support/task/processor/BertCluAnnotationOptions.md
new file mode 100644
index 00000000000..e28e4ea598c
--- /dev/null
+++ b/site/en/lite/api_docs/python/tflite_support/task/processor/BertCluAnnotationOptions.md
@@ -0,0 +1,192 @@
+page_type: reference
+description: Options for Bert CLU Annotator processor.
+
+
+
+
+
+
+
+
+
+
+
+
+Category is a util class, contains a label, its display name, a float
+value as score, and the index of the label in the corresponding label file.
+Typically it's used as the result of classification tasks.
+
+
+
+
+
+
+
Attributes
+
+
+
+`index`
+
+
+The index of the label in the corresponding label file.
+
+
+
+`score`
+
+
+The probability score of this label category.
+
+
+
+`display_name`
+
+
+The display name of the label, which may be translated for
+different locales. For example, a label, "apple", may be translated into
+Spanish for display purpose, so that the `display_name` is "manzana".
+
+The locale to use for display names specified through
+the TFLite Model Metadata.
+
+
+
+`max_results`
+
+
+The maximum number of top-scored classification results to
+return.
+
+
+
+`score_threshold`
+
+
+Overrides the ones provided in the model metadata. Results
+below this value are rejected.
+
+
+
+`category_name_allowlist`
+
+
+If non-empty, classifications whose class name is
+not in this set will be filtered out. Duplicate or unknown class names are
+ignored. Mutually exclusive with `category_name_denylist`.
+
+
+
+`category_name_denylist`
+
+
+If non-empty, classifications whose class name is in
+this set will be filtered out. Duplicate or unknown class names are
+ignored. Mutually exclusive with `category_name_allowlist`.
+
+
+
+
+
+
+For each pixel, the value indicates the prediction confidence usually
+in the [0, 1] range where higher values represent a stronger confidence.
+Ultimately this is model specific, and other range of values might be used.
+
+
+
+
+
+
+
Attributes
+
+
+
+`value`
+
+
+A NumPy 2D-array indicating the prediction confidence values usually
+in the range [0, 1].
+
+The locale to use for display names specified through
+the TFLite Model Metadata.
+
+
+
+`max_results`
+
+
+The maximum number of top-scored classification results to
+return.
+
+
+
+`score_threshold`
+
+
+Overrides the ones provided in the model metadata. Results
+below this value are rejected.
+
+
+
+`category_name_allowlist`
+
+
+If non-empty, classifications whose class name is
+not in this set will be filtered out. Duplicate or unknown class names are
+ignored. Mutually exclusive with `category_name_denylist`.
+
+
+
+`category_name_denylist`
+
+
+If non-empty, classifications whose class name is in
+this set will be filtered out. Duplicate or unknown class names are
+ignored. Mutually exclusive with `category_name_allowlist`.
+
+
+Checks if this object is equal to the given object.
+
+
+
+
+
+
Args
+
+
+
+`other`
+
+
+The object to be compared with.
+
+
+
+
+
+
+
+
+
+
Returns
+
+
+True if the objects are equal.
+
+
+
+
diff --git a/site/en/lite/api_docs/python/tflite_support/task/processor/Embedding.md b/site/en/lite/api_docs/python/tflite_support/task/processor/Embedding.md
new file mode 100644
index 00000000000..c5f46137dc1
--- /dev/null
+++ b/site/en/lite/api_docs/python/tflite_support/task/processor/Embedding.md
@@ -0,0 +1,114 @@
+page_type: reference
+description: Result produced by one of the embedder model output layers.
+
+
+
+
+
+
+
+Whether to normalize the returned feature vector with L2 norm.
+Use this option only if the model does not already contain a native
+L2_NORMALIZATION TF Lite Op. In most cases, this is already the case and
+L2 norm is thus achieved through TF Lite inference.
+
+
+
+`quantize`
+
+
+Whether the returned embedding should be quantized to bytes via
+scalar quantization. Embeddings are implicitly assumed to be unit-norm and
+therefore any dimension is guaranteed to have a value in [-1.0, 1.0]. Use
+the l2_normalize option if this is not the case.
+
+The embeddings produced by each of the model output layers.
+Except in advanced cases, the embedding model has a single output layer,
+and this list is thus made of a single element feature vector.
+
+
+
+
+
+
+Only one of the two fields is ever present.
+Feature vectors are assumed to be one-dimensional and L2-normalized.
+
+
+
+
+
+
+
Attributes
+
+
+
+`value`
+
+
+A NumPy array indidcating the raw output of the embedding layer. The
+datatype of elements in the array can be either float or uint8 if
+`quantize` is set to True in `EmbeddingOptions`.
+
+
+
+
+
+
+The index file to search into. Mandatory only if the index is not attached
+to the output tensor metadata as an AssociatedFile with type SCANN_INDEX_FILE.
+The index file can be specified by one of the following two ways:
+
+(1) file contents loaded in `index_file_content`.
+(2) file path in `index_file_name`.
+
+If more than one field of these fields is provided, they are used in this
+precedence order.
+
+
+
+
+
+
+
Attributes
+
+
+
+`index_file_name`
+
+
+Path to the index.
+
+
+
+`index_file_content`
+
+
+The index file contents as bytes.
+
+
+
+`max_results`
+
+
+Maximum number of nearest neighbor results to return.
+
+
+Checks if this object is equal to the given object.
+
+
+
+
+
+
Args
+
+
+
+`other`
+
+
+The object to be compared with.
+
+
+
+
+
+
+
+
+
+
Returns
+
+
+True if the objects are equal.
+
+
+
+
+
+
+
+
+
+
+
+
+
+
+
Class Variables
+
+
+
+index_file_content
+
+
+`None`
+
+
+
+index_file_name
+
+
+`None`
+
+
+
+max_results
+
+
+`5`
+
+
+
diff --git a/site/en/lite/api_docs/python/tflite_support/task/processor/SearchResult.md b/site/en/lite/api_docs/python/tflite_support/task/processor/SearchResult.md
new file mode 100644
index 00000000000..dabf4ad1d83
--- /dev/null
+++ b/site/en/lite/api_docs/python/tflite_support/task/processor/SearchResult.md
@@ -0,0 +1,106 @@
+page_type: reference
+description: Results from a search as a list of nearest neigbors.
+
+
+
+
+
+
+
+
+
+
+
+
+Note that at the time, a single `Segmentation` element is expected to be
+returned; the field is made repeated for later extension to e.g. instance
+segmentation models, which may return one segmentation per object.
+
+
+
+
+
+
+
+
+TensorFlow Lite Task Library Text APIs.
+
+
+This module provides interface to run TensorFlow Lite natural language
+processing models.
+
+## Classes
+
+[`class BertCluAnnotator`](../../tflite_support/task/text/BertCluAnnotator): Class that performs Bert CLU Annotation on text.
+
+[`class BertCluAnnotatorOptions`](../../tflite_support/task/text/BertCluAnnotatorOptions): Options for the Bert CLU Annotator task.
+
+[`class BertNLClassifier`](../../tflite_support/task/text/BertNLClassifier): Class that performs Bert NL classification on text.
+
+[`class BertNLClassifierOptions`](../../tflite_support/task/text/BertNLClassifierOptions): Options for the Bert NL classifier task.
+
+[`class BertQuestionAnswerer`](../../tflite_support/task/text/BertQuestionAnswerer): Class that performs Bert question answering on text.
+
+[`class BertQuestionAnswererOptions`](../../tflite_support/task/text/BertQuestionAnswererOptions): Options for the Bert question answerer task.
+
+[`class NLClassifier`](../../tflite_support/task/text/NLClassifier): Class that performs NL classification on text.
+
+[`class NLClassifierOptions`](../../tflite_support/task/text/NLClassifierOptions): Options for the NL classifier task.
+
+[`class TextEmbedder`](../../tflite_support/task/text/TextEmbedder): Class that performs dense feature vector extraction on text.
+
+[`class TextEmbedderOptions`](../../tflite_support/task/text/TextEmbedderOptions): Options for the text embedder task.
+
+[`class TextSearcher`](../../tflite_support/task/text/TextSearcher): Class to performs text search.
+
+[`class TextSearcherOptions`](../../tflite_support/task/text/TextSearcherOptions): Options for the text search task.
diff --git a/site/en/lite/api_docs/python/tflite_support/task/text/BertCluAnnotator.md b/site/en/lite/api_docs/python/tflite_support/task/text/BertCluAnnotator.md
new file mode 100644
index 00000000000..086fd5d6a30
--- /dev/null
+++ b/site/en/lite/api_docs/python/tflite_support/task/text/BertCluAnnotator.md
@@ -0,0 +1,272 @@
+page_type: reference
+description: Class that performs Bert CLU Annotation on text.
+
+
+
+
+
+
+
+
+Creates the `NLClassifier` object from NL classifier options.
+
+
+
+
+
+
Args
+
+
+
+`options`
+
+
+Options for the NL classifier task.
+
+
+
+
+
+
+
+
+
+
Returns
+
+
+`NLClassifier` object that's created from `options`.
+
+
+
+
+
+
+
+
+
+
+
Raises
+
+
+
+`ValueError`
+
+
+If failed to create `NLClassifier` object from
+`NLClassifierOptions` such as missing the model or if any of the
+classification options is invalid.
+
+
+
+`RuntimeError`
+
+
+If other types of error occurred.
+
+
+
diff --git a/site/en/lite/api_docs/python/tflite_support/task/text/NLClassifierOptions.md b/site/en/lite/api_docs/python/tflite_support/task/text/NLClassifierOptions.md
new file mode 100644
index 00000000000..6c8e76fba11
--- /dev/null
+++ b/site/en/lite/api_docs/python/tflite_support/task/text/NLClassifierOptions.md
@@ -0,0 +1,71 @@
+page_type: reference
+description: Options for the NL classifier task.
+
+
+
+
+
+
+
+
+
+
+
+
+It works by performing embedding extraction on text, followed by
+nearest-neighbor search in an index of embeddings through ScaNN.
+
+
+
+
+
+
+Search for text with similar semantic meaning.
+
+This method performs actual feature extraction on the provided text input,
+followed by nearest-neighbor search in the index.
+
+
+
+
+
+
+TensorFlow Lite Task Library Vision APIs.
+
+
+This module provides interface to run TensorFlow Lite computer vision models.
+
+## Classes
+
+[`class ImageClassifier`](../../tflite_support/task/vision/ImageClassifier): Class that performs classification on images.
+
+[`class ImageClassifierOptions`](../../tflite_support/task/vision/ImageClassifierOptions): Options for the image classifier task.
+
+[`class ImageEmbedder`](../../tflite_support/task/vision/ImageEmbedder): Class that performs dense feature vector extraction on images.
+
+[`class ImageEmbedderOptions`](../../tflite_support/task/vision/ImageEmbedderOptions): Options for the image embedder task.
+
+[`class ImageSearcher`](../../tflite_support/task/vision/ImageSearcher): Class to performs image search.
+
+[`class ImageSearcherOptions`](../../tflite_support/task/vision/ImageSearcherOptions): Options for the image search task.
+
+[`class ImageSegmenter`](../../tflite_support/task/vision/ImageSegmenter): Class that performs segmentation on images.
+
+[`class ImageSegmenterOptions`](../../tflite_support/task/vision/ImageSegmenterOptions): Options for the image segmenter task.
+
+[`class ObjectDetector`](../../tflite_support/task/vision/ObjectDetector): Class that performs object detection on images.
+
+[`class ObjectDetectorOptions`](../../tflite_support/task/vision/ObjectDetectorOptions): Options for the object detector task.
+
+[`class TensorImage`](../../tflite_support/task/vision/TensorImage): Wrapper class for the Image object.
diff --git a/site/en/lite/api_docs/python/tflite_support/task/vision/ImageClassifier.md b/site/en/lite/api_docs/python/tflite_support/task/vision/ImageClassifier.md
new file mode 100644
index 00000000000..fdc71b4afe9
--- /dev/null
+++ b/site/en/lite/api_docs/python/tflite_support/task/vision/ImageClassifier.md
@@ -0,0 +1,283 @@
+page_type: reference
+description: Class that performs classification on images.
+
+
+
+
+
+
+
+
+Performs classification on the provided TensorImage.
+
+
+
+
+
+
Args
+
+
+
+`image`
+
+
+Tensor image, used to extract the feature vectors.
+
+
+
+`bounding_box`
+
+
+Bounding box, optional. If set, performed feature vector
+extraction only on the provided region of interest. Note that the region
+of interest is not clamped, so this method will fail if the region is
+out of bounds of the input image.
+
+
+Performs actual feature vector extraction on the provided TensorImage.
+
+
+
+
+
+
Args
+
+
+
+`image`
+
+
+Tensor image, used to extract the feature vectors.
+
+
+
+`bounding_box`
+
+
+Bounding box, optional. If set, performed feature vector
+extraction only on the provided region of interest. Note that the region
+of interest is not clamped, so this method will fail if the region is
+out of bounds of the input image.
+
+
+Gets the embedding in the embedding result by `output_index`.
+
+
+
+
+
+
Args
+
+
+
+`result`
+
+
+embedding result.
+
+
+
+`output_index`
+
+
+output index of the output layer.
+
+
+
+
+
+
+
+
+
+
Returns
+
+
+The Embedding output by the output_index'th layer. In (the most common)
+case where a single embedding is produced, you can just call
+get_feature_vector_by_index(result, 0).
+
+
+
+
+
+
+
+
+
+
+
Raises
+
+
+ValueError if the output index is out of bound.
+
+
+
+
+
+
+It works by performing embedding extraction on images, followed by
+nearest-neighbor search in an index of embeddings through ScaNN.
+
+
+
+
+
+
+Search for image with similar semantic meaning.
+
+This method performs actual feature extraction on the provided image input,
+followed by nearest-neighbor search in the index.
+
+
+
+
+
Args
+
+
+
+`image`
+
+
+Tensor image, used to extract the feature vectors.
+
+
+
+`bounding_box`
+
+
+Bounding box, optional. If set, performed feature vector
+extraction only on the provided region of interest. Note that the region
+of interest is not clamped, so this method will fail if the region is
+out of bounds of the input image.
+
+
+
+
+
+
+
+
+
+
Returns
+
+
+Search result.
+
+
+
+
+
+
+
+
+
+
+
Raises
+
+
+
+`ValueError`
+
+
+If any of the input arguments is invalid.
+
+
+
+`RuntimeError`
+
+
+If failed to perform nearest-neighbor search.
+
+
+
diff --git a/site/en/lite/api_docs/python/tflite_support/task/vision/ImageSearcherOptions.md b/site/en/lite/api_docs/python/tflite_support/task/vision/ImageSearcherOptions.md
new file mode 100644
index 00000000000..9b4ff75ac84
--- /dev/null
+++ b/site/en/lite/api_docs/python/tflite_support/task/vision/ImageSearcherOptions.md
@@ -0,0 +1,87 @@
+page_type: reference
+description: Options for the image search task.
+
+
+
+
+
+
+
+boolean, whether `image_data` is loaded from
+numpy array. if False, it means that `image_data` is loaded from
+stbi_load** function in C++ and need to free the storage of ImageData in
+the destructor.
+
+
+
+
+
+
+
+
+
+
+
+
Attributes
+
+
+
+`buffer`
+
+
+Gets the numpy array that represents `self.image_data`.
+
+
+Creates `TensorImage` object from the numpy array.
+
+
+
+
+
+
Args
+
+
+
+`array`
+
+
+numpy array with dtype=uint8. Its shape should be either (h, w, 3)
+or (1, h, w, 3) for RGB images, either (h, w) or (1, h, w) for GRAYSCALE
+images and either (h, w, 4) or (1, h, w, 4) for RGBA images.
+
+
+
+
+
+
+
+
+
+
Returns
+
+
+`TensorImage` object.
+
+
+
+
+
+
+
+
+
+
+
Raises
+
+
+ValueError if the dytype of the numpy array is not `uint8` or the
+dimention is not the valid dimention.
+
Creates a new instance configured with the given options. Returns nil if the underlying
+Core ML delegate could not be created because Options.enabledDevices was set to
+neuralEngine but the device does not have the Neural Engine.
A type indicating which devices the Core ML delegate should be enabled for. The default
+value is .neuralEngine indicating that the delegate is enabled for Neural Engine devices
+only.
The maximum number of Core ML delegate partitions created. Each graph corresponds to one
+delegated node subset in the TFLite model. The default value is 0 indicating that all
+possible partitions are delegated.
+ An error if the index is invalid, tensors haven’t been allocated, or interpreter
+has not been invoked for models that dynamically compute output tensors based on the
+values of its input tensors.
+
+
Resizes the input Tensor at the given index to the specified Tensor.Shape.
+
+
Note
+ After resizing an input tensor, the client must explicitly call
+allocateTensors() before attempting to access the resized tensor data or invoking the
+interpreter to perform inference.
+
+
+
Throws
+ An error if the input tensor at the given index could not be resized.
+
+
The maximum number of CPU threads that the interpreter should run on. The default is nil
+indicating that the Interpreter will decide the number of threads to use.
Indicates whether an optimized set of floating point CPU kernels, provided by XNNPACK, is
+enabled.
+
+
Experiment
+
Enabling this flag will enable use of a new, highly optimized set of CPU kernels provided
+via the XNNPACK delegate. Currently, this is restricted to a subset of floating point
+operations. Eventually, we plan to enable this by default, as it can provide significant
+performance benefits for many classes of floating point models. See
+https://github.com/tensorflow/tensorflow/blob/master/tensorflow/lite/delegates/xnnpack/README.md
+for more details.
+
+
+
Important
+
Things to keep in mind when enabling this flag:
+
+
+
Startup time and resize time may increase.
+
Baseline memory consumption may increase.
+
Compatibility with other delegates (e.g., GPU) has not been fully validated.
+
Quantized models will not see any benefit.
+
+
+
+
Warning
+
This is an experimental interface that is subject to change.
Parameters that determine the mapping of quantized values to real values. Quantized values can
+be mapped to float values using the following conversion:
+realValue = scale * (quantizedValue - zeroPoint).
Parameters that determine the mapping of quantized values to real values. Quantized values can
+be mapped to float values using the following conversion:
+realValue = scale * (quantizedValue - zeroPoint).
A type alias for Interpreter.Options to support backwards compatibility with the deprecated"},"Structs/Tensor/Shape.html#/s:19TensorFlowLiteSwift0A0V5ShapeV4rankSivp":{"name":"rank","abstract":"
A string describing the semantic versioning information for the runtime. Is an empty string if","parent_name":"Runtime"},"Enums/ThreadWaitType.html#/s:19TensorFlowLiteSwift14ThreadWaitTypeO4noneyA2CmF":{"name":"none","abstract":"
The thread does not wait for the work to complete. Useful when the output of the work is used","parent_name":"ThreadWaitType"},"Enums/ThreadWaitType.html#/s:19TensorFlowLiteSwift14ThreadWaitTypeO7passiveyA2CmF":{"name":"passive","abstract":"
The thread waits for the work to complete with minimal latency, which may require additional","parent_name":"ThreadWaitType"},"Enums/ThreadWaitType.html#/s:19TensorFlowLiteSwift14ThreadWaitTypeO10aggressiveyA2CmF":{"name":"aggressive","abstract":"
The thread waits for the work while trying to prevent the GPU from going into sleep mode.
Indicates whether the GPU delegate allows precision loss, such as allowing Float16","parent_name":"Options"},"Classes/MetalDelegate/Options.html#/s:19TensorFlowLiteSwift13MetalDelegateC7OptionsV19allowsPrecisionLossSbvp":{"name":"allowsPrecisionLoss","abstract":"
A type indicating how the current thread should wait for work on the GPU to complete. The","parent_name":"Options"},"Classes/MetalDelegate/Options.html#/s:19TensorFlowLiteSwift13MetalDelegateC7OptionsV21isQuantizationEnabledSbvp":{"name":"isQuantizationEnabled","abstract":"
Indicates whether the GPU delegate allows execution of an 8-bit quantized model. The default","parent_name":"Options"},"Classes/MetalDelegate/Options.html#/s:19TensorFlowLiteSwift13MetalDelegateC7OptionsVAEycfc":{"name":"init()","abstract":"
The maximum number of CPU threads that the interpreter should run on. The default is nil","parent_name":"Options"},"Classes/Interpreter/Options.html#/s:19TensorFlowLiteSwift11InterpreterC7OptionsV16isXNNPackEnabledSbvp":{"name":"isXNNPackEnabled","abstract":"
Indicates whether an optimized set of floating point CPU kernels, provided by XNNPACK, is","parent_name":"Options"},"Classes/Interpreter/Options.html#/s:19TensorFlowLiteSwift11InterpreterC7OptionsVAEycfc":{"name":"init()","abstract":"
A type indicating which devices the Core ML delegate should be enabled for. The default","parent_name":"Options"},"Classes/CoreMLDelegate/Options.html#/s:19TensorFlowLiteSwift14CoreMLDelegateC7OptionsV13coreMLVersionSivp":{"name":"coreMLVersion","abstract":"
Target Core ML version for the model conversion. When it’s not set, Core ML version will","parent_name":"Options"},"Classes/CoreMLDelegate/Options.html#/s:19TensorFlowLiteSwift14CoreMLDelegateC7OptionsV22maxDelegatedPartitionsSivp":{"name":"maxDelegatedPartitions","abstract":"
The maximum number of Core ML delegate partitions created. Each graph corresponds to one","parent_name":"Options"},"Classes/CoreMLDelegate/Options.html#/s:19TensorFlowLiteSwift14CoreMLDelegateC7OptionsV20minNodesPerPartitionSivp":{"name":"minNodesPerPartition","abstract":"
The minimum number of nodes per partition to be delegated by the Core ML delegate. The","parent_name":"Options"},"Classes/CoreMLDelegate/Options.html#/s:19TensorFlowLiteSwift14CoreMLDelegateC7OptionsVAEycfc":{"name":"init()","abstract":"
Creates a new instance configured with the given options. Returns nil if the underlying","parent_name":"CoreMLDelegate"},"Classes/CoreMLDelegate/EnabledDevices.html":{"name":"EnabledDevices","abstract":"
A type indicating which devices the Core ML delegate should be enabled for.
The following type aliases are available globally.
"}}
\ No newline at end of file
diff --git a/site/en/r1/guide/autograph.ipynb b/site/en/r1/guide/autograph.ipynb
index f028b33ce9f..64d631a52b3 100644
--- a/site/en/r1/guide/autograph.ipynb
+++ b/site/en/r1/guide/autograph.ipynb
@@ -78,7 +78,7 @@
"id": "CydFK2CL7ZHA"
},
"source": [
- "[AutoGraph](https://github.com/tensorflow/tensorflow/blob/master/tensorflow/python/autograph/) helps you write complicated graph code using normal Python. Behind the scenes, AutoGraph automatically transforms your code into the equivalent [TensorFlow graph code](https://www.tensorflow.org/r1/guide/graphs). AutoGraph already supports much of the Python language, and that coverage continues to grow. For a list of supported Python language features, see the [Autograph capabilities and limitations](https://github.com/tensorflow/tensorflow/blob/master/tensorflow/python/autograph/g3doc/reference/limitations.md)."
+ "[AutoGraph](https://github.com/tensorflow/tensorflow/blob/r1.15/tensorflow/python/autograph/) helps you write complicated graph code using normal Python. Behind the scenes, AutoGraph automatically transforms your code into the equivalent [TensorFlow graph code](https://www.tensorflow.org/r1/guide/graphs). AutoGraph already supports much of the Python language, and that coverage continues to grow. For a list of supported Python language features, see the [Autograph capabilities and limitations](https://github.com/tensorflow/tensorflow/blob/r1.15/tensorflow/python/autograph/g3doc/reference/limitations.md)."
]
},
{
@@ -241,7 +241,7 @@
"id": "m-jWmsCmByyw"
},
"source": [
- "AutoGraph supports common Python statements like `while`, `for`, `if`, `break`, and `return`, with support for nesting. Compare this function with the complicated graph verson displayed in the following code blocks:"
+ "AutoGraph supports common Python statements like `while`, `for`, `if`, `break`, and `return`, with support for nesting. Compare this function with the complicated graph version displayed in the following code blocks:"
]
},
{
diff --git a/site/en/r1/guide/custom_estimators.md b/site/en/r1/guide/custom_estimators.md
index 87dce26a0dc..7bbf3573909 100644
--- a/site/en/r1/guide/custom_estimators.md
+++ b/site/en/r1/guide/custom_estimators.md
@@ -592,10 +592,10 @@ function for custom Estimators; everything else is the same.
For more details, be sure to check out:
* The
- [official TensorFlow implementation of MNIST](https://github.com/tensorflow/models/tree/master/official/r1/mnist),
+ [official TensorFlow implementation of MNIST](https://github.com/tensorflow/models/tree/r1.15/official/r1/mnist),
which uses a custom estimator.
* The TensorFlow
- [official models repository](https://github.com/tensorflow/models/tree/master/official),
+ [official models repository](https://github.com/tensorflow/models/tree/r1.15/official),
which contains more curated examples using custom estimators.
* This [TensorBoard video](https://youtu.be/eBbEDRsCmv4), which introduces
TensorBoard.
diff --git a/site/en/r1/guide/datasets.md b/site/en/r1/guide/datasets.md
index b1ed1b6e113..d7c38bf2f92 100644
--- a/site/en/r1/guide/datasets.md
+++ b/site/en/r1/guide/datasets.md
@@ -437,7 +437,7 @@ dataset = dataset.batch(32)
iterator = dataset.make_initializable_iterator()
# You can feed the initializer with the appropriate filenames for the current
-# phase of execution, e.g. training vs. validation.
+# phase of execution, e.g., training vs. validation.
# Initialize `iterator` with training data.
training_filenames = ["/var/data/file1.tfrecord", "/var/data/file2.tfrecord"]
@@ -639,7 +639,7 @@ TODO(mrry): Add this section.
The simplest form of batching stacks `n` consecutive elements of a dataset into
a single element. The `Dataset.batch()` transformation does exactly this, with
the same constraints as the `tf.stack()` operator, applied to each component
-of the elements: i.e. for each component *i*, all elements must have a tensor
+of the elements: i.e., for each component *i*, all elements must have a tensor
of the exact same shape.
```python
diff --git a/site/en/r1/guide/debugger.md b/site/en/r1/guide/debugger.md
index 2b4b6497ec4..963765b97db 100644
--- a/site/en/r1/guide/debugger.md
+++ b/site/en/r1/guide/debugger.md
@@ -10,7 +10,7 @@ due to TensorFlow's computation-graph paradigm.
This guide focuses on the command-line interface (CLI) of `tfdbg`. For guide on
how to use the graphical user interface (GUI) of tfdbg, i.e., the
**TensorBoard Debugger Plugin**, please visit
-[its README](https://github.com/tensorflow/tensorboard/blob/master/tensorboard/plugins/debugger/README.md).
+[its README](https://github.com/tensorflow/tensorboard/blob/r1.15/tensorboard/plugins/debugger/README.md).
Note: The TensorFlow debugger uses a
[curses](https://en.wikipedia.org/wiki/Curses_\(programming_library\))-based text
@@ -35,7 +35,7 @@ TensorFlow. Later sections of this document describe how to use **tfdbg** with
higher-level APIs of TensorFlow, including `tf.estimator`, `tf.keras` / `keras`
and `tf.contrib.slim`. To *observe* such an issue, run the following command
without the debugger (the source code can be found
-[here](https://github.com/tensorflow/tensorflow/blob/master/tensorflow/python/debug/examples/v1/debug_mnist.py)):
+[here](https://github.com/tensorflow/tensorflow/blob/r1.15/tensorflow/python/debug/examples/v1/debug_mnist.py)):
python -m tensorflow.python.debug.examples.v1.debug_mnist
@@ -64,7 +64,7 @@ numeric problem first surfaced.
To add support for tfdbg in our example, all that is needed is to add the
following lines of code and wrap the Session object with a debugger wrapper.
This code is already added in
-[debug_mnist.py](https://github.com/tensorflow/tensorflow/blob/master/tensorflow/python/debug/examples/v1/debug_mnist.py),
+[debug_mnist.py](https://github.com/tensorflow/tensorflow/blob/r1.15/tensorflow/python/debug/examples/v1/debug_mnist.py),
so you can activate tfdbg CLI with the `--debug` flag at the command line.
```python
@@ -370,7 +370,7 @@ traceback of the node's construction.
From the traceback, you can see that the op is constructed at the following
line:
-[`debug_mnist.py`](https://www.tensorflow.org/code/tensorflow/python/debug/examples/v1/debug_mnist.py):
+[`debug_mnist.py`](https://github.com/tensorflow/tensorflow/blob/r1.15/tensorflow/python/debug/examples/v1/debug_mnist.py):
```python
diff = y_ * tf.log(y)
@@ -457,7 +457,7 @@ accuracy_score = classifier.evaluate(eval_input_fn,
predict_results = classifier.predict(predict_input_fn, hooks=hooks)
```
-[debug_tflearn_iris.py](https://www.tensorflow.org/code/tensorflow/python/debug/examples/v1/debug_tflearn_iris.py),
+[debug_tflearn_iris.py](https://github.com/tensorflow/tensorflow/blob/r1.15/tensorflow/python/debug/examples/v1/debug_tflearn_iris.py),
contains a full example of how to use the tfdbg with `Estimator`s. To run this
example, do:
@@ -501,7 +501,7 @@ TensorFlow backend. You just need to replace `tf.keras.backend` with
## Debugging tf-slim with TFDBG
TFDBG supports debugging of training and evaluation with
-[tf-slim](https://github.com/tensorflow/tensorflow/tree/master/tensorflow/contrib/slim).
+[tf-slim](https://github.com/tensorflow/tensorflow/tree/r1.15/tensorflow/contrib/slim).
As detailed below, training and evaluation require slightly different debugging
workflows.
@@ -605,7 +605,7 @@ The `watch_fn` argument accepts a `Callable` that allows you to configure what
If your model code is written in C++ or other languages, you can also
modify the `debug_options` field of `RunOptions` to generate debug dumps that
can be inspected offline. See
-[the proto definition](https://www.tensorflow.org/code/tensorflow/core/protobuf/debug.proto)
+[the proto definition](https://github.com/tensorflow/tensorflow/blob/r1.15/tensorflow/core/protobuf/debug.proto)
for more details.
### Debugging Remotely-Running Estimators
@@ -648,7 +648,7 @@ python -m tensorflow.python.debug.cli.offline_analyzer \
model, check out
1. The profiling mode of tfdbg: `tfdbg> run -p`.
- 2. [tfprof](https://github.com/tensorflow/tensorflow/tree/master/tensorflow/core/profiler)
+ 2. [tfprof](https://github.com/tensorflow/tensorflow/tree/r1.15/tensorflow/core/profiler)
and other profiling tools for TensorFlow.
**Q**: _How do I link tfdbg against my `Session` in Bazel? Why do I see an
@@ -808,4 +808,4 @@ tensor dumps.
and conditional breakpoints, and tying tensors to their
graph-construction source code, all in the browser environment.
To get started, please visit
- [its README](https://github.com/tensorflow/tensorboard/blob/master/tensorboard/plugins/debugger/README.md).
+ [its README](https://github.com/tensorflow/tensorboard/blob/r1.15/tensorboard/plugins/debugger/README.md).
diff --git a/site/en/r1/guide/distribute_strategy.ipynb b/site/en/r1/guide/distribute_strategy.ipynb
index 79d6293eba7..4dd502d331b 100644
--- a/site/en/r1/guide/distribute_strategy.ipynb
+++ b/site/en/r1/guide/distribute_strategy.ipynb
@@ -118,7 +118,7 @@
"## Types of strategies\n",
"`tf.distribute.Strategy` intends to cover a number of use cases along different axes. Some of these combinations are currently supported and others will be added in the future. Some of these axes are:\n",
"\n",
- "* Syncronous vs asynchronous training: These are two common ways of distributing training with data parallelism. In sync training, all workers train over different slices of input data in sync, and aggregating gradients at each step. In async training, all workers are independently training over the input data and updating variables asynchronously. Typically sync training is supported via all-reduce and async through parameter server architecture.\n",
+ "* Synchronous vs asynchronous training: These are two common ways of distributing training with data parallelism. In sync training, all workers train over different slices of input data in sync, and aggregating gradients at each step. In async training, all workers are independently training over the input data and updating variables asynchronously. Typically sync training is supported via all-reduce and async through parameter server architecture.\n",
"* Hardware platform: Users may want to scale their training onto multiple GPUs on one machine, or multiple machines in a network (with 0 or more GPUs each), or on Cloud TPUs.\n",
"\n",
"In order to support these use cases, we have 4 strategies available. In the next section we will talk about which of these are supported in which scenarios in TF."
@@ -245,7 +245,7 @@
"\n",
"`tf.distribute.experimental.MultiWorkerMirroredStrategy` is very similar to `MirroredStrategy`. It implements synchronous distributed training across multiple workers, each with potentially multiple GPUs. Similar to `MirroredStrategy`, it creates copies of all variables in the model on each device across all workers.\n",
"\n",
- "It uses [CollectiveOps](https://github.com/tensorflow/tensorflow/blob/master/tensorflow/python/ops/collective_ops.py) as the multi-worker all-reduce communication method used to keep variables in sync. A collective op is a single op in the TensorFlow graph which can automatically choose an all-reduce algorithm in the TensorFlow runtime according to hardware, network topology and tensor sizes.\n",
+ "It uses [CollectiveOps](https://github.com/tensorflow/tensorflow/blob/r1.15/tensorflow/python/ops/collective_ops.py) as the multi-worker all-reduce communication method used to keep variables in sync. A collective op is a single op in the TensorFlow graph which can automatically choose an all-reduce algorithm in the TensorFlow runtime according to hardware, network topology and tensor sizes.\n",
"\n",
"It also implements additional performance optimizations. For example, it includes a static optimization that converts multiple all-reductions on small tensors into fewer all-reductions on larger tensors. In addition, we are designing it to have a plugin architecture - so that in the future, users will be able to plugin algorithms that are better tuned for their hardware. Note that collective ops also implement other collective operations such as broadcast and all-gather.\n",
"\n",
@@ -371,7 +371,7 @@
"id": "hQv1lm9UPDFy"
},
"source": [
- "So far we've talked about what are the different stategies available and how you can instantiate them. In the next few sections, we will talk about the different ways in which you can use them to distribute your training. We will show short code snippets in this guide and link off to full tutorials which you can run end to end."
+ "So far we've talked about what are the different strategies available and how you can instantiate them. In the next few sections, we will talk about the different ways in which you can use them to distribute your training. We will show short code snippets in this guide and link off to full tutorials which you can run end to end."
]
},
{
@@ -490,8 +490,8 @@
"Here is a list of tutorials and examples that illustrate the above integration end to end with Keras:\n",
"\n",
"1. [Tutorial](../tutorials/distribute/keras.ipynb) to train MNIST with `MirroredStrategy`.\n",
- "2. Official [ResNet50](https://github.com/tensorflow/models/blob/master/official/vision/image_classification/resnet_imagenet_main.py) training with ImageNet data using `MirroredStrategy`.\n",
- "3. [ResNet50](https://github.com/tensorflow/tpu/blob/master/models/experimental/resnet50_keras/resnet50.py) trained with Imagenet data on Cloud TPus with `TPUStrategy`."
+ "2. Official [ResNet50](https://github.com/tensorflow/models/blob/r1.15/official/vision/image_classification/resnet_imagenet_main.py) training with ImageNet data using `MirroredStrategy`.\n",
+ "3. [ResNet50](https://github.com/tensorflow/tpu/blob/1.15/models/experimental/resnet50_keras/resnet50.py) trained with Imagenet data on Cloud TPus with `TPUStrategy`."
]
},
{
@@ -595,9 +595,9 @@
"### Examples and Tutorials\n",
"Here are some examples that show end to end usage of various strategies with Estimator:\n",
"\n",
- "1. [End to end example](https://github.com/tensorflow/ecosystem/tree/master/distribution_strategy) for multi worker training in tensorflow/ecosystem using Kuberentes templates. This example starts with a Keras model and converts it to an Estimator using the `tf.keras.estimator.model_to_estimator` API.\n",
- "2. Official [ResNet50](https://github.com/tensorflow/models/blob/master/official/r1/resnet/imagenet_main.py) model, which can be trained using either `MirroredStrategy` or `MultiWorkerMirroredStrategy`.\n",
- "3. [ResNet50](https://github.com/tensorflow/tpu/blob/master/models/experimental/distribution_strategy/resnet_estimator.py) example with TPUStrategy."
+ "1. [End to end example](https://github.com/tensorflow/ecosystem/tree/r1.15/distribution_strategy) for multi worker training in tensorflow/ecosystem using Kuberentes templates. This example starts with a Keras model and converts it to an Estimator using the `tf.keras.estimator.model_to_estimator` API.\n",
+ "2. Official [ResNet50](https://github.com/tensorflow/models/blob/r1.15/official/r1/resnet/imagenet_main.py) model, which can be trained using either `MirroredStrategy` or `MultiWorkerMirroredStrategy`.\n",
+ "3. [ResNet50](https://github.com/tensorflow/tpu/blob/1.15/models/experimental/distribution_strategy/resnet_estimator.py) example with TPUStrategy."
]
},
{
@@ -607,7 +607,7 @@
},
"source": [
"## Using `tf.distribute.Strategy` with custom training loops\n",
- "As you've seen, using `tf.distrbute.Strategy` with high level APIs is only a couple lines of code change. With a little more effort, `tf.distrbute.Strategy` can also be used by other users who are not using these frameworks.\n",
+ "As you've seen, using `tf.distribute.Strategy` with high level APIs is only a couple lines of code change. With a little more effort, `tf.distribute.Strategy` can also be used by other users who are not using these frameworks.\n",
"\n",
"TensorFlow is used for a wide variety of use cases and some users (such as researchers) require more flexibility and control over their training loops. This makes it hard for them to use the high level frameworks such as Estimator or Keras. For instance, someone using a GAN may want to take a different number of generator or discriminator steps each round. Similarly, the high level frameworks are not very suitable for Reinforcement Learning training. So these users will usually write their own training loops.\n",
"\n",
diff --git a/site/en/r1/guide/eager.ipynb b/site/en/r1/guide/eager.ipynb
index 6a0a78c2443..f76acb4b702 100644
--- a/site/en/r1/guide/eager.ipynb
+++ b/site/en/r1/guide/eager.ipynb
@@ -95,7 +95,7 @@
"\n",
"Eager execution supports most TensorFlow operations and GPU acceleration. For a\n",
"collection of examples running in eager execution, see:\n",
- "[tensorflow/contrib/eager/python/examples](https://github.com/tensorflow/tensorflow/tree/master/tensorflow/contrib/eager/python/examples).\n",
+ "[tensorflow/contrib/eager/python/examples](https://github.com/tensorflow/tensorflow/tree/r1.15/tensorflow/contrib/eager/python/examples).\n",
"\n",
"Note: Some models may experience increased overhead with eager execution\n",
"enabled. Performance improvements are ongoing, but please\n",
@@ -1160,7 +1160,7 @@
"### Benchmarks\n",
"\n",
"For compute-heavy models, such as\n",
- "[ResNet50](https://github.com/tensorflow/tensorflow/tree/master/tensorflow/contrib/eager/python/examples/resnet50)\n",
+ "[ResNet50](https://github.com/tensorflow/tensorflow/tree/r1.15/tensorflow/contrib/eager/python/examples/resnet50)\n",
"training on a GPU, eager execution performance is comparable to graph execution.\n",
"But this gap grows larger for models with less computation and there is work to\n",
"be done for optimizing hot code paths for models with lots of small operations."
@@ -1225,7 +1225,7 @@
"production deployment. Use `tf.train.Checkpoint` to save and restore model\n",
"variables, this allows movement between eager and graph execution environments.\n",
"See the examples in:\n",
- "[tensorflow/contrib/eager/python/examples](https://github.com/tensorflow/tensorflow/tree/master/tensorflow/contrib/eager/python/examples).\n"
+ "[tensorflow/contrib/eager/python/examples](https://github.com/tensorflow/tensorflow/tree/r1.15/tensorflow/contrib/eager/python/examples).\n"
]
},
{
diff --git a/site/en/r1/guide/extend/architecture.md b/site/en/r1/guide/extend/architecture.md
index 1f2ac53066f..0753824e15e 100644
--- a/site/en/r1/guide/extend/architecture.md
+++ b/site/en/r1/guide/extend/architecture.md
@@ -34,7 +34,7 @@ This document focuses on the following layers:
* **Client**:
* Defines the computation as a dataflow graph.
* Initiates graph execution using a [**session**](
- https://www.tensorflow.org/code/tensorflow/python/client/session.py).
+ https://github.com/tensorflow/tensorflow/blob/r1.15/tensorflow/python/client/session.py).
* **Distributed Master**
* Prunes a specific subgraph from the graph, as defined by the arguments
to Session.run().
@@ -144,8 +144,8 @@ The distributed master then ships the graph pieces to the distributed tasks.
### Code
-* [MasterService API definition](https://www.tensorflow.org/code/tensorflow/core/protobuf/master_service.proto)
-* [Master interface](https://www.tensorflow.org/code/tensorflow/core/distributed_runtime/master_interface.h)
+* [MasterService API definition](https://github.com/tensorflow/tensorflow/blob/r1.15/tensorflow/core/protobuf/master_service.proto)
+* [Master interface](https://github.com/tensorflow/tensorflow/blob/r1.15/tensorflow/core/distributed_runtime/master_interface.h)
## Worker Service
@@ -178,7 +178,7 @@ For transfers between tasks, TensorFlow uses multiple protocols, including:
We also have preliminary support for NVIDIA's NCCL library for multi-GPU
communication, see:
-[`tf.contrib.nccl`](https://github.com/tensorflow/tensorflow/blob/master/tensorflow/python/ops/nccl_ops.py).
+[`tf.contrib.nccl`](https://github.com/tensorflow/tensorflow/blob/r1.15/tensorflow/python/ops/nccl_ops.py).
@@ -186,9 +186,9 @@ communication, see:
### Code
-* [WorkerService API definition](https://www.tensorflow.org/code/tensorflow/core/protobuf/worker_service.proto)
-* [Worker interface](https://www.tensorflow.org/code/tensorflow/core/distributed_runtime/worker_interface.h)
-* [Remote rendezvous (for Send and Recv implementations)](https://www.tensorflow.org/code/tensorflow/core/distributed_runtime/rpc/rpc_rendezvous_mgr.h)
+* [WorkerService API definition](https://github.com/tensorflow/tensorflow/blob/r1.15/tensorflow/core/protobuf/worker_service.proto)
+* [Worker interface](https://github.com/tensorflow/tensorflow/blob/r1.15/tensorflow/core/distributed_runtime/worker_interface.h)
+* [Remote rendezvous (for Send and Recv implementations)](https://github.com/tensorflow/tensorflow/blob/r1.15/tensorflow/core/distributed_runtime/rpc/rpc_rendezvous_mgr.h)
## Kernel Implementations
@@ -199,7 +199,7 @@ Many of the operation kernels are implemented using Eigen::Tensor, which uses
C++ templates to generate efficient parallel code for multicore CPUs and GPUs;
however, we liberally use libraries like cuDNN where a more efficient kernel
implementation is possible. We have also implemented
-[quantization](https://github.com/tensorflow/tensorflow/blob/master/tensorflow/lite/g3doc/performance/post_training_quantization.md), which enables
+[quantization](https://github.com/tensorflow/tensorflow/blob/r1.15/tensorflow/lite/g3doc/performance/post_training_quantization.md), which enables
faster inference in environments such as mobile devices and high-throughput
datacenter applications, and use the
[gemmlowp](https://github.com/google/gemmlowp) low-precision matrix library to
@@ -215,4 +215,4 @@ experimental implementation of automatic kernel fusion.
### Code
-* [`OpKernel` interface](https://www.tensorflow.org/code/tensorflow/core/framework/op_kernel.h)
+* [`OpKernel` interface](https://github.com/tensorflow/tensorflow/blob/r1.15/tensorflow/core/framework/op_kernel.h)
diff --git a/site/en/r1/guide/extend/bindings.md b/site/en/r1/guide/extend/bindings.md
index 9c10e90840f..7daa2212106 100644
--- a/site/en/r1/guide/extend/bindings.md
+++ b/site/en/r1/guide/extend/bindings.md
@@ -112,11 +112,11 @@ There are a few ways to get a list of the `OpDef`s for the registered ops:
to interpret the `OpDef` messages.
- The C++ function `OpRegistry::Global()->GetRegisteredOps()` returns the same
list of all registered `OpDef`s (defined in
- [`tensorflow/core/framework/op.h`](https://www.tensorflow.org/code/tensorflow/core/framework/op.h)). This can be used to write the generator
+ [`tensorflow/core/framework/op.h`](https://github.com/tensorflow/tensorflow/blob/r1.15/tensorflow/core/framework/op.h)). This can be used to write the generator
in C++ (particularly useful for languages that do not have protocol buffer
support).
- The ASCII-serialized version of that list is periodically checked in to
- [`tensorflow/core/ops/ops.pbtxt`](https://www.tensorflow.org/code/tensorflow/core/ops/ops.pbtxt) by an automated process.
+ [`tensorflow/core/ops/ops.pbtxt`](https://github.com/tensorflow/tensorflow/blob/r1.15/tensorflow/core/ops/ops.pbtxt) by an automated process.
The `OpDef` specifies the following:
@@ -159,7 +159,7 @@ between the generated code and the `OpDef`s checked into the repository, but is
useful for languages where code is expected to be generated ahead of time like
`go get` for Go and `cargo ops` for Rust. At the other end of the spectrum, for
some languages the code could be generated dynamically from
-[`tensorflow/core/ops/ops.pbtxt`](https://www.tensorflow.org/code/tensorflow/core/ops/ops.pbtxt).
+[`tensorflow/core/ops/ops.pbtxt`](https://github.com/tensorflow/tensorflow/blob/r1.15/tensorflow/core/ops/ops.pbtxt).
#### Handling Constants
@@ -228,4 +228,4 @@ At this time, support for gradients, functions and control flow operations ("if"
and "while") is not available in languages other than Python. This will be
updated when the [C API] provides necessary support.
-[C API]: https://www.tensorflow.org/code/tensorflow/c/c_api.h
+[C API]: https://github.com/tensorflow/tensorflow/blob/r1.15/tensorflow/c/c_api.h
diff --git a/site/en/r1/guide/extend/filesystem.md b/site/en/r1/guide/extend/filesystem.md
index 4d34c07102e..2d6ea0c4645 100644
--- a/site/en/r1/guide/extend/filesystem.md
+++ b/site/en/r1/guide/extend/filesystem.md
@@ -54,7 +54,7 @@ To implement a custom filesystem plugin, you must do the following:
### The FileSystem interface
The `FileSystem` interface is an abstract C++ interface defined in
-[file_system.h](https://github.com/tensorflow/tensorflow/blob/master/tensorflow/core/platform/file_system.h).
+[file_system.h](https://github.com/tensorflow/tensorflow/blob/r1.15/tensorflow/core/platform/file_system.h).
An implementation of the `FileSystem` interface should implement all relevant
the methods defined by the interface. Implementing the interface requires
defining operations such as creating `RandomAccessFile`, `WritableFile`, and
@@ -70,26 +70,26 @@ involves calling `stat()` on the file and then returns the filesize as reported
by the return of the stat object. Similarly, for the `HDFSFileSystem`
implementation, these calls simply delegate to the `libHDFS` implementation of
similar functionality, such as `hdfsDelete` for
-[DeleteFile](https://github.com/tensorflow/tensorflow/blob/master/tensorflow/core/platform/hadoop/hadoop_file_system.cc#L386).
+[DeleteFile](https://github.com/tensorflow/tensorflow/blob/r1.15/tensorflow/core/platform/hadoop/hadoop_file_system.cc#L386).
We suggest looking through these code examples to get an idea of how different
filesystem implementations call their existing libraries. Examples include:
* [POSIX
- plugin](https://github.com/tensorflow/tensorflow/blob/master/tensorflow/core/platform/posix/posix_file_system.h)
+ plugin](https://github.com/tensorflow/tensorflow/blob/r1.15/tensorflow/core/platform/posix/posix_file_system.h)
* [HDFS
- plugin](https://github.com/tensorflow/tensorflow/blob/master/tensorflow/core/platform/hadoop/hadoop_file_system.h)
+ plugin](https://github.com/tensorflow/tensorflow/blob/r1.15/tensorflow/core/platform/hadoop/hadoop_file_system.h)
* [GCS
- plugin](https://github.com/tensorflow/tensorflow/blob/master/tensorflow/core/platform/cloud/gcs_file_system.h)
+ plugin](https://github.com/tensorflow/tensorflow/blob/r1.15/tensorflow/core/platform/cloud/gcs_file_system.h)
* [S3
- plugin](https://github.com/tensorflow/tensorflow/blob/master/tensorflow/core/platform/s3/s3_file_system.h)
+ plugin](https://github.com/tensorflow/tensorflow/blob/r1.15/tensorflow/core/platform/s3/s3_file_system.h)
#### The File interfaces
Beyond operations that allow you to query and manipulate files and directories
in a filesystem, the `FileSystem` interface requires you to implement factories
that return implementations of abstract objects such as the
-[RandomAccessFile](https://github.com/tensorflow/tensorflow/blob/master/tensorflow/core/platform/file_system.h#L223),
+[RandomAccessFile](https://github.com/tensorflow/tensorflow/blob/r1.15/tensorflow/core/platform/file_system.h#L223),
the `WritableFile`, so that TensorFlow code and read and write to files in that
`FileSystem` implementation.
@@ -224,7 +224,7 @@ it will use the `FooBarFileSystem` implementation.
Next, you must build a shared object containing this implementation. An example
of doing so using bazel's `cc_binary` rule can be found
-[here](https://github.com/tensorflow/tensorflow/blob/master/tensorflow/python/BUILD#L244),
+[here](https://github.com/tensorflow/tensorflow/blob/r1.15/tensorflow/python/BUILD#L244),
but you may use any build system to do so. See the section on [building the op library](../extend/op.md#build_the_op_library) for similar
instructions.
@@ -236,7 +236,7 @@ passing the path to the shared object. Calling this in your client program loads
the shared object in the process, thus registering your implementation as
available for any file operations going through the `FileSystem` interface. You
can see
-[test_file_system.py](https://github.com/tensorflow/tensorflow/blob/master/tensorflow/python/framework/file_system_test.py)
+[test_file_system.py](https://github.com/tensorflow/tensorflow/blob/r1.15/tensorflow/python/framework/file_system_test.py)
for an example.
## What goes through this interface?
diff --git a/site/en/r1/guide/extend/formats.md b/site/en/r1/guide/extend/formats.md
index 3b7b4aafbd6..bdebee5487d 100644
--- a/site/en/r1/guide/extend/formats.md
+++ b/site/en/r1/guide/extend/formats.md
@@ -28,11 +28,11 @@ individual records in a file. There are several examples of "reader" datasets
that are already built into TensorFlow:
* `tf.data.TFRecordDataset`
- ([source in `kernels/data/reader_dataset_ops.cc`](https://www.tensorflow.org/code/tensorflow/core/kernels/data/reader_dataset_ops.cc))
+ ([source in `kernels/data/reader_dataset_ops.cc`](https://github.com/tensorflow/tensorflow/blob/r1.15/tensorflow/core/kernels/data/reader_dataset_ops.cc))
* `tf.data.FixedLengthRecordDataset`
- ([source in `kernels/data/reader_dataset_ops.cc`](https://www.tensorflow.org/code/tensorflow/core/kernels/data/reader_dataset_ops.cc))
+ ([source in `kernels/data/reader_dataset_ops.cc`](https://github.com/tensorflow/tensorflow/blob/r1.15/tensorflow/core/kernels/data/reader_dataset_ops.cc))
* `tf.data.TextLineDataset`
- ([source in `kernels/data/reader_dataset_ops.cc`](https://www.tensorflow.org/code/tensorflow/core/kernels/data/reader_dataset_ops.cc))
+ ([source in `kernels/data/reader_dataset_ops.cc`](https://github.com/tensorflow/tensorflow/blob/r1.15/tensorflow/core/kernels/data/reader_dataset_ops.cc))
Each of these implementations comprises three related classes:
@@ -279,7 +279,7 @@ if __name__ == "__main__":
```
You can see some examples of `Dataset` wrapper classes in
-[`tensorflow/python/data/ops/dataset_ops.py`](https://www.tensorflow.org/code/tensorflow/python/data/ops/dataset_ops.py).
+[`tensorflow/python/data/ops/dataset_ops.py`](https://github.com/tensorflow/tensorflow/blob/r1.15/tensorflow/python/data/ops/dataset_ops.py).
## Writing an Op for a record format
@@ -297,7 +297,7 @@ Examples of Ops useful for decoding records:
Note that it can be useful to use multiple Ops to decode a particular record
format. For example, you may have an image saved as a string in
-[a `tf.train.Example` protocol buffer](https://www.tensorflow.org/code/tensorflow/core/example/example.proto).
+[a `tf.train.Example` protocol buffer](https://github.com/tensorflow/tensorflow/blob/r1.15/tensorflow/core/example/example.proto).
Depending on the format of that image, you might take the corresponding output
from a `tf.parse_single_example` op and call `tf.image.decode_jpeg`,
`tf.image.decode_png`, or `tf.decode_raw`. It is common to take the output
diff --git a/site/en/r1/guide/extend/model_files.md b/site/en/r1/guide/extend/model_files.md
index 30e73a5169e..e590fcf1f27 100644
--- a/site/en/r1/guide/extend/model_files.md
+++ b/site/en/r1/guide/extend/model_files.md
@@ -28,7 +28,7 @@ by calling `as_graph_def()`, which returns a `GraphDef` object.
The GraphDef class is an object created by the ProtoBuf library from the
definition in
-[tensorflow/core/framework/graph.proto](https://github.com/tensorflow/tensorflow/blob/master/tensorflow/core/framework/graph.proto). The protobuf tools parse
+[tensorflow/core/framework/graph.proto](https://github.com/tensorflow/tensorflow/blob/r1.15/tensorflow/core/framework/graph.proto). The protobuf tools parse
this text file, and generate the code to load, store, and manipulate graph
definitions. If you see a standalone TensorFlow file representing a model, it's
likely to contain a serialized version of one of these `GraphDef` objects
@@ -87,7 +87,7 @@ for node in graph_def.node
```
Each node is a `NodeDef` object, defined in
-[tensorflow/core/framework/node_def.proto](https://github.com/tensorflow/tensorflow/blob/master/tensorflow/core/framework/node_def.proto). These
+[tensorflow/core/framework/node_def.proto](https://github.com/tensorflow/tensorflow/blob/r1.15/tensorflow/core/framework/node_def.proto). These
are the fundamental building blocks of TensorFlow graphs, with each one defining
a single operation along with its input connections. Here are the members of a
`NodeDef`, and what they mean.
@@ -107,7 +107,7 @@ This defines what operation to run, for example `"Add"`, `"MatMul"`, or
`"Conv2D"`. When a graph is run, this op name is looked up in a registry to
find an implementation. The registry is populated by calls to the
`REGISTER_OP()` macro, like those in
-[tensorflow/core/ops/nn_ops.cc](https://github.com/tensorflow/tensorflow/blob/master/tensorflow/core/ops/nn_ops.cc).
+[tensorflow/core/ops/nn_ops.cc](https://github.com/tensorflow/tensorflow/blob/r1.15/tensorflow/core/ops/nn_ops.cc).
### `input`
@@ -133,7 +133,7 @@ size of filters for convolutions, or the values of constant ops. Because there
can be so many different types of attribute values, from strings, to ints, to
arrays of tensor values, there's a separate protobuf file defining the data
structure that holds them, in
-[tensorflow/core/framework/attr_value.proto](https://github.com/tensorflow/tensorflow/blob/master/tensorflow/core/framework/attr_value.proto).
+[tensorflow/core/framework/attr_value.proto](https://github.com/tensorflow/tensorflow/blob/r1.15/tensorflow/core/framework/attr_value.proto).
Each attribute has a unique name string, and the expected attributes are listed
when the operation is defined. If an attribute isn't present in a node, but it
@@ -151,7 +151,7 @@ the file format during training. Instead, they're held in separate checkpoint
files, and there are `Variable` ops in the graph that load the latest values
when they're initialized. It's often not very convenient to have separate files
when you're deploying to production, so there's the
-[freeze_graph.py](https://github.com/tensorflow/tensorflow/blob/master/tensorflow/python/tools/freeze_graph.py) script that takes a graph definition and a set
+[freeze_graph.py](https://github.com/tensorflow/tensorflow/blob/r1.15/tensorflow/python/tools/freeze_graph.py) script that takes a graph definition and a set
of checkpoints and freezes them together into a single file.
What this does is load the `GraphDef`, pull in the values for all the variables
@@ -167,7 +167,7 @@ the most common problems is extracting and interpreting the weight values. A
common way to store them, for example in graphs created by the freeze_graph
script, is as `Const` ops containing the weights as `Tensors`. These are
defined in
-[tensorflow/core/framework/tensor.proto](https://github.com/tensorflow/tensorflow/blob/master/tensorflow/core/framework/tensor.proto), and contain information
+[tensorflow/core/framework/tensor.proto](https://github.com/tensorflow/tensorflow/blob/r1.15/tensorflow/core/framework/tensor.proto), and contain information
about the size and type of the data, as well as the values themselves. In
Python, you get a `TensorProto` object from a `NodeDef` representing a `Const`
op by calling something like `some_node_def.attr['value'].tensor`.
diff --git a/site/en/r1/guide/extend/op.md b/site/en/r1/guide/extend/op.md
index dc2d9fbe678..186d9c28c04 100644
--- a/site/en/r1/guide/extend/op.md
+++ b/site/en/r1/guide/extend/op.md
@@ -47,7 +47,7 @@ To incorporate your custom op you'll need to:
test the op in C++. If you define gradients, you can verify them with the
Python `tf.test.compute_gradient_error`.
See
- [`relu_op_test.py`](https://www.tensorflow.org/code/tensorflow/python/kernel_tests/relu_op_test.py) as
+ [`relu_op_test.py`](https://github.com/tensorflow/tensorflow/blob/r1.15/tensorflow/python/kernel_tests/relu_op_test.py) as
an example that tests the forward functions of Relu-like operators and
their gradients.
@@ -155,17 +155,17 @@ REGISTER_KERNEL_BUILDER(Name("ZeroOut").Device(DEVICE_CPU), ZeroOutOp);
> Important: Instances of your OpKernel may be accessed concurrently.
> Your `Compute` method must be thread-safe. Guard any access to class
> members with a mutex. Or better yet, don't share state via class members!
-> Consider using a [`ResourceMgr`](https://www.tensorflow.org/code/tensorflow/core/framework/resource_mgr.h)
+> Consider using a [`ResourceMgr`](https://github.com/tensorflow/tensorflow/blob/r1.15/tensorflow/core/framework/resource_mgr.h)
> to keep track of op state.
### Multi-threaded CPU kernels
To write a multi-threaded CPU kernel, the Shard function in
-[`work_sharder.h`](https://www.tensorflow.org/code/tensorflow/core/util/work_sharder.h)
+[`work_sharder.h`](https://github.com/tensorflow/tensorflow/blob/r1.15/tensorflow/core/util/work_sharder.h)
can be used. This function shards a computation function across the
threads configured to be used for intra-op threading (see
intra_op_parallelism_threads in
-[`config.proto`](https://www.tensorflow.org/code/tensorflow/core/protobuf/config.proto)).
+[`config.proto`](https://github.com/tensorflow/tensorflow/blob/r1.15/tensorflow/core/protobuf/config.proto)).
### GPU kernels
@@ -486,13 +486,13 @@ This asserts that the input is a vector, and returns having set the
* The `context`, which can either be an `OpKernelContext` or
`OpKernelConstruction` pointer (see
- [`tensorflow/core/framework/op_kernel.h`](https://www.tensorflow.org/code/tensorflow/core/framework/op_kernel.h)),
+ [`tensorflow/core/framework/op_kernel.h`](https://github.com/tensorflow/tensorflow/blob/r1.15/tensorflow/core/framework/op_kernel.h)),
for its `SetStatus()` method.
* The condition. For example, there are functions for validating the shape
of a tensor in
- [`tensorflow/core/framework/tensor_shape.h`](https://www.tensorflow.org/code/tensorflow/core/framework/tensor_shape.h)
+ [`tensorflow/core/framework/tensor_shape.h`](https://github.com/tensorflow/tensorflow/blob/r1.15/tensorflow/core/framework/tensor_shape.h)
* The error itself, which is represented by a `Status` object, see
- [`tensorflow/core/lib/core/status.h`](https://www.tensorflow.org/code/tensorflow/core/lib/core/status.h). A
+ [`tensorflow/core/lib/core/status.h`](https://github.com/tensorflow/tensorflow/blob/r1.15/tensorflow/core/lib/core/status.h). A
`Status` has both a type (frequently `InvalidArgument`, but see the list of
types) and a message. Functions for constructing an error may be found in
[`tensorflow/core/lib/core/errors.h`][validation-macros].
@@ -633,7 +633,7 @@ define an attr with constraints, you can use the following ``s:
The specific lists of types allowed by these are defined by the functions
(like `NumberTypes()`) in
- [`tensorflow/core/framework/types.h`](https://www.tensorflow.org/code/tensorflow/core/framework/types.h).
+ [`tensorflow/core/framework/types.h`](https://github.com/tensorflow/tensorflow/blob/r1.15/tensorflow/core/framework/types.h).
In this example the attr `t` must be one of the numeric types:
```c++
@@ -1180,7 +1180,7 @@ There are several ways to preserve backwards-compatibility.
type into a list of varying types).
The full list of safe and unsafe changes can be found in
-[`tensorflow/core/framework/op_compatibility_test.cc`](https://www.tensorflow.org/code/tensorflow/core/framework/op_compatibility_test.cc).
+[`tensorflow/core/framework/op_compatibility_test.cc`](https://github.com/tensorflow/tensorflow/blob/r1.15/tensorflow/core/framework/op_compatibility_test.cc).
If you cannot make your change to an operation backwards compatible, then create
a new operation with a new name with the new semantics.
@@ -1197,16 +1197,16 @@ made when TensorFlow changes major versions, and must conform to the
You can implement different OpKernels and register one for CPU and another for
GPU, just like you can [register kernels for different types](#polymorphism).
There are several examples of kernels with GPU support in
-[`tensorflow/core/kernels/`](https://www.tensorflow.org/code/tensorflow/core/kernels/).
+[`tensorflow/core/kernels/`](https://github.com/tensorflow/tensorflow/blob/r1.15/tensorflow/core/kernels/).
Notice some kernels have a CPU version in a `.cc` file, a GPU version in a file
ending in `_gpu.cu.cc`, and some code shared in common in a `.h` file.
For example, the `tf.pad` has
everything but the GPU kernel in [`tensorflow/core/kernels/pad_op.cc`][pad_op].
The GPU kernel is in
-[`tensorflow/core/kernels/pad_op_gpu.cu.cc`](https://www.tensorflow.org/code/tensorflow/core/kernels/pad_op_gpu.cu.cc),
+[`tensorflow/core/kernels/pad_op_gpu.cu.cc`](https://github.com/tensorflow/tensorflow/blob/r1.15/tensorflow/core/kernels/pad_op_gpu.cu.cc),
and the shared code is a templated class defined in
-[`tensorflow/core/kernels/pad_op.h`](https://www.tensorflow.org/code/tensorflow/core/kernels/pad_op.h).
+[`tensorflow/core/kernels/pad_op.h`](https://github.com/tensorflow/tensorflow/blob/r1.15/tensorflow/core/kernels/pad_op.h).
We organize the code this way for two reasons: it allows you to share common
code among the CPU and GPU implementations, and it puts the GPU implementation
into a separate file so that it can be compiled only by the GPU compiler.
@@ -1227,16 +1227,16 @@ kept on the CPU, add a `HostMemory()` call to the kernel registration, e.g.:
#### Compiling the kernel for the GPU device
Look at
-[cuda_op_kernel.cu.cc](https://www.tensorflow.org/code/tensorflow/examples/adding_an_op/cuda_op_kernel.cu.cc)
+[cuda_op_kernel.cu.cc](https://github.com/tensorflow/tensorflow/blob/r1.15/tensorflow/examples/adding_an_op/cuda_op_kernel.cu.cc)
for an example that uses a CUDA kernel to implement an op. The
`tf_custom_op_library` accepts a `gpu_srcs` argument in which the list of source
files containing the CUDA kernels (`*.cu.cc` files) can be specified. For use
with a binary installation of TensorFlow, the CUDA kernels have to be compiled
with NVIDIA's `nvcc` compiler. Here is the sequence of commands you can use to
compile the
-[cuda_op_kernel.cu.cc](https://www.tensorflow.org/code/tensorflow/examples/adding_an_op/cuda_op_kernel.cu.cc)
+[cuda_op_kernel.cu.cc](https://github.com/tensorflow/tensorflow/blob/r1.15/tensorflow/examples/adding_an_op/cuda_op_kernel.cu.cc)
and
-[cuda_op_kernel.cc](https://www.tensorflow.org/code/tensorflow/examples/adding_an_op/cuda_op_kernel.cc)
+[cuda_op_kernel.cc](https://github.com/tensorflow/tensorflow/blob/r1.15/tensorflow/examples/adding_an_op/cuda_op_kernel.cc)
into a single dynamically loadable library:
```bash
@@ -1361,7 +1361,7 @@ be set to the first input's shape. If the output is selected by its index as in
There are a number of common shape functions
that apply to many ops, such as `shape_inference::UnchangedShape` which can be
-found in [common_shape_fns.h](https://www.tensorflow.org/code/tensorflow/core/framework/common_shape_fns.h) and used as follows:
+found in [common_shape_fns.h](https://github.com/tensorflow/tensorflow/blob/r1.15/tensorflow/core/framework/common_shape_fns.h) and used as follows:
```c++
REGISTER_OP("ZeroOut")
@@ -1408,7 +1408,7 @@ provides access to the attributes of the op).
Since shape inference is an optional feature, and the shapes of tensors may vary
dynamically, shape functions must be robust to incomplete shape information for
-any of the inputs. The `Merge` method in [`InferenceContext`](https://www.tensorflow.org/code/tensorflow/core/framework/shape_inference.h)
+any of the inputs. The `Merge` method in [`InferenceContext`](https://github.com/tensorflow/tensorflow/blob/r1.15/tensorflow/core/framework/shape_inference.h)
allows the caller to assert that two shapes are the same, even if either
or both of them do not have complete information. Shape functions are defined
for all of the core TensorFlow ops and provide many different usage examples.
@@ -1433,7 +1433,7 @@ If you have a complicated shape function, you should consider adding a test for
validating that various input shape combinations produce the expected output
shape combinations. You can see examples of how to write these tests in some
our
-[core ops tests](https://www.tensorflow.org/code/tensorflow/core/ops/array_ops_test.cc).
+[core ops tests](https://github.com/tensorflow/tensorflow/blob/r1.15/tensorflow/core/ops/array_ops_test.cc).
(The syntax of `INFER_OK` and `INFER_ERROR` are a little cryptic, but try to be
compact in representing input and output shape specifications in tests. For
now, see the surrounding comments in those tests to get a sense of the shape
@@ -1446,20 +1446,20 @@ To build a `pip` package for your op, see the
guide shows how to build custom ops from the TensorFlow pip package instead
of building TensorFlow from source.
-[core-array_ops]:https://www.tensorflow.org/code/tensorflow/core/ops/array_ops.cc
-[python-user_ops]:https://www.tensorflow.org/code/tensorflow/python/user_ops/user_ops.py
-[tf-kernels]:https://www.tensorflow.org/code/tensorflow/core/kernels/
-[user_ops]:https://www.tensorflow.org/code/tensorflow/core/user_ops/
-[pad_op]:https://www.tensorflow.org/code/tensorflow/core/kernels/pad_op.cc
-[standard_ops-py]:https://www.tensorflow.org/code/tensorflow/python/ops/standard_ops.py
-[standard_ops-cc]:https://www.tensorflow.org/code/tensorflow/cc/ops/standard_ops.h
-[python-BUILD]:https://www.tensorflow.org/code/tensorflow/python/BUILD
-[validation-macros]:https://www.tensorflow.org/code/tensorflow/core/lib/core/errors.h
-[op_def_builder]:https://www.tensorflow.org/code/tensorflow/core/framework/op_def_builder.h
-[register_types]:https://www.tensorflow.org/code/tensorflow/core/framework/register_types.h
-[FinalizeAttr]:https://www.tensorflow.org/code/tensorflow/core/framework/op_def_builder.cc
-[DataTypeString]:https://www.tensorflow.org/code/tensorflow/core/framework/types.cc
-[python-BUILD]:https://www.tensorflow.org/code/tensorflow/python/BUILD
-[types-proto]:https://www.tensorflow.org/code/tensorflow/core/framework/types.proto
-[TensorShapeProto]:https://www.tensorflow.org/code/tensorflow/core/framework/tensor_shape.proto
-[TensorProto]:https://www.tensorflow.org/code/tensorflow/core/framework/tensor.proto
+[core-array_ops]:https://github.com/tensorflow/tensorflow/blob/r1.15/tensorflow/core/ops/array_ops.cc
+[python-user_ops]:https://github.com/tensorflow/tensorflow/blob/r1.15/tensorflow/python/user_ops/user_ops.py
+[tf-kernels]:https://github.com/tensorflow/tensorflow/blob/r1.15/tensorflow/core/kernels/
+[user_ops]:https://github.com/tensorflow/tensorflow/blob/r1.15/tensorflow/core/user_ops/
+[pad_op]:https://github.com/tensorflow/tensorflow/blob/r1.15/tensorflow/core/kernels/pad_op.cc
+[standard_ops-py]:https://github.com/tensorflow/tensorflow/blob/r1.15/tensorflow/python/ops/standard_ops.py
+[standard_ops-cc]:https://github.com/tensorflow/tensorflow/blob/r1.15/tensorflow/cc/ops/standard_ops.h
+[python-BUILD]:https://github.com/tensorflow/tensorflow/blob/r1.15/tensorflow/python/BUILD
+[validation-macros]:https://github.com/tensorflow/tensorflow/blob/r1.15/tensorflow/core/lib/core/errors.h
+[op_def_builder]:https://github.com/tensorflow/tensorflow/blob/r1.15/tensorflow/core/framework/op_def_builder.h
+[register_types]:https://github.com/tensorflow/tensorflow/blob/r1.15/tensorflow/core/framework/register_types.h
+[FinalizeAttr]:https://github.com/tensorflow/tensorflow/blob/r1.15/tensorflow/core/framework/op_def_builder.cc
+[DataTypeString]:https://github.com/tensorflow/tensorflow/blob/r1.15/tensorflow/core/framework/types.cc
+[python-BUILD]:https://github.com/tensorflow/tensorflow/blob/r1.15/tensorflow/python/BUILD
+[types-proto]:https://github.com/tensorflow/tensorflow/blob/r1.15/tensorflow/core/framework/types.proto
+[TensorShapeProto]:https://github.com/tensorflow/tensorflow/blob/r1.15/tensorflow/core/framework/tensor_shape.proto
+[TensorProto]:https://github.com/tensorflow/tensorflow/blob/r1.15/tensorflow/core/framework/tensor.proto
diff --git a/site/en/r1/guide/feature_columns.md b/site/en/r1/guide/feature_columns.md
index 5a4dfbbf46d..e4259f85e9f 100644
--- a/site/en/r1/guide/feature_columns.md
+++ b/site/en/r1/guide/feature_columns.md
@@ -562,7 +562,7 @@ For more examples on feature columns, view the following:
* The [Low Level Introduction](../guide/low_level_intro.md#feature_columns) demonstrates how
experiment directly with `feature_columns` using TensorFlow's low level APIs.
-* The [Estimator wide and deep learning tutorial](https://github.com/tensorflow/models/tree/master/official/r1/wide_deep)
+* The [Estimator wide and deep learning tutorial](https://github.com/tensorflow/models/tree/r1.15/official/r1/wide_deep)
solves a binary classification problem using `feature_columns` on a variety of
input data types.
diff --git a/site/en/r1/guide/graph_viz.md b/site/en/r1/guide/graph_viz.md
index 1965378e03e..1e3780e7928 100644
--- a/site/en/r1/guide/graph_viz.md
+++ b/site/en/r1/guide/graph_viz.md
@@ -251,7 +251,7 @@ is a snippet from the train and test section of a modification of the
[Estimators MNIST tutorial](../tutorials/estimators/cnn.md), in which we have
recorded summaries and
runtime statistics. See the
-[Tensorboard](https://tensorflow.org/tensorboard)
+[TensorBoard](https://tensorflow.org/tensorboard)
for details on how to record summaries.
Full source is [here](https://github.com/tensorflow/tensorflow/tree/r1.15/tensorflow/examples/tutorials/mnist/mnist_with_summaries.py).
diff --git a/site/en/r1/guide/performance/benchmarks.md b/site/en/r1/guide/performance/benchmarks.md
index 8998c0723db..a56959ea416 100644
--- a/site/en/r1/guide/performance/benchmarks.md
+++ b/site/en/r1/guide/performance/benchmarks.md
@@ -401,7 +401,7 @@ GPUs | InceptionV3 (batch size 32) | ResNet-50 (batch size 32)
## Methodology
This
-[script](https://github.com/tensorflow/benchmarks/tree/master/scripts/tf_cnn_benchmarks)
+[script](https://github.com/tensorflow/benchmarks/tree/r1.15/scripts/tf_cnn_benchmarks)
was run on the various platforms to generate the above results.
In order to create results that are as repeatable as possible, each test was run
diff --git a/site/en/r1/guide/performance/overview.md b/site/en/r1/guide/performance/overview.md
index af74f0f28c6..be7217f4b99 100644
--- a/site/en/r1/guide/performance/overview.md
+++ b/site/en/r1/guide/performance/overview.md
@@ -19,9 +19,9 @@ Reading large numbers of small files significantly impacts I/O performance.
One approach to get maximum I/O throughput is to preprocess input data into
larger (~100MB) `TFRecord` files. For smaller data sets (200MB-1GB), the best
approach is often to load the entire data set into memory. The document
-[Downloading and converting to TFRecord format](https://github.com/tensorflow/models/tree/master/research/slim#downloading-and-converting-to-tfrecord-format)
+[Downloading and converting to TFRecord format](https://github.com/tensorflow/models/tree/r1.15/research/slim#downloading-and-converting-to-tfrecord-format)
includes information and scripts for creating `TFRecord`s, and this
-[script](https://github.com/tensorflow/models/tree/master/research/tutorials/image/cifar10_estimator/generate_cifar10_tfrecords.py)
+[script](https://github.com/tensorflow/models/tree/r1.15/research/tutorials/image/cifar10_estimator/generate_cifar10_tfrecords.py)
converts the CIFAR-10 dataset into `TFRecord`s.
While feeding data using a `feed_dict` offers a high level of flexibility, in
@@ -122,7 +122,7 @@ tf.Session(config=config)
Intel® has added optimizations to TensorFlow for Intel® Xeon® and Intel® Xeon
Phi™ through the use of the Intel® Math Kernel Library for Deep Neural Networks
(Intel® MKL-DNN) optimized primitives. The optimizations also provide speedups
-for the consumer line of processors, e.g. i5 and i7 Intel processors. The Intel
+for the consumer line of processors, e.g., i5 and i7 Intel processors. The Intel
published paper
[TensorFlow* Optimizations on Modern Intel® Architecture](https://software.intel.com/en-us/articles/tensorflow-optimizations-on-modern-intel-architecture)
contains additional details on the implementation.
@@ -255,7 +255,7 @@ bazel build -c opt --copt=-march="broadwell" --config=cuda //tensorflow/tools/pi
a docker container, the data is not cached and the penalty is paid each time
TensorFlow starts. The best practice is to include the
[compute capabilities](http://developer.nvidia.com/cuda-gpus)
- of the GPUs that will be used, e.g. P100: 6.0, Titan X (Pascal): 6.1,
+ of the GPUs that will be used, e.g., P100: 6.0, Titan X (Pascal): 6.1,
Titan X (Maxwell): 5.2, and K80: 3.7.
* Use a version of `gcc` that supports all of the optimizations of the target
CPU. The recommended minimum gcc version is 4.8.3. On macOS, upgrade to the
diff --git a/site/en/r1/guide/saved_model.md b/site/en/r1/guide/saved_model.md
index 623863a9df9..34447ffe861 100644
--- a/site/en/r1/guide/saved_model.md
+++ b/site/en/r1/guide/saved_model.md
@@ -23,7 +23,7 @@ TensorFlow saves variables in binary *checkpoint files* that map variable
names to tensor values.
Caution: TensorFlow model files are code. Be careful with untrusted code.
-See [Using TensorFlow Securely](https://github.com/tensorflow/tensorflow/blob/master/SECURITY.md)
+See [Using TensorFlow Securely](https://github.com/tensorflow/tensorflow/blob/r1.15/SECURITY.md)
for details.
### Save variables
@@ -148,7 +148,7 @@ Notes:
`tf.variables_initializer` for more information.
* To inspect the variables in a checkpoint, you can use the
- [`inspect_checkpoint`](https://www.tensorflow.org/code/tensorflow/python/tools/inspect_checkpoint.py)
+ [`inspect_checkpoint`](https://github.com/tensorflow/tensorflow/blob/r1.15/tensorflow/python/tools/inspect_checkpoint.py)
library, particularly the `print_tensors_in_checkpoint_file` function.
* By default, `Saver` uses the value of the `tf.Variable.name` property
@@ -159,7 +159,7 @@ Notes:
### Inspect variables in a checkpoint
We can quickly inspect variables in a checkpoint with the
-[`inspect_checkpoint`](https://www.tensorflow.org/code/tensorflow/python/tools/inspect_checkpoint.py) library.
+[`inspect_checkpoint`](https://github.com/tensorflow/tensorflow/blob/r1.15/tensorflow/python/tools/inspect_checkpoint.py) library.
Continuing from the save/restore examples shown earlier:
@@ -216,7 +216,7 @@ simple_save(session,
This configures the `SavedModel` so it can be loaded by
[TensorFlow serving](https://www.tensorflow.org/tfx/tutorials/serving/rest_simple) and supports the
-[Predict API](https://github.com/tensorflow/serving/blob/master/tensorflow_serving/apis/predict.proto).
+[Predict API](https://github.com/tensorflow/serving/blob/r1.15/tensorflow_serving/apis/predict.proto).
To access the classify, regress, or multi-inference APIs, use the manual
`SavedModel` builder APIs or an `tf.estimator.Estimator`.
@@ -328,7 +328,7 @@ with tf.Session(graph=tf.Graph()) as sess:
### Load a SavedModel in C++
The C++ version of the SavedModel
-[loader](https://github.com/tensorflow/tensorflow/blob/master/tensorflow/cc/saved_model/loader.h)
+[loader](https://github.com/tensorflow/tensorflow/blob/r1.15/tensorflow/cc/saved_model/loader.h)
provides an API to load a SavedModel from a path, while allowing
`SessionOptions` and `RunOptions`.
You have to specify the tags associated with the graph to be loaded.
@@ -383,20 +383,20 @@ reuse and share across tools consistently.
You may use sets of tags to uniquely identify a `MetaGraphDef` saved in a
SavedModel. A subset of commonly used tags is specified in:
-* [Python](https://github.com/tensorflow/tensorflow/blob/master/tensorflow/python/saved_model/tag_constants.py)
-* [C++](https://github.com/tensorflow/tensorflow/blob/master/tensorflow/cc/saved_model/tag_constants.h)
+* [Python](https://github.com/tensorflow/tensorflow/blob/r1.15/tensorflow/python/saved_model/tag_constants.py)
+* [C++](https://github.com/tensorflow/tensorflow/blob/r1.15/tensorflow/cc/saved_model/tag_constants.h)
#### Standard SignatureDef constants
-A [**SignatureDef**](https://github.com/tensorflow/tensorflow/blob/master/tensorflow/core/protobuf/meta_graph.proto)
+A [**SignatureDef**](https://github.com/tensorflow/tensorflow/blob/r1.15/tensorflow/core/protobuf/meta_graph.proto)
is a protocol buffer that defines the signature of a computation
supported by a graph.
Commonly used input keys, output keys, and method names are
defined in:
-* [Python](https://github.com/tensorflow/tensorflow/blob/master/tensorflow/python/saved_model/signature_constants.py)
-* [C++](https://github.com/tensorflow/tensorflow/blob/master/tensorflow/cc/saved_model/signature_constants.h)
+* [Python](https://github.com/tensorflow/tensorflow/blob/r1.15/tensorflow/python/saved_model/signature_constants.py)
+* [C++](https://github.com/tensorflow/tensorflow/blob/r1.15/tensorflow/cc/saved_model/signature_constants.h)
## Using SavedModel with Estimators
@@ -408,7 +408,7 @@ To prepare a trained Estimator for serving, you must export it in the standard
SavedModel format. This section explains how to:
* Specify the output nodes and the corresponding
- [APIs](https://github.com/tensorflow/serving/blob/master/tensorflow_serving/apis/prediction_service.proto)
+ [APIs](https://github.com/tensorflow/serving/blob/r1.15/tensorflow_serving/apis/prediction_service.proto)
that can be served (Classify, Regress, or Predict).
* Export your model to the SavedModel format.
* Serve the model from a local server and request predictions.
@@ -506,7 +506,7 @@ Each `output` value must be an `ExportOutput` object such as
`tf.estimator.export.PredictOutput`.
These output types map straightforwardly to the
-[TensorFlow Serving APIs](https://github.com/tensorflow/serving/blob/master/tensorflow_serving/apis/prediction_service.proto),
+[TensorFlow Serving APIs](https://github.com/tensorflow/serving/blob/r1.15/tensorflow_serving/apis/prediction_service.proto),
and so determine which request types will be honored.
Note: In the multi-headed case, a `SignatureDef` will be generated for each
@@ -515,7 +515,7 @@ the same keys. These `SignatureDef`s differ only in their outputs, as
provided by the corresponding `ExportOutput` entry. The inputs are always
those provided by the `serving_input_receiver_fn`.
An inference request may specify the head by name. One head must be named
-using [`signature_constants.DEFAULT_SERVING_SIGNATURE_DEF_KEY`](https://www.tensorflow.org/code/tensorflow/python/saved_model/signature_constants.py)
+using [`signature_constants.DEFAULT_SERVING_SIGNATURE_DEF_KEY`](https://github.com/tensorflow/tensorflow/blob/r1.15/tensorflow/python/saved_model/signature_constants.py)
indicating which `SignatureDef` will be served when an inference request
does not specify one.
@@ -566,9 +566,9 @@ Now you have a server listening for inference requests via gRPC on port 9000!
### Request predictions from a local server
The server responds to gRPC requests according to the
-[PredictionService](https://github.com/tensorflow/serving/blob/master/tensorflow_serving/apis/prediction_service.proto#L15)
+[PredictionService](https://github.com/tensorflow/serving/blob/r1.15/tensorflow_serving/apis/prediction_service.proto#L15)
gRPC API service definition. (The nested protocol buffers are defined in
-various [neighboring files](https://github.com/tensorflow/serving/blob/master/tensorflow_serving/apis)).
+various [neighboring files](https://github.com/tensorflow/serving/blob/r1.15/tensorflow_serving/apis)).
From the API service definition, the gRPC framework generates client libraries
in various languages providing remote access to the API. In a project using the
@@ -620,7 +620,7 @@ The returned result in this example is a `ClassificationResponse` protocol
buffer.
This is a skeletal example; please see the [Tensorflow Serving](../deploy/index.md)
-documentation and [examples](https://github.com/tensorflow/serving/tree/master/tensorflow_serving/example)
+documentation and [examples](https://github.com/tensorflow/serving/tree/r1.15/tensorflow_serving/example)
for more details.
> Note: `ClassificationRequest` and `RegressionRequest` contain a
diff --git a/site/en/r1/guide/using_tpu.md b/site/en/r1/guide/using_tpu.md
index 74169092189..e3e338adf49 100644
--- a/site/en/r1/guide/using_tpu.md
+++ b/site/en/r1/guide/using_tpu.md
@@ -7,8 +7,8 @@ changing the *hardware accelerator* in your notebook settings:
TPU-enabled Colab notebooks are available to test:
1. [A quick test, just to measure FLOPS](https://colab.research.google.com/notebooks/tpu.ipynb).
- 2. [A CNN image classifier with `tf.keras`](https://colab.research.google.com/github/tensorflow/tpu/blob/master/tools/colab/fashion_mnist.ipynb).
- 3. [An LSTM markov chain text generator with `tf.keras`](https://colab.research.google.com/github/tensorflow/tpu/blob/master/tools/colab/shakespeare_with_tpu_and_keras.ipynb)
+ 2. [A CNN image classifier with `tf.keras`](https://colab.research.google.com/github/tensorflow/tpu/blob/r1.15/tools/colab/fashion_mnist.ipynb).
+ 3. [An LSTM markov chain text generator with `tf.keras`](https://colab.research.google.com/github/tensorflow/tpu/blob/r1.15/tools/colab/shakespeare_with_tpu_and_keras.ipynb)
## TPUEstimator
@@ -25,7 +25,7 @@ Cloud TPU is to define the model's inference phase (from inputs to predictions)
outside of the `model_fn`. Then maintain separate implementations of the
`Estimator` setup and `model_fn`, both wrapping this inference step. For an
example of this pattern compare the `mnist.py` and `mnist_tpu.py` implementation in
-[tensorflow/models](https://github.com/tensorflow/models/tree/master/official/r1/mnist).
+[tensorflow/models](https://github.com/tensorflow/models/tree/r1.15/official/r1/mnist).
### Run a TPUEstimator locally
@@ -350,10 +350,10 @@ in bytes. A minimum of a few MB (`buffer_size=8*1024*1024`) is recommended so
that data is available when needed.
The TPU-demos repo includes
-[a script](https://github.com/tensorflow/tpu/blob/master/tools/datasets/imagenet_to_gcs.py)
+[a script](https://github.com/tensorflow/tpu/blob/1.15/tools/datasets/imagenet_to_gcs.py)
for downloading the imagenet dataset and converting it to an appropriate format.
This together with the imagenet
-[models](https://github.com/tensorflow/tpu/tree/master/models)
+[models](https://github.com/tensorflow/tpu/tree/r1.15/models)
included in the repo demonstrate all of these best-practices.
## Next steps
diff --git a/site/en/r1/guide/version_compat.md b/site/en/r1/guide/version_compat.md
index 6702f6e0819..a765620518d 100644
--- a/site/en/r1/guide/version_compat.md
+++ b/site/en/r1/guide/version_compat.md
@@ -49,19 +49,19 @@ patch versions. The public APIs consist of
submodules, but is not documented, then it is **not** considered part of the
public API.
-* The [C API](https://github.com/tensorflow/tensorflow/blob/master/tensorflow/c/c_api.h).
+* The [C API](https://github.com/tensorflow/tensorflow/blob/r1.15/tensorflow/c/c_api.h).
* The following protocol buffer files:
- * [`attr_value`](https://github.com/tensorflow/tensorflow/blob/master/tensorflow/core/framework/attr_value.proto)
- * [`config`](https://github.com/tensorflow/tensorflow/blob/master/tensorflow/core/protobuf/config.proto)
- * [`event`](https://github.com/tensorflow/tensorflow/blob/master/tensorflow/core/util/event.proto)
- * [`graph`](https://github.com/tensorflow/tensorflow/blob/master/tensorflow/core/framework/graph.proto)
- * [`op_def`](https://github.com/tensorflow/tensorflow/blob/master/tensorflow/core/framework/op_def.proto)
- * [`reader_base`](https://github.com/tensorflow/tensorflow/blob/master/tensorflow/core/framework/reader_base.proto)
- * [`summary`](https://github.com/tensorflow/tensorflow/blob/master/tensorflow/core/framework/summary.proto)
- * [`tensor`](https://github.com/tensorflow/tensorflow/blob/master/tensorflow/core/framework/tensor.proto)
- * [`tensor_shape`](https://github.com/tensorflow/tensorflow/blob/master/tensorflow/core/framework/tensor_shape.proto)
- * [`types`](https://github.com/tensorflow/tensorflow/blob/master/tensorflow/core/framework/types.proto)
+ * [`attr_value`](https://github.com/tensorflow/tensorflow/blob/r1.15/tensorflow/core/framework/attr_value.proto)
+ * [`config`](https://github.com/tensorflow/tensorflow/blob/r1.15/tensorflow/core/protobuf/config.proto)
+ * [`event`](https://github.com/tensorflow/tensorflow/blob/r1.15/tensorflow/core/util/event.proto)
+ * [`graph`](https://github.com/tensorflow/tensorflow/blob/r1.15/tensorflow/core/framework/graph.proto)
+ * [`op_def`](https://github.com/tensorflow/tensorflow/blob/r1.15/tensorflow/core/framework/op_def.proto)
+ * [`reader_base`](https://github.com/tensorflow/tensorflow/blob/r1.15/tensorflow/core/framework/reader_base.proto)
+ * [`summary`](https://github.com/tensorflow/tensorflow/blob/r1.15/tensorflow/core/framework/summary.proto)
+ * [`tensor`](https://github.com/tensorflow/tensorflow/blob/r1.15/tensorflow/core/framework/tensor.proto)
+ * [`tensor_shape`](https://github.com/tensorflow/tensorflow/blob/r1.15/tensorflow/core/framework/tensor_shape.proto)
+ * [`types`](https://github.com/tensorflow/tensorflow/blob/r1.15/tensorflow/core/framework/types.proto)
## What is *not* covered
@@ -79,7 +79,7 @@ backward incompatible ways between minor releases. These include:
such as:
- [C++](./extend/cc.md) (exposed through header files in
- [`tensorflow/cc`](https://github.com/tensorflow/tensorflow/tree/master/tensorflow/cc)).
+ [`tensorflow/cc`](https://github.com/tensorflow/tensorflow/tree/r1.15/tensorflow/cc)).
- [Java](../api_docs/java/reference/org/tensorflow/package-summary),
- [Go](https://pkg.go.dev/github.com/tensorflow/tensorflow/tensorflow/go)
- [JavaScript](https://js.tensorflow.org)
@@ -209,7 +209,7 @@ guidelines for evolving `GraphDef` versions.
There are different data versions for graphs and checkpoints. The two data
formats evolve at different rates from each other and also at different rates
from TensorFlow. Both versioning systems are defined in
-[`core/public/version.h`](https://github.com/tensorflow/tensorflow/blob/master/tensorflow/core/public/version.h).
+[`core/public/version.h`](https://github.com/tensorflow/tensorflow/blob/r1.15/tensorflow/core/public/version.h).
Whenever a new version is added, a note is added to the header detailing what
changed and the date.
@@ -224,7 +224,7 @@ We distinguish between the following kinds of data version information:
(`min_producer`).
Each piece of versioned data has a [`VersionDef
-versions`](https://github.com/tensorflow/tensorflow/blob/master/tensorflow/core/framework/versions.proto)
+versions`](https://github.com/tensorflow/tensorflow/blob/r1.15/tensorflow/core/framework/versions.proto)
field which records the `producer` that made the data, the `min_consumer`
that it is compatible with, and a list of `bad_consumers` versions that are
disallowed.
@@ -239,7 +239,7 @@ accept a piece of data if the following are all true:
* `consumer` not in data's `bad_consumers`
Since both producers and consumers come from the same TensorFlow code base,
-[`core/public/version.h`](https://github.com/tensorflow/tensorflow/blob/master/tensorflow/core/public/version.h)
+[`core/public/version.h`](https://github.com/tensorflow/tensorflow/blob/r1.15/tensorflow/core/public/version.h)
contains a main data version which is treated as either `producer` or
`consumer` depending on context and both `min_consumer` and `min_producer`
(needed by producers and consumers, respectively). Specifically,
@@ -309,7 +309,7 @@ existing producer scripts will not suddenly use the new functionality.
1. Add a new similar op named `SomethingV2` or similar and go through the
process of adding it and switching existing Python wrappers to use it.
To ensure forward compatibility use the checks suggested in
- [compat.py](https://www.tensorflow.org/code/tensorflow/python/compat/compat.py)
+ [compat.py](https://github.com/tensorflow/tensorflow/blob/r1.15/tensorflow/python/compat/compat.py)
when changing the Python wrappers.
2. Remove the old op (Can only take place with a major version change due to
backward compatibility).
diff --git a/site/en/r1/tutorials/README.md b/site/en/r1/tutorials/README.md
index 5094e645e6e..9ff164ad77c 100644
--- a/site/en/r1/tutorials/README.md
+++ b/site/en/r1/tutorials/README.md
@@ -68,4 +68,4 @@ implement common ML algorithms. See the
* [Boosted trees](./estimators/boosted_trees.ipynb)
* [Gradient Boosted Trees: Model understanding](./estimators/boosted_trees_model_understanding.ipynb)
* [Build a Convolutional Neural Network using Estimators](./estimators/cnn.ipynb)
-* [Wide and deep learning with Estimators](https://github.com/tensorflow/models/tree/master/official/r1/wide_deep)
+* [Wide and deep learning with Estimators](https://github.com/tensorflow/models/tree/r1.15/official/r1/wide_deep)
diff --git a/site/en/r1/tutorials/distribute/keras.ipynb b/site/en/r1/tutorials/distribute/keras.ipynb
index 059b8c2d66f..14e8bf739a9 100644
--- a/site/en/r1/tutorials/distribute/keras.ipynb
+++ b/site/en/r1/tutorials/distribute/keras.ipynb
@@ -86,7 +86,7 @@
"Essentially, it copies all of the model's variables to each processor.\n",
"Then, it uses [all-reduce](http://mpitutorial.com/tutorials/mpi-reduce-and-allreduce/) to combine the gradients from all processors and applies the combined value to all copies of the model.\n",
"\n",
- "`MirroredStategy` is one of several distribution strategy available in TensorFlow core. You can read about more strategies at [distribution strategy guide](../../guide/distribute_strategy.ipynb).\n"
+ "`MirroredStrategy` is one of several distribution strategy available in TensorFlow core. You can read about more strategies at [distribution strategy guide](../../guide/distribute_strategy.ipynb).\n"
]
},
{
diff --git a/site/en/r1/tutorials/images/deep_cnn.md b/site/en/r1/tutorials/images/deep_cnn.md
index 00a914d8976..885f3907aa7 100644
--- a/site/en/r1/tutorials/images/deep_cnn.md
+++ b/site/en/r1/tutorials/images/deep_cnn.md
@@ -80,15 +80,15 @@ for details. It consists of 1,068,298 learnable parameters and requires about
## Code Organization
The code for this tutorial resides in
-[`models/tutorials/image/cifar10/`](https://github.com/tensorflow/models/tree/master/research/tutorials/image/cifar10/).
+[`models/tutorials/image/cifar10/`](https://github.com/tensorflow/models/tree/r1.15/research/tutorials/image/cifar10/).
File | Purpose
--- | ---
-[`cifar10_input.py`](https://github.com/tensorflow/models/tree/master/research/tutorials/image/cifar10/cifar10_input.py) | Loads CIFAR-10 dataset using [tensorflow-datasets library](https://github.com/tensorflow/datasets).
-[`cifar10.py`](https://github.com/tensorflow/models/tree/master/research/tutorials/image/cifar10/cifar10.py) | Builds the CIFAR-10 model.
-[`cifar10_train.py`](https://github.com/tensorflow/models/tree/master/research/tutorials/image/cifar10/cifar10_train.py) | Trains a CIFAR-10 model on a CPU or GPU.
-[`cifar10_multi_gpu_train.py`](https://github.com/tensorflow/models/tree/master/research/tutorials/image/cifar10/cifar10_multi_gpu_train.py) | Trains a CIFAR-10 model on multiple GPUs.
-[`cifar10_eval.py`](https://github.com/tensorflow/models/tree/master/research/tutorials/image/cifar10/cifar10_eval.py) | Evaluates the predictive performance of a CIFAR-10 model.
+[`cifar10_input.py`](https://github.com/tensorflow/models/tree/r1.15/research/tutorials/image/cifar10/cifar10_input.py) | Loads CIFAR-10 dataset using [tensorflow-datasets library](https://github.com/tensorflow/datasets).
+[`cifar10.py`](https://github.com/tensorflow/models/tree/r1.15/research/tutorials/image/cifar10/cifar10.py) | Builds the CIFAR-10 model.
+[`cifar10_train.py`](https://github.com/tensorflow/models/tree/r1.15/research/tutorials/image/cifar10/cifar10_train.py) | Trains a CIFAR-10 model on a CPU or GPU.
+[`cifar10_multi_gpu_train.py`](https://github.com/tensorflow/models/tree/r1.15/research/tutorials/image/cifar10/cifar10_multi_gpu_train.py) | Trains a CIFAR-10 model on multiple GPUs.
+[`cifar10_eval.py`](https://github.com/tensorflow/models/tree/r1.15/research/tutorials/image/cifar10/cifar10_eval.py) | Evaluates the predictive performance of a CIFAR-10 model.
To run this tutorial, you will need to:
@@ -99,7 +99,7 @@ pip install tensorflow-datasets
## CIFAR-10 Model
The CIFAR-10 network is largely contained in
-[`cifar10.py`](https://github.com/tensorflow/models/tree/master/research/tutorials/image/cifar10/cifar10.py).
+[`cifar10.py`](https://github.com/tensorflow/models/tree/r1.15/research/tutorials/image/cifar10/cifar10.py).
The complete training
graph contains roughly 765 operations. We find that we can make the code most
reusable by constructing the graph with the following modules:
@@ -108,7 +108,7 @@ reusable by constructing the graph with the following modules:
operations that read and preprocess CIFAR images for evaluation and training,
respectively.
1. [**Model prediction:**](#model-prediction) `inference()`
-adds operations that perform inference, i.e. classification, on supplied images.
+adds operations that perform inference, i.e., classification, on supplied images.
1. [**Model training:**](#model-training) `loss()` and `train()`
add operations that compute the loss,
gradients, variable updates and visualization summaries.
@@ -405,7 +405,7 @@ a "tower". We must set two attributes for each tower:
* A unique name for all operations within a tower.
`tf.name_scope` provides
this unique name by prepending a scope. For instance, all operations in
-the first tower are prepended with `tower_0`, e.g. `tower_0/conv1/Conv2D`.
+the first tower are prepended with `tower_0`, e.g., `tower_0/conv1/Conv2D`.
* A preferred hardware device to run the operation within a tower.
`tf.device` specifies this. For
diff --git a/site/en/r1/tutorials/images/image_recognition.md b/site/en/r1/tutorials/images/image_recognition.md
index 0be884de403..cb66e594629 100644
--- a/site/en/r1/tutorials/images/image_recognition.md
+++ b/site/en/r1/tutorials/images/image_recognition.md
@@ -140,13 +140,13 @@ score of 0.8.
-Next, try it out on your own images by supplying the --image= argument, e.g.
+Next, try it out on your own images by supplying the --image= argument, e.g.,
```bash
bazel-bin/tensorflow/examples/label_image/label_image --image=my_image.png
```
-If you look inside the [`tensorflow/examples/label_image/main.cc`](https://github.com/tensorflow/tensorflow/blob/master/tensorflow/examples/label_image/main.cc)
+If you look inside the [`tensorflow/examples/label_image/main.cc`](https://github.com/tensorflow/tensorflow/blob/r1.15/tensorflow/examples/label_image/main.cc)
file, you can find out
how it works. We hope this code will help you integrate TensorFlow into
your own applications, so we will walk step by step through the main functions:
@@ -164,7 +164,7 @@ training. If you have a graph that you've trained yourself, you'll just need
to adjust the values to match whatever you used during your training process.
You can see how they're applied to an image in the
-[`ReadTensorFromImageFile()`](https://github.com/tensorflow/tensorflow/blob/master/tensorflow/examples/label_image/main.cc#L88)
+[`ReadTensorFromImageFile()`](https://github.com/tensorflow/tensorflow/blob/r1.15/tensorflow/examples/label_image/main.cc#L88)
function.
```C++
@@ -334,7 +334,7 @@ The `PrintTopLabels()` function takes those sorted results, and prints them out
friendly way. The `CheckTopLabel()` function is very similar, but just makes sure that
the top label is the one we expect, for debugging purposes.
-At the end, [`main()`](https://github.com/tensorflow/tensorflow/blob/master/tensorflow/examples/label_image/main.cc#L252)
+At the end, [`main()`](https://github.com/tensorflow/tensorflow/blob/r1.15/tensorflow/examples/label_image/main.cc#L252)
ties together all of these calls.
```C++
diff --git a/site/en/r1/tutorials/keras/save_and_restore_models.ipynb b/site/en/r1/tutorials/keras/save_and_restore_models.ipynb
index e9d112bd3f3..04cc94417a9 100644
--- a/site/en/r1/tutorials/keras/save_and_restore_models.ipynb
+++ b/site/en/r1/tutorials/keras/save_and_restore_models.ipynb
@@ -115,7 +115,7 @@
"\n",
"Sharing this data helps others understand how the model works and try it themselves with new data.\n",
"\n",
- "Caution: Be careful with untrusted code—TensorFlow models are code. See [Using TensorFlow Securely](https://github.com/tensorflow/tensorflow/blob/master/SECURITY.md) for details.\n",
+ "Caution: Be careful with untrusted code—TensorFlow models are code. See [Using TensorFlow Securely](https://github.com/tensorflow/tensorflow/blob/r1.15/SECURITY.md) for details.\n",
"\n",
"### Options\n",
"\n",
diff --git a/site/en/r1/tutorials/load_data/tf_records.ipynb b/site/en/r1/tutorials/load_data/tf_records.ipynb
index fa7bf83c8bb..45635034c69 100644
--- a/site/en/r1/tutorials/load_data/tf_records.ipynb
+++ b/site/en/r1/tutorials/load_data/tf_records.ipynb
@@ -141,7 +141,7 @@
"source": [
"Fundamentally a `tf.Example` is a `{\"string\": tf.train.Feature}` mapping.\n",
"\n",
- "The `tf.train.Feature` message type can accept one of the following three types (See the [`.proto` file](https://github.com/tensorflow/tensorflow/blob/master/tensorflow/core/example/feature.proto) for reference). Most other generic types can be coerced into one of these.\n",
+ "The `tf.train.Feature` message type can accept one of the following three types (See the [`.proto` file](https://github.com/tensorflow/tensorflow/blob/r1.15/tensorflow/core/example/feature.proto) for reference). Most other generic types can be coerced into one of these.\n",
"\n",
"1. `tf.train.BytesList` (the following types can be coerced)\n",
"\n",
@@ -276,7 +276,7 @@
"\n",
"1. We create a map (dictionary) from the feature name string to the encoded feature value produced in #1.\n",
"\n",
- "1. The map produced in #2 is converted to a [`Features` message](https://github.com/tensorflow/tensorflow/blob/master/tensorflow/core/example/feature.proto#L85)."
+ "1. The map produced in #2 is converted to a [`Features` message](https://github.com/tensorflow/tensorflow/blob/r1.15/tensorflow/core/example/feature.proto#L85)."
]
},
{
@@ -365,7 +365,7 @@
"id": "XftzX9CN_uGT"
},
"source": [
- "For example, suppose we have a single observation from the dataset, `[False, 4, bytes('goat'), 0.9876]`. We can create and print the `tf.Example` message for this observation using `create_message()`. Each single observation will be written as a `Features` message as per the above. Note that the `tf.Example` [message](https://github.com/tensorflow/tensorflow/blob/master/tensorflow/core/example/example.proto#L88) is just a wrapper around the `Features` message."
+ "For example, suppose we have a single observation from the dataset, `[False, 4, bytes('goat'), 0.9876]`. We can create and print the `tf.Example` message for this observation using `create_message()`. Each single observation will be written as a `Features` message as per the above. Note that the `tf.Example` [message](https://github.com/tensorflow/tensorflow/blob/r1.15/tensorflow/core/example/example.proto#L88) is just a wrapper around the `Features` message."
]
},
{
diff --git a/site/en/r1/tutorials/representation/kernel_methods.md b/site/en/r1/tutorials/representation/kernel_methods.md
index 67adc4951c6..227fe81d515 100644
--- a/site/en/r1/tutorials/representation/kernel_methods.md
+++ b/site/en/r1/tutorials/representation/kernel_methods.md
@@ -24,7 +24,7 @@ following sources for an introduction:
Currently, TensorFlow supports explicit kernel mappings for dense features only;
TensorFlow will provide support for sparse features at a later release.
-This tutorial uses [tf.contrib.learn](https://www.tensorflow.org/code/tensorflow/contrib/learn/python/learn)
+This tutorial uses [tf.contrib.learn](https://github.com/tensorflow/tensorflow/blob/r1.15/tensorflow/contrib/learn/python/learn)
(TensorFlow's high-level Machine Learning API) Estimators for our ML models.
If you are not familiar with this API, The [Estimator guide](../../guide/estimators.md)
is a good place to start. We will use the MNIST dataset. The tutorial consists
@@ -131,7 +131,7 @@ In addition to experimenting with the (training) batch size and the number of
training steps, there are a couple other parameters that can be tuned as well.
For instance, you can change the optimization method used to minimize the loss
by explicitly selecting another optimizer from the collection of
-[available optimizers](https://www.tensorflow.org/code/tensorflow/python/training).
+[available optimizers](https://github.com/tensorflow/tensorflow/blob/r1.15/tensorflow/python/training).
As an example, the following code constructs a LinearClassifier estimator that
uses the Follow-The-Regularized-Leader (FTRL) optimization strategy with a
specific learning rate and L2-regularization.
diff --git a/site/en/r1/tutorials/representation/linear.md b/site/en/r1/tutorials/representation/linear.md
index 5516672b34a..d996a13bc1f 100644
--- a/site/en/r1/tutorials/representation/linear.md
+++ b/site/en/r1/tutorials/representation/linear.md
@@ -12,7 +12,7 @@ those tools. It explains:
Read this overview to decide whether the Estimator's linear model tools might
be useful to you. Then work through the
-[Estimator wide and deep learning tutorial](https://github.com/tensorflow/models/tree/master/official/r1/wide_deep)
+[Estimator wide and deep learning tutorial](https://github.com/tensorflow/models/tree/r1.15/official/r1/wide_deep)
to give it a try. This overview uses code samples from the tutorial, but the
tutorial walks through the code in greater detail.
@@ -177,7 +177,7 @@ the name of a `FeatureColumn`. Each key's value is a tensor containing the
values of that feature for all data instances. See
[Premade Estimators](../../guide/premade_estimators.md#input_fn) for a
more comprehensive look at input functions, and `input_fn` in the
-[wide and deep learning tutorial](https://github.com/tensorflow/models/tree/master/official/r1/wide_deep)
+[wide and deep learning tutorial](https://github.com/tensorflow/models/tree/r1.15/official/r1/wide_deep)
for an example implementation of an input function.
The input function is passed to the `train()` and `evaluate()` calls that
@@ -236,4 +236,4 @@ e = tf.estimator.DNNLinearCombinedClassifier(
dnn_hidden_units=[100, 50])
```
For more information, see the
-[wide and deep learning tutorial](https://github.com/tensorflow/models/tree/master/official/r1/wide_deep).
+[wide and deep learning tutorial](https://github.com/tensorflow/models/tree/r1.15/official/r1/wide_deep).
diff --git a/site/en/r1/tutorials/representation/unicode.ipynb b/site/en/r1/tutorials/representation/unicode.ipynb
index 98aaacff5b9..f76977c3c92 100644
--- a/site/en/r1/tutorials/representation/unicode.ipynb
+++ b/site/en/r1/tutorials/representation/unicode.ipynb
@@ -136,7 +136,7 @@
"id": "jsMPnjb6UDJ1"
},
"source": [
- "Note: When using python to construct strings, the handling of unicode differs betweeen v2 and v3. In v2, unicode strings are indicated by the \"u\" prefix, as above. In v3, strings are unicode-encoded by default."
+ "Note: When using python to construct strings, the handling of unicode differs between v2 and v3. In v2, unicode strings are indicated by the \"u\" prefix, as above. In v3, strings are unicode-encoded by default."
]
},
{
@@ -425,7 +425,7 @@
"source": [
"### Character substrings\n",
"\n",
- "Similarly, the `tf.strings.substr` operation accepts the \"`unit`\" parameter, and uses it to determine what kind of offsets the \"`pos`\" and \"`len`\" paremeters contain."
+ "Similarly, the `tf.strings.substr` operation accepts the \"`unit`\" parameter, and uses it to determine what kind of offsets the \"`pos`\" and \"`len`\" parameters contain."
]
},
{
@@ -587,7 +587,7 @@
"id": "CapnbShuGU8i"
},
"source": [
- "First, we decode the sentences into character codepoints, and find the script identifeir for each character."
+ "First, we decode the sentences into character codepoints, and find the script identifier for each character."
]
},
{
diff --git a/site/en/r1/tutorials/representation/word2vec.md b/site/en/r1/tutorials/representation/word2vec.md
index f6a27c68f3c..517a5dbc5c5 100644
--- a/site/en/r1/tutorials/representation/word2vec.md
+++ b/site/en/r1/tutorials/representation/word2vec.md
@@ -36,7 +36,7 @@ like to get your hands dirty with the details.
Image and audio processing systems work with rich, high-dimensional datasets
encoded as vectors of the individual raw pixel-intensities for image data, or
-e.g. power spectral density coefficients for audio data. For tasks like object
+e.g., power spectral density coefficients for audio data. For tasks like object
or speech recognition we know that all the information required to successfully
perform the task is encoded in the data (because humans can perform these tasks
from the raw data). However, natural language processing systems traditionally
@@ -109,7 +109,7 @@ $$
where \\(\text{score}(w_t, h)\\) computes the compatibility of word \\(w_t\\)
with the context \\(h\\) (a dot product is commonly used). We train this model
by maximizing its [log-likelihood](https://en.wikipedia.org/wiki/Likelihood_function)
-on the training set, i.e. by maximizing
+on the training set, i.e., by maximizing
$$
\begin{align}
@@ -176,7 +176,7 @@ As an example, let's consider the dataset
We first form a dataset of words and the contexts in which they appear. We
could define 'context' in any way that makes sense, and in fact people have
looked at syntactic contexts (i.e. the syntactic dependents of the current
-target word, see e.g.
+target word, see e.g.,
[Levy et al.](https://levyomer.files.wordpress.com/2014/04/dependency-based-word-embeddings-acl-2014.pdf)),
words-to-the-left of the target, words-to-the-right of the target, etc. For now,
let's stick to the vanilla definition and define 'context' as the window
@@ -204,7 +204,7 @@ where the goal is to predict `the` from `quick`. We select `num_noise` number
of noisy (contrastive) examples by drawing from some noise distribution,
typically the unigram distribution, \\(P(w)\\). For simplicity let's say
`num_noise=1` and we select `sheep` as a noisy example. Next we compute the
-loss for this pair of observed and noisy examples, i.e. the objective at time
+loss for this pair of observed and noisy examples, i.e., the objective at time
step \\(t\\) becomes
$$J^{(t)}_\text{NEG} = \log Q_\theta(D=1 | \text{the, quick}) +
@@ -212,7 +212,7 @@ $$J^{(t)}_\text{NEG} = \log Q_\theta(D=1 | \text{the, quick}) +
The goal is to make an update to the embedding parameters \\(\theta\\) to improve
(in this case, maximize) this objective function. We do this by deriving the
-gradient of the loss with respect to the embedding parameters \\(\theta\\), i.e.
+gradient of the loss with respect to the embedding parameters \\(\theta\\), i.e.,
\\(\frac{\partial}{\partial \theta} J_\text{NEG}\\) (luckily TensorFlow provides
easy helper functions for doing this!). We then perform an update to the
embeddings by taking a small step in the direction of the gradient. When this
@@ -227,7 +227,7 @@ When we inspect these visualizations it becomes apparent that the vectors
capture some general, and in fact quite useful, semantic information about
words and their relationships to one another. It was very interesting when we
first discovered that certain directions in the induced vector space specialize
-towards certain semantic relationships, e.g. *male-female*, *verb tense* and
+towards certain semantic relationships, e.g., *male-female*, *verb tense* and
even *country-capital* relationships between words, as illustrated in the figure
below (see also for example
[Mikolov et al., 2013](https://www.aclweb.org/anthology/N13-1090)).
@@ -327,7 +327,7 @@ for inputs, labels in generate_batch(...):
```
See the full example code in
-[tensorflow/examples/tutorials/word2vec/word2vec_basic.py](https://www.tensorflow.org/code/tensorflow/examples/tutorials/word2vec/word2vec_basic.py).
+[tensorflow/examples/tutorials/word2vec/word2vec_basic.py](https://github.com/tensorflow/tensorflow/blob/r1.15/tensorflow/examples/tutorials/word2vec/word2vec_basic.py).
## Visualizing the learned embeddings
@@ -341,7 +341,7 @@ t-SNE.
Et voila! As expected, words that are similar end up clustering nearby each
other. For a more heavyweight implementation of word2vec that showcases more of
the advanced features of TensorFlow, see the implementation in
-[models/tutorials/embedding/word2vec.py](https://github.com/tensorflow/models/tree/master/research/tutorials/embedding/word2vec.py).
+[models/tutorials/embedding/word2vec.py](https://github.com/tensorflow/models/tree/r1.15/research/tutorials/embedding/word2vec.py).
## Evaluating embeddings: analogical reasoning
@@ -357,7 +357,7 @@ Download the dataset for this task from
To see how we do this evaluation, have a look at the `build_eval_graph()` and
`eval()` functions in
-[models/tutorials/embedding/word2vec.py](https://github.com/tensorflow/models/tree/master/research/tutorials/embedding/word2vec.py).
+[models/tutorials/embedding/word2vec.py](https://github.com/tensorflow/models/tree/r1.15/research/tutorials/embedding/word2vec.py).
The choice of hyperparameters can strongly influence the accuracy on this task.
To achieve state-of-the-art performance on this task requires training over a
diff --git a/site/en/r1/tutorials/sequences/audio_recognition.md b/site/en/r1/tutorials/sequences/audio_recognition.md
index 8ad71b88a3c..0388514ec92 100644
--- a/site/en/r1/tutorials/sequences/audio_recognition.md
+++ b/site/en/r1/tutorials/sequences/audio_recognition.md
@@ -159,9 +159,9 @@ accuracy. If the training accuracy increases but the validation doesn't, that's
a sign that overfitting is occurring, and your model is only learning things
about the training clips, not broader patterns that generalize.
-## Tensorboard
+## TensorBoard
-A good way to visualize how the training is progressing is using Tensorboard. By
+A good way to visualize how the training is progressing is using TensorBoard. By
default, the script saves out events to /tmp/retrain_logs, and you can load
these by running:
diff --git a/site/en/r1/tutorials/sequences/recurrent.md b/site/en/r1/tutorials/sequences/recurrent.md
index 6654795d944..e7c1f8c0b16 100644
--- a/site/en/r1/tutorials/sequences/recurrent.md
+++ b/site/en/r1/tutorials/sequences/recurrent.md
@@ -2,7 +2,7 @@
## Introduction
-See [Understanding LSTM Networks](https://colah.github.io/posts/2015-08-Understanding-LSTMs/){:.external}
+See [Understanding LSTM Networks](https://colah.github.io/posts/2015-08-Understanding-LSTMs/)
for an introduction to recurrent neural networks and LSTMs.
## Language Modeling
diff --git a/site/en/r1/tutorials/sequences/recurrent_quickdraw.md b/site/en/r1/tutorials/sequences/recurrent_quickdraw.md
index 435076f629c..d6a85377d17 100644
--- a/site/en/r1/tutorials/sequences/recurrent_quickdraw.md
+++ b/site/en/r1/tutorials/sequences/recurrent_quickdraw.md
@@ -109,7 +109,7 @@ This download will take a while and download a bit more than 23GB of data.
To convert the `ndjson` files to
[TFRecord](../../api_guides/python/python_io.md#TFRecords_Format_Details) files containing
-[`tf.train.Example`](https://www.tensorflow.org/code/tensorflow/core/example/example.proto)
+[`tf.train.Example`](https://github.com/tensorflow/tensorflow/blob/r1.15/tensorflow/core/example/example.proto)
protos run the following command.
```shell
@@ -213,7 +213,7 @@ screen coordinates and normalize the size such that the drawing has unit height.
Finally, we compute the differences between consecutive points and store these
as a `VarLenFeature` in a
-[tensorflow.Example](https://www.tensorflow.org/code/tensorflow/core/example/example.proto)
+[tensorflow.Example](https://github.com/tensorflow/tensorflow/blob/r1.15/tensorflow/core/example/example.proto)
under the key `ink`. In addition we store the `class_index` as a single entry
`FixedLengthFeature` and the `shape` of the `ink` as a `FixedLengthFeature` of
length 2.
diff --git a/site/en/tutorials/distribute/multi_worker_with_estimator.ipynb b/site/en/tutorials/distribute/multi_worker_with_estimator.ipynb
index 2abf05aa9f8..fcee0618854 100644
--- a/site/en/tutorials/distribute/multi_worker_with_estimator.ipynb
+++ b/site/en/tutorials/distribute/multi_worker_with_estimator.ipynb
@@ -186,7 +186,7 @@
"\n",
"There are two components of `TF_CONFIG`: `cluster` and `task`. `cluster` provides information about the entire cluster, namely the workers and parameter servers in the cluster. `task` provides information about the current task. The first component `cluster` is the same for all workers and parameter servers in the cluster, and the second component `task` is different on each worker and parameter server and specifies its own `type` and `index`. In this example, the task `type` is `worker` and the task `index` is `0`.\n",
"\n",
- "For illustration purposes, this tutorial shows how to set a `TF_CONFIG` with 2 workers on `localhost`. In practice, you would create multiple workers on an external IP address and port, and set `TF_CONFIG` on each worker appropriately, i.e. modify the task `index`.\n",
+ "For illustration purposes, this tutorial shows how to set a `TF_CONFIG` with 2 workers on `localhost`. In practice, you would create multiple workers on an external IP address and port, and set `TF_CONFIG` on each worker appropriately, i.e., modify the task `index`.\n",
"\n",
"Warning: *Do not execute the following code in Colab.* TensorFlow's runtime will attempt to create a gRPC server at the specified IP address and port, which will likely fail. See the [keras version](multi_worker_with_keras.ipynb) of this tutorial for an example of how you can test run multiple workers on a single machine.\n",
"\n",
diff --git a/site/en/tutorials/estimator/keras_model_to_estimator.ipynb b/site/en/tutorials/estimator/keras_model_to_estimator.ipynb
index 7b34e283ef3..be97a38b6eb 100644
--- a/site/en/tutorials/estimator/keras_model_to_estimator.ipynb
+++ b/site/en/tutorials/estimator/keras_model_to_estimator.ipynb
@@ -68,7 +68,7 @@
"id": "Dhcq8Ds4mCtm"
},
"source": [
- "> Warning: Estimators are not recommended for new code. Estimators run `v1.Session`-style code which is more difficult to write correctly, and can behave unexpectedly, especially when combined with TF 2 code. Estimators do fall under our [compatibility guarantees](https://tensorflow.org/guide/versions), but will receive no fixes other than security vulnerabilities. See the [migration guide](https://tensorflow.org/guide/migrate) for details."
+ "> Warning: TensorFlow 2.15 included the final release of the `tf-estimator` package. Estimators will not be available in TensorFlow 2.16 or after. See the [migration guide](https://tensorflow.org/guide/migrate/migrating_estimator) for more information about how to convert off of Estimators."
]
},
{
diff --git a/site/en/tutorials/estimator/linear.ipynb b/site/en/tutorials/estimator/linear.ipynb
index 7732ebe3b9e..a26ffe2df4f 100644
--- a/site/en/tutorials/estimator/linear.ipynb
+++ b/site/en/tutorials/estimator/linear.ipynb
@@ -61,7 +61,7 @@
"id": "JOccPOFMm5Tc"
},
"source": [
- "> Warning: Estimators are not recommended for new code. Estimators run `v1.Session`-style code which is more difficult to write correctly, and can behave unexpectedly, especially when combined with TF 2 code. Estimators do fall under our [compatibility guarantees](https://tensorflow.org/guide/versions), but will receive no fixes other than security vulnerabilities. See the [migration guide](https://tensorflow.org/guide/migrate) for details."
+ "> Warning: TensorFlow 2.15 included the final release of the `tf-estimator` package. Estimators will not be available in TensorFlow 2.16 or after. See the [migration guide](https://tensorflow.org/guide/migrate/migrating_estimator) for more information about how to convert off of Estimators."
]
},
{
diff --git a/site/en/tutorials/estimator/premade.ipynb b/site/en/tutorials/estimator/premade.ipynb
index a34096ea2b8..dc81847c7cd 100644
--- a/site/en/tutorials/estimator/premade.ipynb
+++ b/site/en/tutorials/estimator/premade.ipynb
@@ -68,7 +68,7 @@
"id": "stQiPWL6ni6_"
},
"source": [
- "> Warning: Estimators are not recommended for new code. Estimators run `v1.Session`-style code which is more difficult to write correctly, and can behave unexpectedly, especially when combined with TF 2 code. Estimators do fall under [compatibility guarantees](https://tensorflow.org/guide/versions), but will receive no fixes other than security vulnerabilities. See the [migration guide](https://tensorflow.org/guide/migrate) for details."
+ "> Warning: TensorFlow 2.15 included the final release of the `tf-estimator` package. Estimators will not be available in TensorFlow 2.16 or after. See the [migration guide](https://tensorflow.org/guide/migrate/migrating_estimator) for more information about how to convert off of Estimators."
]
},
{
diff --git a/site/en/tutorials/generative/autoencoder.ipynb b/site/en/tutorials/generative/autoencoder.ipynb
index d81628fb401..1b2a6fcd2a8 100644
--- a/site/en/tutorials/generative/autoencoder.ipynb
+++ b/site/en/tutorials/generative/autoencoder.ipynb
@@ -6,9 +6,16 @@
"id": "Ndo4ERqnwQOU"
},
"source": [
- "##### Copyright 2020 The TensorFlow Authors."
+ "##### Copyright 2024 The TensorFlow Authors."
]
},
+ {
+ "metadata": {
+ "id": "13rwRG5Jec7n"
+ },
+ "cell_type": "markdown",
+ "source": []
+ },
{
"cell_type": "code",
"execution_count": null,
@@ -76,7 +83,7 @@
"source": [
"This tutorial introduces autoencoders with three examples: the basics, image denoising, and anomaly detection.\n",
"\n",
- "An autoencoder is a special type of neural network that is trained to copy its input to its output. For example, given an image of a handwritten digit, an autoencoder first encodes the image into a lower dimensional latent representation, then decodes the latent representation back to an image. An autoencoder learns to compress the data while minimizing the reconstruction error. \n",
+ "An autoencoder is a special type of neural network that is trained to copy its input to its output. For example, given an image of a handwritten digit, an autoencoder first encodes the image into a lower dimensional latent representation, then decodes the latent representation back to an image. An autoencoder learns to compress the data while minimizing the reconstruction error.\n",
"\n",
"To learn more about autoencoders, please consider reading chapter 14 from [Deep Learning](https://www.deeplearningbook.org/) by Ian Goodfellow, Yoshua Bengio, and Aaron Courville."
]
@@ -117,7 +124,7 @@
},
"source": [
"## Load the dataset\n",
- "To start, you will train the basic autoencoder using the Fashion MNIST dataset. Each image in this dataset is 28x28 pixels. "
+ "To start, you will train the basic autoencoder using the Fashion MNIST dataset. Each image in this dataset is 28x28 pixels."
]
},
{
@@ -169,7 +176,7 @@
" layers.Dense(latent_dim, activation='relu'),\n",
" ])\n",
" self.decoder = tf.keras.Sequential([\n",
- " layers.Dense(tf.math.reduce_prod(shape), activation='sigmoid'),\n",
+ " layers.Dense(tf.math.reduce_prod(shape).numpy(), activation='sigmoid'),\n",
" layers.Reshape(shape)\n",
" ])\n",
"\n",
@@ -331,8 +338,8 @@
"outputs": [],
"source": [
"noise_factor = 0.2\n",
- "x_train_noisy = x_train + noise_factor * tf.random.normal(shape=x_train.shape) \n",
- "x_test_noisy = x_test + noise_factor * tf.random.normal(shape=x_test.shape) \n",
+ "x_train_noisy = x_train + noise_factor * tf.random.normal(shape=x_train.shape)\n",
+ "x_test_noisy = x_test + noise_factor * tf.random.normal(shape=x_test.shape)\n",
"\n",
"x_train_noisy = tf.clip_by_value(x_train_noisy, clip_value_min=0., clip_value_max=1.)\n",
"x_test_noisy = tf.clip_by_value(x_test_noisy, clip_value_min=0., clip_value_max=1.)"
@@ -657,7 +664,7 @@
"id": "wVcTBDo-CqFS"
},
"source": [
- "Plot a normal ECG. "
+ "Plot a normal ECG."
]
},
{
@@ -721,12 +728,12 @@
" layers.Dense(32, activation=\"relu\"),\n",
" layers.Dense(16, activation=\"relu\"),\n",
" layers.Dense(8, activation=\"relu\")])\n",
- " \n",
+ "\n",
" self.decoder = tf.keras.Sequential([\n",
" layers.Dense(16, activation=\"relu\"),\n",
" layers.Dense(32, activation=\"relu\"),\n",
" layers.Dense(140, activation=\"sigmoid\")])\n",
- " \n",
+ "\n",
" def call(self, x):\n",
" encoded = self.encoder(x)\n",
" decoded = self.decoder(encoded)\n",
@@ -763,8 +770,8 @@
},
"outputs": [],
"source": [
- "history = autoencoder.fit(normal_train_data, normal_train_data, \n",
- " epochs=20, \n",
+ "history = autoencoder.fit(normal_train_data, normal_train_data,\n",
+ " epochs=20,\n",
" batch_size=512,\n",
" validation_data=(test_data, test_data),\n",
" shuffle=True)"
@@ -908,7 +915,7 @@
"id": "uEGlA1Be50Nj"
},
"source": [
- "Note: There are other strategies you could use to select a threshold value above which test examples should be classified as anomalous, the correct approach will depend on your dataset. You can learn more with the links at the end of this tutorial. "
+ "Note: There are other strategies you could use to select a threshold value above which test examples should be classified as anomalous, the correct approach will depend on your dataset. You can learn more with the links at the end of this tutorial."
]
},
{
@@ -917,7 +924,7 @@
"id": "zpLSDAeb51D_"
},
"source": [
- "If you examine the reconstruction error for the anomalous examples in the test set, you'll notice most have greater reconstruction error than the threshold. By varing the threshold, you can adjust the [precision](https://developers.google.com/machine-learning/glossary#precision) and [recall](https://developers.google.com/machine-learning/glossary#recall) of your classifier. "
+ "If you examine the reconstruction error for the anomalous examples in the test set, you'll notice most have greater reconstruction error than the threshold. By varing the threshold, you can adjust the [precision](https://developers.google.com/machine-learning/glossary#precision) and [recall](https://developers.google.com/machine-learning/glossary#recall) of your classifier."
]
},
{
@@ -992,8 +999,18 @@
"metadata": {
"accelerator": "GPU",
"colab": {
- "collapsed_sections": [],
- "name": "autoencoder.ipynb",
+ "gpuType": "T4",
+ "private_outputs": true,
+ "provenance": [
+ {
+ "file_id": "17gKB2bKebV2DzoYIMFzyEXA5uDnwWOvT",
+ "timestamp": 1712793165979
+ },
+ {
+ "file_id": "https://github.com/tensorflow/docs/blob/master/site/en/tutorials/generative/autoencoder.ipynb",
+ "timestamp": 1712792176273
+ }
+ ],
"toc_visible": true
},
"kernelspec": {
diff --git a/site/en/tutorials/generative/cyclegan.ipynb b/site/en/tutorials/generative/cyclegan.ipynb
index 4c2b3ba8777..313be519591 100644
--- a/site/en/tutorials/generative/cyclegan.ipynb
+++ b/site/en/tutorials/generative/cyclegan.ipynb
@@ -154,7 +154,7 @@
"This is similar to what was done in [pix2pix](https://www.tensorflow.org/tutorials/generative/pix2pix#load_the_dataset)\n",
"\n",
"* In random jittering, the image is resized to `286 x 286` and then randomly cropped to `256 x 256`.\n",
- "* In random mirroring, the image is randomly flipped horizontally i.e. left to right."
+ "* In random mirroring, the image is randomly flipped horizontally i.e., left to right."
]
},
{
diff --git a/site/en/tutorials/generative/data_compression.ipynb b/site/en/tutorials/generative/data_compression.ipynb
index b6c043c0598..f756f088acd 100644
--- a/site/en/tutorials/generative/data_compression.ipynb
+++ b/site/en/tutorials/generative/data_compression.ipynb
@@ -821,7 +821,7 @@
{
"cell_type": "markdown",
"metadata": {
- "id": "3ELLMAN1OwMQ"
+ "id": "3ELLMANN1OwMQ"
},
"source": [
"The strings begin to get much shorter now, on the order of one byte per digit. However, this comes at a cost. More digits are becoming unrecognizable.\n",
diff --git a/site/en/tutorials/generative/pix2pix.ipynb b/site/en/tutorials/generative/pix2pix.ipynb
index 5912fab9be3..0709353942d 100644
--- a/site/en/tutorials/generative/pix2pix.ipynb
+++ b/site/en/tutorials/generative/pix2pix.ipynb
@@ -70,16 +70,16 @@
"id": "ITZuApL56Mny"
},
"source": [
- "This tutorial demonstrates how to build and train a conditional generative adversarial network (cGAN) called pix2pix that learns a mapping from input images to output images, as described in [Image-to-image translation with conditional adversarial networks](https://arxiv.org/abs/1611.07004){:.external} by Isola et al. (2017). pix2pix is not application specific—it can be applied to a wide range of tasks, including synthesizing photos from label maps, generating colorized photos from black and white images, turning Google Maps photos into aerial images, and even transforming sketches into photos.\n",
+ "This tutorial demonstrates how to build and train a conditional generative adversarial network (cGAN) called pix2pix that learns a mapping from input images to output images, as described in [Image-to-image translation with conditional adversarial networks](https://arxiv.org/abs/1611.07004) by Isola et al. (2017). pix2pix is not application specific—it can be applied to a wide range of tasks, including synthesizing photos from label maps, generating colorized photos from black and white images, turning Google Maps photos into aerial images, and even transforming sketches into photos.\n",
"\n",
- "In this example, your network will generate images of building facades using the [CMP Facade Database](http://cmp.felk.cvut.cz/~tylecr1/facade/) provided by the [Center for Machine Perception](http://cmp.felk.cvut.cz/){:.external} at the [Czech Technical University in Prague](https://www.cvut.cz/){:.external}. To keep it short, you will use a [preprocessed copy](https://efrosgans.eecs.berkeley.edu/pix2pix/datasets/){:.external} of this dataset created by the pix2pix authors.\n",
+ "In this example, your network will generate images of building facades using the [CMP Facade Database](http://cmp.felk.cvut.cz/~tylecr1/facade/) provided by the [Center for Machine Perception](http://cmp.felk.cvut.cz/) at the [Czech Technical University in Prague](https://www.cvut.cz/). To keep it short, you will use a [preprocessed copy](https://efrosgans.eecs.berkeley.edu/pix2pix/datasets/) of this dataset created by the pix2pix authors.\n",
"\n",
"In the pix2pix cGAN, you condition on input images and generate corresponding output images. cGANs were first proposed in [Conditional Generative Adversarial Nets](https://arxiv.org/abs/1411.1784) (Mirza and Osindero, 2014)\n",
"\n",
"The architecture of your network will contain:\n",
"\n",
- "- A generator with a [U-Net](https://arxiv.org/abs/1505.04597){:.external}-based architecture.\n",
- "- A discriminator represented by a convolutional PatchGAN classifier (proposed in the [pix2pix paper](https://arxiv.org/abs/1611.07004){:.external}).\n",
+ "- A generator with a [U-Net](https://arxiv.org/abs/1505.04597)-based architecture.\n",
+ "- A discriminator represented by a convolutional PatchGAN classifier (proposed in the [pix2pix paper](https://arxiv.org/abs/1611.07004)).\n",
"\n",
"Note that each epoch can take around 15 seconds on a single V100 GPU.\n",
"\n",
@@ -125,7 +125,7 @@
"source": [
"## Load the dataset\n",
"\n",
- "Download the CMP Facade Database data (30MB). Additional datasets are available in the same format [here](http://efrosgans.eecs.berkeley.edu/pix2pix/datasets/){:.external}. In Colab you can select other datasets from the drop-down menu. Note that some of the other datasets are significantly larger (`edges2handbags` is 8GB in size). "
+ "Download the CMP Facade Database data (30MB). Additional datasets are available in the same format [here](http://efrosgans.eecs.berkeley.edu/pix2pix/datasets/). In Colab you can select other datasets from the drop-down menu. Note that some of the other datasets are significantly larger (`edges2handbags` is 8GB in size). "
]
},
{
@@ -274,13 +274,13 @@
"id": "PVuZQTfI_c-s"
},
"source": [
- "As described in the [pix2pix paper](https://arxiv.org/abs/1611.07004){:.external}, you need to apply random jittering and mirroring to preprocess the training set.\n",
+ "As described in the [pix2pix paper](https://arxiv.org/abs/1611.07004), you need to apply random jittering and mirroring to preprocess the training set.\n",
"\n",
"Define several functions that:\n",
"\n",
"1. Resize each `256 x 256` image to a larger height and width—`286 x 286`.\n",
"2. Randomly crop it back to `256 x 256`.\n",
- "3. Randomly flip the image horizontally i.e. left to right (random mirroring).\n",
+ "3. Randomly flip the image horizontally i.e., left to right (random mirroring).\n",
"4. Normalize the images to the `[-1, 1]` range."
]
},
@@ -490,7 +490,7 @@
"source": [
"## Build the generator\n",
"\n",
- "The generator of your pix2pix cGAN is a _modified_ [U-Net](https://arxiv.org/abs/1505.04597){:.external}. A U-Net consists of an encoder (downsampler) and decoder (upsampler). (You can find out more about it in the [Image segmentation](../images/segmentation.ipynb) tutorial and on the [U-Net project website](https://lmb.informatik.uni-freiburg.de/people/ronneber/u-net/){:.external}.)\n",
+ "The generator of your pix2pix cGAN is a _modified_ [U-Net](https://arxiv.org/abs/1505.04597). A U-Net consists of an encoder (downsampler) and decoder (upsampler). (You can find out more about it in the [Image segmentation](../images/segmentation.ipynb) tutorial and on the [U-Net project website](https://lmb.informatik.uni-freiburg.de/people/ronneber/u-net/).)\n",
"\n",
"- Each block in the encoder is: Convolution -> Batch normalization -> Leaky ReLU\n",
"- Each block in the decoder is: Transposed convolution -> Batch normalization -> Dropout (applied to the first 3 blocks) -> ReLU\n",
@@ -722,7 +722,7 @@
"source": [
"### Define the generator loss\n",
"\n",
- "GANs learn a loss that adapts to the data, while cGANs learn a structured loss that penalizes a possible structure that differs from the network output and the target image, as described in the [pix2pix paper](https://arxiv.org/abs/1611.07004){:.external}.\n",
+ "GANs learn a loss that adapts to the data, while cGANs learn a structured loss that penalizes a possible structure that differs from the network output and the target image, as described in the [pix2pix paper](https://arxiv.org/abs/1611.07004).\n",
"\n",
"- The generator loss is a sigmoid cross-entropy loss of the generated images and an **array of ones**.\n",
"- The pix2pix paper also mentions the L1 loss, which is a MAE (mean absolute error) between the generated image and the target image.\n",
@@ -797,7 +797,7 @@
"source": [
"## Build the discriminator\n",
"\n",
- "The discriminator in the pix2pix cGAN is a convolutional PatchGAN classifier—it tries to classify if each image _patch_ is real or not real, as described in the [pix2pix paper](https://arxiv.org/abs/1611.07004){:.external}.\n",
+ "The discriminator in the pix2pix cGAN is a convolutional PatchGAN classifier—it tries to classify if each image _patch_ is real or not real, as described in the [pix2pix paper](https://arxiv.org/abs/1611.07004).\n",
"\n",
"- Each block in the discriminator is: Convolution -> Batch normalization -> Leaky ReLU.\n",
"- The shape of the output after the last layer is `(batch_size, 30, 30, 1)`.\n",
@@ -937,7 +937,7 @@
"source": [
"The training procedure for the discriminator is shown below.\n",
"\n",
- "To learn more about the architecture and the hyperparameters you can refer to the [pix2pix paper](https://arxiv.org/abs/1611.07004){:.external}."
+ "To learn more about the architecture and the hyperparameters you can refer to the [pix2pix paper](https://arxiv.org/abs/1611.07004)."
]
},
{
diff --git a/site/en/tutorials/generative/style_transfer.ipynb b/site/en/tutorials/generative/style_transfer.ipynb
index 06469c33c91..c8f1376624e 100644
--- a/site/en/tutorials/generative/style_transfer.ipynb
+++ b/site/en/tutorials/generative/style_transfer.ipynb
@@ -1110,10 +1110,9 @@
"\n",
"try:\n",
" from google.colab import files\n",
- "except ImportError:\n",
- " pass\n",
- "else:\n",
- " files.download(file_name)"
+ " files.download(file_name)\n",
+ "except (ImportError, AttributeError):\n",
+ " pass"
]
},
{
diff --git a/site/en/tutorials/images/classification.ipynb b/site/en/tutorials/images/classification.ipynb
index f54da222cce..73a0dafcd07 100644
--- a/site/en/tutorials/images/classification.ipynb
+++ b/site/en/tutorials/images/classification.ipynb
@@ -797,7 +797,7 @@
"source": [
"## Dropout\n",
"\n",
- "Another technique to reduce overfitting is to introduce [dropout](https://developers.google.com/machine-learning/glossary#dropout_regularization){:.external} regularization to the network.\n",
+ "Another technique to reduce overfitting is to introduce [dropout](https://developers.google.com/machine-learning/glossary#dropout_regularization) regularization to the network.\n",
"\n",
"When you apply dropout to a layer, it randomly drops out (by setting the activation to zero) a number of output units from the layer during the training process. Dropout takes a fractional number as its input value, in the form such as 0.1, 0.2, 0.4, etc. This means dropping out 10%, 20% or 40% of the output units randomly from the applied layer.\n",
"\n",
diff --git a/site/en/tutorials/images/data_augmentation.ipynb b/site/en/tutorials/images/data_augmentation.ipynb
index bdc7ae0c56a..8a1eaaabec4 100644
--- a/site/en/tutorials/images/data_augmentation.ipynb
+++ b/site/en/tutorials/images/data_augmentation.ipynb
@@ -1273,7 +1273,7 @@
"source": [
"# Create a wrapper function for updating seeds.\n",
"def f(x, y):\n",
- " seed = rng.make_seeds(2)[0]\n",
+ " seed = rng.make_seeds(1)[:, 0]\n",
" image, label = augment((x, y), seed)\n",
" return image, label"
]
diff --git a/site/en/tutorials/images/segmentation.ipynb b/site/en/tutorials/images/segmentation.ipynb
index 4bf59cbbd5a..285ef538664 100644
--- a/site/en/tutorials/images/segmentation.ipynb
+++ b/site/en/tutorials/images/segmentation.ipynb
@@ -97,7 +97,10 @@
},
"outputs": [],
"source": [
- "!pip install git+https://github.com/tensorflow/examples.git"
+ "!pip install git+https://github.com/tensorflow/examples.git\n",
+ "!pip install -U keras\n",
+ "!pip install -q tensorflow_datasets\n",
+ "!pip install -q -U tensorflow-text tensorflow"
]
},
{
@@ -108,8 +111,9 @@
},
"outputs": [],
"source": [
- "import tensorflow as tf\n",
+ "import numpy as np\n",
"\n",
+ "import tensorflow as tf\n",
"import tensorflow_datasets as tfds"
]
},
@@ -252,7 +256,7 @@
" # both use the same seed, so they'll make the same random changes.\n",
" self.augment_inputs = tf.keras.layers.RandomFlip(mode=\"horizontal\", seed=seed)\n",
" self.augment_labels = tf.keras.layers.RandomFlip(mode=\"horizontal\", seed=seed)\n",
- " \n",
+ "\n",
" def call(self, inputs, labels):\n",
" inputs = self.augment_inputs(inputs)\n",
" labels = self.augment_labels(labels)\n",
@@ -450,7 +454,7 @@
"source": [
"## Train the model\n",
"\n",
- "Now, all that is left to do is to compile and train the model. \n",
+ "Now, all that is left to do is to compile and train the model.\n",
"\n",
"Since this is a multiclass classification problem, use the `tf.keras.losses.SparseCategoricalCrossentropy` loss function with the `from_logits` argument set to `True`, since the labels are scalar integers instead of vectors of scores for each pixel of every class.\n",
"\n",
@@ -490,7 +494,7 @@
},
"outputs": [],
"source": [
- "tf.keras.utils.plot_model(model, show_shapes=True)"
+ "tf.keras.utils.plot_model(model, show_shapes=True, expand_nested=True, dpi=64)"
]
},
{
@@ -695,12 +699,14 @@
},
"outputs": [],
"source": [
- "label = [0,0]\n",
- "prediction = [[-3., 0], [-3, 0]] \n",
- "sample_weight = [1, 10] \n",
+ "label = np.array([0,0])\n",
+ "prediction = np.array([[-3., 0], [-3, 0]])\n",
+ "sample_weight = [1, 10]\n",
"\n",
- "loss = tf.keras.losses.SparseCategoricalCrossentropy(from_logits=True,\n",
- " reduction=tf.keras.losses.Reduction.NONE)\n",
+ "loss = tf.keras.losses.SparseCategoricalCrossentropy(\n",
+ " from_logits=True,\n",
+ " reduction=tf.keras.losses.Reduction.NONE\n",
+ ")\n",
"loss(label, prediction, sample_weight).numpy()"
]
},
@@ -729,7 +735,7 @@
" class_weights = tf.constant([2.0, 2.0, 1.0])\n",
" class_weights = class_weights/tf.reduce_sum(class_weights)\n",
"\n",
- " # Create an image of `sample_weights` by using the label at each pixel as an \n",
+ " # Create an image of `sample_weights` by using the label at each pixel as an\n",
" # index into the `class weights` .\n",
" sample_weights = tf.gather(class_weights, indices=tf.cast(label, tf.int32))\n",
"\n",
@@ -811,7 +817,6 @@
"metadata": {
"accelerator": "GPU",
"colab": {
- "collapsed_sections": [],
"name": "segmentation.ipynb",
"toc_visible": true
},
diff --git a/site/en/tutorials/images/transfer_learning.ipynb b/site/en/tutorials/images/transfer_learning.ipynb
index 6406ccdce74..172bb2700b4 100644
--- a/site/en/tutorials/images/transfer_learning.ipynb
+++ b/site/en/tutorials/images/transfer_learning.ipynb
@@ -585,7 +585,7 @@
},
"outputs": [],
"source": [
- "prediction_layer = tf.keras.layers.Dense(1)\n",
+ "prediction_layer = tf.keras.layers.Dense(1, activation='sigmoid')\n",
"prediction_batch = prediction_layer(feature_batch_average)\n",
"print(prediction_batch.shape)"
]
@@ -667,7 +667,7 @@
"source": [
"### Compile the model\n",
"\n",
- "Compile the model before training it. Since there are two classes, use the `tf.keras.losses.BinaryCrossentropy` loss with `from_logits=True` since the model provides a linear output."
+ "Compile the model before training it. Since there are two classes and a sigmoid oputput, use the `BinaryAccuracy`."
]
},
{
@@ -680,8 +680,8 @@
"source": [
"base_learning_rate = 0.0001\n",
"model.compile(optimizer=tf.keras.optimizers.Adam(learning_rate=base_learning_rate),\n",
- " loss=tf.keras.losses.BinaryCrossentropy(from_logits=True),\n",
- " metrics=[tf.keras.metrics.BinaryAccuracy(threshold=0, name='accuracy')])"
+ " loss=tf.keras.losses.BinaryCrossentropy(),\n",
+ " metrics=[tf.keras.metrics.BinaryAccuracy(threshold=0.5, name='accuracy')])"
]
},
{
@@ -872,9 +872,9 @@
},
"outputs": [],
"source": [
- "model.compile(loss=tf.keras.losses.BinaryCrossentropy(from_logits=True),\n",
+ "model.compile(loss=tf.keras.losses.BinaryCrossentropy(),\n",
" optimizer = tf.keras.optimizers.RMSprop(learning_rate=base_learning_rate/10),\n",
- " metrics=[tf.keras.metrics.BinaryAccuracy(threshold=0, name='accuracy')])"
+ " metrics=[tf.keras.metrics.BinaryAccuracy(threshold=0.5, name='accuracy')])"
]
},
{
@@ -930,7 +930,7 @@
"\n",
"history_fine = model.fit(train_dataset,\n",
" epochs=total_epochs,\n",
- " initial_epoch=history.epoch[-1],\n",
+ " initial_epoch=len(history.epoch),\n",
" validation_data=validation_dataset)"
]
},
@@ -1049,9 +1049,6 @@
"# Retrieve a batch of images from the test set\n",
"image_batch, label_batch = test_dataset.as_numpy_iterator().next()\n",
"predictions = model.predict_on_batch(image_batch).flatten()\n",
- "\n",
- "# Apply a sigmoid since our model returns logits\n",
- "predictions = tf.nn.sigmoid(predictions)\n",
"predictions = tf.where(predictions < 0.5, 0, 1)\n",
"\n",
"print('Predictions:\\n', predictions.numpy())\n",
@@ -1081,22 +1078,12 @@
"\n",
"To learn more, visit the [Transfer learning guide](https://www.tensorflow.org/guide/keras/transfer_learning).\n"
]
- },
- {
- "cell_type": "code",
- "execution_count": null,
- "metadata": {
- "id": "uKIByL01da8c"
- },
- "outputs": [],
- "source": []
}
],
"metadata": {
"accelerator": "GPU",
"colab": {
"name": "transfer_learning.ipynb",
- "private_outputs": true,
"toc_visible": true
},
"kernelspec": {
diff --git a/site/en/tutorials/interpretability/integrated_gradients.ipynb b/site/en/tutorials/interpretability/integrated_gradients.ipynb
index 2ee792aa4e2..e63c8cdb7a2 100644
--- a/site/en/tutorials/interpretability/integrated_gradients.ipynb
+++ b/site/en/tutorials/interpretability/integrated_gradients.ipynb
@@ -724,7 +724,7 @@
"ax2 = plt.subplot(1, 2, 2)\n",
"# Average across interpolation steps\n",
"average_grads = tf.reduce_mean(path_gradients, axis=[1, 2, 3])\n",
- "# Normalize gradients to 0 to 1 scale. E.g. (x - min(x))/(max(x)-min(x))\n",
+ "# Normalize gradients to 0 to 1 scale. E.g., (x - min(x))/(max(x)-min(x))\n",
"average_grads_norm = (average_grads-tf.math.reduce_min(average_grads))/(tf.math.reduce_max(average_grads)-tf.reduce_min(average_grads))\n",
"ax2.plot(alphas, average_grads_norm)\n",
"ax2.set_title('Average pixel gradients (normalized) over alpha')\n",
diff --git a/site/en/tutorials/keras/save_and_load.ipynb b/site/en/tutorials/keras/save_and_load.ipynb
index 02c8af3a71d..404fa1ee8be 100644
--- a/site/en/tutorials/keras/save_and_load.ipynb
+++ b/site/en/tutorials/keras/save_and_load.ipynb
@@ -854,7 +854,7 @@
" * `from_config(cls, config)` uses the returned config from `get_config` to create a new object. By default, this function will use the config as initialization kwargs (`return cls(**config)`).\n",
"2. Pass the custom objects to the model in one of three ways:\n",
" - Register the custom object with the `@tf.keras.utils.register_keras_serializable` decorator. **(recommended)**\n",
- " - Directly pass the object to the `custom_objects` argument when loading the model. The argument must be a dictionary mapping the string class name to the Python class. E.g. `tf.keras.models.load_model(path, custom_objects={'CustomLayer': CustomLayer})`\n",
+ " - Directly pass the object to the `custom_objects` argument when loading the model. The argument must be a dictionary mapping the string class name to the Python class. E.g., `tf.keras.models.load_model(path, custom_objects={'CustomLayer': CustomLayer})`\n",
" - Use a `tf.keras.utils.custom_object_scope` with the object included in the `custom_objects` dictionary argument, and place a `tf.keras.models.load_model(path)` call within the scope.\n",
"\n",
"Refer to the [Writing layers and models from scratch](https://www.tensorflow.org/guide/keras/custom_layers_and_models) tutorial for examples of custom objects and `get_config`.\n"
diff --git a/site/en/tutorials/keras/text_classification.ipynb b/site/en/tutorials/keras/text_classification.ipynb
index f14964207ff..02e768d7415 100644
--- a/site/en/tutorials/keras/text_classification.ipynb
+++ b/site/en/tutorials/keras/text_classification.ipynb
@@ -267,9 +267,9 @@
"id": "95kkUdRoaeMw"
},
"source": [
- "Next, you will use the `text_dataset_from_directory` utility to create a labeled `tf.data.Dataset`. [tf.data](https://www.tensorflow.org/guide/data) is a powerful collection of tools for working with data. \n",
+ "Next, you will use the `text_dataset_from_directory` utility to create a labeled `tf.data.Dataset`. [tf.data](https://www.tensorflow.org/guide/data) is a powerful collection of tools for working with data.\n",
"\n",
- "When running a machine learning experiment, it is a best practice to divide your dataset into three splits: [train](https://developers.google.com/machine-learning/glossary#training_set), [validation](https://developers.google.com/machine-learning/glossary#validation_set), and [test](https://developers.google.com/machine-learning/glossary#test-set). \n",
+ "When running a machine learning experiment, it is a best practice to divide your dataset into three splits: [train](https://developers.google.com/machine-learning/glossary#training_set), [validation](https://developers.google.com/machine-learning/glossary#validation_set), and [test](https://developers.google.com/machine-learning/glossary#test-set).\n",
"\n",
"The IMDB dataset has already been divided into train and test, but it lacks a validation set. Let's create a validation set using an 80:20 split of the training data by using the `validation_split` argument below."
]
@@ -286,10 +286,10 @@
"seed = 42\n",
"\n",
"raw_train_ds = tf.keras.utils.text_dataset_from_directory(\n",
- " 'aclImdb/train', \n",
- " batch_size=batch_size, \n",
- " validation_split=0.2, \n",
- " subset='training', \n",
+ " 'aclImdb/train',\n",
+ " batch_size=batch_size,\n",
+ " validation_split=0.2,\n",
+ " subset='training',\n",
" seed=seed)"
]
},
@@ -322,7 +322,7 @@
"id": "JWq1SUIrp1a-"
},
"source": [
- "Notice the reviews contain raw text (with punctuation and occasional HTML tags like ` `). You will show how to handle these in the following section. \n",
+ "Notice the reviews contain raw text (with punctuation and occasional HTML tags like ` `). You will show how to handle these in the following section.\n",
"\n",
"The labels are 0 or 1. To see which of these correspond to positive and negative movie reviews, you can check the `class_names` property on the dataset.\n"
]
@@ -366,10 +366,10 @@
"outputs": [],
"source": [
"raw_val_ds = tf.keras.utils.text_dataset_from_directory(\n",
- " 'aclImdb/train', \n",
- " batch_size=batch_size, \n",
- " validation_split=0.2, \n",
- " subset='validation', \n",
+ " 'aclImdb/train',\n",
+ " batch_size=batch_size,\n",
+ " validation_split=0.2,\n",
+ " subset='validation',\n",
" seed=seed)"
]
},
@@ -382,7 +382,7 @@
"outputs": [],
"source": [
"raw_test_ds = tf.keras.utils.text_dataset_from_directory(\n",
- " 'aclImdb/test', \n",
+ " 'aclImdb/test',\n",
" batch_size=batch_size)"
]
},
@@ -394,7 +394,7 @@
"source": [
"### Prepare the dataset for training\n",
"\n",
- "Next, you will standardize, tokenize, and vectorize the data using the helpful `tf.keras.layers.TextVectorization` layer. \n",
+ "Next, you will standardize, tokenize, and vectorize the data using the helpful `tf.keras.layers.TextVectorization` layer.\n",
"\n",
"Standardization refers to preprocessing the text, typically to remove punctuation or HTML elements to simplify the dataset. Tokenization refers to splitting strings into tokens (for example, splitting a sentence into individual words, by splitting on whitespace). Vectorization refers to converting tokens into numbers so they can be fed into a neural network. All of these tasks can be accomplished with this layer.\n",
"\n",
@@ -580,7 +580,7 @@
"\n",
"`.cache()` keeps data in memory after it's loaded off disk. This will ensure the dataset does not become a bottleneck while training your model. If your dataset is too large to fit into memory, you can also use this method to create a performant on-disk cache, which is more efficient to read than many small files.\n",
"\n",
- "`.prefetch()` overlaps data preprocessing and model execution while training. \n",
+ "`.prefetch()` overlaps data preprocessing and model execution while training.\n",
"\n",
"You can learn more about both methods, as well as how to cache data to disk in the [data performance guide](https://www.tensorflow.org/guide/data_performance)."
]
@@ -635,7 +635,7 @@
" layers.Dropout(0.2),\n",
" layers.GlobalAveragePooling1D(),\n",
" layers.Dropout(0.2),\n",
- " layers.Dense(1)])\n",
+ " layers.Dense(1, activation='sigmoid')])\n",
"\n",
"model.summary()"
]
@@ -674,9 +674,9 @@
},
"outputs": [],
"source": [
- "model.compile(loss=losses.BinaryCrossentropy(from_logits=True),\n",
+ "model.compile(loss=losses.BinaryCrossentropy(),\n",
" optimizer='adam',\n",
- " metrics=tf.metrics.BinaryAccuracy(threshold=0.0))"
+ " metrics=[tf.metrics.BinaryAccuracy(threshold=0.5)])"
]
},
{
@@ -861,8 +861,8 @@
")\n",
"\n",
"# Test it with `raw_test_ds`, which yields raw strings\n",
- "loss, accuracy = export_model.evaluate(raw_test_ds)\n",
- "print(accuracy)"
+ "metrics = export_model.evaluate(raw_test_ds, return_dict=True)\n",
+ "print(metrics)"
]
},
{
@@ -884,11 +884,11 @@
},
"outputs": [],
"source": [
- "examples = [\n",
+ "examples = tf.constant([\n",
" \"The movie was great!\",\n",
" \"The movie was okay.\",\n",
" \"The movie was terrible...\"\n",
- "]\n",
+ "])\n",
"\n",
"export_model.predict(examples)"
]
@@ -916,7 +916,7 @@
"\n",
"This tutorial showed how to train a binary classifier from scratch on the IMDB dataset. As an exercise, you can modify this notebook to train a multi-class classifier to predict the tag of a programming question on [Stack Overflow](http://stackoverflow.com/).\n",
"\n",
- "A [dataset](https://storage.googleapis.com/download.tensorflow.org/data/stack_overflow_16k.tar.gz) has been prepared for you to use containing the body of several thousand programming questions (for example, \"How can I sort a dictionary by value in Python?\") posted to Stack Overflow. Each of these is labeled with exactly one tag (either Python, CSharp, JavaScript, or Java). Your task is to take a question as input, and predict the appropriate tag, in this case, Python. \n",
+ "A [dataset](https://storage.googleapis.com/download.tensorflow.org/data/stack_overflow_16k.tar.gz) has been prepared for you to use containing the body of several thousand programming questions (for example, \"How can I sort a dictionary by value in Python?\") posted to Stack Overflow. Each of these is labeled with exactly one tag (either Python, CSharp, JavaScript, or Java). Your task is to take a question as input, and predict the appropriate tag, in this case, Python.\n",
"\n",
"The dataset you will work with contains several thousand questions extracted from the much larger public Stack Overflow dataset on [BigQuery](https://console.cloud.google.com/marketplace/details/stack-exchange/stack-overflow), which contains more than 17 million posts.\n",
"\n",
@@ -950,7 +950,7 @@
"\n",
"1. When plotting accuracy over time, change `binary_accuracy` and `val_binary_accuracy` to `accuracy` and `val_accuracy`, respectively.\n",
"\n",
- "1. Once these changes are complete, you will be able to train a multi-class classifier. "
+ "1. Once these changes are complete, you will be able to train a multi-class classifier."
]
},
{
@@ -968,7 +968,6 @@
"metadata": {
"accelerator": "GPU",
"colab": {
- "collapsed_sections": [],
"name": "text_classification.ipynb",
"toc_visible": true
},
diff --git a/site/en/tutorials/load_data/csv.ipynb b/site/en/tutorials/load_data/csv.ipynb
index 0d4287a425e..7778af974b3 100644
--- a/site/en/tutorials/load_data/csv.ipynb
+++ b/site/en/tutorials/load_data/csv.ipynb
@@ -449,8 +449,8 @@
},
"outputs": [],
"source": [
- "print(calc(1).numpy())\n",
- "print(calc(2).numpy())"
+ "print(calc(np.array([1])).numpy())\n",
+ "print(calc(np.array([2])).numpy())"
]
},
{
@@ -1751,7 +1751,7 @@
"\n",
"for row in font_rows.take(10):\n",
" fonts_dict['font_name'].append(row[0].numpy().decode())\n",
- " fonts_dict['character'].append(chr(row[2].numpy()))\n",
+ " fonts_dict['character'].append(chr(int(row[2].numpy())))\n",
"\n",
"pd.DataFrame(fonts_dict)"
]
diff --git a/site/en/tutorials/load_data/pandas_dataframe.ipynb b/site/en/tutorials/load_data/pandas_dataframe.ipynb
index cee2483a350..b9d0763e068 100644
--- a/site/en/tutorials/load_data/pandas_dataframe.ipynb
+++ b/site/en/tutorials/load_data/pandas_dataframe.ipynb
@@ -92,6 +92,7 @@
},
"outputs": [],
"source": [
+ "import numpy as np\n",
"import pandas as pd\n",
"import tensorflow as tf\n",
"\n",
@@ -292,7 +293,7 @@
"outputs": [],
"source": [
"normalizer = tf.keras.layers.Normalization(axis=-1)\n",
- "normalizer.adapt(numeric_features)"
+ "normalizer.adapt(np.array(numeric_features))"
]
},
{
@@ -446,79 +447,77 @@
"cell_type": "code",
"execution_count": null,
"metadata": {
- "id": "U3QDo-jwHYXc"
+ "id": "voDoA447GBC3"
},
"outputs": [],
"source": [
- "numeric_dict_ds = tf.data.Dataset.from_tensor_slices((dict(numeric_features), target))"
+ "numeric_features_dict = {key: value.to_numpy()[:, tf.newaxis] for key, value in dict(numeric_features).items()}\n",
+ "target_array = target.to_numpy()[:, tf.newaxis]"
]
},
{
- "cell_type": "markdown",
+ "cell_type": "code",
+ "execution_count": null,
"metadata": {
- "id": "yyEERK9ldIi_"
+ "id": "U3QDo-jwHYXc"
},
+ "outputs": [],
"source": [
- "Here are the first three examples from that dataset:"
+ "numeric_dict_ds = tf.data.Dataset.from_tensor_slices((numeric_features_dict , target_array))"
]
},
{
"cell_type": "code",
"execution_count": null,
"metadata": {
- "id": "q0tDwk0VdH6D"
+ "id": "HL4Bf1b7M7DT"
},
"outputs": [],
"source": [
- "for row in numeric_dict_ds.take(3):\n",
- " print(row)"
+ "len(numeric_features_dict)"
]
},
{
"cell_type": "markdown",
"metadata": {
- "id": "DEAM6HAFxlMy"
+ "id": "yyEERK9ldIi_"
},
"source": [
- "### Dictionaries with Keras"
+ "Here are the first three examples from that dataset:"
]
},
{
- "cell_type": "markdown",
+ "cell_type": "code",
+ "execution_count": null,
"metadata": {
- "id": "dnoyoWLWx07i"
+ "id": "q0tDwk0VdH6D"
},
+ "outputs": [],
"source": [
- "Typically, Keras models and layers expect a single input tensor, but these classes can accept and return nested structures of dictionaries, tuples and tensors. These structures are known as \"nests\" (refer to the `tf.nest` module for details).\n",
- "\n",
- "There are two equivalent ways you can write a Keras model that accepts a dictionary as input."
+ "for row in numeric_dict_ds.take(3):\n",
+ " print(row)"
]
},
{
"cell_type": "markdown",
"metadata": {
- "id": "5xUTrm0apDTr"
+ "id": "dnoyoWLWx07i"
},
"source": [
- "#### 1. The Model-subclass style\n",
+ "Typically, Keras models and layers expect a single input tensor, but these classes can accept and return nested structures of dictionaries, tuples and tensors. These structures are known as \"nests\" (refer to the `tf.nest` module for details).\n",
"\n",
- "You write a subclass of `tf.keras.Model` (or `tf.keras.Layer`). You directly handle the inputs, and create the outputs:"
+ "There are two equivalent ways you can write a Keras model that accepts a dictionary as input."
]
},
{
- "cell_type": "code",
- "execution_count": null,
+ "cell_type": "markdown",
"metadata": {
- "id": "Zc3HV99CFRWL"
+ "id": "5xUTrm0apDTr"
},
- "outputs": [],
"source": [
- " def stack_dict(inputs, fun=tf.stack):\n",
- " values = []\n",
- " for key in sorted(inputs.keys()):\n",
- " values.append(tf.cast(inputs[key], tf.float32))\n",
+ "### 1. The Model-subclass style\n",
"\n",
- " return fun(values, axis=-1)"
+ "You write a subclass of `tf.keras.Model` (or `tf.keras.Layer`). You directly handle the inputs, and create the outputs:"
]
},
{
@@ -544,14 +543,23 @@
" tf.keras.layers.Dense(1)\n",
" ])\n",
"\n",
+ " self.concat = tf.keras.layers.Concatenate(axis=1)\n",
+ "\n",
+ " def _stack(self, input_dict):\n",
+ " values = []\n",
+ " for key, value in sorted(input_dict.items()):\n",
+ " values.append(value)\n",
+ "\n",
+ " return self.concat(values)\n",
+ "\n",
" def adapt(self, inputs):\n",
" # Stack the inputs and `adapt` the normalization layer.\n",
- " inputs = stack_dict(inputs)\n",
+ " inputs = self._stack(inputs)\n",
" self.normalizer.adapt(inputs)\n",
"\n",
" def call(self, inputs):\n",
" # Stack the inputs\n",
- " inputs = stack_dict(inputs)\n",
+ " inputs = self._stack(inputs)\n",
" # Run them through all the layers.\n",
" result = self.seq(inputs)\n",
"\n",
@@ -559,7 +567,7 @@
"\n",
"model = MyModel()\n",
"\n",
- "model.adapt(dict(numeric_features))\n",
+ "model.adapt(numeric_features_dict)\n",
"\n",
"model.compile(optimizer='adam',\n",
" loss=tf.keras.losses.BinaryCrossentropy(from_logits=True),\n",
@@ -584,7 +592,7 @@
},
"outputs": [],
"source": [
- "model.fit(dict(numeric_features), target, epochs=5, batch_size=BATCH_SIZE)"
+ "model.fit(numeric_features_dict, target_array, epochs=5, batch_size=BATCH_SIZE)"
]
},
{
@@ -625,7 +633,7 @@
"id": "QIIdxIYm13Ik"
},
"source": [
- "#### 2. The Keras functional style"
+ "### 2. The Keras functional style"
]
},
{
@@ -652,10 +660,13 @@
},
"outputs": [],
"source": [
- "x = stack_dict(inputs, fun=tf.concat)\n",
+ "xs = [value for key, value in sorted(inputs.items())]\n",
+ "\n",
+ "concat = tf.keras.layers.Concatenate(axis=1)\n",
+ "x = concat(xs)\n",
"\n",
"normalizer = tf.keras.layers.Normalization(axis=-1)\n",
- "normalizer.adapt(stack_dict(dict(numeric_features)))\n",
+ "normalizer.adapt(np.concatenate([value for key, value in sorted(numeric_features_dict.items())], axis=1))\n",
"\n",
"x = normalizer(x)\n",
"x = tf.keras.layers.Dense(10, activation='relu')(x)\n",
@@ -678,7 +689,7 @@
},
"outputs": [],
"source": [
- "tf.keras.utils.plot_model(model, rankdir=\"LR\", show_shapes=True)"
+ "tf.keras.utils.plot_model(model, rankdir=\"LR\", show_shapes=True, show_layer_names=True)"
]
},
{
@@ -698,7 +709,7 @@
},
"outputs": [],
"source": [
- "model.fit(dict(numeric_features), target, epochs=5, batch_size=BATCH_SIZE)"
+ "model.fit(numeric_features_dict, target, epochs=5, batch_size=BATCH_SIZE)"
]
},
{
@@ -806,7 +817,7 @@
" else:\n",
" dtype = tf.float32\n",
"\n",
- " inputs[name] = tf.keras.Input(shape=(), name=name, dtype=dtype)"
+ " inputs[name] = tf.keras.Input(shape=(1,), name=name, dtype=dtype)"
]
},
{
@@ -852,9 +863,7 @@
"\n",
"for name in binary_feature_names:\n",
" inp = inputs[name]\n",
- " inp = inp[:, tf.newaxis]\n",
- " float_value = tf.cast(inp, tf.float32)\n",
- " preprocessed.append(float_value)\n",
+ " preprocessed.append(inp)\n",
"\n",
"preprocessed"
]
@@ -879,7 +888,7 @@
"outputs": [],
"source": [
"normalizer = tf.keras.layers.Normalization(axis=-1)\n",
- "normalizer.adapt(stack_dict(dict(numeric_features)))"
+ "normalizer.adapt(np.concatenate([value for key, value in sorted(numeric_features_dict.items())], axis=1))"
]
},
{
@@ -899,11 +908,11 @@
},
"outputs": [],
"source": [
- "numeric_inputs = {}\n",
+ "numeric_inputs = []\n",
"for name in numeric_feature_names:\n",
- " numeric_inputs[name]=inputs[name]\n",
+ " numeric_inputs.append(inputs[name])\n",
"\n",
- "numeric_inputs = stack_dict(numeric_inputs)\n",
+ "numeric_inputs = tf.keras.layers.Concatenate(axis=-1)(numeric_inputs)\n",
"numeric_normalized = normalizer(numeric_inputs)\n",
"\n",
"preprocessed.append(numeric_normalized)\n",
@@ -985,7 +994,7 @@
" else:\n",
" lookup = tf.keras.layers.IntegerLookup(vocabulary=vocab, output_mode='one_hot')\n",
"\n",
- " x = inputs[name][:, tf.newaxis]\n",
+ " x = inputs[name]\n",
" x = lookup(x)\n",
" preprocessed.append(x)"
]
@@ -1036,8 +1045,8 @@
},
"outputs": [],
"source": [
- "preprocesssed_result = tf.concat(preprocessed, axis=-1)\n",
- "preprocesssed_result"
+ "preprocessed_result = tf.keras.layers.Concatenate(axis=1)(preprocessed)\n",
+ "preprocessed_result"
]
},
{
@@ -1057,7 +1066,7 @@
},
"outputs": [],
"source": [
- "preprocessor = tf.keras.Model(inputs, preprocesssed_result)"
+ "preprocessor = tf.keras.Model(inputs, preprocessed_result)"
]
},
{
@@ -1068,7 +1077,7 @@
},
"outputs": [],
"source": [
- "tf.keras.utils.plot_model(preprocessor, rankdir=\"LR\", show_shapes=True)"
+ "tf.keras.utils.plot_model(preprocessor, rankdir=\"LR\", show_shapes=True, show_layer_names=True)"
]
},
{
@@ -1183,6 +1192,17 @@
" metrics=['accuracy'])"
]
},
+ {
+ "cell_type": "code",
+ "execution_count": null,
+ "metadata": {
+ "id": "i_Z2C2ZcZ3oC"
+ },
+ "outputs": [],
+ "source": [
+ "tf.keras.utils.plot_model(model, show_shapes=True, show_layer_names=True)"
+ ]
+ },
{
"cell_type": "markdown",
"metadata": {
@@ -1258,7 +1278,6 @@
],
"metadata": {
"colab": {
- "collapsed_sections": [],
"name": "pandas_dataframe.ipynb",
"toc_visible": true
},
diff --git a/site/en/tutorials/load_data/tfrecord.ipynb b/site/en/tutorials/load_data/tfrecord.ipynb
index 68708157fcb..905e1a3745b 100644
--- a/site/en/tutorials/load_data/tfrecord.ipynb
+++ b/site/en/tutorials/load_data/tfrecord.ipynb
@@ -356,7 +356,7 @@
"id": "XftzX9CN_uGT"
},
"source": [
- "For example, suppose you have a single observation from the dataset, `[False, 4, bytes('goat'), 0.9876]`. You can create and print the `tf.train.Example` message for this observation using `create_message()`. Each single observation will be written as a `Features` message as per the above. Note that the `tf.train.Example` [message](https://github.com/tensorflow/tensorflow/blob/master/tensorflow/core/example/example.proto#L88) is just a wrapper around the `Features` message:"
+ "For example, suppose you have a single observation from the dataset, `[False, 4, bytes('goat'), 0.9876]`. You can create and print the `tf.train.Example` message for this observation using `serialize_example()`. Each single observation will be written as a `Features` message as per the above. Note that the `tf.train.Example` [message](https://github.com/tensorflow/tensorflow/blob/master/tensorflow/core/example/example.proto#L88) is just a wrapper around the `Features` message:"
]
},
{
@@ -369,10 +369,10 @@
"source": [
"# This is an example observation from the dataset.\n",
"\n",
- "example_observation = []\n",
- "\n",
- "serialized_example = serialize_example(False, 4, b'goat', 0.9876)\n",
- "serialized_example.numpy()"
+ "example_observation = [False, 4, b'goat', 0.9876]\n",
+ "serialized_example = serialize_example(*example_observation)\n",
+ "serialized_example"
+
]
},
{
diff --git a/site/en/tutorials/load_data/video.ipynb b/site/en/tutorials/load_data/video.ipynb
index 46bafb31d1c..42439404948 100644
--- a/site/en/tutorials/load_data/video.ipynb
+++ b/site/en/tutorials/load_data/video.ipynb
@@ -660,7 +660,7 @@
"source": [
"## Visualize video data\n",
"\n",
- "The `frames_from_video_file` function that returns a set of frames as a NumPy array. Try using this function on a new video from [Wikimedia](https://commons.wikimedia.org/wiki/Category:Videos_of_sports){:.external} by Patrick Gillett:"
+ "The `frames_from_video_file` function that returns a set of frames as a NumPy array. Try using this function on a new video from [Wikimedia](https://commons.wikimedia.org/wiki/Category:Videos_of_sports) by Patrick Gillett:"
]
},
{
@@ -965,7 +965,7 @@
"source": [
"## Next steps\n",
"\n",
- "Now that you have created a TensorFlow `Dataset` of video frames with their labels, you can use it with a deep learning model. The following classification model that uses a pre-trained [EfficientNet](https://arxiv.org/abs/1905.11946){:.external} trains to high accuracy in a few minutes:"
+ "Now that you have created a TensorFlow `Dataset` of video frames with their labels, you can use it with a deep learning model. The following classification model that uses a pre-trained [EfficientNet](https://arxiv.org/abs/1905.11946) trains to high accuracy in a few minutes:"
]
},
{
diff --git a/site/en/tutorials/quickstart/advanced.ipynb b/site/en/tutorials/quickstart/advanced.ipynb
index 2fe0ce85773..7cc134b2613 100644
--- a/site/en/tutorials/quickstart/advanced.ipynb
+++ b/site/en/tutorials/quickstart/advanced.ipynb
@@ -200,7 +200,7 @@
"id": "uGih-c2LgbJu"
},
"source": [
- "Choose an optimizer and loss function for training: "
+ "Choose an optimizer and loss function for training:"
]
},
{
@@ -311,10 +311,10 @@
"\n",
"for epoch in range(EPOCHS):\n",
" # Reset the metrics at the start of the next epoch\n",
- " train_loss.reset_states()\n",
- " train_accuracy.reset_states()\n",
- " test_loss.reset_states()\n",
- " test_accuracy.reset_states()\n",
+ " train_loss.reset_state()\n",
+ " train_accuracy.reset_state()\n",
+ " test_loss.reset_state()\n",
+ " test_accuracy.reset_state()\n",
"\n",
" for images, labels in train_ds:\n",
" train_step(images, labels)\n",
@@ -324,10 +324,10 @@
"\n",
" print(\n",
" f'Epoch {epoch + 1}, '\n",
- " f'Loss: {train_loss.result()}, '\n",
- " f'Accuracy: {train_accuracy.result() * 100}, '\n",
- " f'Test Loss: {test_loss.result()}, '\n",
- " f'Test Accuracy: {test_accuracy.result() * 100}'\n",
+ " f'Loss: {train_loss.result():0.2f}, '\n",
+ " f'Accuracy: {train_accuracy.result() * 100:0.2f}, '\n",
+ " f'Test Loss: {test_loss.result():0.2f}, '\n",
+ " f'Test Accuracy: {test_accuracy.result() * 100:0.2f}'\n",
" )"
]
},
@@ -344,8 +344,8 @@
"metadata": {
"accelerator": "GPU",
"colab": {
- "collapsed_sections": [],
"name": "advanced.ipynb",
+ "provenance": [],
"toc_visible": true
},
"kernelspec": {
diff --git a/site/en/tutorials/structured_data/imbalanced_data.ipynb b/site/en/tutorials/structured_data/imbalanced_data.ipynb
index 0d9578b30dc..25b55071817 100644
--- a/site/en/tutorials/structured_data/imbalanced_data.ipynb
+++ b/site/en/tutorials/structured_data/imbalanced_data.ipynb
@@ -258,10 +258,10 @@
"train_df, val_df = train_test_split(train_df, test_size=0.2)\n",
"\n",
"# Form np arrays of labels and features.\n",
- "train_labels = np.array(train_df.pop('Class'))\n",
- "bool_train_labels = train_labels != 0\n",
- "val_labels = np.array(val_df.pop('Class'))\n",
- "test_labels = np.array(test_df.pop('Class'))\n",
+ "train_labels = np.array(train_df.pop('Class')).reshape(-1, 1)\n",
+ "bool_train_labels = train_labels[:, 0] != 0\n",
+ "val_labels = np.array(val_df.pop('Class')).reshape(-1, 1)\n",
+ "test_labels = np.array(test_df.pop('Class')).reshape(-1, 1)\n",
"\n",
"train_features = np.array(train_df)\n",
"val_features = np.array(val_df)\n",
@@ -291,10 +291,9 @@
]
},
{
- "attachments": {},
"cell_type": "markdown",
"metadata": {
- "id": "8a_Z_kBmr7Oh"
+ "id": "ueKV4cmcoRnf"
},
"source": [
"Given the small number of positive labels, this seems about right.\n",
@@ -302,7 +301,7 @@
"Normalize the input features using the sklearn StandardScaler.\n",
"This will set the mean to 0 and standard deviation to 1.\n",
"\n",
- "Note: The `StandardScaler` is only fit using the `train_features` to be sure the model is not peeking at the validation or test sets. "
+ "Note: The `StandardScaler` is only fit using the `train_features` to be sure the model is not peeking at the validation or test sets."
]
},
{
@@ -352,7 +351,7 @@
"\n",
"Next compare the distributions of the positive and negative examples over a few features. Good questions to ask yourself at this point are:\n",
"\n",
- "* Do these distributions make sense? \n",
+ "* Do these distributions make sense?\n",
" * Yes. You've normalized the input and these are mostly concentrated in the `+/- 2` range.\n",
"* Can you see the difference between the distributions?\n",
" * Yes the positive examples contain a much higher rate of extreme values."
@@ -386,7 +385,7 @@
"source": [
"## Define the model and metrics\n",
"\n",
- "Define a function that creates a simple neural network with a densly connected hidden layer, a [dropout](https://developers.google.com/machine-learning/glossary/#dropout_regularization) layer to reduce overfitting, and an output sigmoid layer that returns the probability of a transaction being fraudulent: "
+ "Define a function that creates a simple neural network with a densly connected hidden layer, a [dropout](https://developers.google.com/machine-learning/glossary/#dropout_regularization) layer to reduce overfitting, and an output sigmoid layer that returns the probability of a transaction being fraudulent:"
]
},
{
@@ -403,7 +402,7 @@
" keras.metrics.TruePositives(name='tp'),\n",
" keras.metrics.FalsePositives(name='fp'),\n",
" keras.metrics.TrueNegatives(name='tn'),\n",
- " keras.metrics.FalseNegatives(name='fn'), \n",
+ " keras.metrics.FalseNegatives(name='fn'),\n",
" keras.metrics.BinaryAccuracy(name='accuracy'),\n",
" keras.metrics.Precision(name='precision'),\n",
" keras.metrics.Recall(name='recall'),\n",
@@ -432,7 +431,6 @@
]
},
{
- "attachments": {},
"cell_type": "markdown",
"metadata": {
"id": "SU0GX6E6mieP"
@@ -445,7 +443,7 @@
"\n",
"#### Metrics for probability predictions\n",
"\n",
- "As we train our network with the cross entropy as a loss function, it is fully capable of predicting class probabilities, i.e. it is a probabilistic classifier.\n",
+ "As we train our network with the cross entropy as a loss function, it is fully capable of predicting class probabilities, i.e., it is a probabilistic classifier.\n",
"Good metrics to assess probabilistic predictions are, in fact, **proper scoring rules**. Their key property is that predicting the true probability is optimal. We give two well-known examples:\n",
"\n",
"* **cross entropy** also known as log loss\n",
@@ -456,7 +454,7 @@
"In the end, one often wants to predict a class label, 0 or 1, *no fraud* or *fraud*.\n",
"This is called a deterministic classifier.\n",
"To get a label prediction from our probabilistic classifier, one needs to choose a probability threshold $t$.\n",
- "The default is to predict label 1 (fraud) if the predicted probability is larger than $t=50\\%$ and all the following metrics implicitly use this default. \n",
+ "The default is to predict label 1 (fraud) if the predicted probability is larger than $t=50\\%$ and all the following metrics implicitly use this default.\n",
"\n",
"* **False** negatives and **false** positives are samples that were **incorrectly** classified\n",
"* **True** negatives and **true** positives are samples that were **correctly** classified\n",
@@ -474,7 +472,7 @@
"The following metrics take into account all possible choices of thresholds $t$.\n",
"\n",
"* **AUC** refers to the Area Under the Curve of a Receiver Operating Characteristic curve (ROC-AUC). This metric is equal to the probability that a classifier will rank a random positive sample higher than a random negative sample.\n",
- "* **AUPRC** refers to Area Under the Curve of the Precision-Recall Curve. This metric computes precision-recall pairs for different probability thresholds. \n",
+ "* **AUPRC** refers to Area Under the Curve of the Precision-Recall Curve. This metric computes precision-recall pairs for different probability thresholds.\n",
"\n",
"\n",
"#### Read more:\n",
@@ -520,8 +518,9 @@
"EPOCHS = 100\n",
"BATCH_SIZE = 2048\n",
"\n",
- "early_stopping = tf.keras.callbacks.EarlyStopping(\n",
- " monitor='val_prc', \n",
+ "def early_stopping():\n",
+ " return tf.keras.callbacks.EarlyStopping(\n",
+ " monitor='val_prc',\n",
" verbose=1,\n",
" patience=10,\n",
" mode='max',\n",
@@ -584,7 +583,7 @@
"id": "PdbfWDuVpo6k"
},
"source": [
- "With the default bias initialization the loss should be about `math.log(2) = 0.69314` "
+ "With the default bias initialization the loss should be about `math.log(2) = 0.69314`"
]
},
{
@@ -630,7 +629,7 @@
"id": "d1juXI9yY1KD"
},
"source": [
- "Set that as the initial bias, and the model will give much more reasonable initial guesses. \n",
+ "Set that as the initial bias, and the model will give much more reasonable initial guesses.\n",
"\n",
"It should be near: `pos/total = 0.0018`"
]
@@ -700,7 +699,7 @@
},
"outputs": [],
"source": [
- "initial_weights = os.path.join(tempfile.mkdtemp(), 'initial_weights')\n",
+ "initial_weights = os.path.join(tempfile.mkdtemp(), 'initial.weights.h5')\n",
"model.save_weights(initial_weights)"
]
},
@@ -714,7 +713,7 @@
"\n",
"Before moving on, confirm quick that the careful bias initialization actually helped.\n",
"\n",
- "Train the model for 20 epochs, with and without this careful initialization, and compare the losses: "
+ "Train the model for 20 epochs, with and without this careful initialization, and compare the losses:"
]
},
{
@@ -733,7 +732,7 @@
" train_labels,\n",
" batch_size=BATCH_SIZE,\n",
" epochs=20,\n",
- " validation_data=(val_features, val_labels), \n",
+ " validation_data=(val_features, val_labels),\n",
" verbose=0)"
]
},
@@ -752,7 +751,7 @@
" train_labels,\n",
" batch_size=BATCH_SIZE,\n",
" epochs=20,\n",
- " validation_data=(val_features, val_labels), \n",
+ " validation_data=(val_features, val_labels),\n",
" verbose=0)"
]
},
@@ -794,7 +793,7 @@
"id": "fKMioV0ddG3R"
},
"source": [
- "The above figure makes it clear: In terms of validation loss, on this problem, this careful initialization gives a clear advantage. "
+ "The above figure makes it clear: In terms of validation loss, on this problem, this careful initialization gives a clear advantage."
]
},
{
@@ -821,7 +820,7 @@
" train_labels,\n",
" batch_size=BATCH_SIZE,\n",
" epochs=EPOCHS,\n",
- " callbacks=[early_stopping],\n",
+ " callbacks=[early_stopping()],\n",
" validation_data=(val_features, val_labels))"
]
},
@@ -996,10 +995,9 @@
]
},
{
- "attachments": {},
"cell_type": "markdown",
"metadata": {
- "id": "P-QpQsip_F2Q"
+ "id": "kF8k-g9goRni"
},
"source": [
"### Plot the ROC\n",
@@ -1161,10 +1159,10 @@
" train_labels,\n",
" batch_size=BATCH_SIZE,\n",
" epochs=EPOCHS,\n",
- " callbacks=[early_stopping],\n",
+ " callbacks=[early_stopping()],\n",
" validation_data=(val_features, val_labels),\n",
" # The class weights go here\n",
- " class_weight=class_weight) "
+ " class_weight=class_weight)"
]
},
{
@@ -1333,7 +1331,7 @@
"source": [
"#### Using NumPy\n",
"\n",
- "You can balance the dataset manually by choosing the right number of random \n",
+ "You can balance the dataset manually by choosing the right number of random\n",
"indices from the positive examples:"
]
},
@@ -1485,7 +1483,7 @@
},
"outputs": [],
"source": [
- "resampled_steps_per_epoch = np.ceil(2.0*neg/BATCH_SIZE)\n",
+ "resampled_steps_per_epoch = int(np.ceil(2.0*neg/BATCH_SIZE))\n",
"resampled_steps_per_epoch"
]
},
@@ -1499,7 +1497,7 @@
"\n",
"Now try training the model with the resampled data set instead of using class weights to see how these methods compare.\n",
"\n",
- "Note: Because the data was balanced by replicating the positive examples, the total dataset size is larger, and each epoch runs for more training steps. "
+ "Note: Because the data was balanced by replicating the positive examples, the total dataset size is larger, and each epoch runs for more training steps."
]
},
{
@@ -1514,17 +1512,17 @@
"resampled_model.load_weights(initial_weights)\n",
"\n",
"# Reset the bias to zero, since this dataset is balanced.\n",
- "output_layer = resampled_model.layers[-1] \n",
+ "output_layer = resampled_model.layers[-1]\n",
"output_layer.bias.assign([0])\n",
"\n",
"val_ds = tf.data.Dataset.from_tensor_slices((val_features, val_labels)).cache()\n",
- "val_ds = val_ds.batch(BATCH_SIZE).prefetch(2) \n",
+ "val_ds = val_ds.batch(BATCH_SIZE).prefetch(2)\n",
"\n",
"resampled_history = resampled_model.fit(\n",
" resampled_ds,\n",
" epochs=EPOCHS,\n",
" steps_per_epoch=resampled_steps_per_epoch,\n",
- " callbacks=[early_stopping],\n",
+ " callbacks=[early_stopping()],\n",
" validation_data=val_ds)"
]
},
@@ -1536,7 +1534,7 @@
"source": [
"If the training process were considering the whole dataset on each gradient update, this oversampling would be basically identical to the class weighting.\n",
"\n",
- "But when training the model batch-wise, as you did here, the oversampled data provides a smoother gradient signal: Instead of each positive example being shown in one batch with a large weight, they're shown in many different batches each time with a small weight. \n",
+ "But when training the model batch-wise, as you did here, the oversampled data provides a smoother gradient signal: Instead of each positive example being shown in one batch with a large weight, they're shown in many different batches each time with a small weight.\n",
"\n",
"This smoother gradient signal makes it easier to train the model."
]
@@ -1549,7 +1547,7 @@
"source": [
"### Check training history\n",
"\n",
- "Note that the distributions of metrics will be different here, because the training data has a totally different distribution from the validation and test data. "
+ "Note that the distributions of metrics will be different here, because the training data has a totally different distribution from the validation and test data."
]
},
{
@@ -1578,7 +1576,7 @@
"id": "KFLxRL8eoDE5"
},
"source": [
- "Because training is easier on the balanced data, the above training procedure may overfit quickly. \n",
+ "Because training is easier on the balanced data, the above training procedure may overfit quickly.\n",
"\n",
"So break up the epochs to give the `tf.keras.callbacks.EarlyStopping` finer control over when to stop training."
]
@@ -1595,7 +1593,7 @@
"resampled_model.load_weights(initial_weights)\n",
"\n",
"# Reset the bias to zero, since this dataset is balanced.\n",
- "output_layer = resampled_model.layers[-1] \n",
+ "output_layer = resampled_model.layers[-1]\n",
"output_layer.bias.assign([0])\n",
"\n",
"resampled_history = resampled_model.fit(\n",
@@ -1603,7 +1601,7 @@
" # These are not real epochs\n",
" steps_per_epoch=20,\n",
" epochs=10*EPOCHS,\n",
- " callbacks=[early_stopping],\n",
+ " callbacks=[early_stopping()],\n",
" validation_data=(val_ds))"
]
},
@@ -1696,7 +1694,7 @@
"id": "vayGnv0VOe_v"
},
"source": [
- "### Plot the AUPRC\r\n"
+ "### Plot the AUPRC\n"
]
},
{
@@ -1707,14 +1705,14 @@
},
"outputs": [],
"source": [
- "plot_prc(\"Train Baseline\", train_labels, train_predictions_baseline, color=colors[0])\r\n",
- "plot_prc(\"Test Baseline\", test_labels, test_predictions_baseline, color=colors[0], linestyle='--')\r\n",
- "\r\n",
- "plot_prc(\"Train Weighted\", train_labels, train_predictions_weighted, color=colors[1])\r\n",
- "plot_prc(\"Test Weighted\", test_labels, test_predictions_weighted, color=colors[1], linestyle='--')\r\n",
- "\r\n",
- "plot_prc(\"Train Resampled\", train_labels, train_predictions_resampled, color=colors[2])\r\n",
- "plot_prc(\"Test Resampled\", test_labels, test_predictions_resampled, color=colors[2], linestyle='--')\r\n",
+ "plot_prc(\"Train Baseline\", train_labels, train_predictions_baseline, color=colors[0])\n",
+ "plot_prc(\"Test Baseline\", test_labels, test_predictions_baseline, color=colors[0], linestyle='--')\n",
+ "\n",
+ "plot_prc(\"Train Weighted\", train_labels, train_predictions_weighted, color=colors[1])\n",
+ "plot_prc(\"Test Weighted\", test_labels, test_predictions_weighted, color=colors[1], linestyle='--')\n",
+ "\n",
+ "plot_prc(\"Train Resampled\", train_labels, train_predictions_resampled, color=colors[2])\n",
+ "plot_prc(\"Test Resampled\", test_labels, test_predictions_resampled, color=colors[2], linestyle='--')\n",
"plt.legend(loc='lower right');"
]
},
@@ -1732,7 +1730,6 @@
],
"metadata": {
"colab": {
- "collapsed_sections": [],
"name": "imbalanced_data.ipynb",
"toc_visible": true
},
diff --git a/site/en/tutorials/structured_data/preprocessing_layers.ipynb b/site/en/tutorials/structured_data/preprocessing_layers.ipynb
index 928a56eb8bc..d05df3c6d21 100644
--- a/site/en/tutorials/structured_data/preprocessing_layers.ipynb
+++ b/site/en/tutorials/structured_data/preprocessing_layers.ipynb
@@ -297,7 +297,7 @@
"def df_to_dataset(dataframe, shuffle=True, batch_size=32):\n",
" df = dataframe.copy()\n",
" labels = df.pop('target')\n",
- " df = {key: value[:,tf.newaxis] for key, value in dataframe.items()}\n",
+ " df = {key: value.to_numpy()[:,tf.newaxis] for key, value in dataframe.items()}\n",
" ds = tf.data.Dataset.from_tensor_slices((dict(df), labels))\n",
" if shuffle:\n",
" ds = ds.shuffle(buffer_size=len(dataframe))\n",
@@ -447,7 +447,7 @@
"source": [
"### Categorical columns\n",
"\n",
- "Pet `Type`s in the dataset are represented as strings—`Dog`s and `Cat`s—which need to be multi-hot encoded before being fed into the model. The `Age` feature \n",
+ "Pet `Type`s in the dataset are represented as strings—`Dog`s and `Cat`s—which need to be multi-hot encoded before being fed into the model. The `Age` feature\n",
"\n",
"Define another new utility function that returns a layer which maps values from a vocabulary to integer indices and multi-hot encodes the features using the `tf.keras.layers.StringLookup`, `tf.keras.layers.IntegerLookup`, and `tf.keras.CategoryEncoding` preprocessing layers:"
]
@@ -589,7 +589,7 @@
},
"outputs": [],
"source": [
- "all_inputs = []\n",
+ "all_inputs = {}\n",
"encoded_features = []\n",
"\n",
"# Numerical features.\n",
@@ -597,7 +597,7 @@
" numeric_col = tf.keras.Input(shape=(1,), name=header)\n",
" normalization_layer = get_normalization_layer(header, train_ds)\n",
" encoded_numeric_col = normalization_layer(numeric_col)\n",
- " all_inputs.append(numeric_col)\n",
+ " all_inputs[header] = numeric_col\n",
" encoded_features.append(encoded_numeric_col)"
]
},
@@ -625,7 +625,7 @@
" dtype='int64',\n",
" max_tokens=5)\n",
"encoded_age_col = encoding_layer(age_col)\n",
- "all_inputs.append(age_col)\n",
+ "all_inputs['Age'] = age_col\n",
"encoded_features.append(encoded_age_col)"
]
},
@@ -656,7 +656,7 @@
" dtype='string',\n",
" max_tokens=5)\n",
" encoded_categorical_col = encoding_layer(categorical_col)\n",
- " all_inputs.append(categorical_col)\n",
+ " all_inputs[header] = categorical_col\n",
" encoded_features.append(encoded_categorical_col)"
]
},
@@ -678,6 +678,17 @@
"The next step is to create a model using the [Keras Functional API](https://www.tensorflow.org/guide/keras/functional). For the first layer in your model, merge the list of feature inputs—`encoded_features`—into one vector via concatenation with `tf.keras.layers.concatenate`."
]
},
+ {
+ "cell_type": "code",
+ "execution_count": null,
+ "metadata": {
+ "id": "EtkwHC-akvcv"
+ },
+ "outputs": [],
+ "source": [
+ "encoded_features"
+ ]
+ },
{
"cell_type": "code",
"execution_count": null,
@@ -713,7 +724,8 @@
"source": [
"model.compile(optimizer='adam',\n",
" loss=tf.keras.losses.BinaryCrossentropy(from_logits=True),\n",
- " metrics=[\"accuracy\"])"
+ " metrics=[\"accuracy\"],\n",
+ " run_eagerly=True)"
]
},
{
@@ -734,7 +746,7 @@
"outputs": [],
"source": [
"# Use `rankdir='LR'` to make the graph horizontal.\n",
- "tf.keras.utils.plot_model(model, show_shapes=True, rankdir=\"LR\")"
+ "tf.keras.utils.plot_model(model, show_shapes=True, show_layer_names=True, rankdir=\"LR\")"
]
},
{
@@ -765,8 +777,8 @@
},
"outputs": [],
"source": [
- "loss, accuracy = model.evaluate(test_ds)\n",
- "print(\"Accuracy\", accuracy)"
+ "result = model.evaluate(test_ds, return_dict=True)\n",
+ "print(result)"
]
},
{
@@ -869,7 +881,6 @@
],
"metadata": {
"colab": {
- "collapsed_sections": [],
"name": "preprocessing_layers.ipynb",
"toc_visible": true
},
diff --git a/site/en/tutorials/structured_data/time_series.ipynb b/site/en/tutorials/structured_data/time_series.ipynb
index 0b0eb55bce3..31aab384859 100644
--- a/site/en/tutorials/structured_data/time_series.ipynb
+++ b/site/en/tutorials/structured_data/time_series.ipynb
@@ -70,7 +70,7 @@
"source": [
"This tutorial is an introduction to time series forecasting using TensorFlow. It builds a few different styles of models including Convolutional and Recurrent Neural Networks (CNNs and RNNs).\n",
"\n",
- "This is covered in two main parts, with subsections: \n",
+ "This is covered in two main parts, with subsections:\n",
"\n",
"* Forecast for a single time step:\n",
" * A single feature.\n",
@@ -452,7 +452,7 @@
"id": "HiurzTGQgf_D"
},
"source": [
- "This gives the model access to the most important frequency features. In this case you knew ahead of time which frequencies were important. \n",
+ "This gives the model access to the most important frequency features. In this case you knew ahead of time which frequencies were important.\n",
"\n",
"If you don't have that information, you can determine which frequencies are important by extracting features with Fast Fourier Transform. To check the assumptions, here is the `tf.signal.rfft` of the temperature over time. Note the obvious peaks at frequencies near `1/year` and `1/day`:\n"
]
@@ -590,13 +590,13 @@
"source": [
"## Data windowing\n",
"\n",
- "The models in this tutorial will make a set of predictions based on a window of consecutive samples from the data. \n",
+ "The models in this tutorial will make a set of predictions based on a window of consecutive samples from the data.\n",
"\n",
"The main features of the input windows are:\n",
"\n",
"- The width (number of time steps) of the input and label windows.\n",
"- The time offset between them.\n",
- "- Which features are used as inputs, labels, or both. \n",
+ "- Which features are used as inputs, labels, or both.\n",
"\n",
"This tutorial builds a variety of models (including Linear, DNN, CNN and RNN models), and uses them for both:\n",
"\n",
@@ -616,11 +616,11 @@
"\n",
"1. For example, to make a single prediction 24 hours into the future, given 24 hours of history, you might define a window like this:\n",
"\n",
- " \n",
+ " 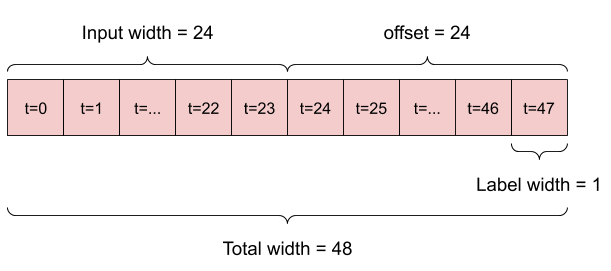\n",
"\n",
"2. A model that makes a prediction one hour into the future, given six hours of history, would need a window like this:\n",
"\n",
- " "
+ " 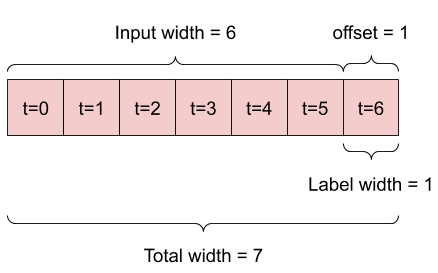"
]
},
{
@@ -744,7 +744,7 @@
"\n",
"The example `w2` you define earlier will be split like this:\n",
"\n",
- "\n",
+ "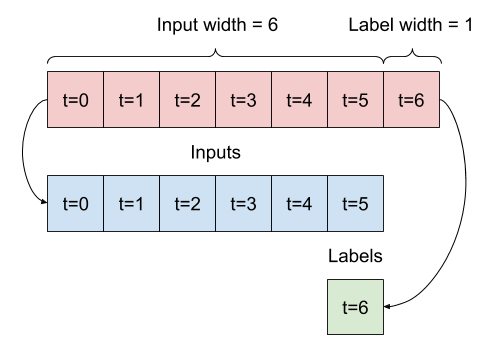\n",
"\n",
"This diagram doesn't show the `features` axis of the data, but this `split_window` function also handles the `label_columns` so it can be used for both the single output and multi-output examples."
]
@@ -1069,7 +1069,7 @@
"\n",
"So, start by building models to predict the `T (degC)` value one hour into the future.\n",
"\n",
- "\n",
+ "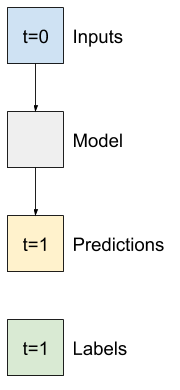\n",
"\n",
"Configure a `WindowGenerator` object to produce these single-step `(input, label)` pairs:"
]
@@ -1120,11 +1120,11 @@
"\n",
"Before building a trainable model it would be good to have a performance baseline as a point for comparison with the later more complicated models.\n",
"\n",
- "This first task is to predict temperature one hour into the future, given the current value of all features. The current values include the current temperature. \n",
+ "This first task is to predict temperature one hour into the future, given the current value of all features. The current values include the current temperature.\n",
"\n",
"So, start with a model that just returns the current temperature as the prediction, predicting \"No change\". This is a reasonable baseline since temperature changes slowly. Of course, this baseline will work less well if you make a prediction further in the future.\n",
"\n",
- ""
+ "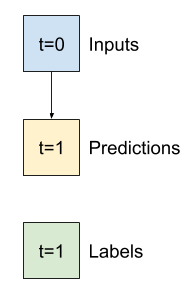"
]
},
{
@@ -1171,8 +1171,8 @@
"\n",
"val_performance = {}\n",
"performance = {}\n",
- "val_performance['Baseline'] = baseline.evaluate(single_step_window.val)\n",
- "performance['Baseline'] = baseline.evaluate(single_step_window.test, verbose=0)"
+ "val_performance['Baseline'] = baseline.evaluate(single_step_window.val, return_dict=True)\n",
+ "performance['Baseline'] = baseline.evaluate(single_step_window.test, verbose=0, return_dict=True)"
]
},
{
@@ -1211,7 +1211,7 @@
"source": [
"This expanded window can be passed directly to the same `baseline` model without any code changes. This is possible because the inputs and labels have the same number of time steps, and the baseline just forwards the input to the output:\n",
"\n",
- ""
+ "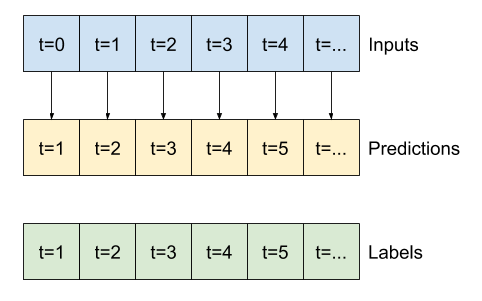"
]
},
{
@@ -1269,7 +1269,7 @@
"\n",
"The simplest **trainable** model you can apply to this task is to insert linear transformation between the input and output. In this case the output from a time step only depends on that step:\n",
"\n",
- "\n",
+ "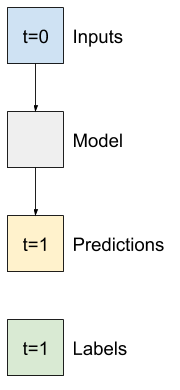\n",
"\n",
"A `tf.keras.layers.Dense` layer with no `activation` set is a linear model. The layer only transforms the last axis of the data from `(batch, time, inputs)` to `(batch, time, units)`; it is applied independently to every item across the `batch` and `time` axes."
]
@@ -1352,8 +1352,8 @@
"source": [
"history = compile_and_fit(linear, single_step_window)\n",
"\n",
- "val_performance['Linear'] = linear.evaluate(single_step_window.val)\n",
- "performance['Linear'] = linear.evaluate(single_step_window.test, verbose=0)"
+ "val_performance['Linear'] = linear.evaluate(single_step_window.val, return_dict=True)\n",
+ "performance['Linear'] = linear.evaluate(single_step_window.test, verbose=0, return_dict=True)"
]
},
{
@@ -1364,7 +1364,7 @@
"source": [
"Like the `baseline` model, the linear model can be called on batches of wide windows. Used this way the model makes a set of independent predictions on consecutive time steps. The `time` axis acts like another `batch` axis. There are no interactions between the predictions at each time step.\n",
"\n",
- ""
+ "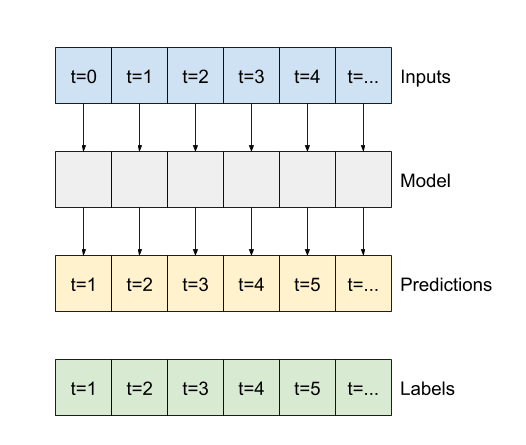"
]
},
{
@@ -1430,7 +1430,7 @@
"id": "Ylng7215boIY"
},
"source": [
- "Sometimes the model doesn't even place the most weight on the input `T (degC)`. This is one of the risks of random initialization. "
+ "Sometimes the model doesn't even place the most weight on the input `T (degC)`. This is one of the risks of random initialization."
]
},
{
@@ -1443,7 +1443,7 @@
"\n",
"Before applying models that actually operate on multiple time-steps, it's worth checking the performance of deeper, more powerful, single input step models.\n",
"\n",
- "Here's a model similar to the `linear` model, except it stacks several a few `Dense` layers between the input and the output: "
+ "Here's a model similar to the `linear` model, except it stacks several a few `Dense` layers between the input and the output:"
]
},
{
@@ -1462,8 +1462,8 @@
"\n",
"history = compile_and_fit(dense, single_step_window)\n",
"\n",
- "val_performance['Dense'] = dense.evaluate(single_step_window.val)\n",
- "performance['Dense'] = dense.evaluate(single_step_window.test, verbose=0)"
+ "val_performance['Dense'] = dense.evaluate(single_step_window.val, return_dict=True)\n",
+ "performance['Dense'] = dense.evaluate(single_step_window.test, verbose=0, return_dict=True)"
]
},
{
@@ -1476,7 +1476,7 @@
"\n",
"A single-time-step model has no context for the current values of its inputs. It can't see how the input features are changing over time. To address this issue the model needs access to multiple time steps when making predictions:\n",
"\n",
- "\n"
+ "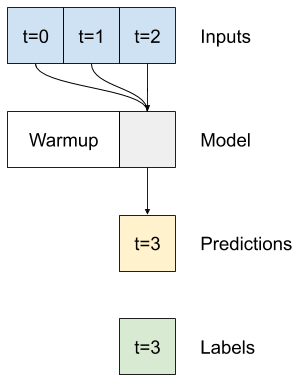\n"
]
},
{
@@ -1526,7 +1526,7 @@
"outputs": [],
"source": [
"conv_window.plot()\n",
- "plt.title(\"Given 3 hours of inputs, predict 1 hour into the future.\")"
+ "plt.suptitle(\"Given 3 hours of inputs, predict 1 hour into the future.\")"
]
},
{
@@ -1581,8 +1581,8 @@
"history = compile_and_fit(multi_step_dense, conv_window)\n",
"\n",
"IPython.display.clear_output()\n",
- "val_performance['Multi step dense'] = multi_step_dense.evaluate(conv_window.val)\n",
- "performance['Multi step dense'] = multi_step_dense.evaluate(conv_window.test, verbose=0)"
+ "val_performance['Multi step dense'] = multi_step_dense.evaluate(conv_window.val, return_dict=True)\n",
+ "performance['Multi step dense'] = multi_step_dense.evaluate(conv_window.test, verbose=0, return_dict=True)"
]
},
{
@@ -1602,7 +1602,7 @@
"id": "gWfrsP8mq8lV"
},
"source": [
- "The main down-side of this approach is that the resulting model can only be executed on input windows of exactly this shape. "
+ "The main down-side of this approach is that the resulting model can only be executed on input windows of exactly this shape."
]
},
{
@@ -1636,7 +1636,7 @@
},
"source": [
"### Convolution neural network\n",
- " \n",
+ "\n",
"A convolution layer (`tf.keras.layers.Conv1D`) also takes multiple time steps as input to each prediction."
]
},
@@ -1646,7 +1646,7 @@
"id": "cdLBwoaHmsWb"
},
"source": [
- "Below is the **same** model as `multi_step_dense`, re-written with a convolution. \n",
+ "Below is the **same** model as `multi_step_dense`, re-written with a convolution.\n",
"\n",
"Note the changes:\n",
"* The `tf.keras.layers.Flatten` and the first `tf.keras.layers.Dense` are replaced by a `tf.keras.layers.Conv1D`.\n",
@@ -1712,8 +1712,8 @@
"history = compile_and_fit(conv_model, conv_window)\n",
"\n",
"IPython.display.clear_output()\n",
- "val_performance['Conv'] = conv_model.evaluate(conv_window.val)\n",
- "performance['Conv'] = conv_model.evaluate(conv_window.test, verbose=0)"
+ "val_performance['Conv'] = conv_model.evaluate(conv_window.val, return_dict=True)\n",
+ "performance['Conv'] = conv_model.evaluate(conv_window.test, verbose=0, return_dict=True)"
]
},
{
@@ -1724,7 +1724,7 @@
"source": [
"The difference between this `conv_model` and the `multi_step_dense` model is that the `conv_model` can be run on inputs of any length. The convolutional layer is applied to a sliding window of inputs:\n",
"\n",
- "\n",
+ "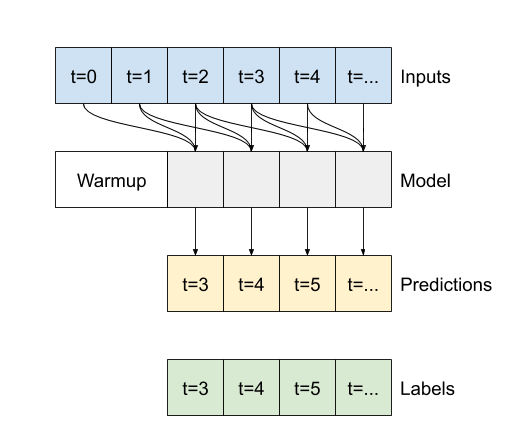\n",
"\n",
"If you run it on wider input, it produces wider output:"
]
@@ -1749,7 +1749,7 @@
"id": "h_WGxtLIHhRF"
},
"source": [
- "Note that the output is shorter than the input. To make training or plotting work, you need the labels, and prediction to have the same length. So build a `WindowGenerator` to produce wide windows with a few extra input time steps so the label and prediction lengths match: "
+ "Note that the output is shorter than the input. To make training or plotting work, you need the labels, and prediction to have the same length. So build a `WindowGenerator` to produce wide windows with a few extra input time steps so the label and prediction lengths match:"
]
},
{
@@ -1828,15 +1828,15 @@
"source": [
"An important constructor argument for all Keras RNN layers, such as `tf.keras.layers.LSTM`, is the `return_sequences` argument. This setting can configure the layer in one of two ways:\n",
"\n",
- "1. If `False`, the default, the layer only returns the output of the final time step, giving the model time to warm up its internal state before making a single prediction: \n",
+ "1. If `False`, the default, the layer only returns the output of the final time step, giving the model time to warm up its internal state before making a single prediction:\n",
"\n",
- "\n",
+ "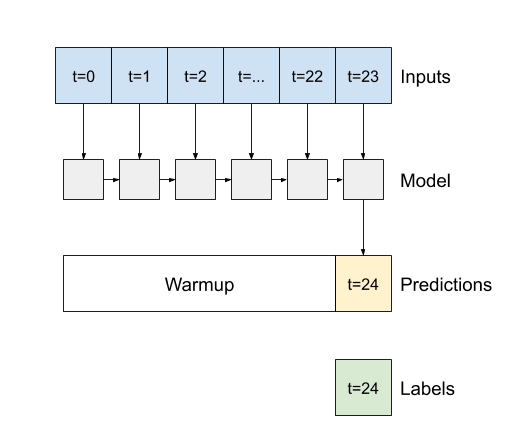\n",
"\n",
"2. If `True`, the layer returns an output for each input. This is useful for:\n",
- " * Stacking RNN layers. \n",
+ " * Stacking RNN layers.\n",
" * Training a model on multiple time steps simultaneously.\n",
"\n",
- ""
+ "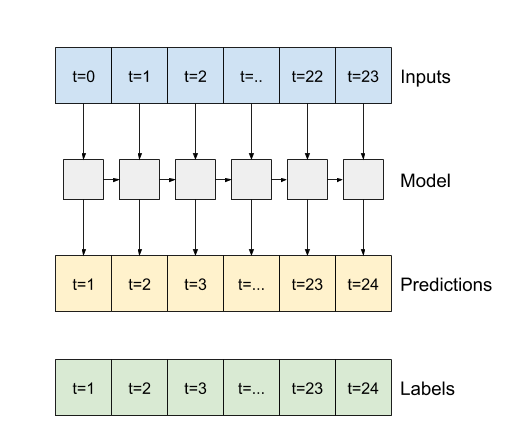"
]
},
{
@@ -1889,8 +1889,8 @@
"history = compile_and_fit(lstm_model, wide_window)\n",
"\n",
"IPython.display.clear_output()\n",
- "val_performance['LSTM'] = lstm_model.evaluate(wide_window.val)\n",
- "performance['LSTM'] = lstm_model.evaluate(wide_window.test, verbose=0)"
+ "val_performance['LSTM'] = lstm_model.evaluate(wide_window.val, return_dict=True)\n",
+ "performance['LSTM'] = lstm_model.evaluate(wide_window.test, verbose=0, return_dict=True)"
]
},
{
@@ -1922,6 +1922,29 @@
"With this dataset typically each of the models does slightly better than the one before it:"
]
},
+ {
+ "cell_type": "code",
+ "execution_count": null,
+ "metadata": {
+ "id": "dMPev9Nzd4mD"
+ },
+ "outputs": [],
+ "source": [
+ "cm = lstm_model.metrics[1]\n",
+ "cm.metrics"
+ ]
+ },
+ {
+ "cell_type": "code",
+ "execution_count": null,
+ "metadata": {
+ "id": "6is3g113eIIa"
+ },
+ "outputs": [],
+ "source": [
+ "val_performance"
+ ]
+ },
{
"cell_type": "code",
"execution_count": null,
@@ -1933,9 +1956,8 @@
"x = np.arange(len(performance))\n",
"width = 0.3\n",
"metric_name = 'mean_absolute_error'\n",
- "metric_index = lstm_model.metrics_names.index('mean_absolute_error')\n",
- "val_mae = [v[metric_index] for v in val_performance.values()]\n",
- "test_mae = [v[metric_index] for v in performance.values()]\n",
+ "val_mae = [v[metric_name] for v in val_performance.values()]\n",
+ "test_mae = [v[metric_name] for v in performance.values()]\n",
"\n",
"plt.ylabel('mean_absolute_error [T (degC), normalized]')\n",
"plt.bar(x - 0.17, val_mae, width, label='Validation')\n",
@@ -1954,7 +1976,7 @@
"outputs": [],
"source": [
"for name, value in performance.items():\n",
- " print(f'{name:12s}: {value[1]:0.4f}')"
+ " print(f'{name:12s}: {value[metric_name]:0.4f}')"
]
},
{
@@ -1979,7 +2001,7 @@
"outputs": [],
"source": [
"single_step_window = WindowGenerator(\n",
- " # `WindowGenerator` returns all features as labels if you \n",
+ " # `WindowGenerator` returns all features as labels if you\n",
" # don't set the `label_columns` argument.\n",
" input_width=1, label_width=1, shift=1)\n",
"\n",
@@ -2034,8 +2056,8 @@
"source": [
"val_performance = {}\n",
"performance = {}\n",
- "val_performance['Baseline'] = baseline.evaluate(wide_window.val)\n",
- "performance['Baseline'] = baseline.evaluate(wide_window.test, verbose=0)"
+ "val_performance['Baseline'] = baseline.evaluate(wide_window.val, return_dict=True)\n",
+ "performance['Baseline'] = baseline.evaluate(wide_window.test, verbose=0, return_dict=True)"
]
},
{
@@ -2073,8 +2095,8 @@
"history = compile_and_fit(dense, single_step_window)\n",
"\n",
"IPython.display.clear_output()\n",
- "val_performance['Dense'] = dense.evaluate(single_step_window.val)\n",
- "performance['Dense'] = dense.evaluate(single_step_window.test, verbose=0)"
+ "val_performance['Dense'] = dense.evaluate(single_step_window.val, return_dict=True)\n",
+ "performance['Dense'] = dense.evaluate(single_step_window.test, verbose=0, return_dict=True)"
]
},
{
@@ -2108,8 +2130,8 @@
"history = compile_and_fit(lstm_model, wide_window)\n",
"\n",
"IPython.display.clear_output()\n",
- "val_performance['LSTM'] = lstm_model.evaluate( wide_window.val)\n",
- "performance['LSTM'] = lstm_model.evaluate( wide_window.test, verbose=0)\n",
+ "val_performance['LSTM'] = lstm_model.evaluate( wide_window.val, return_dict=True)\n",
+ "performance['LSTM'] = lstm_model.evaluate( wide_window.test, verbose=0, return_dict=True)\n",
"\n",
"print()"
]
@@ -2132,7 +2154,7 @@
"\n",
"That is how you take advantage of the knowledge that the change should be small.\n",
"\n",
- "\n",
+ "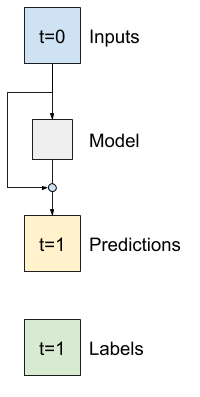\n",
"\n",
"Essentially, this initializes the model to match the `Baseline`. For this task it helps models converge faster, with slightly better performance."
]
@@ -2143,7 +2165,7 @@
"id": "yP58A_ORx0kM"
},
"source": [
- "This approach can be used in conjunction with any model discussed in this tutorial. \n",
+ "This approach can be used in conjunction with any model discussed in this tutorial.\n",
"\n",
"Here, it is being applied to the LSTM model, note the use of the `tf.initializers.zeros` to ensure that the initial predicted changes are small, and don't overpower the residual connection. There are no symmetry-breaking concerns for the gradients here, since the `zeros` are only used on the last layer."
]
@@ -2192,8 +2214,8 @@
"history = compile_and_fit(residual_lstm, wide_window)\n",
"\n",
"IPython.display.clear_output()\n",
- "val_performance['Residual LSTM'] = residual_lstm.evaluate(wide_window.val)\n",
- "performance['Residual LSTM'] = residual_lstm.evaluate(wide_window.test, verbose=0)\n",
+ "val_performance['Residual LSTM'] = residual_lstm.evaluate(wide_window.val, return_dict=True)\n",
+ "performance['Residual LSTM'] = residual_lstm.evaluate(wide_window.test, verbose=0, return_dict=True)\n",
"print()"
]
},
@@ -2227,9 +2249,8 @@
"width = 0.3\n",
"\n",
"metric_name = 'mean_absolute_error'\n",
- "metric_index = lstm_model.metrics_names.index('mean_absolute_error')\n",
- "val_mae = [v[metric_index] for v in val_performance.values()]\n",
- "test_mae = [v[metric_index] for v in performance.values()]\n",
+ "val_mae = [v[metric_name] for v in val_performance.values()]\n",
+ "test_mae = [v[metric_name] for v in performance.values()]\n",
"\n",
"plt.bar(x - 0.17, val_mae, width, label='Validation')\n",
"plt.bar(x + 0.17, test_mae, width, label='Test')\n",
@@ -2248,7 +2269,7 @@
"outputs": [],
"source": [
"for name, value in performance.items():\n",
- " print(f'{name:15s}: {value[1]:0.4f}')"
+ " print(f'{name:15s}: {value[metric_name]:0.4f}')"
]
},
{
@@ -2327,7 +2348,7 @@
"source": [
"A simple baseline for this task is to repeat the last input time step for the required number of output time steps:\n",
"\n",
- ""
+ "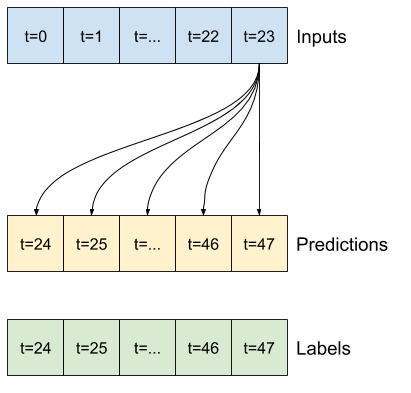"
]
},
{
@@ -2349,8 +2370,8 @@
"multi_val_performance = {}\n",
"multi_performance = {}\n",
"\n",
- "multi_val_performance['Last'] = last_baseline.evaluate(multi_window.val)\n",
- "multi_performance['Last'] = last_baseline.evaluate(multi_window.test, verbose=0)\n",
+ "multi_val_performance['Last'] = last_baseline.evaluate(multi_window.val, return_dict=True)\n",
+ "multi_performance['Last'] = last_baseline.evaluate(multi_window.test, verbose=0, return_dict=True)\n",
"multi_window.plot(last_baseline)"
]
},
@@ -2362,7 +2383,7 @@
"source": [
"Since this task is to predict 24 hours into the future, given 24 hours of the past, another simple approach is to repeat the previous day, assuming tomorrow will be similar:\n",
"\n",
- ""
+ "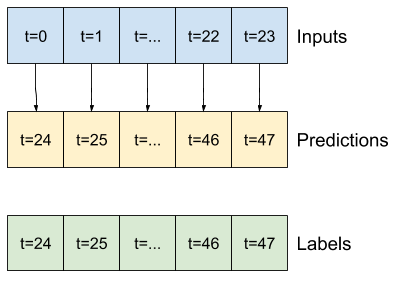"
]
},
{
@@ -2381,8 +2402,8 @@
"repeat_baseline.compile(loss=tf.keras.losses.MeanSquaredError(),\n",
" metrics=[tf.keras.metrics.MeanAbsoluteError()])\n",
"\n",
- "multi_val_performance['Repeat'] = repeat_baseline.evaluate(multi_window.val)\n",
- "multi_performance['Repeat'] = repeat_baseline.evaluate(multi_window.test, verbose=0)\n",
+ "multi_val_performance['Repeat'] = repeat_baseline.evaluate(multi_window.val, return_dict=True)\n",
+ "multi_performance['Repeat'] = repeat_baseline.evaluate(multi_window.test, verbose=0, return_dict=True)\n",
"multi_window.plot(repeat_baseline)"
]
},
@@ -2409,7 +2430,7 @@
"\n",
"A simple linear model based on the last input time step does better than either baseline, but is underpowered. The model needs to predict `OUTPUT_STEPS` time steps, from a single input time step with a linear projection. It can only capture a low-dimensional slice of the behavior, likely based mainly on the time of day and time of year.\n",
"\n",
- ""
+ "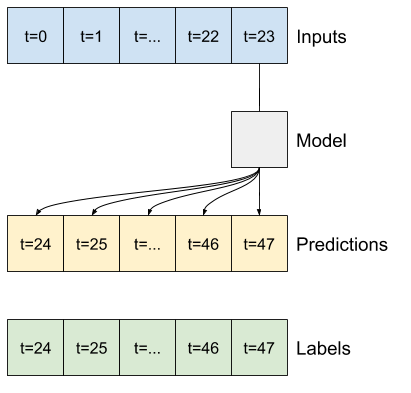"
]
},
{
@@ -2434,8 +2455,8 @@
"history = compile_and_fit(multi_linear_model, multi_window)\n",
"\n",
"IPython.display.clear_output()\n",
- "multi_val_performance['Linear'] = multi_linear_model.evaluate(multi_window.val)\n",
- "multi_performance['Linear'] = multi_linear_model.evaluate(multi_window.test, verbose=0)\n",
+ "multi_val_performance['Linear'] = multi_linear_model.evaluate(multi_window.val, return_dict=True)\n",
+ "multi_performance['Linear'] = multi_linear_model.evaluate(multi_window.test, verbose=0, return_dict=True)\n",
"multi_window.plot(multi_linear_model)"
]
},
@@ -2474,8 +2495,8 @@
"history = compile_and_fit(multi_dense_model, multi_window)\n",
"\n",
"IPython.display.clear_output()\n",
- "multi_val_performance['Dense'] = multi_dense_model.evaluate(multi_window.val)\n",
- "multi_performance['Dense'] = multi_dense_model.evaluate(multi_window.test, verbose=0)\n",
+ "multi_val_performance['Dense'] = multi_dense_model.evaluate(multi_window.val, return_dict=True)\n",
+ "multi_performance['Dense'] = multi_dense_model.evaluate(multi_window.test, verbose=0, return_dict=True)\n",
"multi_window.plot(multi_dense_model)"
]
},
@@ -2496,7 +2517,7 @@
"source": [
"A convolutional model makes predictions based on a fixed-width history, which may lead to better performance than the dense model since it can see how things are changing over time:\n",
"\n",
- ""
+ "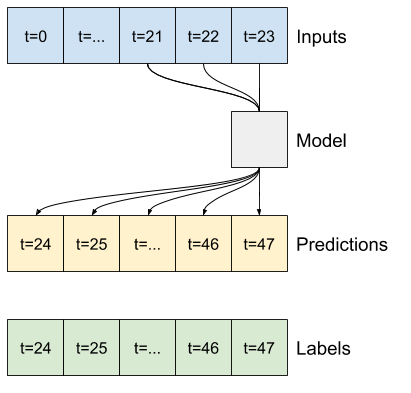"
]
},
{
@@ -2524,8 +2545,8 @@
"\n",
"IPython.display.clear_output()\n",
"\n",
- "multi_val_performance['Conv'] = multi_conv_model.evaluate(multi_window.val)\n",
- "multi_performance['Conv'] = multi_conv_model.evaluate(multi_window.test, verbose=0)\n",
+ "multi_val_performance['Conv'] = multi_conv_model.evaluate(multi_window.val, return_dict=True)\n",
+ "multi_performance['Conv'] = multi_conv_model.evaluate(multi_window.test, verbose=0, return_dict=True)\n",
"multi_window.plot(multi_conv_model)"
]
},
@@ -2548,7 +2569,7 @@
"\n",
"In this single-shot format, the LSTM only needs to produce an output at the last time step, so set `return_sequences=False` in `tf.keras.layers.LSTM`.\n",
"\n",
- "\n"
+ "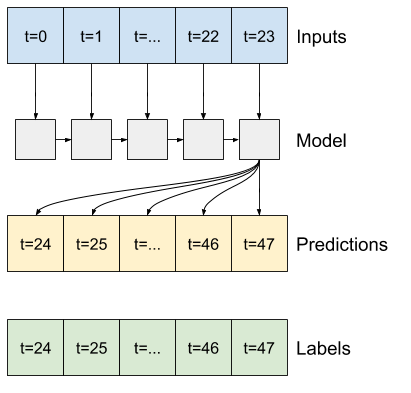\n"
]
},
{
@@ -2574,8 +2595,8 @@
"\n",
"IPython.display.clear_output()\n",
"\n",
- "multi_val_performance['LSTM'] = multi_lstm_model.evaluate(multi_window.val)\n",
- "multi_performance['LSTM'] = multi_lstm_model.evaluate(multi_window.test, verbose=0)\n",
+ "multi_val_performance['LSTM'] = multi_lstm_model.evaluate(multi_window.val, return_dict=True)\n",
+ "multi_performance['LSTM'] = multi_lstm_model.evaluate(multi_window.test, verbose=0, return_dict=True)\n",
"multi_window.plot(multi_lstm_model)"
]
},
@@ -2595,7 +2616,7 @@
"\n",
"You could take any of the single-step multi-output models trained in the first half of this tutorial and run in an autoregressive feedback loop, but here you'll focus on building a model that's been explicitly trained to do that.\n",
"\n",
- ""
+ "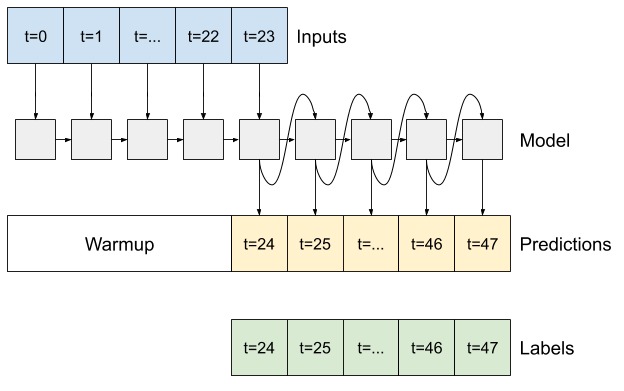"
]
},
{
@@ -2794,8 +2815,8 @@
"\n",
"IPython.display.clear_output()\n",
"\n",
- "multi_val_performance['AR LSTM'] = feedback_model.evaluate(multi_window.val)\n",
- "multi_performance['AR LSTM'] = feedback_model.evaluate(multi_window.test, verbose=0)\n",
+ "multi_val_performance['AR LSTM'] = feedback_model.evaluate(multi_window.val, return_dict=True)\n",
+ "multi_performance['AR LSTM'] = feedback_model.evaluate(multi_window.test, verbose=0, return_dict=True)\n",
"multi_window.plot(feedback_model)"
]
},
@@ -2829,9 +2850,8 @@
"width = 0.3\n",
"\n",
"metric_name = 'mean_absolute_error'\n",
- "metric_index = lstm_model.metrics_names.index('mean_absolute_error')\n",
- "val_mae = [v[metric_index] for v in multi_val_performance.values()]\n",
- "test_mae = [v[metric_index] for v in multi_performance.values()]\n",
+ "val_mae = [v[metric_name] for v in multi_val_performance.values()]\n",
+ "test_mae = [v[metric_name] for v in multi_performance.values()]\n",
"\n",
"plt.bar(x - 0.17, val_mae, width, label='Validation')\n",
"plt.bar(x + 0.17, test_mae, width, label='Test')\n",
@@ -2847,7 +2867,7 @@
"id": "Zq3hUsedCEmJ"
},
"source": [
- "The metrics for the multi-output models in the first half of this tutorial show the performance averaged across all output features. These performances are similar but also averaged across output time steps. "
+ "The metrics for the multi-output models in the first half of this tutorial show the performance averaged across all output features. These performances are similar but also averaged across output time steps."
]
},
{
@@ -2859,7 +2879,7 @@
"outputs": [],
"source": [
"for name, value in multi_performance.items():\n",
- " print(f'{name:8s}: {value[1]:0.4f}')"
+ " print(f'{name:8s}: {value[metric_name]:0.4f}')"
]
},
{
@@ -2894,8 +2914,8 @@
"metadata": {
"accelerator": "GPU",
"colab": {
- "collapsed_sections": [],
"name": "time_series.ipynb",
+ "provenance": [],
"toc_visible": true
},
"kernelspec": {
diff --git a/site/en/tutorials/video/video_classification.ipynb b/site/en/tutorials/video/video_classification.ipynb
index 9356c7cc9d3..4265b6387e3 100644
--- a/site/en/tutorials/video/video_classification.ipynb
+++ b/site/en/tutorials/video/video_classification.ipynb
@@ -84,9 +84,7 @@
"## Setup\n",
"\n",
"Begin by installing and importing some necessary libraries, including:\n",
- "[remotezip](https://github.com/gtsystem/python-remotezip) to inspect the contents of a ZIP file, [tqdm](https://github.com/tqdm/tqdm) to use a progress bar, [OpenCV](https://opencv.org/) to process video files, [einops](https://github.com/arogozhnikov/einops/tree/master/docs) for performing more complex tensor operations, and [`tensorflow_docs`](https://github.com/tensorflow/docs/tree/master/tools/tensorflow_docs) for embedding data in a Jupyter notebook.\n",
- "\n",
- "**Note**: Use TensorFlow 2.10 to run this tutorial. Versions above TensorFlow 2.10 may not run successfully."
+ "[remotezip](https://github.com/gtsystem/python-remotezip) to inspect the contents of a ZIP file, [tqdm](https://github.com/tqdm/tqdm) to use a progress bar, [OpenCV](https://opencv.org/) to process video files, [einops](https://github.com/arogozhnikov/einops/tree/master/docs) for performing more complex tensor operations, and [`tensorflow_docs`](https://github.com/tensorflow/docs/tree/master/tools/tensorflow_docs) for embedding data in a Jupyter notebook."
]
},
{
@@ -98,8 +96,7 @@
"outputs": [],
"source": [
"!pip install remotezip tqdm opencv-python einops \n",
- "# Install TensorFlow 2.10\n",
- "!pip install tensorflow==2.10.0"
+ "!pip install -U tensorflow keras"
]
},
{
diff --git a/tools/tensorflow_docs/api_generator/doc_generator_visitor.py b/tools/tensorflow_docs/api_generator/doc_generator_visitor.py
index 8776644878c..0467f74b153 100644
--- a/tools/tensorflow_docs/api_generator/doc_generator_visitor.py
+++ b/tools/tensorflow_docs/api_generator/doc_generator_visitor.py
@@ -421,6 +421,9 @@ def build(self):
duplicates = {}
for path, node in self.path_tree.items():
+ _LOGGER.debug('DocGeneratorVisitor.build')
+ _LOGGER.debug(' path: %s', path)
+
if not path:
continue
full_name = node.full_name
@@ -593,7 +596,7 @@ def _get_physical_path(self, py_object):
@classmethod
def from_path_tree(cls, path_tree: PathTree, score_name_fn) -> ApiTree:
- """Create an ApiTree from an PathTree.
+ """Create an ApiTree from a PathTree.
Args:
path_tree: The `PathTree` to convert.
diff --git a/tools/tensorflow_docs/api_generator/generate_lib.py b/tools/tensorflow_docs/api_generator/generate_lib.py
index cb0e3916927..fdeb0f60601 100644
--- a/tools/tensorflow_docs/api_generator/generate_lib.py
+++ b/tools/tensorflow_docs/api_generator/generate_lib.py
@@ -15,11 +15,11 @@
"""Generate tensorflow.org style API Reference docs for a Python module."""
import collections
+import logging
import os
import pathlib
import shutil
import tempfile
-
from typing import Any, Optional, Sequence, Type, Union
from tensorflow_docs.api_generator import config
@@ -29,11 +29,8 @@
from tensorflow_docs.api_generator import reference_resolver as reference_resolver_lib
from tensorflow_docs.api_generator import toc as toc_lib
from tensorflow_docs.api_generator import traverse
-
from tensorflow_docs.api_generator.pretty_docs import docs_for_object
-
from tensorflow_docs.api_generator.report import utils
-
import yaml
# Used to add a collections.OrderedDict representer to yaml so that the
@@ -42,6 +39,9 @@
# Using a normal dict doesn't preserve the order of the input dictionary.
_mapping_tag = yaml.resolver.BaseResolver.DEFAULT_MAPPING_TAG
+# To see the logs pass: --logger_levels=tensorflow_docs:DEBUG --alsologtostderr
+_LOGGER = logging.getLogger(__name__)
+
def dict_representer(dumper, data):
return dumper.represent_dict(data.items())
@@ -121,6 +121,9 @@ def write_docs(
# Parse and write Markdown pages, resolving cross-links (`tf.symbol`).
num_docs_output = 0
for api_node in parser_config.api_tree.iter_nodes():
+ _LOGGER.debug('generate_lib.write_docs')
+ _LOGGER.debug(' full_name: %s', api_node.full_name)
+
full_name = api_node.full_name
if api_node.output_type() is api_node.OutputType.FRAGMENT:
@@ -391,7 +394,6 @@ def make_default_filters(self) -> list[public_api.ApiFilter]:
public_api.FailIfNestedTooDeep(10),
public_api.filter_module_all,
public_api.add_proto_fields,
- public_api.filter_builtin_modules,
public_api.filter_private_symbols,
public_api.FilterBaseDirs(self._base_dir),
public_api.FilterPrivateMap(self._private_map),
diff --git a/tools/tensorflow_docs/api_generator/parser.py b/tools/tensorflow_docs/api_generator/parser.py
index b8f906bffd7..f3d087bc6fc 100644
--- a/tools/tensorflow_docs/api_generator/parser.py
+++ b/tools/tensorflow_docs/api_generator/parser.py
@@ -92,15 +92,20 @@ def _get_raw_docstring(py_object):
obj_type = obj_type_lib.ObjType.get(py_object)
if obj_type is obj_type_lib.ObjType.TYPE_ALIAS:
- if inspect.getdoc(py_object) != inspect.getdoc(py_object.__origin__):
- result = inspect.getdoc(py_object)
- else:
+ result = inspect.getdoc(py_object)
+ if result == inspect.getdoc(py_object.__origin__):
result = ''
elif obj_type is obj_type_lib.ObjType.CLASS:
if dataclasses.is_dataclass(py_object):
result = _get_dataclass_docstring(py_object)
else:
result = inspect.getdoc(py_object) or ''
+ if (
+ result == inspect.getdoc(dict)
+ or result == inspect.getdoc(list)
+ or result == inspect.getdoc(tuple)
+ ):
+ result = ''
elif obj_type is obj_type_lib.ObjType.OTHER:
result = ''
else:
diff --git a/tools/tensorflow_docs/api_generator/parser_test.py b/tools/tensorflow_docs/api_generator/parser_test.py
index ee8a55f707f..0bfffeded92 100644
--- a/tools/tensorflow_docs/api_generator/parser_test.py
+++ b/tools/tensorflow_docs/api_generator/parser_test.py
@@ -799,7 +799,7 @@ class A():
self.assertEqual('Instance of `m.A`', result)
- def testIsClasssAttr(self):
+ def testIsClassAttr(self):
result = parser.is_class_attr('test_module.test_function',
{'test_module': test_module})
self.assertFalse(result)
@@ -808,6 +808,7 @@ def testIsClasssAttr(self):
{'TestClass': TestClass})
self.assertTrue(result)
+
RELU_DOC = """Computes rectified linear: `max(features, 0)`
RELU is an activation
diff --git a/tools/tensorflow_docs/api_generator/public_api.py b/tools/tensorflow_docs/api_generator/public_api.py
index c9803ee04e3..e6a994bff5b 100644
--- a/tools/tensorflow_docs/api_generator/public_api.py
+++ b/tools/tensorflow_docs/api_generator/public_api.py
@@ -489,27 +489,3 @@ def add_proto_fields(path: Sequence[str], parent: Any,
children = sorted(children.items(), key=lambda item: item[0])
return children
-
-
-def filter_builtin_modules(path: Sequence[str], parent: Any,
- children: Children) -> Children:
- """Filters module children to remove builtin modules.
-
- Args:
- path: API to this symbol
- parent: The object
- children: A list of (name, object) pairs.
-
- Returns:
- `children` with all builtin modules removed.
- """
- del path
- del parent
- # filter out 'builtin' modules
- filtered_children = []
- for name, child in children:
- # Do not descend into built-in modules
- if inspect.ismodule(child) and child.__name__ in sys.builtin_module_names:
- continue
- filtered_children.append((name, child))
- return filtered_children
diff --git a/tools/tensorflow_docs/api_generator/signature.py b/tools/tensorflow_docs/api_generator/signature.py
index 7ef8f1f856d..dacf5d2bada 100644
--- a/tools/tensorflow_docs/api_generator/signature.py
+++ b/tools/tensorflow_docs/api_generator/signature.py
@@ -580,7 +580,7 @@ def generate_signature(
sig = sig.replace(parameters=params)
- if dataclasses.is_dataclass(func):
+ if dataclasses.is_dataclass(func) and inspect.isclass(func):
sig = sig.replace(return_annotation=EMPTY)
extract_fn = _extract_class_defaults_and_annotations
else:
diff --git a/tools/tensorflow_docs/api_generator/toc.py b/tools/tensorflow_docs/api_generator/toc.py
index 1e72bcda75c..feaa15b8bda 100644
--- a/tools/tensorflow_docs/api_generator/toc.py
+++ b/tools/tensorflow_docs/api_generator/toc.py
@@ -273,7 +273,7 @@ def _is_deprecated(self, api_node: doc_generator_visitor.ApiTreeNode):
api_node: The node to evaluate.
Returns:
- True if depreacted else False.
+ True if deprecated else False.
"""
if doc_controls.is_deprecated(api_node.py_object):
return True
diff --git a/tools/tensorflow_docs/tools/nbfmt/__main__.py b/tools/tensorflow_docs/tools/nbfmt/__main__.py
index 9426e6fd690..b806d093a25 100644
--- a/tools/tensorflow_docs/tools/nbfmt/__main__.py
+++ b/tools/tensorflow_docs/tools/nbfmt/__main__.py
@@ -99,16 +99,17 @@ def clean_root(data: Dict[str, Any], filepath: pathlib.Path) -> None:
data, keep=["cells", "metadata", "nbformat_minor", "nbformat"])
# All metadata is optional according to spec, but we use some of it.
notebook_utils.del_entries_except(
- data["metadata"], keep=["accelerator", "colab", "kernelspec"])
+ data["metadata"], keep=["accelerator", "colab", "kernelspec", "google"]
+ )
metadata = data.get("metadata", {})
- colab = metadata.get("colab", {})
# Set top-level notebook defaults.
data["nbformat"] = 4
data["nbformat_minor"] = 0
# Colab metadata
+ colab = metadata.get("colab", {})
notebook_utils.del_entries_except(
colab, keep=["collapsed_sections", "name", "toc_visible"])
colab["name"] = os.path.basename(filepath)
@@ -128,6 +129,15 @@ def clean_root(data: Dict[str, Any], filepath: pathlib.Path) -> None:
kernelspec["display_name"] = supported_kernels[kernel_name]
metadata["kernelspec"] = kernelspec
+ # Google metadata
+ google = metadata.get("google", {})
+ notebook_utils.del_entries_except(google, keep=["keywords", "image_path"])
+ # Don't add the field if it's empty.
+ if google:
+ metadata["google"] = google
+ else:
+ metadata.pop("google", None)
+
data["metadata"] = metadata
@@ -227,7 +237,7 @@ def update_license_cells(data: Dict[str, Any]) -> None:
data: object representing a parsed JSON notebook.
"""
# This pattern in Apache and MIT license boilerplate.
- license_re = re.compile(r"#@title.*License")
+ license_re = re.compile(r"#\s?@title.*License")
for idx, cell in enumerate(data["cells"]):
src_text = "".join(cell["source"])
diff --git a/tools/tensorflow_docs/tools/nbfmt/nbfmtmain_test.py b/tools/tensorflow_docs/tools/nbfmt/nbfmtmain_test.py
new file mode 100644
index 00000000000..5f07c103cab
--- /dev/null
+++ b/tools/tensorflow_docs/tools/nbfmt/nbfmtmain_test.py
@@ -0,0 +1,74 @@
+# Copyright 2024 The TensorFlow Authors. All Rights Reserved.
+#
+# Licensed under the Apache License, Version 2.0 (the "License");
+# you may not use this file except in compliance with the License.
+# You may obtain a copy of the License at
+#
+# http://www.apache.org/licenses/LICENSE-2.0
+#
+# Unless required by applicable law or agreed to in writing, software
+# distributed under the License is distributed on an "AS IS" BASIS,
+# WITHOUT WARRANTIES OR CONDITIONS OF ANY KIND, either express or implied.
+# See the License for the specific language governing permissions and
+# limitations under the License.
+"""Unit tests for nbfmt."""
+import pathlib
+import unittest
+from nbformat import notebooknode
+from tensorflow_docs.tools.nbfmt import __main__ as nbfmt
+
+
+class NotebookFormatTest(unittest.TestCase):
+
+ def test_metadata_cleansing(self):
+ subject_notebook = notebooknode.NotebookNode({
+ "cells": [],
+ "metadata": {
+ "unknown": ["delete", "me"],
+ "accelerator": "GPU",
+ "colab": {
+ "name": "/this/is/clobbered.ipynb",
+ "collapsed_sections": [],
+ "deleteme": "pls",
+ },
+ "kernelspec": {
+ "display_name": "Python 2 foreverrrr",
+ "name": "python2",
+ "deleteme": "deldeldel",
+ },
+ "google": {
+ "keywords": ["one", "two"],
+ "image_path": "/foo/img.png",
+ "more_stuff": "delete me",
+ },
+ },
+ })
+
+ expected_notebook = notebooknode.NotebookNode({
+ "cells": [],
+ "metadata": {
+ "accelerator": "GPU",
+ "colab": {
+ "name": "test.ipynb",
+ "collapsed_sections": [],
+ "toc_visible": True,
+ },
+ "kernelspec": {
+ "display_name": "Python 3",
+ "name": "python3",
+ },
+ "google": {
+ "keywords": ["one", "two"],
+ "image_path": "/foo/img.png",
+ },
+ },
+ "nbformat": 4,
+ "nbformat_minor": 0,
+ })
+
+ nbfmt.clean_root(subject_notebook, pathlib.Path("/path/test.ipynb"))
+ self.assertEqual(subject_notebook, expected_notebook)
+
+
+if __name__ == "__main__":
+ unittest.main()
diff --git a/tools/tensorflow_docs/tools/nblint/decorator.py b/tools/tensorflow_docs/tools/nblint/decorator.py
index 408fef3d969..d74045c7ca7 100644
--- a/tools/tensorflow_docs/tools/nblint/decorator.py
+++ b/tools/tensorflow_docs/tools/nblint/decorator.py
@@ -161,7 +161,7 @@ def fail(message: Optional[str] = None,
Failure messages come in two flavors:
- conditional: (Default) While this test may fail here, it may succeed
- elsewhere, and thus, the larger condition passes and do not dislay this
+ elsewhere, and thus, the larger condition passes and do not display this
message.
- non-conditional (always show): Regardless if the larger condition is met,
display this error message in the status report. For example, a
diff --git a/tools/tensorflow_docs/tools/nblint/style/tensorflow.py b/tools/tensorflow_docs/tools/nblint/style/tensorflow.py
index f6ca2381a54..49fc9dc1025 100644
--- a/tools/tensorflow_docs/tools/nblint/style/tensorflow.py
+++ b/tools/tensorflow_docs/tools/nblint/style/tensorflow.py
@@ -56,7 +56,7 @@ def copyright_check(args):
return any(re.search(pattern, cell_source) for pattern in copyrights_re)
-license_re = re.compile("#@title Licensed under the Apache License")
+license_re = re.compile("#\s?@title Licensed under the Apache License")
@lint(
@@ -81,7 +81,11 @@ def not_translation(args):
# Button checks
-is_button_cell_re = re.compile(r"class.*tfo-notebook-buttons")
+# Look for class="tfo-notebook-buttons" (CSS used on website versions) or the
+# run-in-colab logo (for notebooks that stick to GitHub/Colab).
+is_button_cell_re = re.compile(
+ r"class.*tfo-notebook-buttons|colab_logo_32px\.png"
+)
def get_arg_or_fail(user_args, arg_name, arg_fmt):
diff --git a/tools/tensorflow_docs/vis/webp_animation.py b/tools/tensorflow_docs/vis/webp_animation.py
deleted file mode 100644
index ae6a8713d4f..00000000000
--- a/tools/tensorflow_docs/vis/webp_animation.py
+++ /dev/null
@@ -1,156 +0,0 @@
-# Copyright 2015 The TensorFlow Authors. All Rights Reserved.
-#
-# Licensed under the Apache License, Version 2.0 (the "License");
-# you may not use this file except in compliance with the License.
-# You may obtain a copy of the License at
-#
-# http://www.apache.org/licenses/LICENSE-2.0
-#
-# Unless required by applicable law or agreed to in writing, software
-# distributed under the License is distributed on an "AS IS" BASIS,
-# WITHOUT WARRANTIES OR CONDITIONS OF ANY KIND, either express or implied.
-# See the License for the specific language governing permissions and
-# limitations under the License.
-# ==============================================================================
-"""Easy notebook embedded webp animations.
-
-```
-import tensorflow_docs.vis.webp_animation as webp_animation
-
-env = gym.make('SpaceInvaders-v0')
-obs = env.reset()
-done = False
-n = 0
-
-anim = webp_animation.Webp()
-
-while not done:
- img = env.render(mode = 'rgb_array')
- anim.append(img)
- act = env.action_space.sample() # take a random action
- obs, reward, done, info = env.step(act)
- n += 1
-
-anim.save("test.webp")
-anim
-```
-"""
-
-import numpy as np
-import PIL.Image
-
-from tensorflow_docs.vis import embed
-import webp
-
-
-class Webp(object):
- """Builds a webp animation.
-
- Attributes:
- frame_rate: The default frame rate for appended images.
- shape: The shape of the animation frames. Will default to the size of the
- first image if not set.
- result: The binary image data string. Once the animation has been used, it
- can no longer updated. And the result field contains the webp encoded
- data.
- """
-
- def __init__(self, shape=None, frame_rate=60.0, **options):
- """A notebook-embedable webp animation.
-
- Args:
- shape: Optional. The image_shape of the animation. Defaults to the shape
- of the first image if unset.
- frame_rate: The default frame rate for the animation.
- **options: Additional arguments passed to `WebPAnimEncoderOptions.new`.
- """
- self.frame_rate = frame_rate
- self._timestamp_ms = 0
- self._empty = True
-
- if options is None:
- options = {}
-
- self._options = webp.WebPAnimEncoderOptions.new(**options)
- self._encoder = None
- self._shape = shape
- self._result = None
-
- def append(self, img, dt_ms=None):
- """Append an image to the animation.
-
- Args:
- img: The image to add.
- dt_ms: override the animation frame rate for this frame with a frame
- length in ms.
-
- Raises:
- ValueError:
- * if the video has already been "assembled" (used).
- * if `img` does not match the shape of the animation.
- """
- if self._result is not None:
- raise ValueError(
- "Can't append to an animation after it has been \"assembled\" (used)."
- )
- self._empty = False
-
- if not isinstance(img, PIL.Image.Image):
- img = np.asarray(img)
- img = PIL.Image.fromarray(img)
-
- if self._shape is None:
- self._shape = img.size
-
- if self._encoder is None:
- self._encoder = webp.WebPAnimEncoder.new(self.shape[0], self.shape[1],
- self._options)
-
- if img.size != self.shape:
- raise ValueError("Image shape does not match video shape")
-
- img = webp.WebPPicture.from_pil(img)
-
- self._encoder.encode_frame(img, int(self._timestamp_ms))
-
- if dt_ms is None:
- self._timestamp_ms += 1000 * (1.0 / self.frame_rate)
- else:
- self._timestamp_ms += dt_ms
-
- def extend(self, imgs, dt_ms=None):
- """Extend tha animation with an iterable if images.
-
- Args:
- imgs: An iterable of images, to pass to `.append`.
- dt_ms: Override the animation frame rate for these frames with a frame
- length in ms.
- """
- for img in imgs:
- self.append(img, dt_ms=dt_ms)
-
- @property
- def result(self):
- result = self._result
- if result is None:
- anim_data = self._encoder.assemble(int(self._timestamp_ms))
- result = anim_data.buffer()
- self._result = result
- return result
-
- @property
- def shape(self):
- """The shape of the animation. Read only once set."""
- return self._shape
-
- def _repr_html_(self):
- """Notebook display hook, embed the image in an tag."""
- if self._empty:
- return "Empty Animation"
-
- return embed.embed_data("image/webp", self.result)._repr_html_() # pylint: disable=protected-access,
-
- def save(self, filename):
- """Write the webp data to a file."""
- with open(filename, "wb") as f:
- f.write(self.result)
diff --git a/tools/tensorflow_docs/vis/webp_test.py b/tools/tensorflow_docs/vis/webp_test.py
deleted file mode 100644
index 0bc1dd28aed..00000000000
--- a/tools/tensorflow_docs/vis/webp_test.py
+++ /dev/null
@@ -1,41 +0,0 @@
-# Copyright 2015 The TensorFlow Authors. All Rights Reserved.
-#
-# Licensed under the Apache License, Version 2.0 (the "License");
-# you may not use this file except in compliance with the License.
-# You may obtain a copy of the License at
-#
-# http://www.apache.org/licenses/LICENSE-2.0
-#
-# Unless required by applicable law or agreed to in writing, software
-# distributed under the License is distributed on an "AS IS" BASIS,
-# WITHOUT WARRANTIES OR CONDITIONS OF ANY KIND, either express or implied.
-# See the License for the specific language governing permissions and
-# limitations under the License.
-# ==============================================================================
-"""Tests for tensorflow_docs.vis.webp."""
-
-import os
-
-from absl.testing import absltest
-
-import numpy as np
-import PIL.Image
-
-from tensorflow_docs.vis import webp_animation
-
-
-class WebpTest(absltest.TestCase):
-
- def test_smoke(self):
- workdir = self.create_tempdir().full_path
-
- img = PIL.Image.fromarray(np.zeros([10, 12, 3], dtype=np.uint8))
- anim = webp_animation.Webp()
-
- anim.append(img)
- anim.extend([img])
- anim.save(os.path.join(workdir, 'test.webp'))
-
-
-if __name__ == '__main__':
- absltest.main()